5-Langchain Series-Advanced RAG Q&A Chatbot With Chain And Retrievers Using Langchain
Summary
TLDRIn this video, Krishak walks viewers through building an advanced RAG (Retrieval-Augmented Generation) pipeline using LangChain's Retriever and Chain concepts. He shares a personal anecdote before diving into the technical aspects, explaining how to retrieve documents and utilize large language models (LLMs) to generate responses based on context. Krishak demonstrates step-by-step how to set up vector stores, split documents, create a Q&A chatbot, and integrate LLMs like LLaMA 2, showcasing the practical application of chains and retrievers for building an intelligent pipeline.
Takeaways
- 🏸 Krishak starts by sharing a funny incident from his badminton routine, where a neighbor recognized him by his shoes but noticed his new look.
- 💡 The video continues the Langchain series, focusing on developing an advanced RAG (Retriever-Augmented Generation) pipeline using retrievers and chain concepts.
- 📄 The initial tutorial discussed creating a simple RAG pipeline with data sources like PDFs and websites, loading and transforming the data into vector stores.
- 🧩 The next step involves improving query efficiency by incorporating large language models (LLMs) and chaining techniques to retrieve more accurate results.
- 🔗 Krishak explains the concept of chains, particularly the 'stuff document chain,' which formats documents into a prompt and passes them to the LLM.
- 🛠️ The practical example demonstrates how to split a document into chunks, convert it into vectors, and store it in a vector store using OpenAI embeddings.
- 🔍 The 'retriever' is introduced as an interface for extracting relevant documents from the vector store based on a user query.
- 🤖 Krishak integrates the retriever with the LLM and the document chain to create a Q&A chatbot that can generate context-based answers.
- 📝 He emphasizes the customization potential of the system, allowing it to work with open-source LLMs like Llama 2 for users without paid access.
- 🚀 The tutorial concludes by showing how combining retrievers, chains, and LLMs creates a more advanced RAG pipeline for efficient document retrieval and query answering.
Q & A
What is the primary focus of this video?
-The primary focus of this video is on developing an advanced RAG (Retrieval-Augmented Generation) pipeline using LangChain, specifically employing Retriever and Chain concepts along with LLMs (Large Language Models).
What was the funny incident that the speaker shared?
-The speaker shared a funny incident about how one of his neighbors identified him just by his shoes after a badminton session, noting that his new low-maintenance look had completely changed his appearance.
What are the main steps in developing the RAG pipeline as described in the video?
-The steps include: 1) Loading documents like PDFs and websites, 2) Breaking down large documents into chunks, 3) Converting those chunks into vectors and storing them in a vector store, 4) Using an LLM with Retriever and Chain concepts to retrieve information and generate a response based on a prompt.
What models can be used in this RAG pipeline?
-The video discusses using both open-source and paid LLM models, such as OpenAI embeddings and LLaMA 2 from Meta. Users can choose between these models depending on their needs and resources.
What is the purpose of using a prompt in this pipeline?
-The prompt is used to guide the LLM to answer a specific question based on the provided context. In the pipeline, the prompt helps format the documents from the vector store into a query that the LLM can use to generate a relevant response.
What is a 'stuff document chain' and how is it used?
-A 'stuff document chain' is a sequence of operations that formats a list of documents into a prompt and passes it to the LLM. It helps combine the documents and the user's query into a format that the LLM can process, allowing for a more coherent response.
How does the retriever function within the pipeline?
-The retriever is an interface connected to the vector store. When the user inputs a query, the retriever fetches the relevant documents from the vector store and passes them to the LLM to generate a response.
What is the role of the Vector Store in the pipeline?
-The Vector Store holds the vectorized representations of the document chunks. It allows for similarity-based searching when queries are made, and the retriever fetches data from it to provide relevant documents to the LLM for processing.
What is the advantage of using LLMs in this pipeline?
-LLMs enhance the RAG pipeline by providing more accurate and context-aware responses based on the documents retrieved from the vector store. LLMs can handle complex queries and generate more refined results compared to simple vector searches.
How can users customize their RAG pipeline based on their needs?
-Users can customize their RAG pipeline by choosing between different LLMs (open-source or paid), adjusting document chunk sizes, tweaking prompts, and using various LangChain functions like retrievers, stuff document chains, and vector stores.
