Python Exercise on kNN and PCA
Summary
TLDRThe video script introduces an educational session on machine learning courses, focusing on the K-Nearest Neighbors (K-NN) classifier algorithm. It explains how to use the K-NN algorithm with available data, highlighting its importance in classification tasks. The session covers key concepts such as the Curse of Dimensionality, Principal Component Analysis (PCA) for dimensionality reduction, and the use of symmetric metrics for feature scaling. The script also touches on the process of converting the algorithm into code, emphasizing the practical application of machine learning techniques in classification problems.
Takeaways
- 📘 The session introduces the concept of K-Nearest Neighbors (K-NN) as a classification algorithm.
- 🔍 K-NN is based on an algorithm that determines how to classify new data based on labeled training data.
- 👉 The script discusses the importance of choosing the right number of neighbors (K) for the K-NN classifier.
- 📊 It mentions the use of the Curse of Dimensionality, which is a challenge in high-dimensional spaces.
- 🧐 The session explains the use of principal component analysis (PCA) to reduce the dimensionality of the data.
- 📈 PCA helps in identifying the principal components that explain the most variance in the data.
- 🤖 The script covers the concept of feature selection and its importance after applying any face recognition algorithm.
- 🛠️ It talks about the process of training the model using the training data.
- 🔧 The script briefly touches on the use of agents and the process of normalization of images for better classification.
- 📝 The importance of symmetric metrics is highlighted for calculating the distance between data points.
- 👨🏫 The session ends with a note on looking forward to understanding how to preprocess data in future videos.
Q & A
What is the main topic of the introduction in the video script?
-The main topic of the introduction is Machine Learning courses, focusing on how to use sessions effectively in the context of the course.
What is K-Nearest Neighbors (K-NN) algorithm mentioned in the script?
-K-Nearest Neighbors (K-NN) is a classification algorithm that is mentioned as the first topic of the course. It is used for making predictions based on the closest data points in the feature space.
How does the script describe the use of the K-NN algorithm in the context of data?
-The script describes that the K-NN algorithm is used to determine how to make predictions based on the available data in a specific dataset.
What is the purpose of the 'Curry Point' mentioned in the script?
-The 'Curry Point' is likely a term used to refer to a specific point or concept in the course that helps in understanding the application of the K-NN algorithm more clearly.
What does the script suggest about the dimensionality of the data used in the course?
-The script suggests that the course deals with high-dimensional vector spaces, implying that it covers complex data sets with many features.
What is Principal Component Analysis (PCA) mentioned in the script?
-Principal Component Analysis (PCA) is a technique mentioned in the script that is used to reduce the dimensionality of the data while retaining the most important patterns or features.
How is the Principal Component Analysis (PCA) algorithm used in the course?
-The PCA algorithm is used to find the principal components in the data, which helps in simplifying the data for better analysis and understanding.
What is the role of 'Agent-Phases' in the context of the script?
-The 'Agent-Phases' mentioned in the script likely refers to different stages or steps in the machine learning process, which the course will cover in detail.
What does the script imply about the importance of understanding the 'Agent-Phases'?
-The script implies that understanding the 'Agent-Phases' is crucial for grasping the concepts of the machine learning course and applying them effectively.
How does the script mention the use of 'Symmetric Metrics'?
-The script mentions the use of 'Symmetric Metrics' as a method to measure or evaluate the performance of the machine learning models, ensuring that the results are consistent and reliable.
What is the final outcome or goal mentioned in the script regarding the machine learning models?
-The final outcome or goal mentioned in the script is to achieve a well-performing machine learning model that can classify data accurately.
