What is a hypothesis test? A beginner's guide to hypothesis testing!
Summary
TLDRThis video explains hypothesis testing, a statistical method for determining if there is sufficient evidence in a sample to make inferences about a population. It distinguishes between populations and samples, outlines the steps involved in hypothesis testing, and clarifies the concepts of null and alternative hypotheses. Key components include calculating the P-value to assess statistical significance and recognizing Type I and Type II errors. The video also highlights tools for selecting and interpreting appropriate hypothesis tests, enhancing the viewer's understanding of data analysis.
Takeaways
- 😀 Hypothesis testing helps determine if there is enough evidence in a sample to infer a condition about a population.
- 👥 The population is the entire group of interest, while the sample is a smaller subset used for analysis.
- 📝 A hypothesis is a statement we test about a population parameter, such as the effect of a drug on blood pressure.
- 🔄 The null hypothesis (H0) assumes no effect, while the alternative hypothesis (H1) suggests there is an effect.
- 📊 To conduct a hypothesis test, researchers collect sample data and calculate how it deviates from the null hypothesis.
- 📉 The P-value indicates the probability of obtaining the sample results under the null hypothesis; a low P-value suggests significant evidence against H0.
- ✅ If the P-value is less than a predetermined threshold (commonly 0.05), the null hypothesis is rejected, indicating statistical significance.
- ⚖️ There are two types of errors in hypothesis testing: Type I error (false positive) occurs when the null hypothesis is wrongly rejected, and Type II error (false negative) occurs when it is not rejected when it should be.
- 🔧 Data analysis tools can help determine the appropriate hypothesis test and interpret the results efficiently.
- 📈 Understanding both parametric and nonparametric tests is crucial for selecting the right method for hypothesis testing.
Q & A
What is the purpose of hypothesis testing?
-Hypothesis testing is used to determine whether there is enough evidence in a sample of data to infer that a certain condition is true for the entire population.
What distinguishes a population from a sample?
-The population is the whole group of interest (e.g., all adults in the U.S.), while a sample is a smaller group selected from that population for analysis (e.g., 150 adults).
What are the two types of hypotheses involved in hypothesis testing?
-The two types of hypotheses are the null hypothesis (H0), which assumes no effect or difference, and the alternative hypothesis (H1), which is what you want to prove.
What is a P-value in hypothesis testing?
-The P-value indicates the probability of observing the sample data, or something more extreme, assuming the null hypothesis is true.
How do you determine whether to reject the null hypothesis?
-You compare the P-value to a predetermined significance level (commonly set at 0.05). If the P-value is less than this threshold, you reject the null hypothesis.
What does a statistically significant result imply?
-A statistically significant result implies that the observed data is unlikely to have occurred by chance, providing evidence against the null hypothesis.
What are Type I and Type II errors in hypothesis testing?
-A Type I error occurs when a true null hypothesis is rejected (false positive), while a Type II error occurs when a false null hypothesis is not rejected (false negative).
How can you find a suitable hypothesis test for your data?
-You can use data analysis tools (like data.net) that suggest appropriate tests based on your data, such as Chi-square or ANOVA, and provide interpretations of the results.
What is the significance of the predetermined threshold in hypothesis testing?
-The predetermined threshold, often set at 0.05, helps researchers decide whether the evidence is strong enough to reject the null hypothesis.
Can a large P-value prove that the null hypothesis is true?
-No, a large P-value does not prove that the null hypothesis is true; it only suggests that the observed data is consistent with the null hypothesis.
Outlines
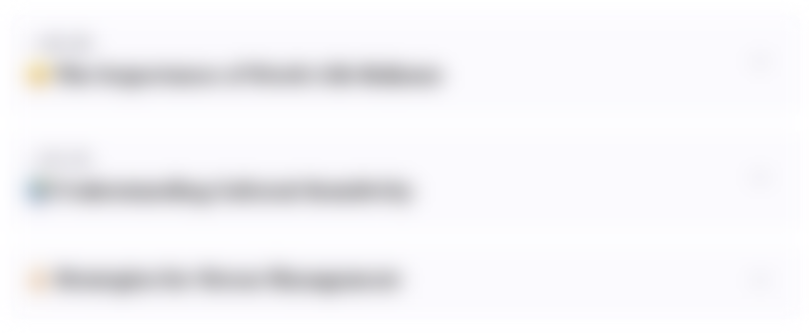
Dieser Bereich ist nur für Premium-Benutzer verfügbar. Bitte führen Sie ein Upgrade durch, um auf diesen Abschnitt zuzugreifen.
