Data Quality Explained
Summary
TLDRThis video script discusses the pivotal role of data quality in business outcomes, using the analogy of a chef with poor ingredients. It emphasizes four key data qualities: accuracy, completeness, consistency, and uniqueness, explaining each with examples from a lead generation company. The script concludes by suggesting the use of machine learning and AI to automate the detection of these qualities, thereby saving time and reducing manual data inspection.
Takeaways
- 🍽️ Data quality is crucial for business outcomes, similar to how quality ingredients are essential for a chef's dishes.
- 📉 Poor data quality can negatively impact a company's reputation, just as bad ingredients can ruin a restaurant's reputation.
- 🔍 Four main qualities of data include accuracy, completeness, consistency, and uniqueness.
- 🎯 Accuracy refers to how well the data reflects the true state of reality, unaffected by anomalies like bot traffic.
- 📝 Completeness is about ensuring all required fields in a dataset are filled out, providing a full picture of the data.
- 🔄 Consistency is about the uniformity of data across different sources to avoid mismatches that can lead to incomplete customer profiles.
- 🌀 Uniqueness is tied to the absence of duplicates in a dataset, which can inflate the perceived volume of data.
- 🤖 Machine learning and AI can be used to automatically detect these data qualities as they enter the system, reducing manual effort.
- 🔗 The script suggests leveraging technology to automate the inspection of data quality, which can save time and improve efficiency.
- 👨💻 The speaker invites viewers to explore more about these features and subscribe to the channel for more insights on technology.
Q & A
What is the main impact of poor data quality on a business?
-Poor data quality can significantly affect business outcomes, causing a company's reputation to suffer, similar to how poor quality ingredients can ruin a chef's dishes and harm a restaurant's reputation.
What are the four main qualities of data that affect its quality?
-The four main qualities of data that affect its quality are accuracy, completeness, consistency, and uniqueness.
How is accuracy in data defined in the context of the script?
-Accuracy in data refers to how well the current state of the data matches reality. For example, if a lead generation company does not account for a spike in bot-generated traffic, the data will not accurately reflect reality.
What does completeness in data mean and why is it important?
-Completeness in data means that all required fields in a dataset are filled out. It is important because incomplete data can lead to an incomplete picture of customers or clients, which can affect business decisions.
Can you explain the concept of consistency in data as mentioned in the script?
-Consistency in data refers to the uniformity of the data set across different sources. If different teams within a company collect the same data in different formats, it can lead to mismatches and an incomplete customer profile when the data is pulled from various systems.
What is uniqueness in data, and how can it affect a lead generation company?
-Uniqueness in data pertains to the absence of duplicate entries within a dataset. For a lead generation company, having a high percentage of duplicate leads can result in an inflated lead count, which can misrepresent the actual number of unique prospects and potentially skew business performance metrics.
How can machine learning and AI help in managing data quality?
-Machine learning and AI can be leveraged to automatically detect and manage key data quality features such as accuracy, completeness, consistency, and uniqueness as data enters the system, which saves time and reduces the need for manual inspection.
What is the analogy used in the script to explain the importance of data quality?
-The analogy used in the script compares a chef with poor quality ingredients to a business with poor data quality, emphasizing that both can lead to a poor end product and damage to reputation.
Why is it crucial for a lead generation company to account for bot-generated traffic?
-It is crucial for a lead generation company to account for bot-generated traffic to ensure that the data reflects actual human users and not automated bots, as this can lead to inaccurate data and misinformed business decisions.
How can the lack of required fields in a survey campaign affect the data collected?
-If a survey campaign does not require certain fields to be filled out, it can result in a dataset with missing information, leading to an incomplete understanding of the respondents and potentially biased or skewed results.
What challenges does a company face when different teams collect the same data in different formats?
-When different teams collect the same data in different formats, it can lead to inconsistencies and difficulties in integrating the data. This can result in an incomplete or inaccurate customer profile and hinder the effectiveness of data-driven decision-making.
