Neural Network Python Project - Handwritten Digit Recognition
Summary
TLDRIn this educational video, the host guides viewers through building a neural network using Python to recognize handwritten digits. They utilize the MNIST dataset from TensorFlow Keras, demonstrating how to preprocess the data, construct a neural network model with layers, and train it. The video also covers model evaluation and prediction using custom digit images. Despite a few misclassifications, the model achieves a high accuracy rate, offering a solid introduction to neural networks for beginners.
Takeaways
- 🌟 The video tutorial focuses on building a neural network using Python to recognize handwritten digits.
- 📚 The tutorial is a remake of an older video to provide a modern approach to a classic machine learning project.
- 📈 The dataset used for training the neural network is the MNIST dataset, which includes 28x28 pixel images of handwritten digits.
- 🛠️ Key libraries required for the project are TensorFlow, Keras, NumPy, OpenCV, and Matplotlib.
- 🔢 The MNIST dataset is loaded directly from TensorFlow, simplifying the process by avoiding manual data handling from CSV files.
- 📊 Normalization of pixel values is crucial, scaling them down to a range between 0 and 1 to aid the neural network's learning process.
- 🧠 The neural network model is built using a sequential model with a combination of flattened and dense layers, culminating in an output layer with softmax activation for classification.
- 🔧 The model is trained using the training dataset and then evaluated on the test dataset to measure its accuracy and loss.
- 🎨 The tutorial includes a practical demonstration of creating and predicting custom handwritten digit images, showcasing the model's real-world application.
- 🔄 The video concludes with suggestions for improving model performance, such as increasing training epochs or using more complex neural network architectures like CNNs.
Q & A
What is the main topic of the video?
-The main topic of the video is building a neural network in Python that recognizes handwritten digits.
Why is the MNIST dataset used in the video?
-The MNIST dataset is used because it contains a large number of labeled 28x28 pixel grayscale images of handwritten digits, which is ideal for training a neural network to recognize such digits.
What libraries are required to build the neural network as described in the video?
-The libraries required are numpy, opencv-python, matplotlib, and tensorflow.
How is the MNIST dataset loaded in the video?
-The MNIST dataset is loaded directly from tensorflow.keras.datasets.mnist by calling the load_data function.
What is the purpose of normalizing the pixel data in the video?
-Normalizing the pixel data scales the values down to a range between 0 and 1, which makes it easier for the neural network to process and learn from the data.
What type of neural network model is created in the video?
-A sequential neural network model is created in the video, which is a basic type of neural network where layers are connected in a linear sequence.
What activation function is used in the output layer of the neural network?
-The softmax activation function is used in the output layer of the neural network to provide probabilities for each digit class.
How is the model trained in the video?
-The model is trained by calling the fit function with the training data (x_train and y_train) and specifying the number of epochs.
What is the purpose of evaluating the model with the test data?
-Evaluating the model with the test data is done to assess the model's performance on data it has not seen before, which helps determine its accuracy and effectiveness.
How are custom handwritten digits prepared and used to test the model?
-Custom handwritten digits are prepared by drawing them in a 28x28 pixel format, converting them to grayscale, inverting the colors, and then using the model's predict function to classify the digit.
What is the final accuracy of the model with the custom handwritten digits as shown in the video?
-The final accuracy of the model with the custom handwritten digits is approximately 91%, with one misclassification out of twelve test images.
Outlines
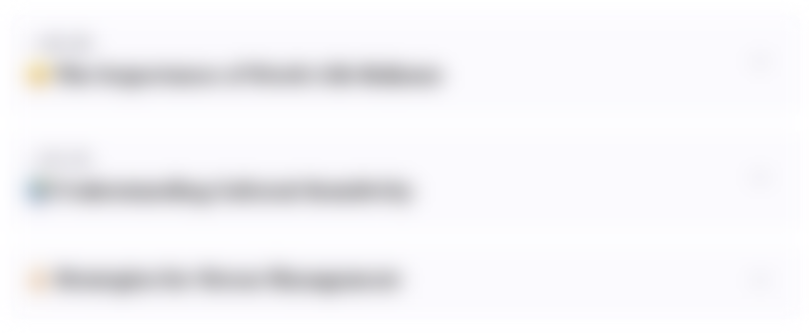
Esta sección está disponible solo para usuarios con suscripción. Por favor, mejora tu plan para acceder a esta parte.
