You NEED to AVOID these Mistakes as a Data Analyst | raw truth
Summary
TLDRIn this video, Rohan discusses three common mistakes made by beginner data analysts and offers advice on how to avoid them. He emphasizes the importance of avoiding sampling bias by ensuring representative data sets, maintaining high data quality to prevent errors in analysis, and cautions against confusing correlation with causation. Rohan also stresses the need for critical thinking and proper data validation to become a high-quality data analyst.
Takeaways
- 😀 Most data analysts struggle not due to coding skills but because they neglect the analysis itself.
- 📊 Statistics is crucial and forms the backbone of data analysis and data science.
- 🚫 Avoiding sampling bias is essential; ensure the sample size is representative of the entire population.
- 🏥 Examples of sampling bias include political polling in urban areas or medical studies focusing only on hospital visitors.
- 💡 Proper sampling methods are critical for accuracy, reliability, and integrity in studies, and are more cost-effective.
- 📈 Poor data quality can severely impact analysis and subsequent business decisions.
- 🔍 Data analysts should validate data from multiple sources to ensure accuracy before conducting analysis.
- 🧼 Data cleaning is vital, but be cautious not to introduce bias through incorrect handling of missing values.
- 🔗 Understanding the difference between correlation and causation is fundamental; correlation does not imply causation.
- 🌡 Common examples used to illustrate this include ice cream sales and shark attacks, or chocolate consumption and Nobel Prizes.
- 🔍 As a data analyst, it's important to critically think and investigate potential confounding variables that may affect data interpretation.
Q & A
What is the main reason most data analysts are not great at their job according to the speaker?
-The speaker suggests that most data analysts are not great at their job not because of their coding skills with SQL, Python, R, or BI tools, but because they neglect the actual analysis, particularly the importance of statistics in data analysis.
What is sampling bias and how does it affect data analysis?
-Sampling bias occurs when a small sample size taken for analysis is not representative of the entire population. This can lead to inaccurate conclusions because the data collected may not reflect the broader population's characteristics, thus affecting the reliability of the analysis.
Why is it important to use proper sampling methods in data analysis?
-Proper sampling methods ensure accuracy, reliability, and integrity in a study. They are also more cost-effective than analyzing an entire population, making it feasible to conduct large-scale analyses without incurring excessive costs.
What is the second biggest mistake data analysts make according to the video?
-The second biggest mistake data analysts make is using poor data quality. This can be due to issues like data pipeline errors, inaccurate data entry, or unreliable data sources, which can significantly impact the accuracy of analysis and subsequent business decisions.
How can data analysts validate the data they are using for analysis?
-Data analysts can validate the data by comparing it with a second or third source, or even third-party data, to ensure consistency and accuracy. This due diligence helps in identifying discrepancies and ensuring the data used is reliable before conducting analysis.
Why is data cleaning important in the data analysis process?
-Data cleaning is crucial to ensure data quality. It involves handling missing values, correcting errors, and removing inconsistencies. However, improper data cleaning can introduce bias or incorrect values, so it should be done carefully with proper automation and testing.
What is the difference between correlation and causation as explained in the video?
-Correlation refers to the relationship between two or more variables, while causation implies that one variable causes the other to change. The video emphasizes that just because two variables are correlated, it does not mean one causes the other, which is a common misconception that data analysts must avoid.
What is a confounding variable and how does it relate to correlation?
-A confounding variable is an external factor that influences two different variables, making them appear correlated when they are not. The video uses the example of hot weather leading to both increased ice cream sales and shark attacks, where the weather is the confounding variable.
Why is it important for data analysts to consider sample size when analyzing correlations?
-Smaller sample sizes can lead to higher correlations that may not accurately represent the true population. Data analysts should ensure their sample size is large enough to provide meaningful and representative correlations in their analysis.
What is the speaker's stance on the future demand for data analysts and data scientists?
-The speaker believes that data analysts and data scientists will continue to be in high demand, as data collection and analysis are still in their early stages and critical for businesses to make informed decisions, especially with advancements in AI.