Outlines
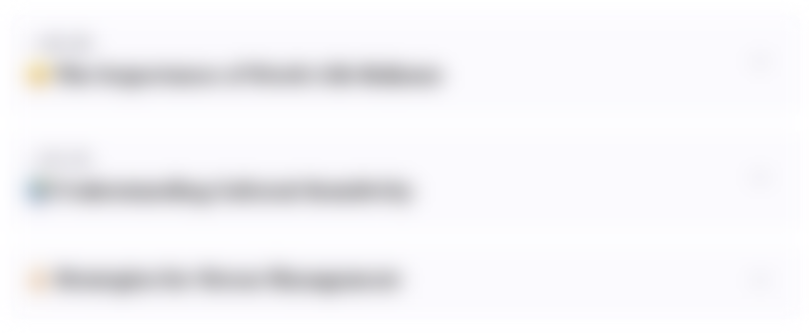
Esta sección está disponible solo para usuarios con suscripción. Por favor, mejora tu plan para acceder a esta parte.
Mejorar ahoraMindmap
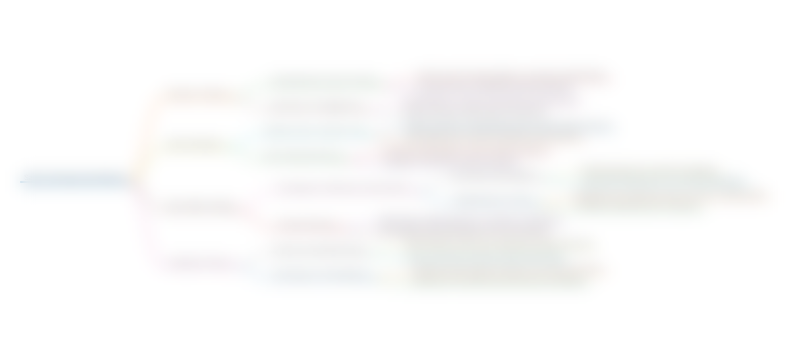
Esta sección está disponible solo para usuarios con suscripción. Por favor, mejora tu plan para acceder a esta parte.
Mejorar ahoraKeywords
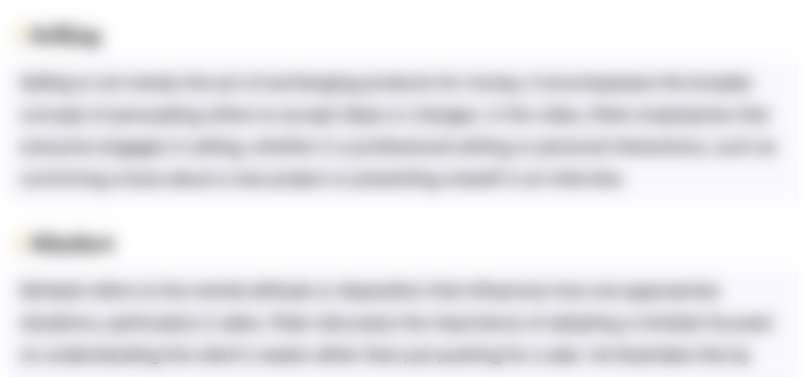
Esta sección está disponible solo para usuarios con suscripción. Por favor, mejora tu plan para acceder a esta parte.
Mejorar ahoraHighlights
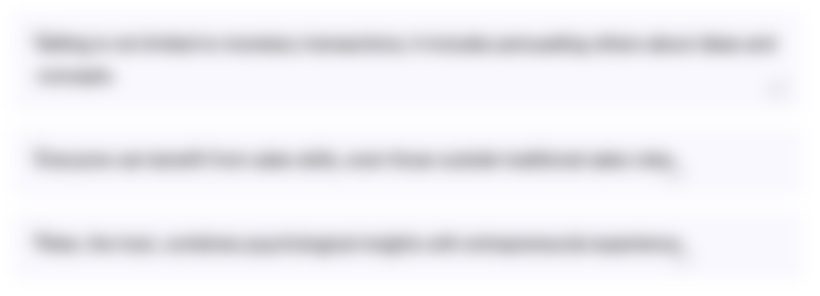
Esta sección está disponible solo para usuarios con suscripción. Por favor, mejora tu plan para acceder a esta parte.
Mejorar ahoraTranscripts
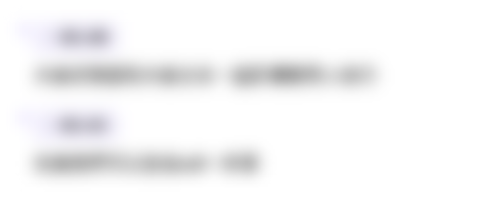
Esta sección está disponible solo para usuarios con suscripción. Por favor, mejora tu plan para acceder a esta parte.