Mejorar ahoraMindmap
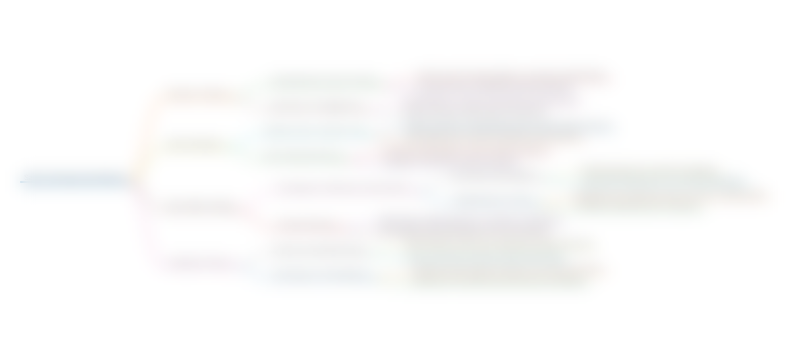
Esta sección está disponible solo para usuarios con suscripción. Por favor, mejora tu plan para acceder a esta parte.
Mejorar ahoraKeywords
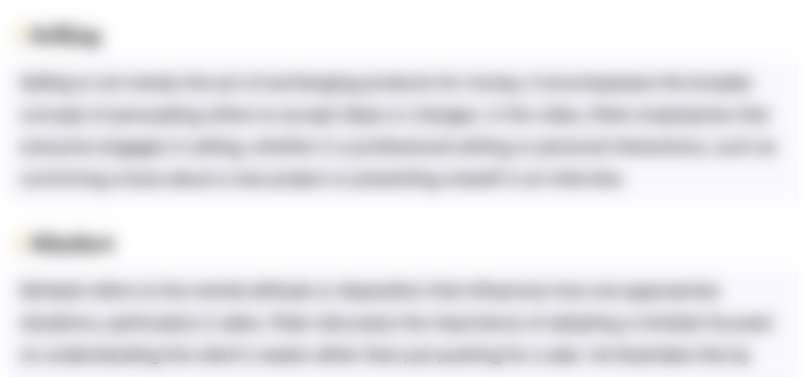
Esta sección está disponible solo para usuarios con suscripción. Por favor, mejora tu plan para acceder a esta parte.
Mejorar ahoraHighlights
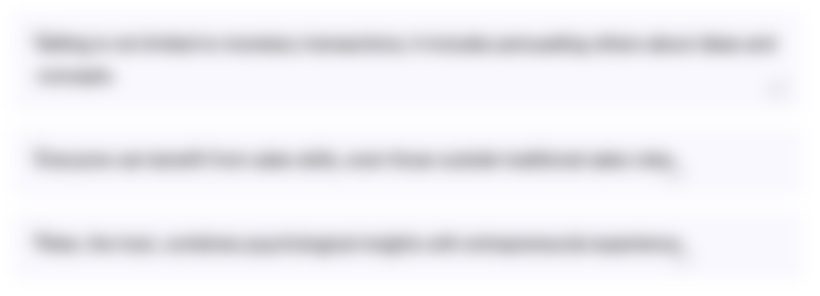
Esta sección está disponible solo para usuarios con suscripción. Por favor, mejora tu plan para acceder a esta parte.
Mejorar ahoraTranscripts
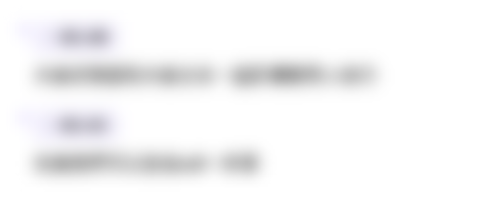
Esta sección está disponible solo para usuarios con suscripción. Por favor, mejora tu plan para acceder a esta parte.