Outlines
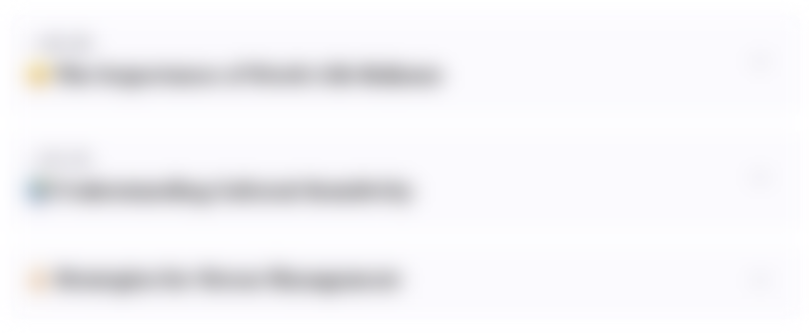
Dieser Bereich ist nur für Premium-Benutzer verfügbar. Bitte führen Sie ein Upgrade durch, um auf diesen Abschnitt zuzugreifen.
Upgrade durchführenMindmap
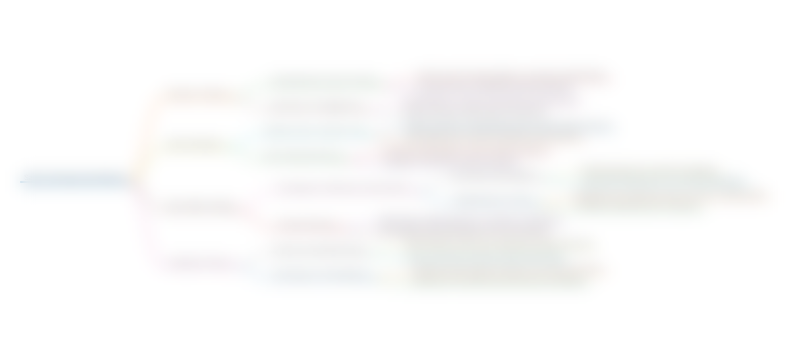
Dieser Bereich ist nur für Premium-Benutzer verfügbar. Bitte führen Sie ein Upgrade durch, um auf diesen Abschnitt zuzugreifen.
Upgrade durchführenKeywords
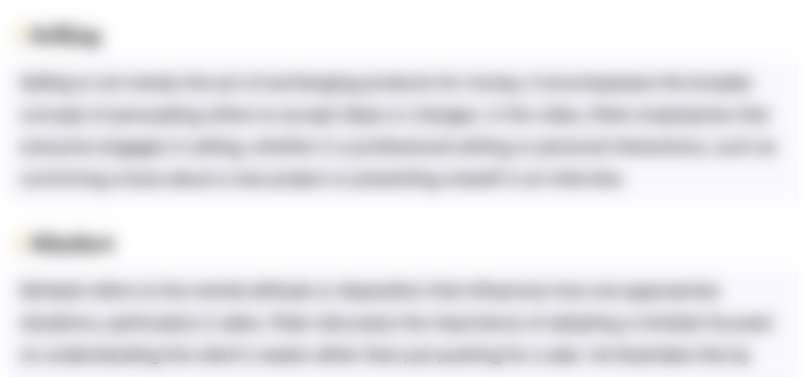
Dieser Bereich ist nur für Premium-Benutzer verfügbar. Bitte führen Sie ein Upgrade durch, um auf diesen Abschnitt zuzugreifen.
Upgrade durchführenHighlights
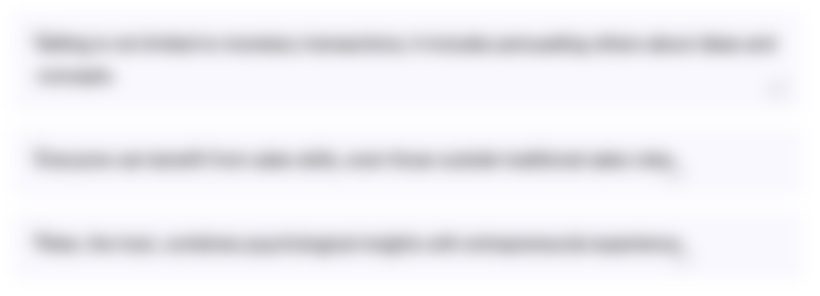
Dieser Bereich ist nur für Premium-Benutzer verfügbar. Bitte führen Sie ein Upgrade durch, um auf diesen Abschnitt zuzugreifen.