Mejorar ahoraVer Más Videos Relacionados
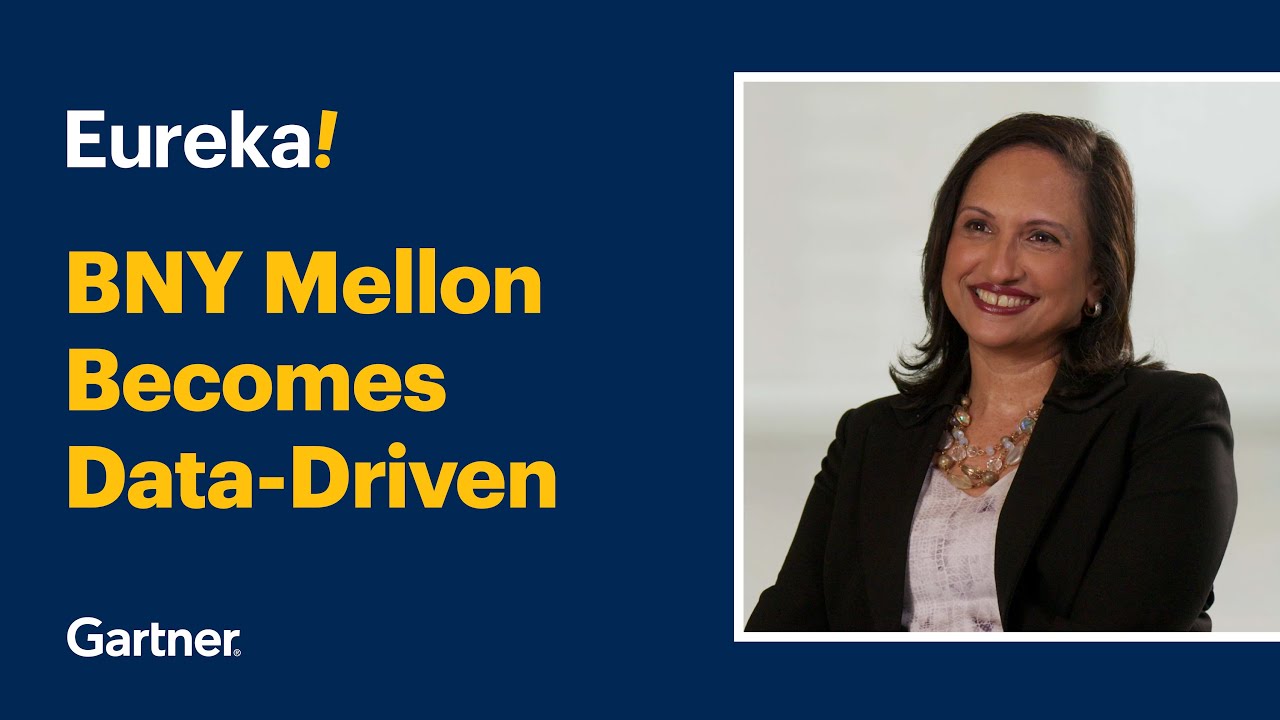
How BNY Mellon Became a Data-Driven Organization | Eureka!