Outlines
📊 Common Mistakes in Data Analysis
The paragraph discusses the prevalent issue of data analysts not being adept at their jobs, not due to a lack of coding skills, but because they often overlook the importance of proper analysis. The speaker emphasizes the significance of statistics in data analysis and data science. The video aims to educate viewers on three common mistakes that novice data analysts make and how to avoid them. The speaker, Rohan, introduces himself as the head of an educational company focused on data analytics and shares his experience in freelancing for e-commerce and tech startups. He invites viewers to join a community on Discord for like-minded individuals interested in data analytics and data science. The first mistake highlighted is sampling bias, which occurs when the sample size taken for analysis does not represent the entire population. Examples are given to illustrate how sampling bias can skew results, such as political polling in urban areas or medical studies focusing only on hospital visitors. The importance of using proper sampling methods for accuracy, reliability, and integrity in studies is stressed, along with the cost-effectiveness of sampling over analyzing entire populations.
🔍 Ensuring Data Quality and Avoiding Correlation-Causation Errors
The second paragraph delves into the second major mistake made by data analysts: using poor data quality. The speaker points out that while this may not always be the analyst's fault, the responsibility lies with them to ensure the data's reliability and accuracy. An example is given where a data pipeline error led to incorrect analysis and subsequent misguided business decisions. The speaker argues for the necessity of data analysts in the modern business landscape, especially with advancements in AI. The paragraph also touches on the importance of validating data from multiple sources and the common issue of data entry errors. The speaker advises on the need for proper data cleaning processes to avoid introducing bias and ensuring data quality. The final mistake discussed is the misconception that correlation implies causation, a critical error that can lead to flawed interpretations of data. The speaker uses the examples of ice cream sales and shark attacks, and chocolate consumption and Nobel Prizes, to illustrate how correlation does not equate to causation and the importance of identifying confounding variables. The paragraph concludes with a call for critical thinking and thorough research to avoid jumping to conclusions based on correlation alone.
Mindmap
Keywords
💡Data Analyst
💡Sampling Bias
💡Data Quality
💡Data Pipeline
💡Data Entry
💡Data Cleaning
💡Correlation
💡Causation
💡Confounding Variable
💡Critical Thinking
💡Sample Size
Highlights
Data analysts often neglect the analysis itself, not just their technical skills.
Statistics are crucial as the backbone of data analysis and data science.
Three common mistakes by beginner data analysts are discussed.
Sampling bias occurs when a sample is not representative of the entire population.
Urban areas may skew political polling data due to their left-leaning nature.
Medical studies can be flawed if only hospital visitors are sampled.
Proper sampling methods ensure accuracy, reliability, and cost-effectiveness.
Data quality is paramount; poor data can lead to incorrect analysis and decisions.
Data pipeline errors can mislead analysis, such as logging data from the wrong quarter.
Data analysts should validate data from multiple sources before conducting analysis.
Data entry errors are common and can significantly affect data accuracy.
Data cleaning should be done carefully to avoid introducing bias.
Correlation does not imply causation, a common mistake in data analysis.
Ice cream sales and shark attacks example illustrates the difference between correlation and causation.
Chocolate consumption and Nobel Prizes example shows the impact of a confounding variable.
Critical thinking is necessary to identify hidden variables influencing data.
Sample size is crucial; smaller sizes can lead to misleading correlations.
Data analysts play a vital role in the data-driven business landscape.
The demand for data analysts is expected to grow with advancements in AI.