Outlines
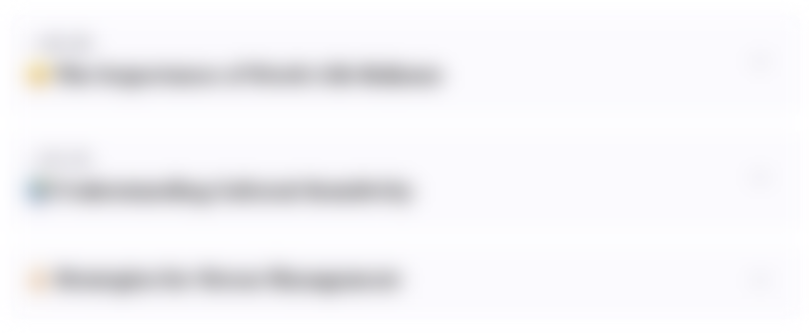
Dieser Bereich ist nur für Premium-Benutzer verfügbar. Bitte führen Sie ein Upgrade durch, um auf diesen Abschnitt zuzugreifen.
Upgrade durchführenMindmap
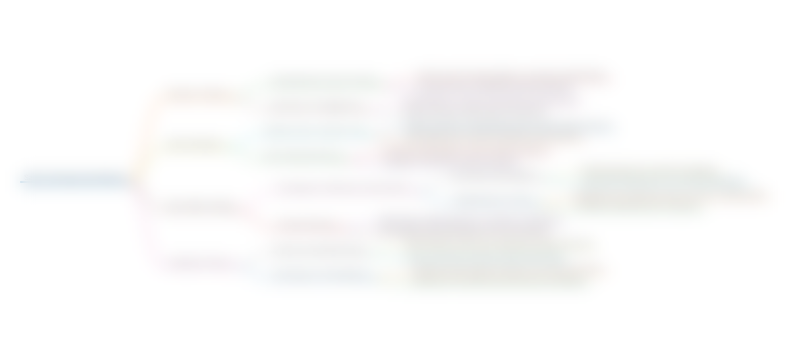
Dieser Bereich ist nur für Premium-Benutzer verfügbar. Bitte führen Sie ein Upgrade durch, um auf diesen Abschnitt zuzugreifen.
Upgrade durchführenKeywords
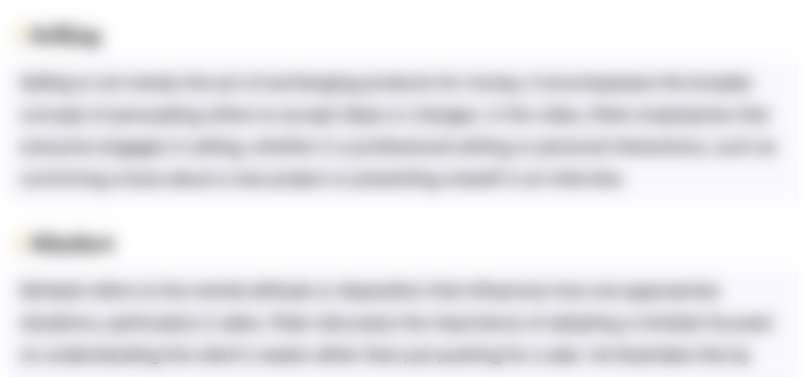
Dieser Bereich ist nur für Premium-Benutzer verfügbar. Bitte führen Sie ein Upgrade durch, um auf diesen Abschnitt zuzugreifen.
Upgrade durchführenHighlights
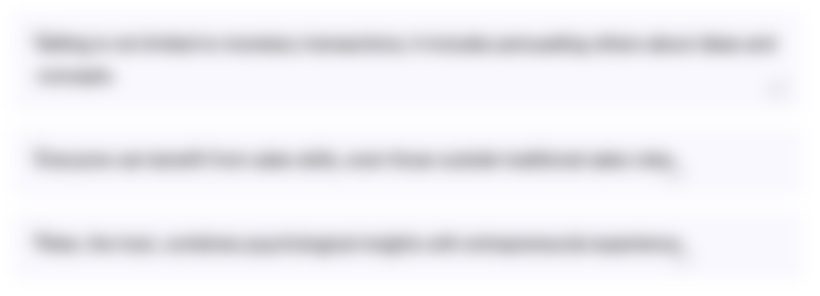
Dieser Bereich ist nur für Premium-Benutzer verfügbar. Bitte führen Sie ein Upgrade durch, um auf diesen Abschnitt zuzugreifen.
Upgrade durchführenTranscripts
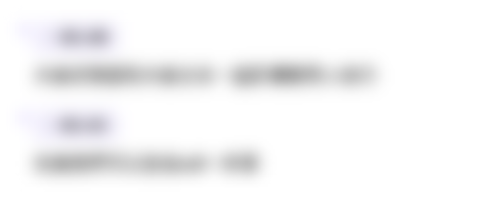
Dieser Bereich ist nur für Premium-Benutzer verfügbar. Bitte führen Sie ein Upgrade durch, um auf diesen Abschnitt zuzugreifen.