Upgrade durchführenMindmap
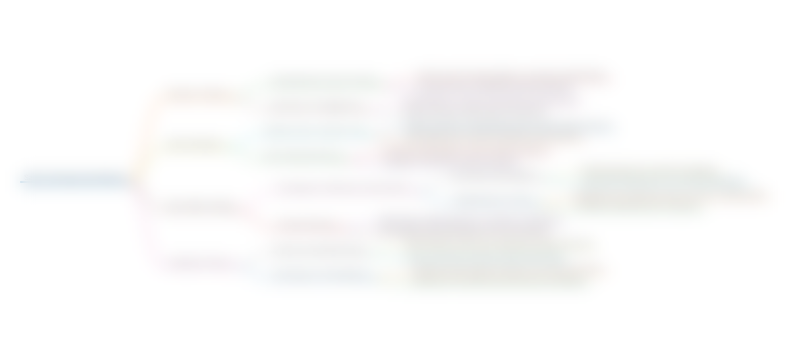
Dieser Bereich ist nur für Premium-Benutzer verfügbar. Bitte führen Sie ein Upgrade durch, um auf diesen Abschnitt zuzugreifen.
Upgrade durchführenKeywords
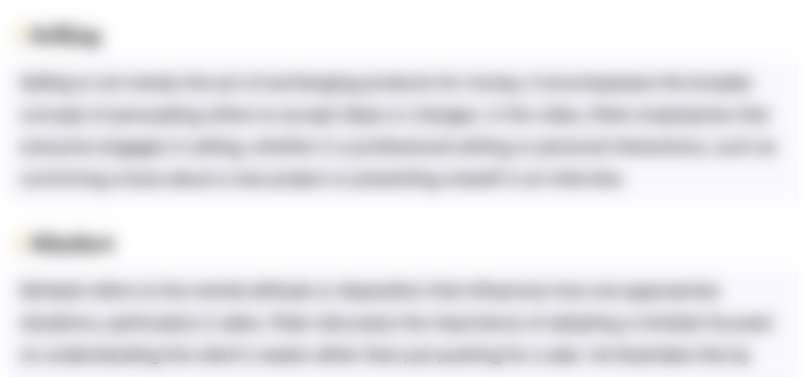
Dieser Bereich ist nur für Premium-Benutzer verfügbar. Bitte führen Sie ein Upgrade durch, um auf diesen Abschnitt zuzugreifen.
Upgrade durchführenHighlights
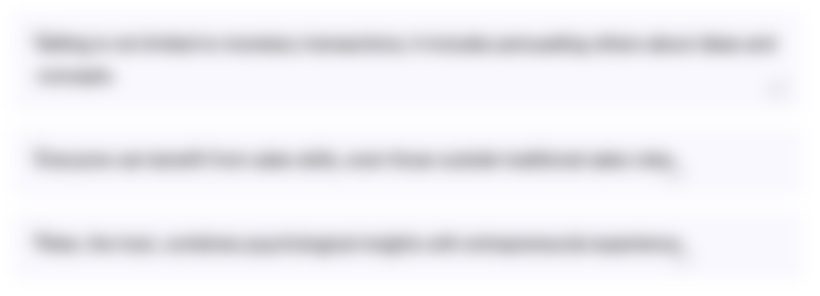
Dieser Bereich ist nur für Premium-Benutzer verfügbar. Bitte führen Sie ein Upgrade durch, um auf diesen Abschnitt zuzugreifen.
Upgrade durchführenTranscripts
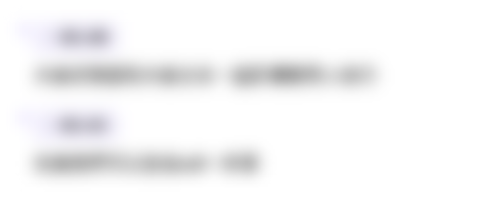
Dieser Bereich ist nur für Premium-Benutzer verfügbar. Bitte führen Sie ein Upgrade durch, um auf diesen Abschnitt zuzugreifen.
Upgrade durchführen5.0 / 5 (0 votes)