Mejorar ahoraVer Más Videos Relacionados
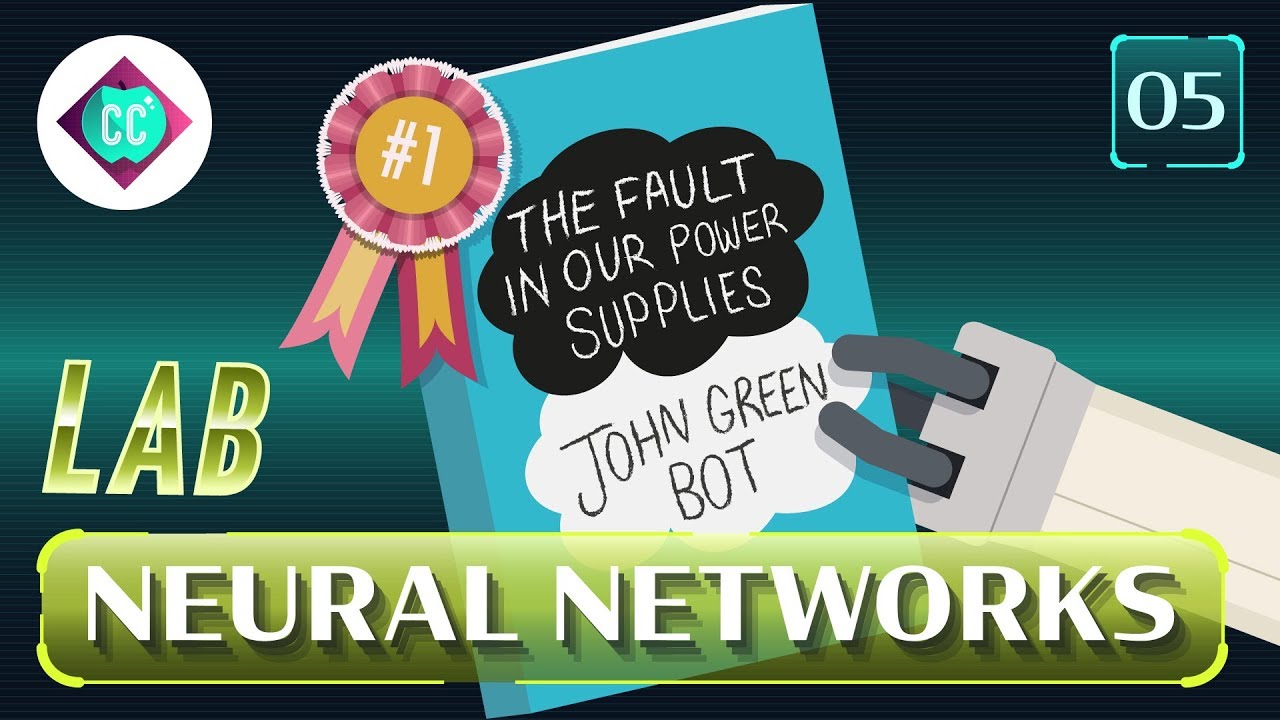
How to make an AI read your handwriting (LAB) : Crash Course Ai #5

