MIT 6.S191 (2018): Issues in Image Classification
Summary
TLDRThe speaker discusses the advancements in deep learning for image classification, highlighting the impressive error rate reductions in recent years. However, they point out the limitations of current models, using examples from the Open Images dataset, which may not accurately classify images due to biases in the training data. The talk emphasizes the importance of considering the differences between training and inference distributions and the potential societal impact of machine learning models. The speaker concludes by advocating for awareness of these issues and provides resources on machine learning fairness.
Takeaways
- 📈 The error rate in image classification on ImageNet has significantly decreased over the years, with contemporary results showing impressive accuracy.
- 🌐 The Open Images dataset, with 9 million images and 6,000 labels, is a more complex and diverse dataset compared to ImageNet.
- 🤖 Deep learning models may sometimes fail to recognize human presence in images, indicating that image classification is not entirely solved.
- 🔍 The concept of stereotypes in machine learning can be related to labels based on experience from the training set, which may not always be causally related to the outcome.
- 🌍 Geo-diversity in datasets is crucial; Open Images dataset is predominantly from North America and Europe, lacking representation from other regions.
- 🔄 The assumption in supervised learning that training and test distributions are identical is often not true in real-world applications, which can lead to biased models.
- 📊 It's important to consider the societal impact of machine learning models, especially when they are based on societally correlated features.
- 📚 Understanding and addressing machine learning fairness issues is becoming increasingly important as these models become more prevalent in everyday life.
- 🔗 Additional resources on machine learning fairness are available, including papers and interactive exercises to explore the topic further.
- 💡 The speaker emphasizes the importance of not just focusing on improving accuracy but also on the societal implications of machine learning models.
Q & A
What is the main topic of the talk?
-The main topic of the talk is the issues with image classification using deep learning, particularly focusing on the challenges and biases in datasets like ImageNet and Open Images.
How has the error rate in image classification changed over the years?
-The error rate in image classification has significantly reduced over the years, with contemporary results showing an error rate of around 2.2%, which is considered astonishing compared to the 25% error rate in 2011.
What is the difference between ImageNet and Open Images datasets?
-ImageNet has around 1 million images with 1,000 labels, while Open Images has about 9 million images with 6,000 labels, and it supports multi-label classification.
Why did the deep learning model fail to recognize a bride in one of the images discussed in the talk?
-The model failed because it was trained on a dataset that did not represent global diversity well, particularly lacking data from regions like India, leading to a biased model that missed the presence of a human in the image.
What is a stereotype in the context of machine learning?
-In the context of machine learning, a stereotype is a statistical confounder that has a societal basis, which may lead to biased models picking up on correlations that are not causally related.
What is the importance of considering the training and inference distributions in machine learning models?
-Considering the training and inference distributions is crucial to ensure that the model's performance is not just limited to the training data but also generalizes well to new, unseen data in the real world.
How does the geolocation diversity in the Open Images dataset compare to global diversity?
-The Open Images dataset is not representative of global diversity, as the majority of the data comes from North America and a few European countries, with very little data from places like India or China.
What is the potential issue with using a feature like shoe type in a machine learning model?
-Using a feature like shoe type can lead to biased predictions if it is strongly correlated with the target variable in the training data but not necessarily causally related, which may not generalize well to the real world.
What is the speaker's advice for individuals interested in machine learning fairness?
-The speaker advises being aware of differences between training and inference distributions, asking questions about confounders in the data, and not just focusing on improving accuracy but also considering the societal impact of the models.
What resources does the speaker recommend for further understanding of machine learning fairness?
-The speaker recommends a website with a collection of papers on machine learning fairness, as well as interactive exercises on adversarial debiasing to explore the topic further.