Upgrade durchführenWeitere ähnliche Videos ansehen
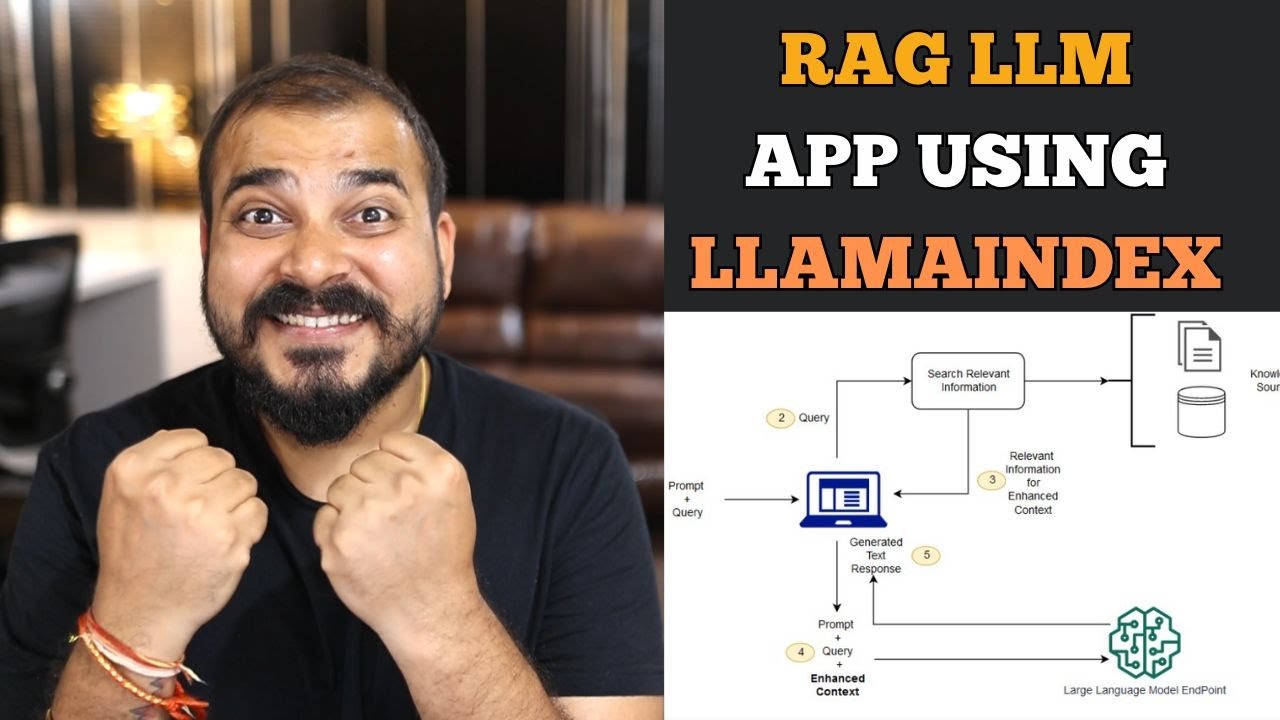
End to end RAG LLM App Using Llamaindex and OpenAI- Indexing and Querying Multiple pdf's

Building Production-Ready RAG Applications: Jerry Liu
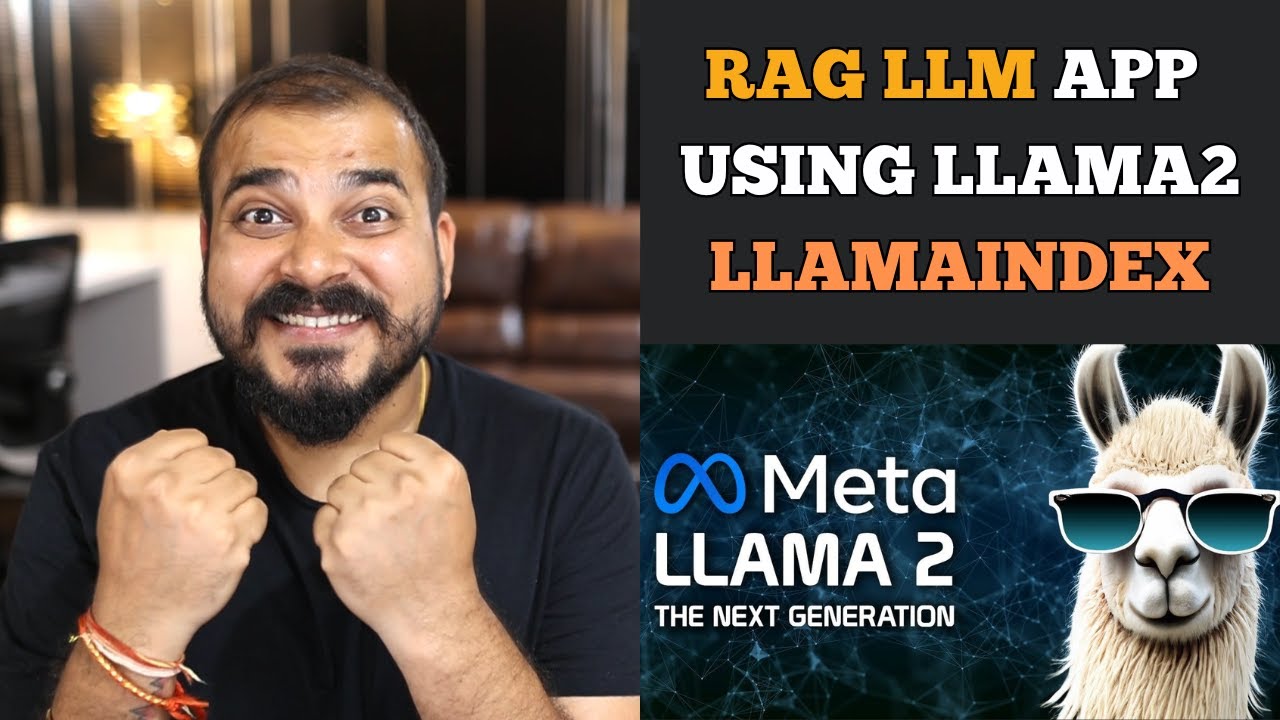