Твоя ПЕРВАЯ НЕЙРОСЕТЬ на Python с нуля! | За 10 минут :3
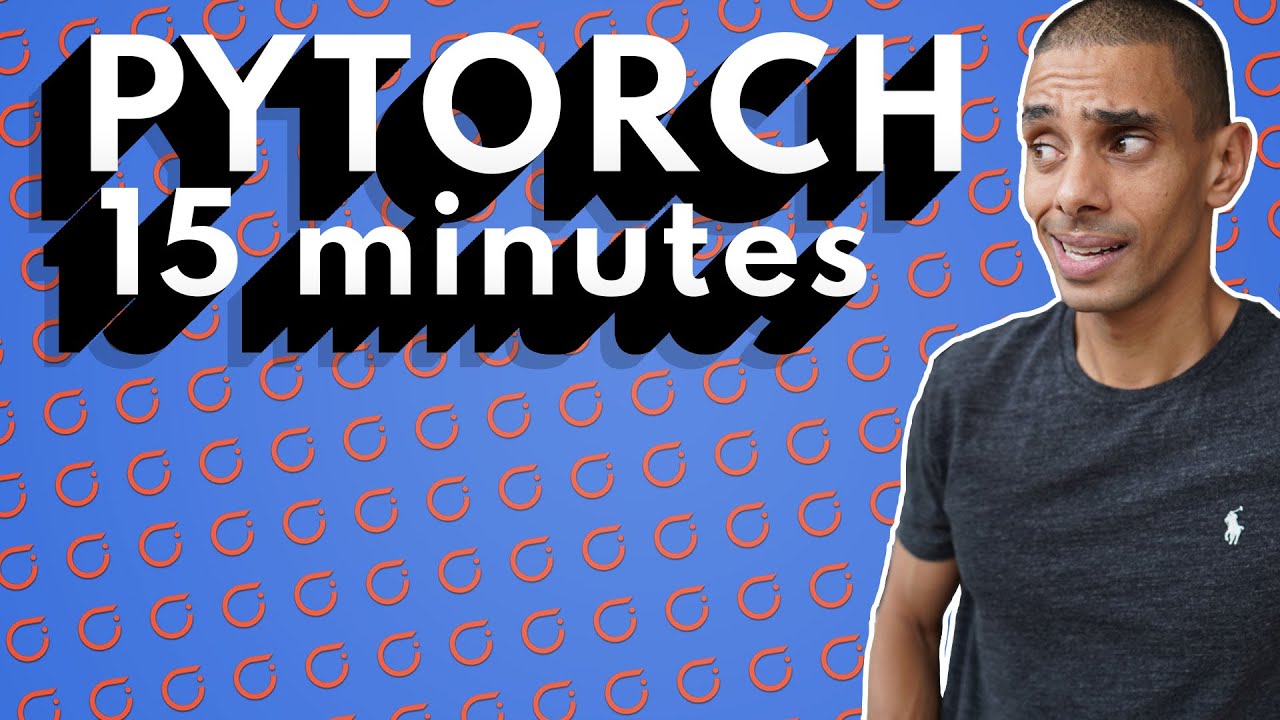
Building a Neural Network with PyTorch in 15 Minutes | Coding Challenge