Outlines
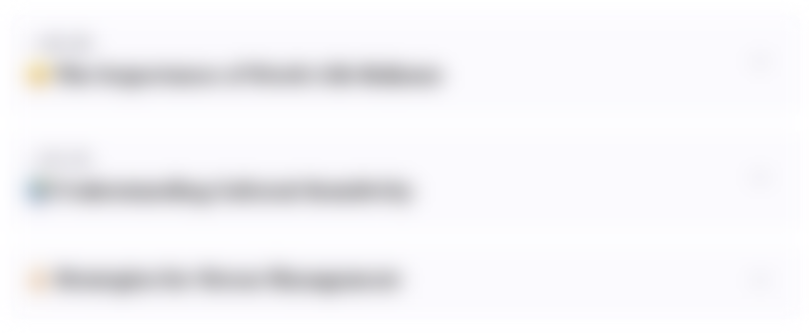
Esta sección está disponible solo para usuarios con suscripción. Por favor, mejora tu plan para acceder a esta parte.
Mejorar ahoraMindmap
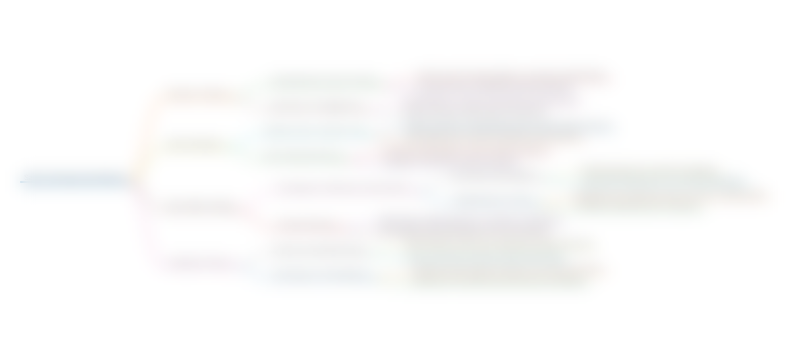
Esta sección está disponible solo para usuarios con suscripción. Por favor, mejora tu plan para acceder a esta parte.
Mejorar ahoraKeywords
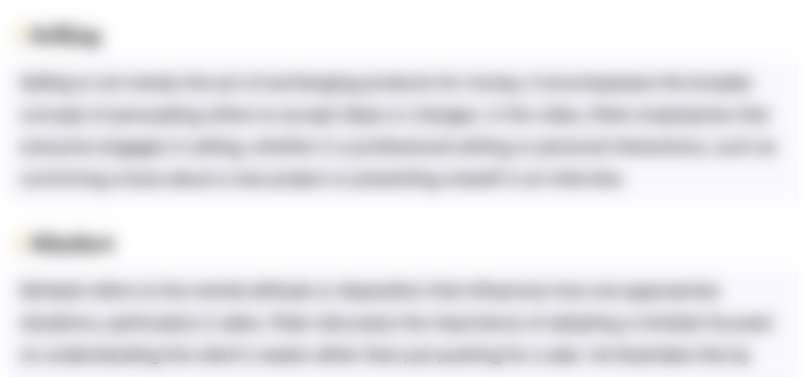
Esta sección está disponible solo para usuarios con suscripción. Por favor, mejora tu plan para acceder a esta parte.
Mejorar ahoraHighlights
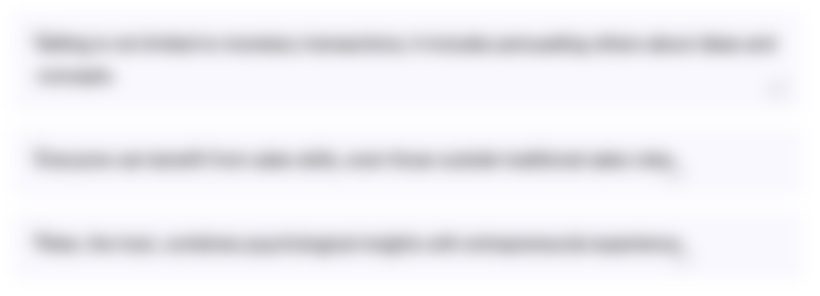
Esta sección está disponible solo para usuarios con suscripción. Por favor, mejora tu plan para acceder a esta parte.