Resep Cuan Jualan Rempeyek Online
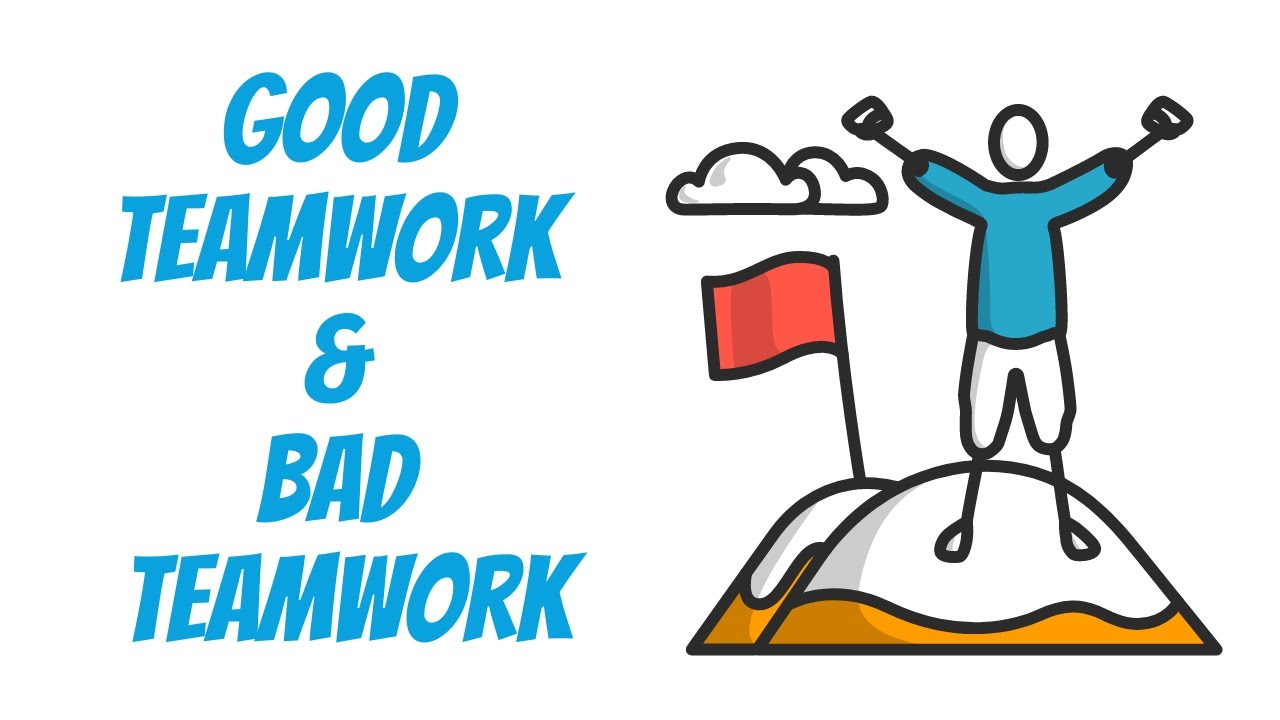
Good Teamwork and Bad Teamwork - Tips for Effective Teamwork

Pedoman Perencanaan Pengawasan Berbasis Risiko (PPBR)
