Metas AI Boss Says He DONE With LLMS...
Summary
TLDRIn a recent discussion, AI expert Yan Lukan expressed skepticism about the future of large language models (LLMs) in AI development, arguing that they are limited and insufficient for achieving general AI (AGI). Lukan emphasizes the importance of building AI systems that can understand the physical world, reason abstractly, and plan effectively. He critiques the reliance on token-based architectures, suggesting that more advanced models like his Joint Predictive Architecture (JPA), which focuses on abstract representation, are key to overcoming current AI limitations. Lukan’s insights highlight the need for a more holistic approach to AI that integrates real-world reasoning and memory.
Takeaways
- 😀 Yan Lukan, a prominent figure in AI research, expresses a reduced interest in Large Language Models (LLMs) as the future of AI.
- 😀 Lukan believes that LLMs, which focus on token prediction, are not suitable for understanding the physical world or achieving AGI.
- 😀 He points out that LLMs are mainly focused on improving at the margin by enhancing data and compute, rather than addressing deeper problems.
- 😀 Lukan emphasizes that AI systems need to understand the physical world, have persistent memory, and be able to reason and plan, which LLMs currently lack.
- 😀 He introduces the concept of 'world models' as a better way to represent the world, compared to token-based approaches used by LLMs.
- 😀 World models allow humans and AI systems to interact with and manipulate the real world, something that LLMs, which predict text, cannot handle effectively.
- 😀 Lukan criticizes attempts to train AI systems to understand the world by predicting video pixels, arguing these efforts fail because they do not work at the abstract representation level.
- 😀 Instead, Lukan advocates for architectures like 'joint embedding predictive architectures' (JEP), which learn abstract representations of the world from data, such as videos.
- 😀 The VJEP (Joint Embedding Predictive Architecture) model is highlighted as a promising approach, where AI learns from abstract video data without relying on pixel-level prediction.
- 😀 Lukan suggests that true AGI will likely emerge from hybrid models that combine various AI capabilities, as opposed to relying solely on LLMs or any single approach.
Q & A
Why is Yan Lukan no longer interested in Large Language Models (LLMs)?
-Yan Lukan suggests that LLMs have reached a point where they are primarily being improved at the margin by industry product people. He believes there are more interesting questions in AI, particularly in areas like machine understanding of the physical world, persistent memory, reasoning, and planning.
What are the four main focuses Yan Lukan is excited about in AI?
-The four areas Yan Lukan is excited about are: 1) How machines can understand the physical world, 2) How they can have persistent memory, 3) How they can reason, and 4) How they can plan. He believes these areas are more important for advancing AI than the current focus on LLMs.
What is Yan Lukan’s critique of using LLMs for reasoning about the physical world?
-Yan Lukan argues that LLMs, with their token-prediction architecture, are not well-suited for reasoning about the physical world. He believes that they focus on discrete tokens, which do not capture the complexity of the continuous, high-dimensional data in the real world.
What is a 'world model' and why is it important for AGI?
-A world model is a mental representation of the physical world, which allows humans (and potentially machines) to understand and interact with the world. Yan Lukan emphasizes that text alone isn't sufficient to form a world model that can lead to Artificial General Intelligence (AGI), as it lacks the ability to model the dynamics of the physical world.
What is the problem with using tokens to represent the physical world?
-Tokens are discrete, finite representations, and when applied to the physical world, they cannot capture the complex, continuous nature of real-world data. Yan Lukan points out that training systems to predict the next token works for text, but fails in real-world applications where higher-level representations are needed.
How does Yan Lukan describe the failure of self-supervised learning in training AI to understand the physical world?
-Yan Lukan explains that attempts to train AI systems using self-supervised learning by predicting video pixels have largely failed. These systems cannot adequately represent the physical world, as they spend too many resources trying to predict unpredictable details, instead of focusing on abstract representations.
What is the VJEP architecture, and why is it important?
-The VJEP (Joint Embedding Predictive Architecture) is a system that learns abstract representations of the physical world through video data. Unlike traditional generative models that fill in missing pixels, VJEP learns to predict missing or masked parts of video at an abstract level, leading to more efficient and realistic learning.
How does the VJEP architecture improve upon traditional methods in training AI systems?
-VJEP improves traditional methods by focusing on abstract representations of video data rather than pixel-level details. This allows it to discard irrelevant information, leading to more efficient training and a better understanding of the physical world, with applications such as detecting whether a video is physically plausible.
What is the significance of the system one and system two thinking model in AI development?
-System one thinking is automatic and reactive, whereas system two is more deliberate and analytical. Yan Lukan compares these two types of thinking to AI systems, suggesting that while current systems are moving towards system one, achieving true AGI will require AI systems that can perform both types of thinking, with a stronger emphasis on system two reasoning.
How does Yan Lukan differentiate between language-based reasoning and reasoning in an abstract mental space?
-Yan Lukan argues that reasoning, as humans experience it, often happens in an abstract mental space, not in the context of language or tokens. He gives the example of visualizing a rotating cube, a task that can be done without language. He believes that for AI to reason effectively, it needs to operate in a similar abstract space rather than token-based language processing.