Transcripts
this is going to sound a bit harsh but
most data analysts quite frankly aren't
that great at their job and it's not
because they aren't good at coding with
SQL python r or even any bi tool it's
simply because they just neglect the
analysis if you've been on this channel
for a while now you know how much I
value statistics and I truly believe
statistics is the backbone of data
analysis and data science so in this
video we're going to be going over three
of the most common mistakes beginner
data analyst make and how you can avoid
them so make sure to actually save this
video take some notes and then refer
back to the later because I promise you
these are things that will not be taught
to you on the job and you just learn
with years of experience if you're new
here my name is Rohan and I run an
education company teaching people data
analytics and also do data analyst
freelancing for e-commerce and Tech
startups if you're interested in joining
the like-minded community all into data
analytics and data science go ahead and
join the Discord down below we have
almost 5,000 people in it now the first
mistake I see people make is sampling
bias sampling bias basically occurs when
a small sample size that you're taking
for your analysis isn't representative
of the entire population so let's say
you're taking a polling analysis and you
want to actually figure out the
political views of a certain region or
the country and let's say you were going
to go to a bunch of urban areas because
you know friends in Chicago San
Francisco New York and you ask all your
friends to take polls of a ton of data
with their friends and their family
members in these urban areas the thing
is most of these Urban environments are
pretty left leaning rather than right
leaning so your poll and your data may
not be as representative of the entire
country where you might be leaving more
rural areas out of the analysis so these
biased collections of data even though
the analysis may be correct may just not
be represented of the entire population
and this is what we called sampling bias
another example of this is a health
study let's say you conduct a medical
study for a new drug that you're
releasing and you're only taking a small
sample size from just people who visit