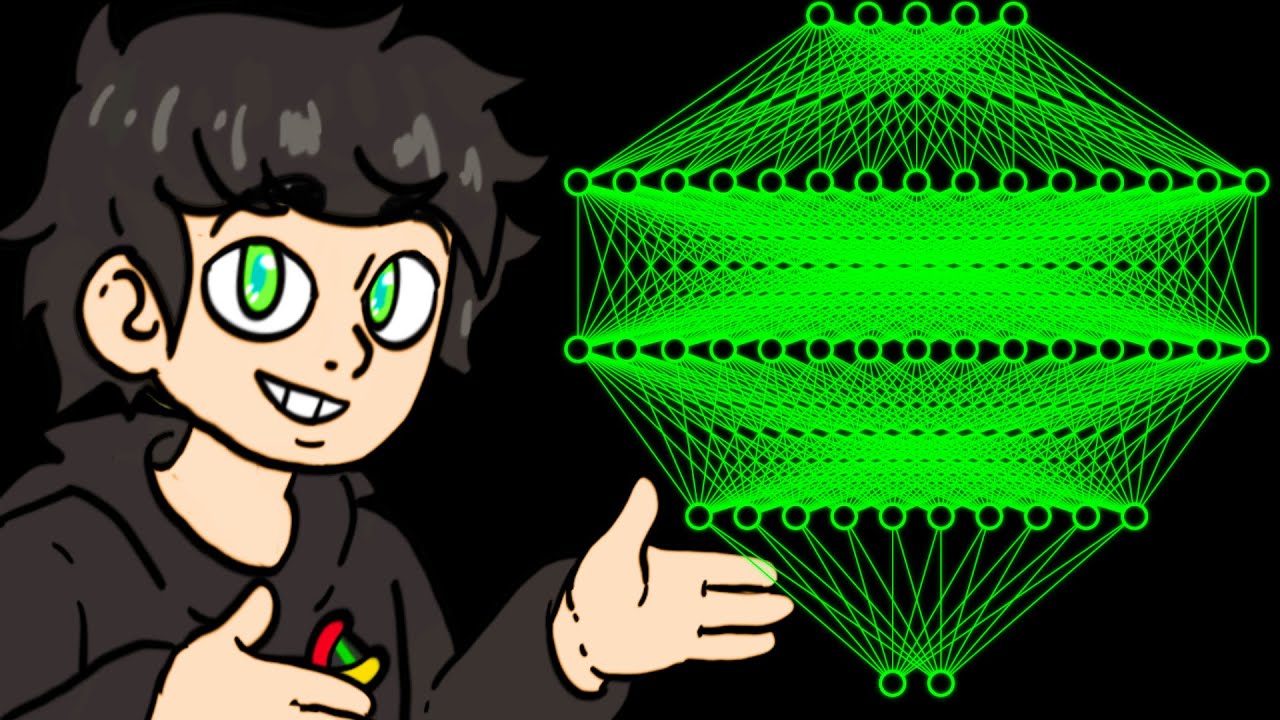
I Built a Neural Network from Scratch
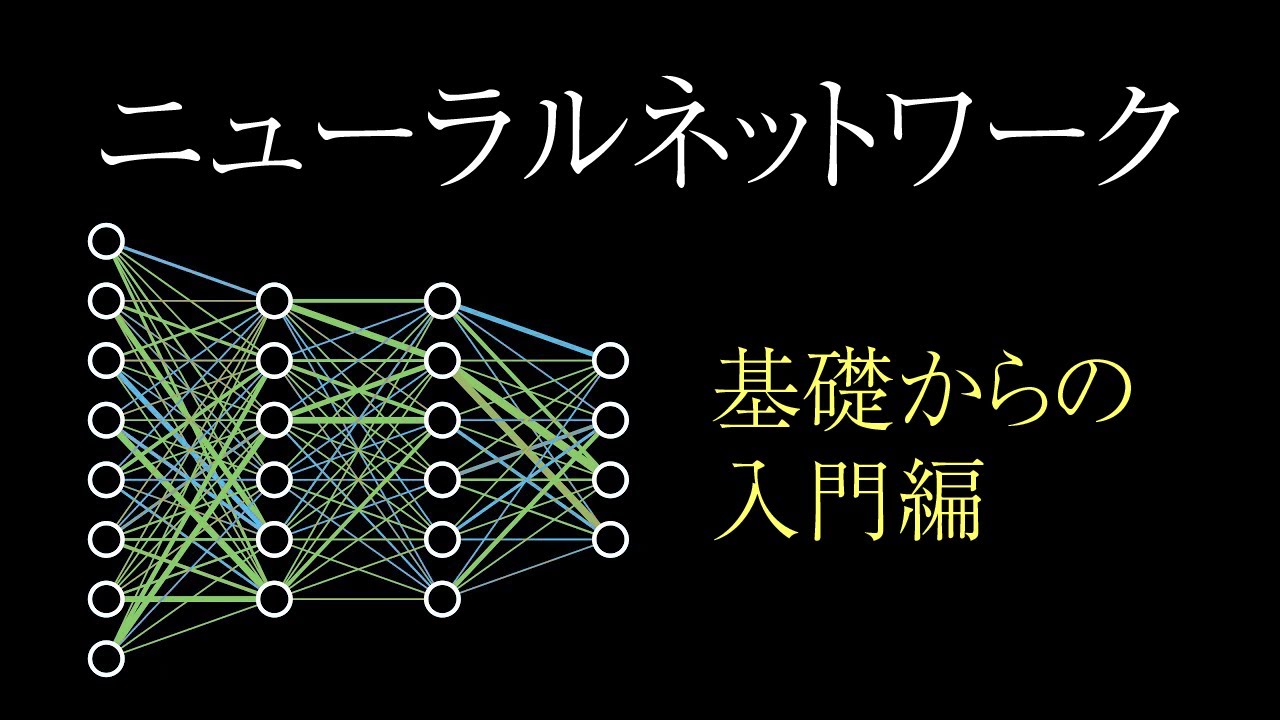