Upgrade durchführenTranscripts
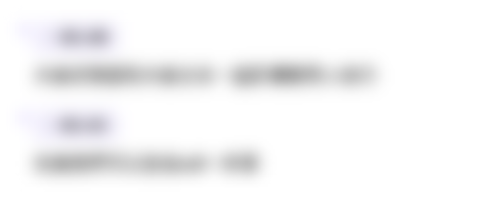
Dieser Bereich ist nur für Premium-Benutzer verfügbar. Bitte führen Sie ein Upgrade durch, um auf diesen Abschnitt zuzugreifen.
Upgrade durchführenWeitere ähnliche Videos ansehen
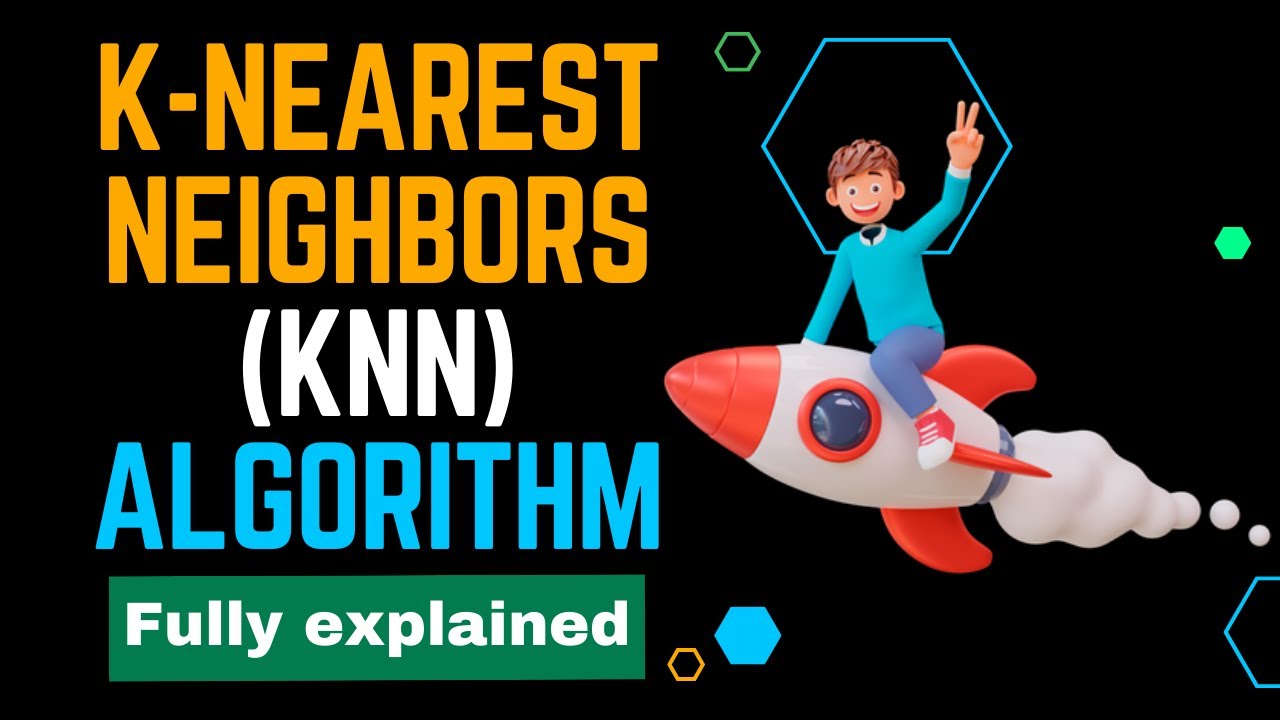
Lecture 3.4 | KNN Algorithm In Machine Learning | K Nearest Neighbor | Classification | #mlt #knn
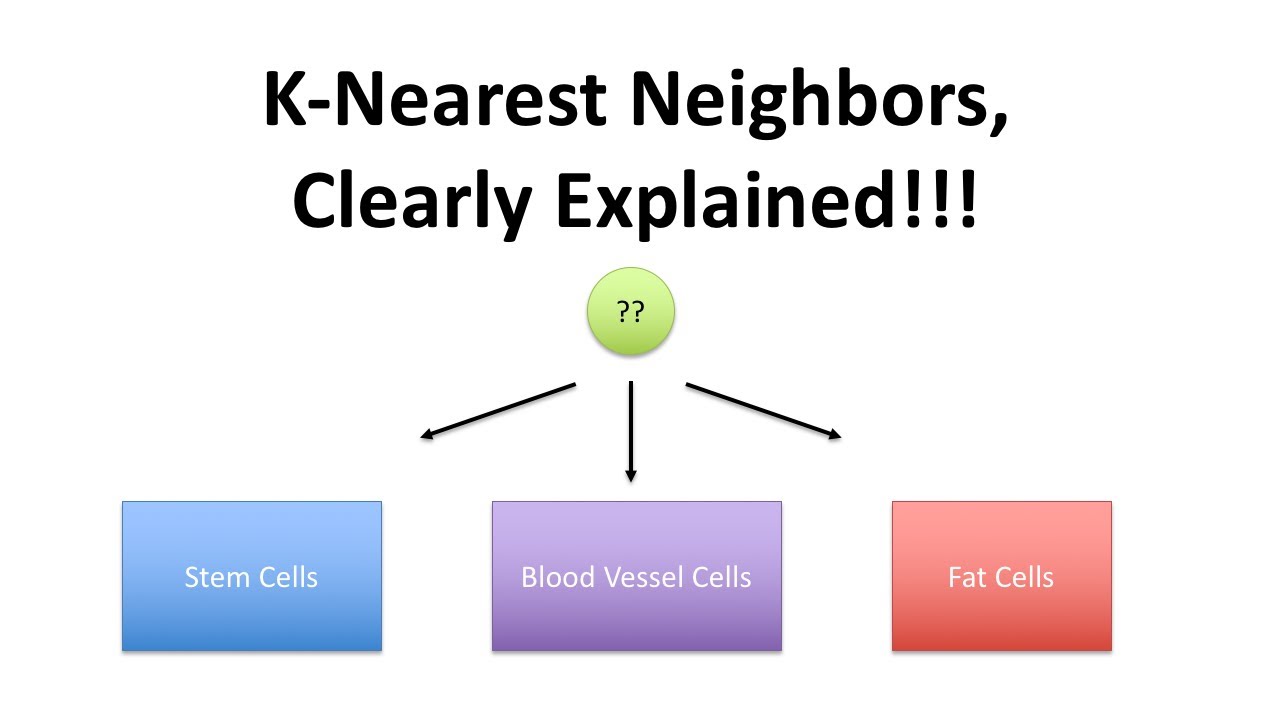