hospitals it might be the easiest for
you to collect data for people who
already visit hospitals versus people
who don't visit hospitals but your study
would be overlooking one thing the
people that may visit hospitals more may
tend to be older and more vulnerable
people to diseases so it may not be that
accurate for the entire population so it
is very important to use proper sampling
methods to make sure you have accuracy
reliability and integrity in your study
it's also much more cost effective to
sample rather than take an entire
population imagine asking all 300
million people in the United States or
the billions of people in the world for
a poll it's just not feasible very
expensive so it is much more cost
effective to just take a sample that is
representative of the entire population
so I think while taking these studies
and the analysis be cognizant of the
data you're actually using while
conducting any analysis for any of your
stakeholders or customers now the second
biggest mistake I see data analyst and
data scientists make is using poor data
quality and I know this may not be your
fault as a data scientist or data
analyst it may be a data engineering's
fault or maybe a software engineer's
fault who's collecting the data itself
but the fact of the matter is if your
data isn't reliable or your data isn't
accurate by any means this will
completely mess up your analysis and
completely mess up the decisions that
you're recommending to your customers so
let's say you're working for company
that has a huge the problem is you don't
understand that there's actually a data
pipeline error in Q4 2024 rather than Q4
2023 so these maybe week or two inside
of the quarter would lead to a decrease
in sales for the entire quarter just
because you didn't log the data so
because of this you think that the sales
are actually going down and you actually
spend more on marketing even though you
probably didn't need to you spend more
on R&D even though you probably didn't
need to and this can hurt the business
in the long run it is always just so
funny to me when people think data
analyst or data scientists aren't needed
at companies but the reality is if you
want to survive in business in this like
honestly Century you need to be using
data to some extent gone are the days