Outlines
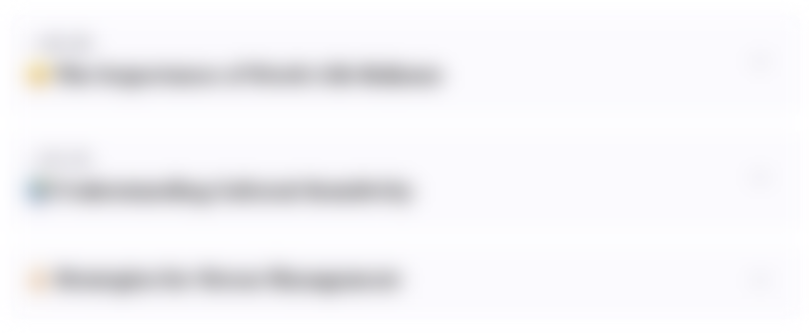
Dieser Bereich ist nur für Premium-Benutzer verfügbar. Bitte führen Sie ein Upgrade durch, um auf diesen Abschnitt zuzugreifen.
Upgrade durchführenMindmap
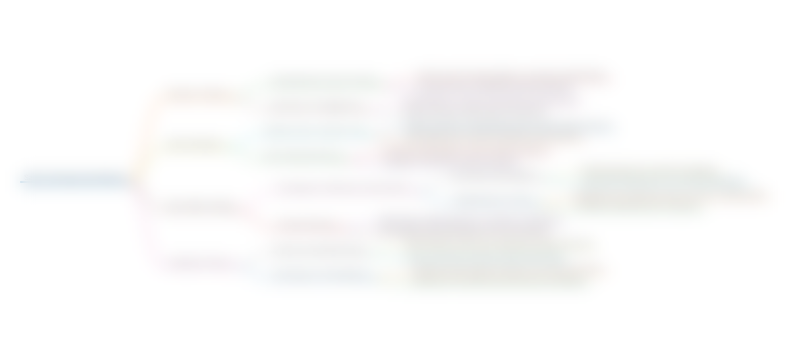
Dieser Bereich ist nur für Premium-Benutzer verfügbar. Bitte führen Sie ein Upgrade durch, um auf diesen Abschnitt zuzugreifen.
Upgrade durchführenKeywords
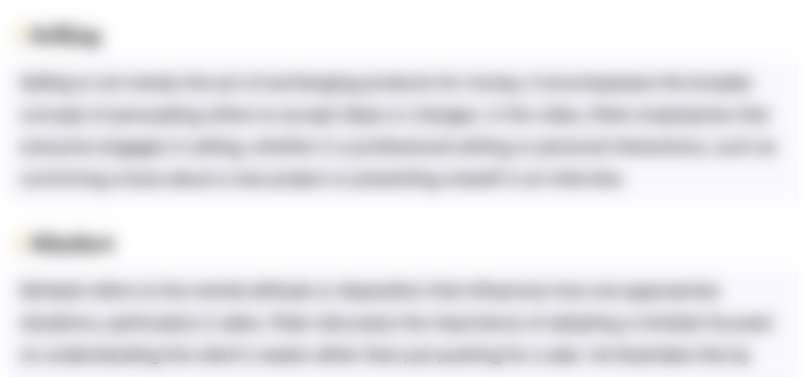
Dieser Bereich ist nur für Premium-Benutzer verfügbar. Bitte führen Sie ein Upgrade durch, um auf diesen Abschnitt zuzugreifen.
Upgrade durchführenHighlights
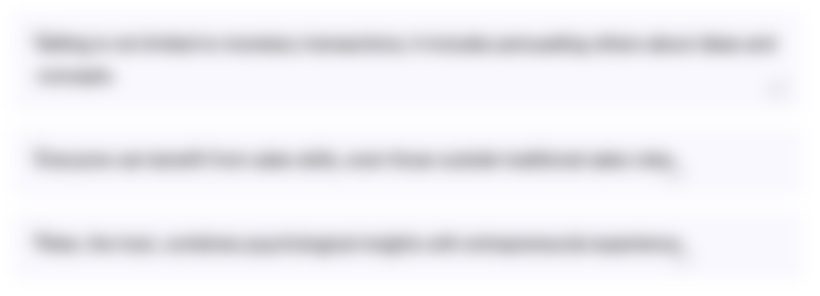
Dieser Bereich ist nur für Premium-Benutzer verfügbar. Bitte führen Sie ein Upgrade durch, um auf diesen Abschnitt zuzugreifen.
Upgrade durchführenTranscripts
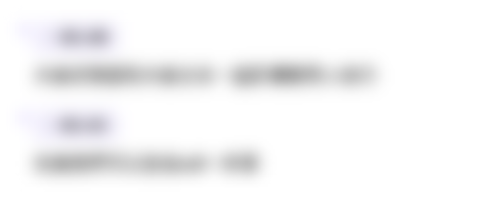
Dieser Bereich ist nur für Premium-Benutzer verfügbar. Bitte führen Sie ein Upgrade durch, um auf diesen Abschnitt zuzugreifen.
Upgrade durchführenWeitere ähnliche Videos ansehen
5.0 / 5 (0 votes)