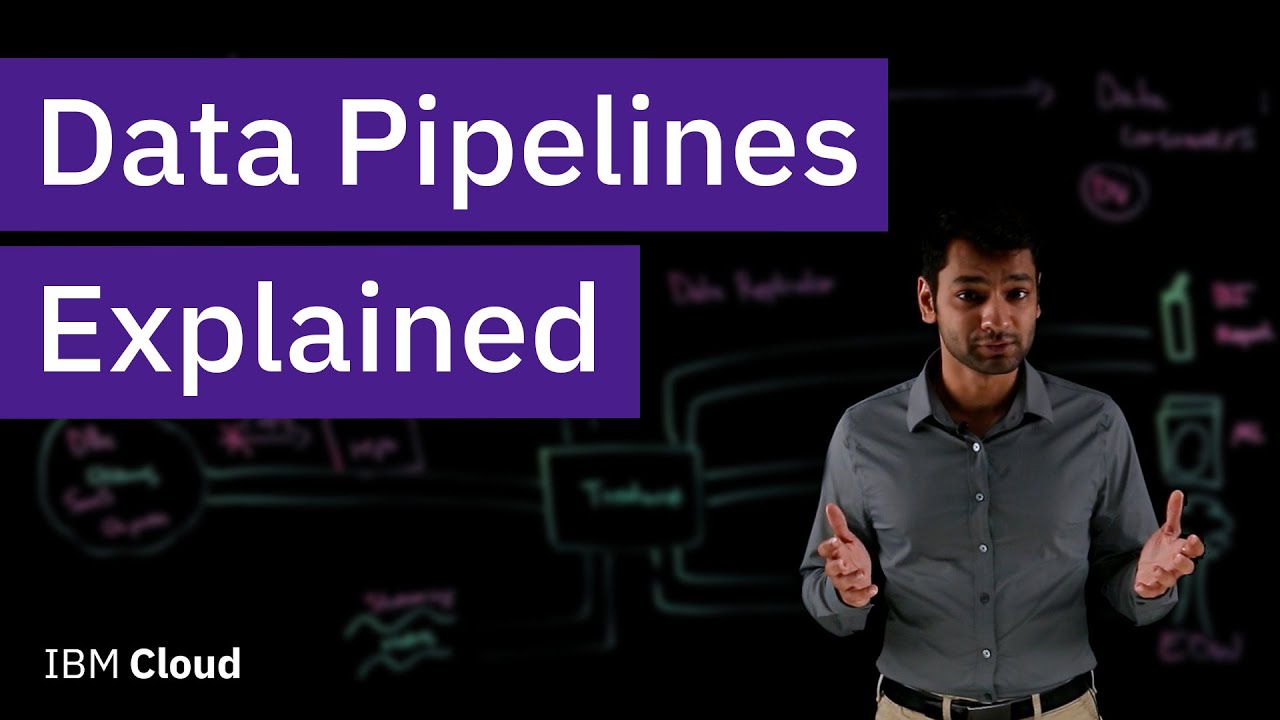
Data Pipelines Explained
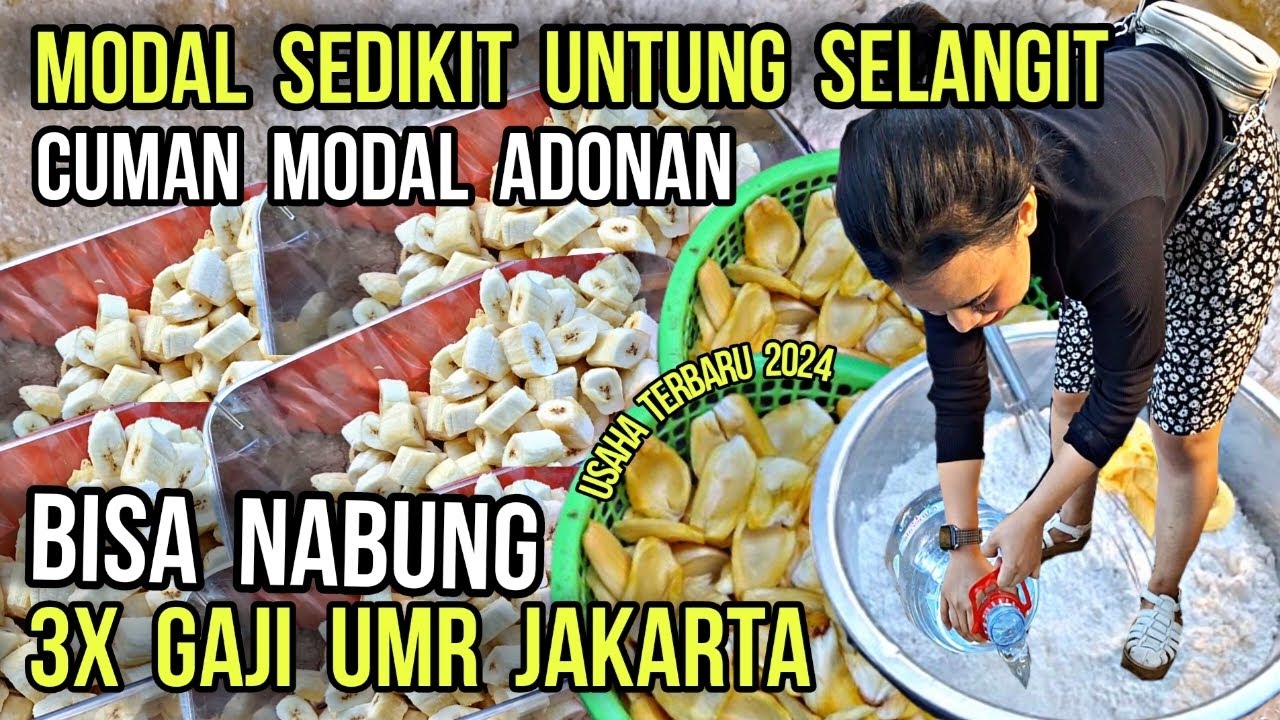
IDE USAHA TERBARU 2024 !! CUMAN MODAL ADONAN BISA NABUNG 3X GAJI UMR JAKARTA/ BULAN
5.0 / 5 (0 votes)