Mejorar ahoraTranscripts
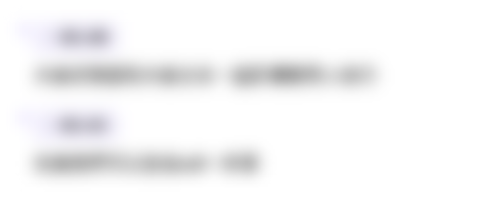
Esta sección está disponible solo para usuarios con suscripción. Por favor, mejora tu plan para acceder a esta parte.
Mejorar ahoraVer Más Videos Relacionados
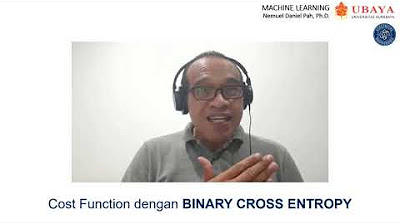
4. Binary Cross Entropy
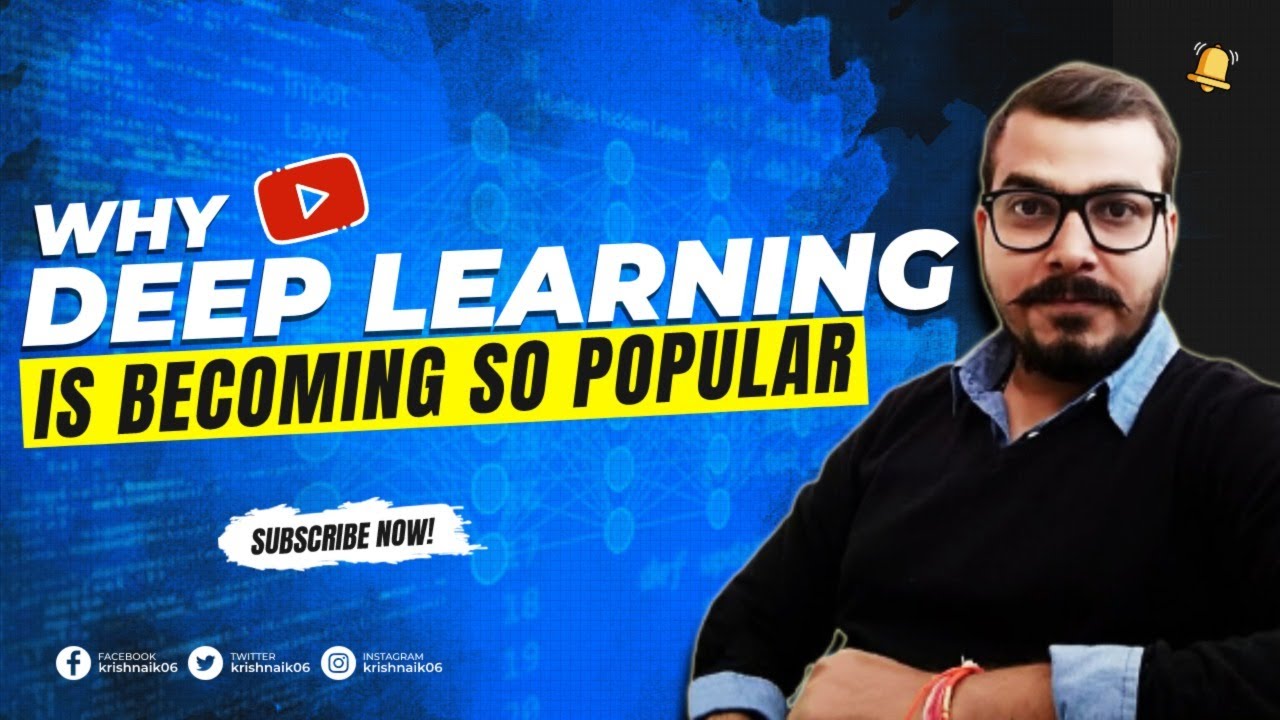
Why Deep Learning Is Becoming So Popular?🔥🔥🔥🔥🔥🔥
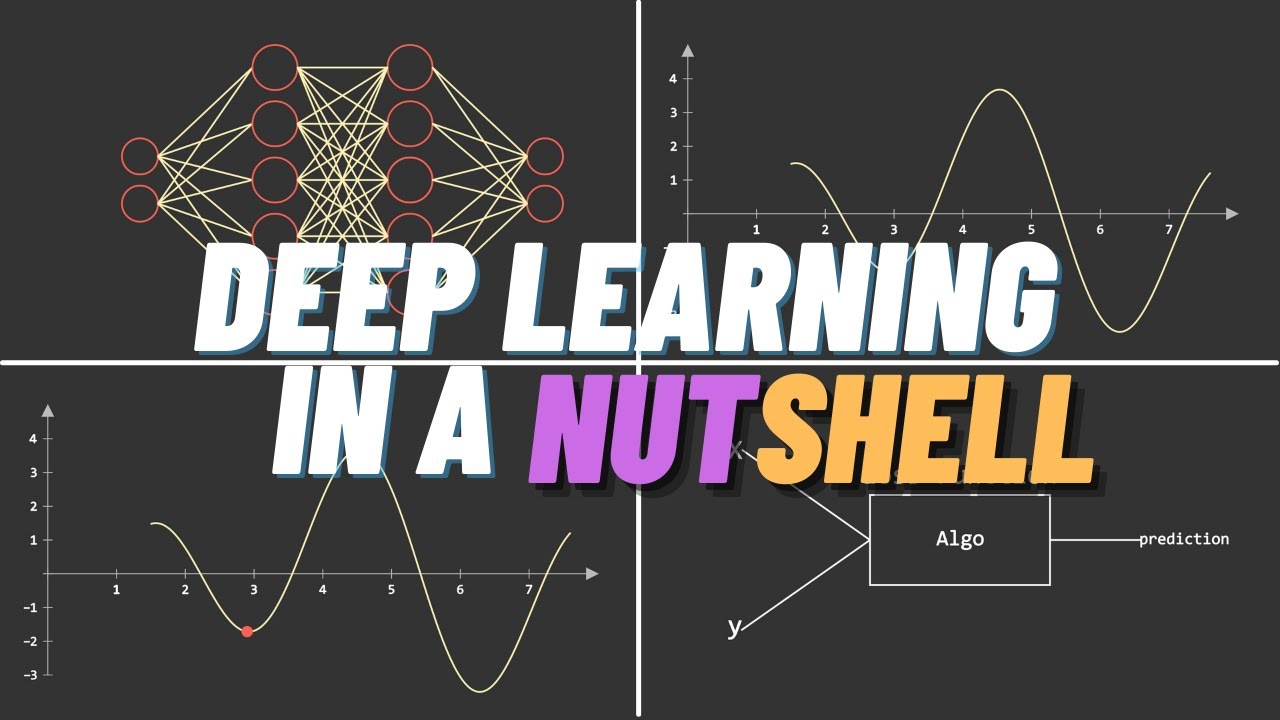