StatQuest: K-nearest neighbors, Clearly Explained
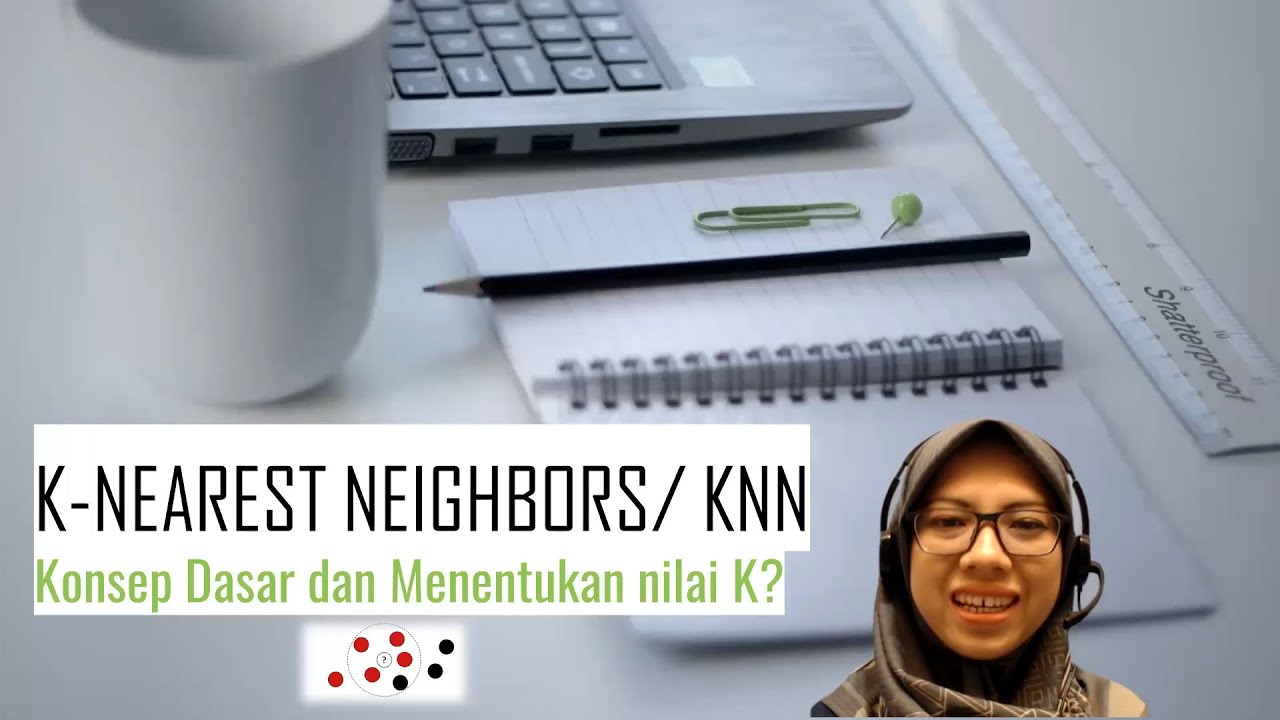
Konsep Algoritma KNN (K-Nearest Neigbors) dan Tips Menentukan Nilai K
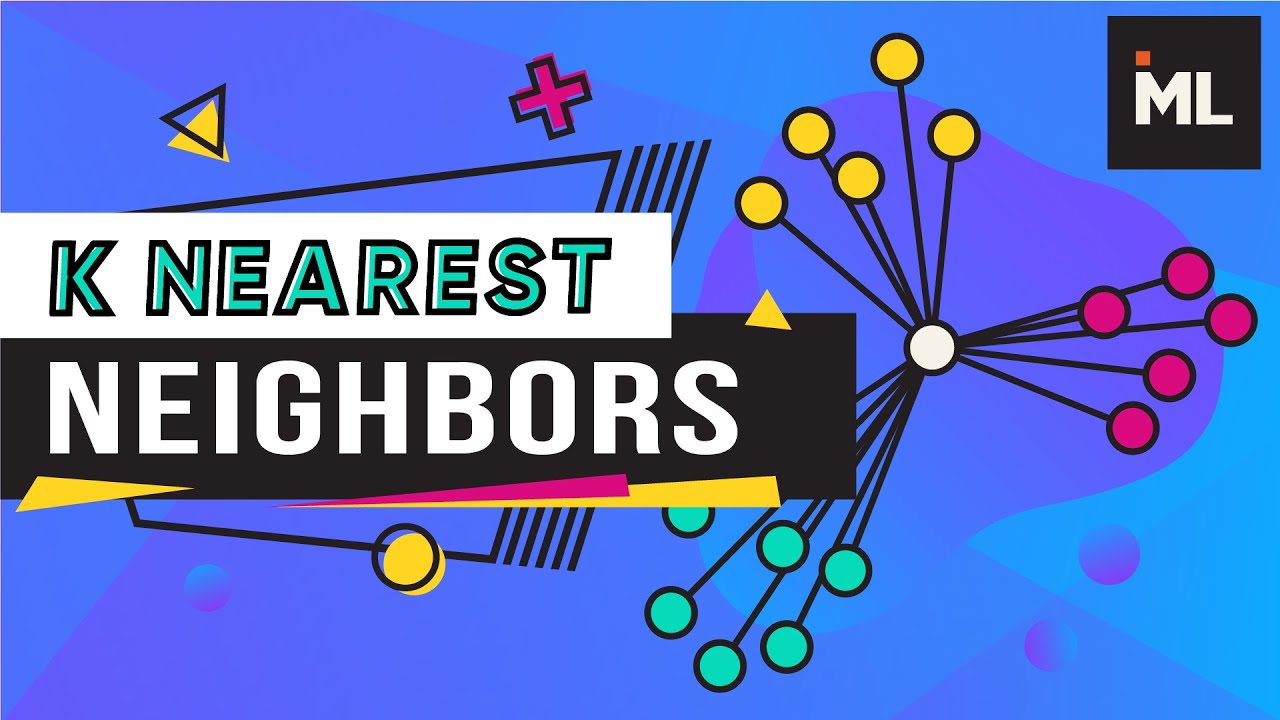