ニューラルネットワークの仕組み | Chapter 1, 深層学習(ディープラーニング)
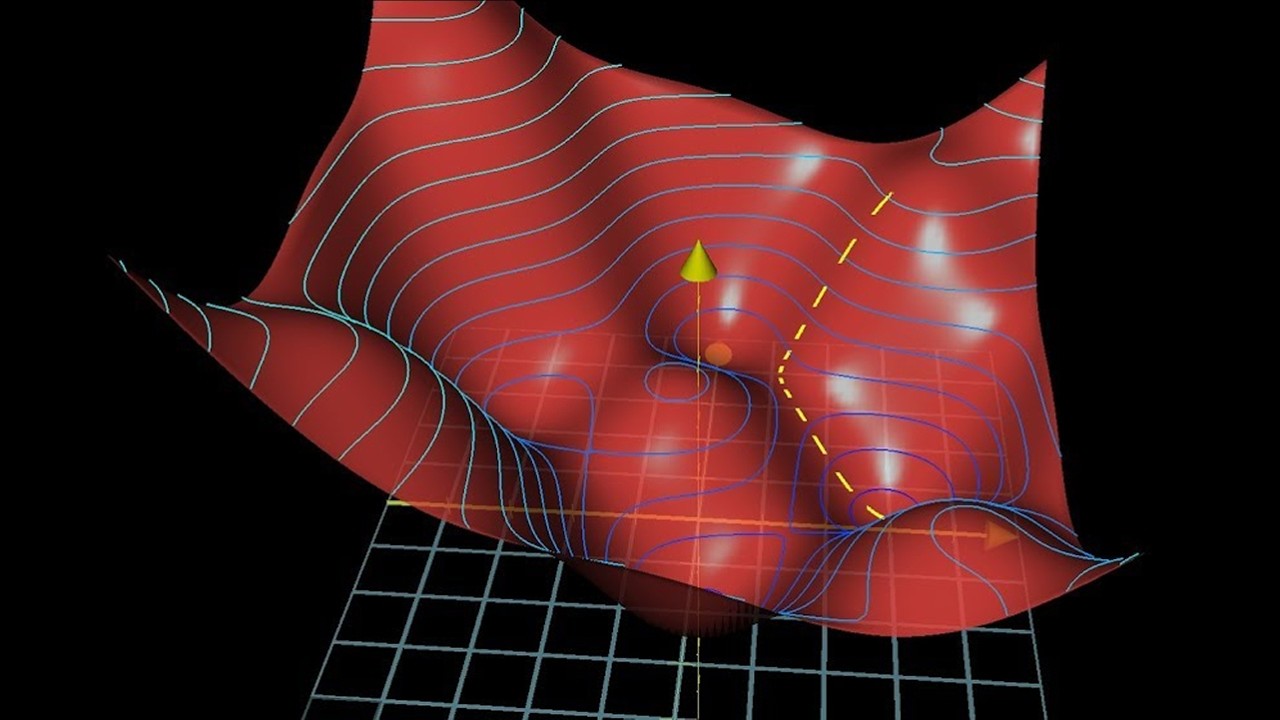
Gradient descent, how neural networks learn | Chapter 2, Deep learning
5.0 / 5 (0 votes)