where you can just use intuition and the
next step is making sure you have
accurate and reliable data sources for
this and I'll be honest like I think
we've only reached the tip of the
iceberg when it comes to data collection
and data analysis so when people ask me
are data analysts still going to be in
demand in 10 years you do the math we
haven't even touched the tip of the
iceberg when it comes to data collection
how are we going to actually measure and
make decisions based on this data if we
don't have data analyst and data
scientists especially with all these AI
advancements another thing I want you to
watch out for in data accuracy is data
entry there are a ton of people who are
doing data entry currently and it is not
uncommon for a human to make a mistake
when it comes to data entry often times
this a very like tedious task and you
just want to want to get done with it
and there's a lot of errors that come
into this so what I recommend you to do
as a data analyst in my career I've had
jobs where I've taken two data sources
that should have yielded the same number
because they're coming from the same
sales data but the problem is they were
different by 5 to 10% and this is maybe
because data entry was incorrect or the
data pipelines weren't working so my
point here is your job as a data
analysis you may not know if a
pipeline's broken you may not know if
the data entry was correct but your job
is to figure out and validate the data
with a Second Source or even a third
Source or even a third party data and
just validate before conducting analysis
this is so important and I recommend
this for every single analysis you do
you do the proper due diligence and make
sure you are validating Data before what
I've done at a lot of companies is I
actually have status reports every
morning I'd get a status report on the
data that was imported from our data
pipelines coming from third party or
even first party data so I'd always just
validate with two different data sources
make sure it's within like a 5% margin
and if it is normally it's good to go
but if it isn't I always bring it up
before doing any work for that day
because the data analysis of poor data
is incorrect next thing I want to
mention is data cleaning you people data