K Nearest Neighbors | Intuitive explained | Machine Learning Basics

All Learning Algorithms Explained in 14 Minutes
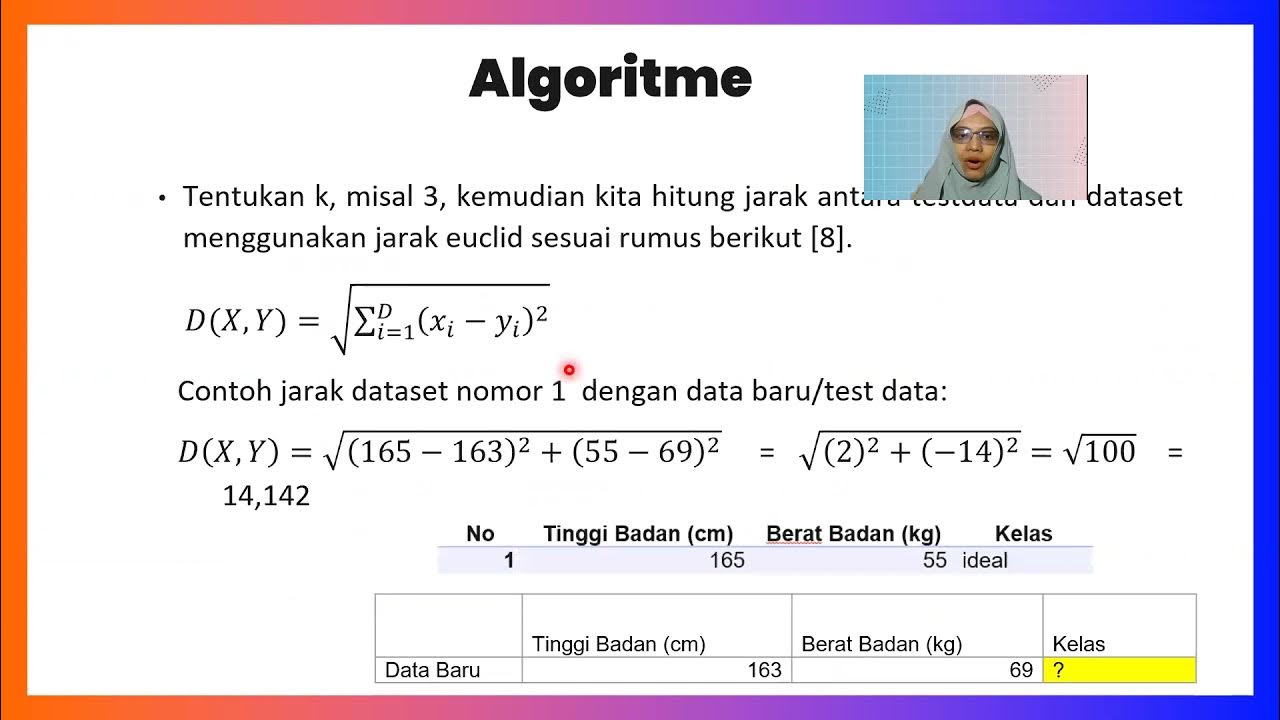
K-Nearest Neighbors Classifier_Medhanita Dewi Renanti
5.0 / 5 (0 votes)