Deep Learning: In a Nutshell
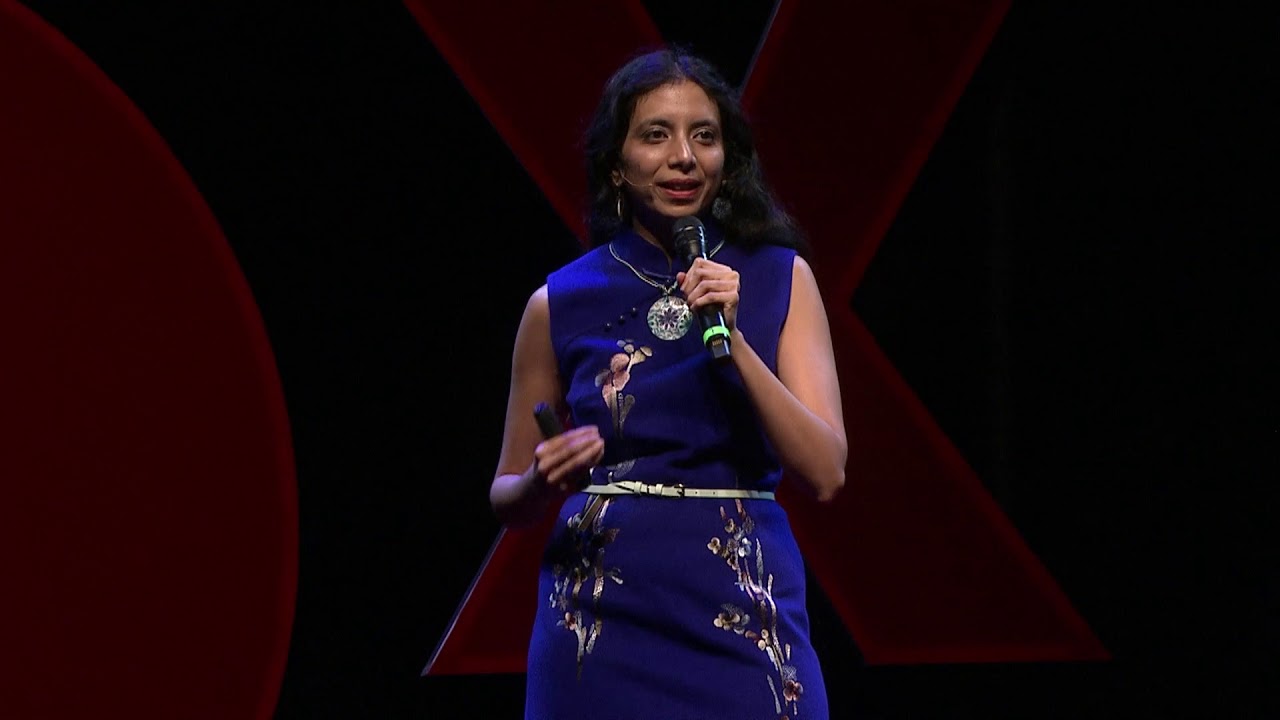
Trinity of Artificial Intelligence | Anima Anandkumar | TEDxIndianaUniversity
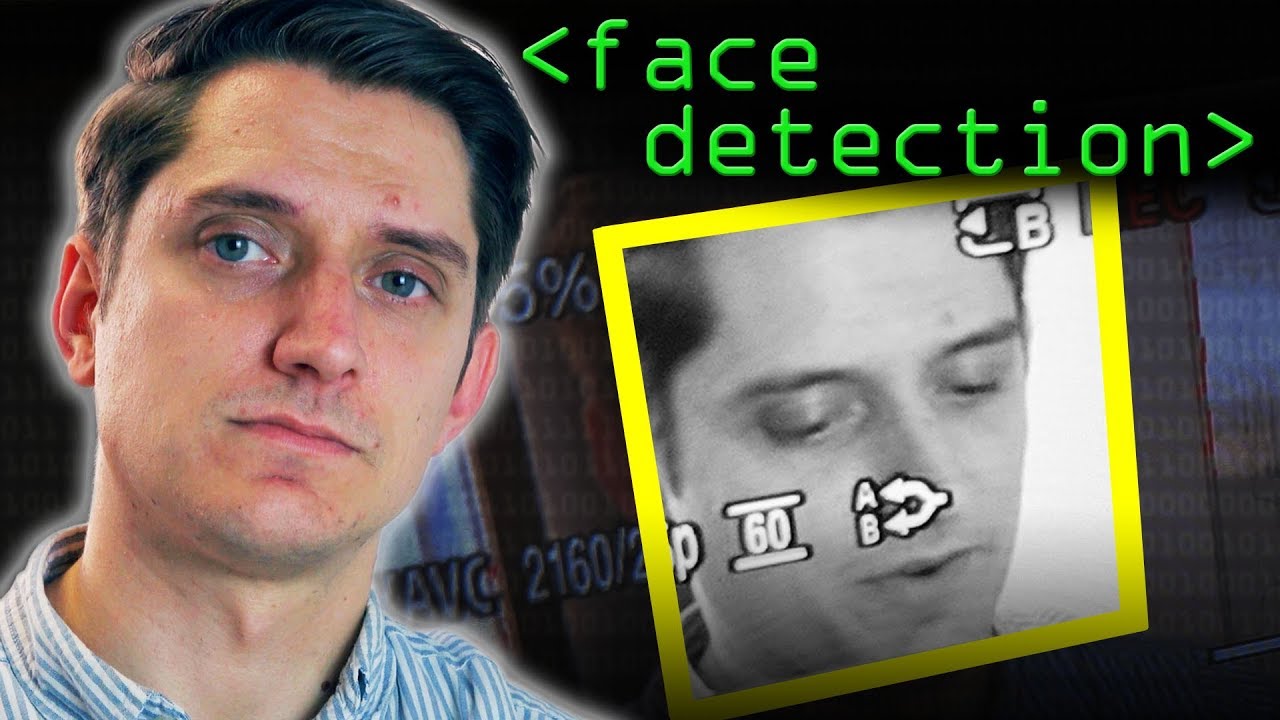