clean to make sure data quality is good
but I've seen a lot of people do this
mistake of actually like inputting in
data and missing values whether it be an
average or something but sometimes it's
just Incorrect and then you can also
have bias where those columns that
aren't filled up with data may not be
filled up for a reason and that could
also cause a bias in the study so I want
you to be very careful while doing data
cleaning make sure you have the proper
automation the proper testing in place
before conducting an analysis okay so
the most common mistake I se you dat
analyst make is they think correlation
is causation and this is probably
drilled into you in your stats classes
or high school math classes or college
classes but it is more important now
than ever before CU you are a data
analyst you are a data scientist and
quite frankly it's your job to make
sense of data and provide decisions for
those of you who don't know correlation
just means the relationship between two
or more variables with each other
however just because two variables are
correlated does not mean they are
causing each other to go up or down so
the most popular example I like to give
is the ice cream example during the
summertime with hotter weather ice cream
sails go up and shark attacks also go up
but is it safe to say just because Cold
Stone decides to sell more ice cream in
the summer this is causing shark attacks
no that's ridiculous right it's because
it's hot outside which is called the
confounding variable a confounding
variable is a variable that influences
two different variables that then appear
to be correlated even though they aren't
causation so because it's hot outside in
the summer more people are likely to go
to the beach to cool off and then more
people are also going to go buy ice
cream because it's hot outside to cool
down so even though two variables have
gone up does not mean that they cause
each other the next example I like to
bring up with this is countries with
higher chocolate consumption tend to
have more Noble Prize winners and you
may be wondering hey that makes sense if
you chocolate I can get smarter and I
can do more noble PRI and I can achieve
more noble prizes but the reality is
think about it where is chocolate more
predominant in terms of consumption