Detecting Faces (Viola Jones Algorithm) - Computerphile
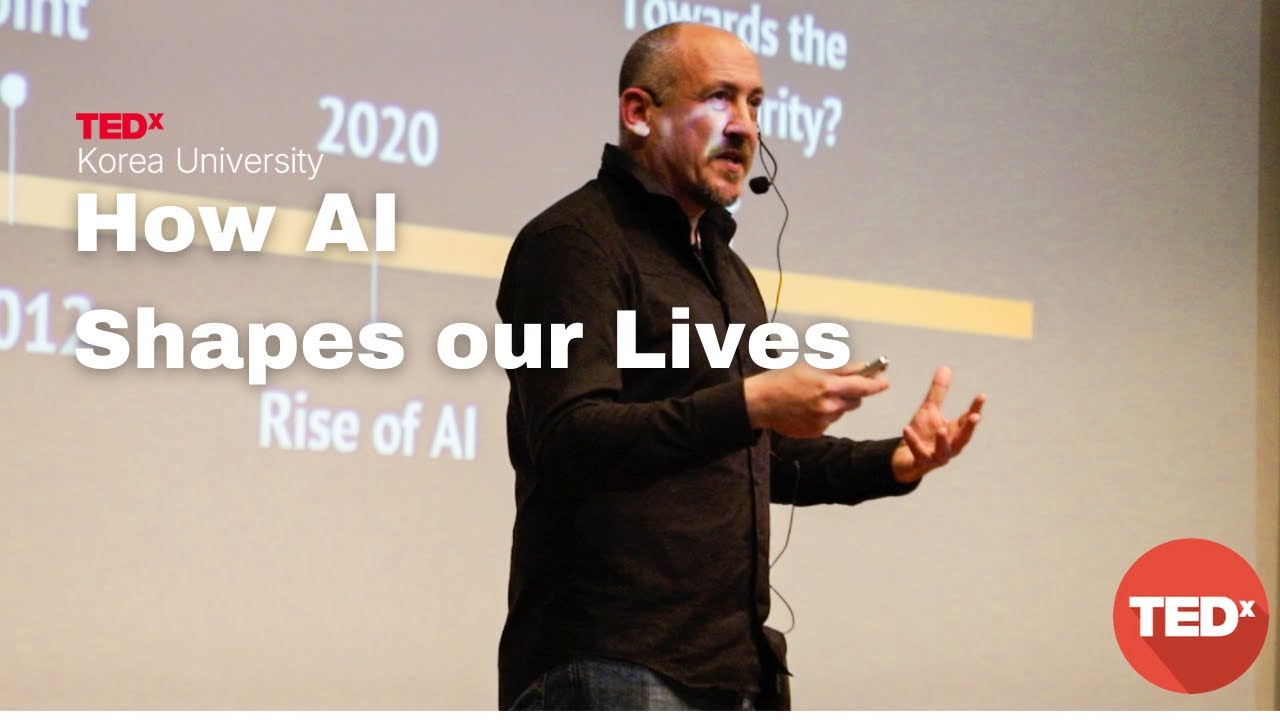
How AI Technology Shapes Our Lives | Christian Wallraven | TEDxKorea University
5.0 / 5 (0 votes)