wealthier countries wealthier countries
tend to also have better education
systems which also leads to more people
being able to do research and more
research equals more noble prizes being
given so it's not necessary that
chocolate is causing people to win all
these Nobel prizes and become a very
prestigious Nation it's because the
wealth in the country has both caused
people to buy more chocolate consume
more chocolate and also achieve winning
a Nobel Peace Prize so always remember
in every single job I have there may be
another variable influencing the two
variables you are comparing
and often times it's hidden in it's your
job as a data analyst and data scientist
to find that variable and bring it to
light in your presentation and in your
analysis so I mean there's really no
clear solution to this it just comes
down to critical thinking even though if
you see a very strong correlation of
like a 6 or 7 I wouldn't necessarily
jump and think a it's causation always
do your research and always find out and
investigate further this is why it takes
time and you shouldn't rush through
analysis my last note on correlation is
make sure your sample size is big enough
typically smaller sample sizes lead to
higher correlations even though they may
not be indicative of a true population
so make sure you have a measure of what
sample size you're using what problem
you're trying to solve and really piece
together all these things together and
that's what forms a highquality data
analyst so these were the three most
common mistakes that I myself have seen
other data analyst make in the beginning
myself included so if you got any value
to this video please leave a like
subscribe and I'll see you the next one
[Music]
Ver Más Videos Relacionados
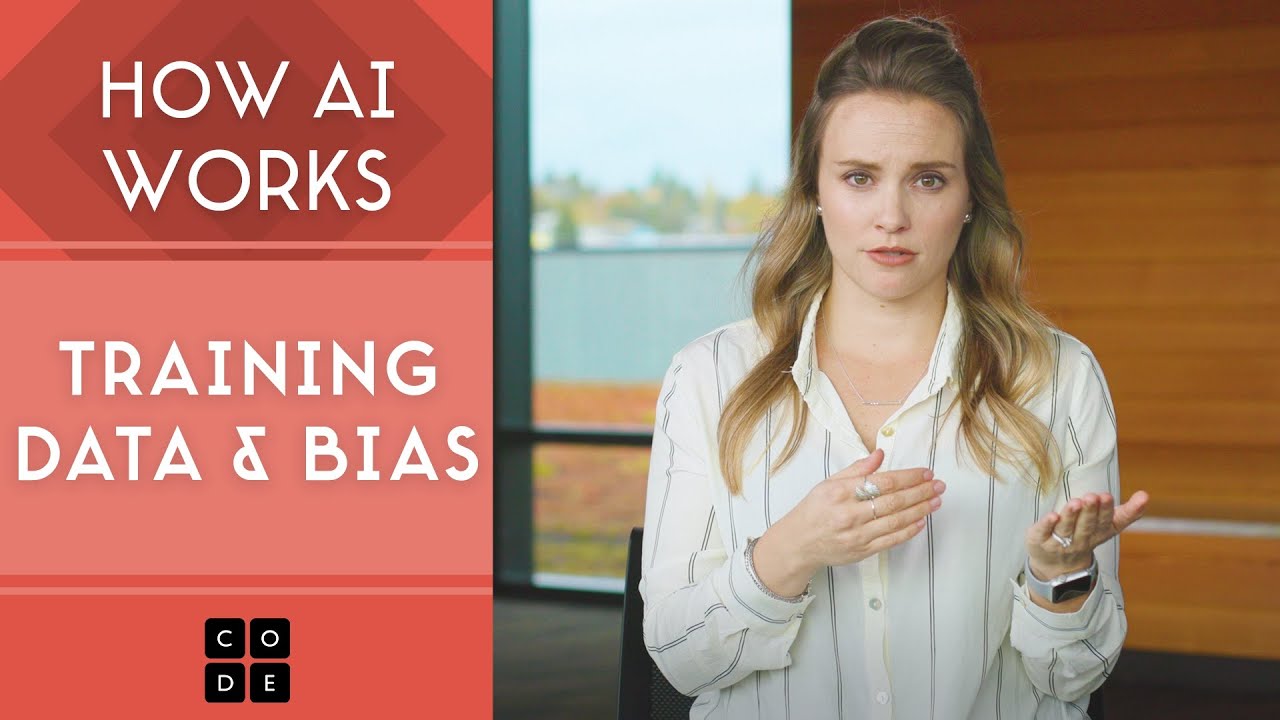
AI: Training Data & Bias

Statistical and Critical Thinking

You need data literacy now more than ever – here’s how to master it | Talithia Williams
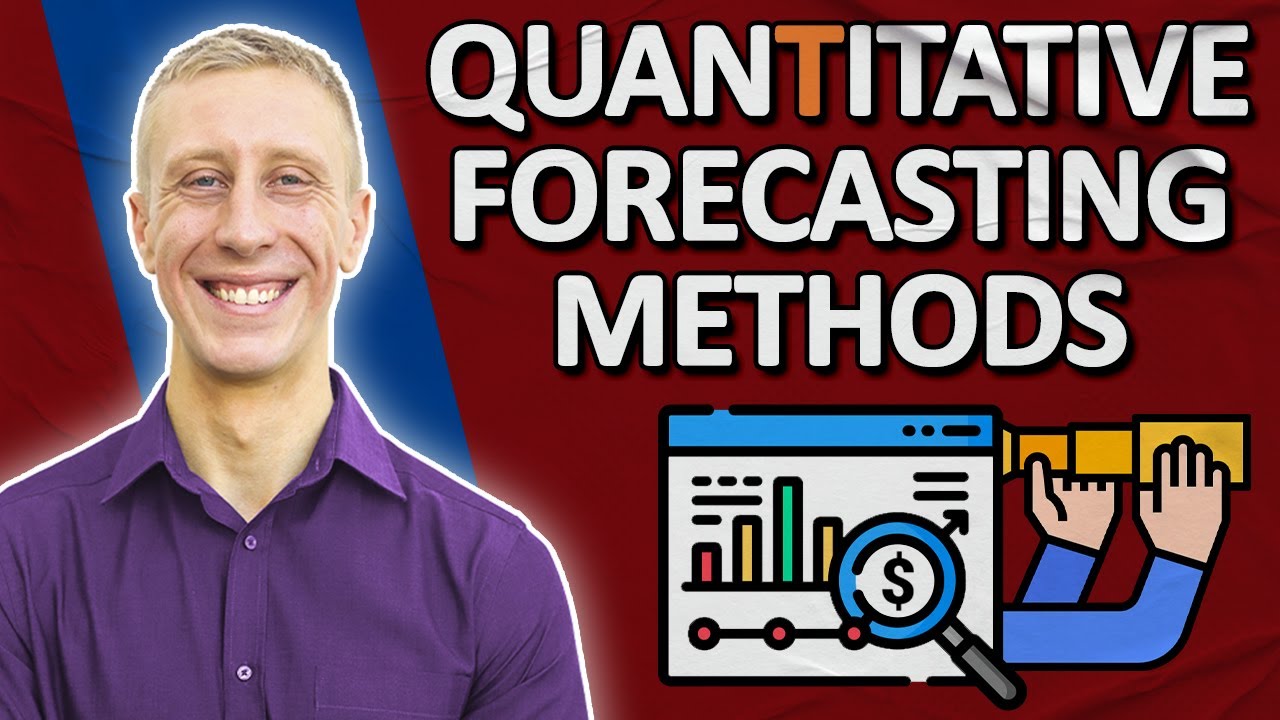
Quantitative Forecasting Methods in Business Operations
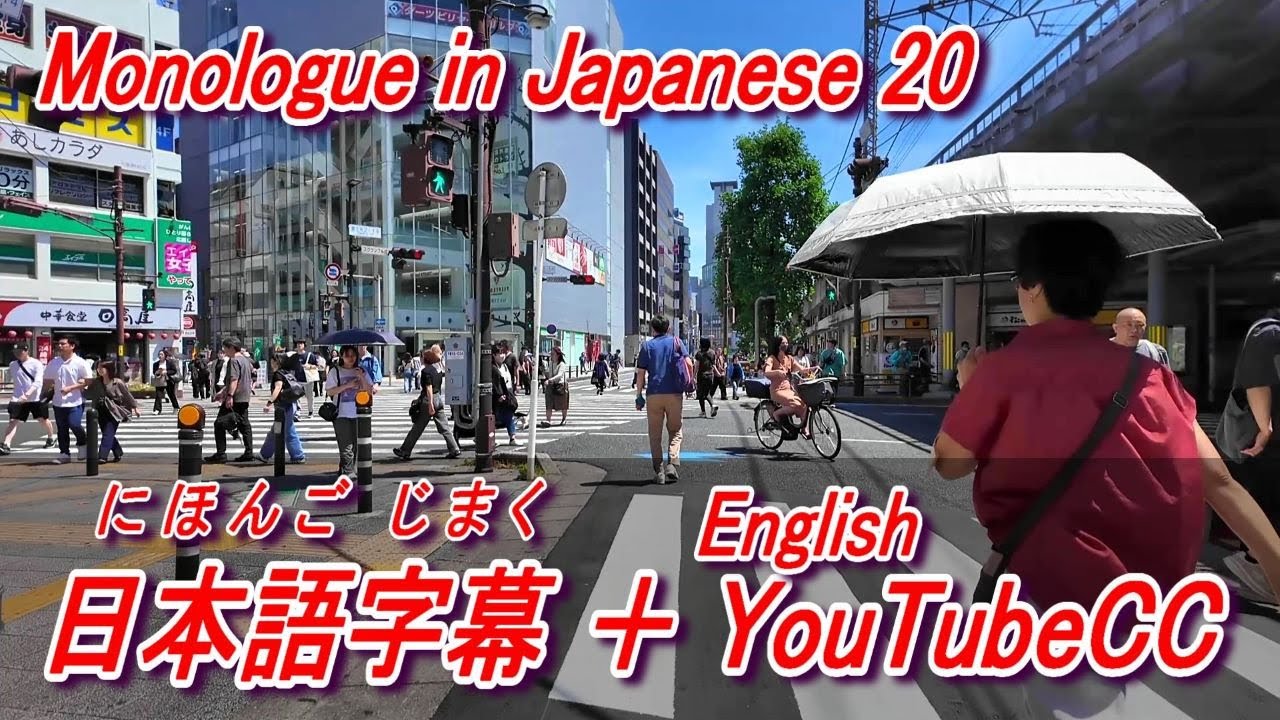
Monologue in Japanese [ 20 ] - 日本語字幕 + YouTubeCC [ Eng ]
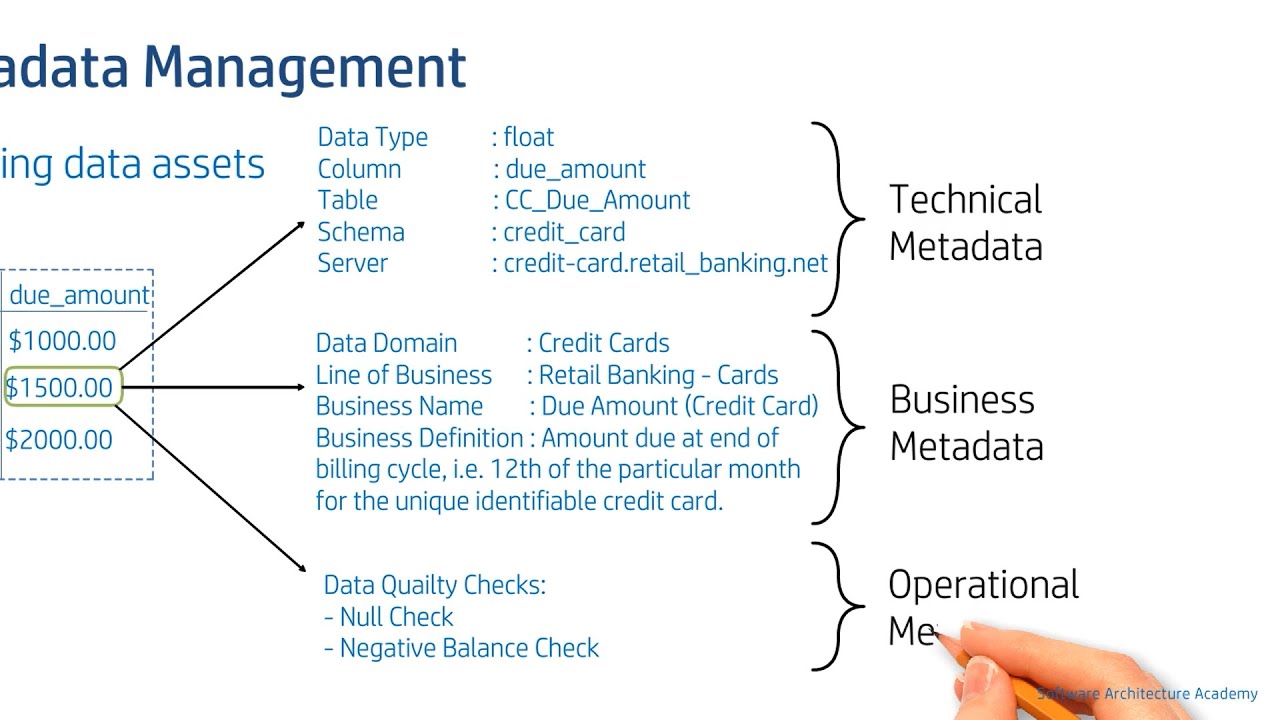
Metadata Management & Data Catalog (Data Architecture | Data Governance)
5.0 / 5 (0 votes)