Step-by-Step Guide to Building a RAG LLM App with LLamA2 and LLaMAindex
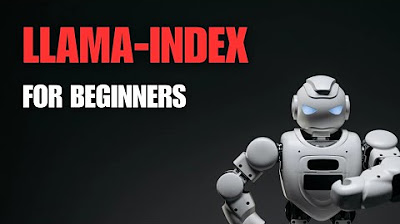
Llama-index for beginners tutorial
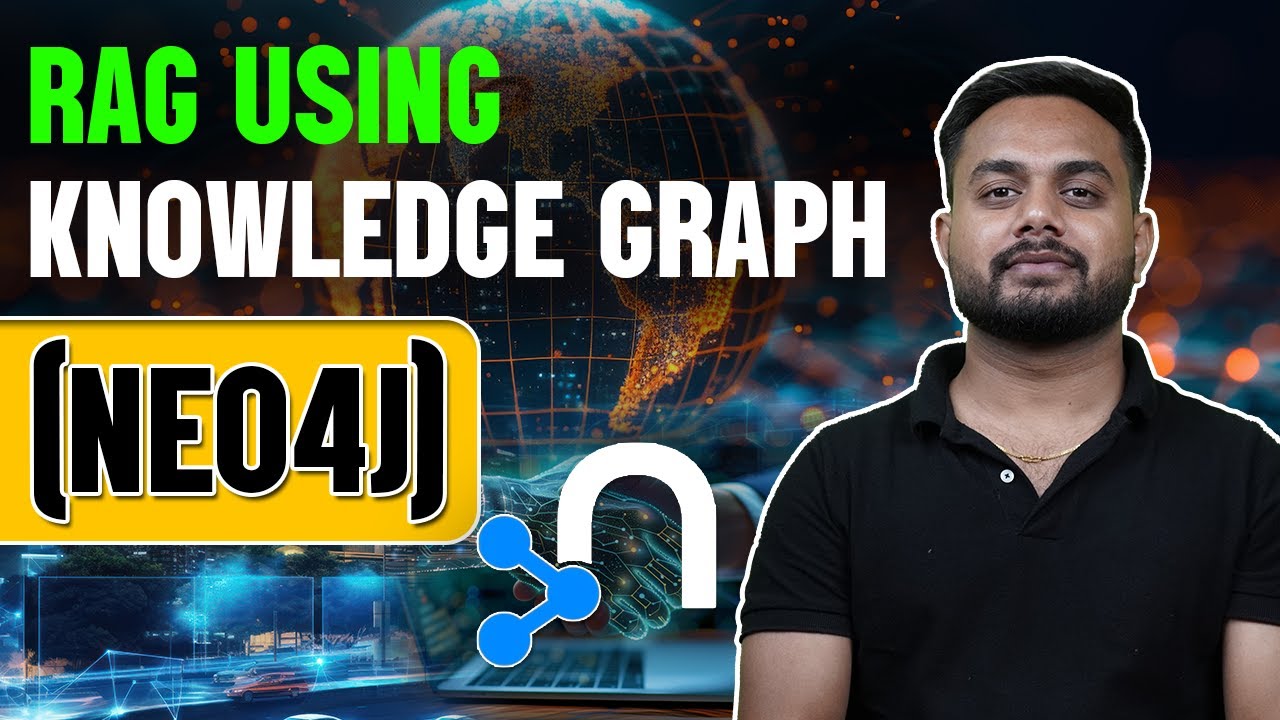
Realtime Powerful RAG Pipeline using Neo4j(Knowledge Graph Db) and Langchain #rag
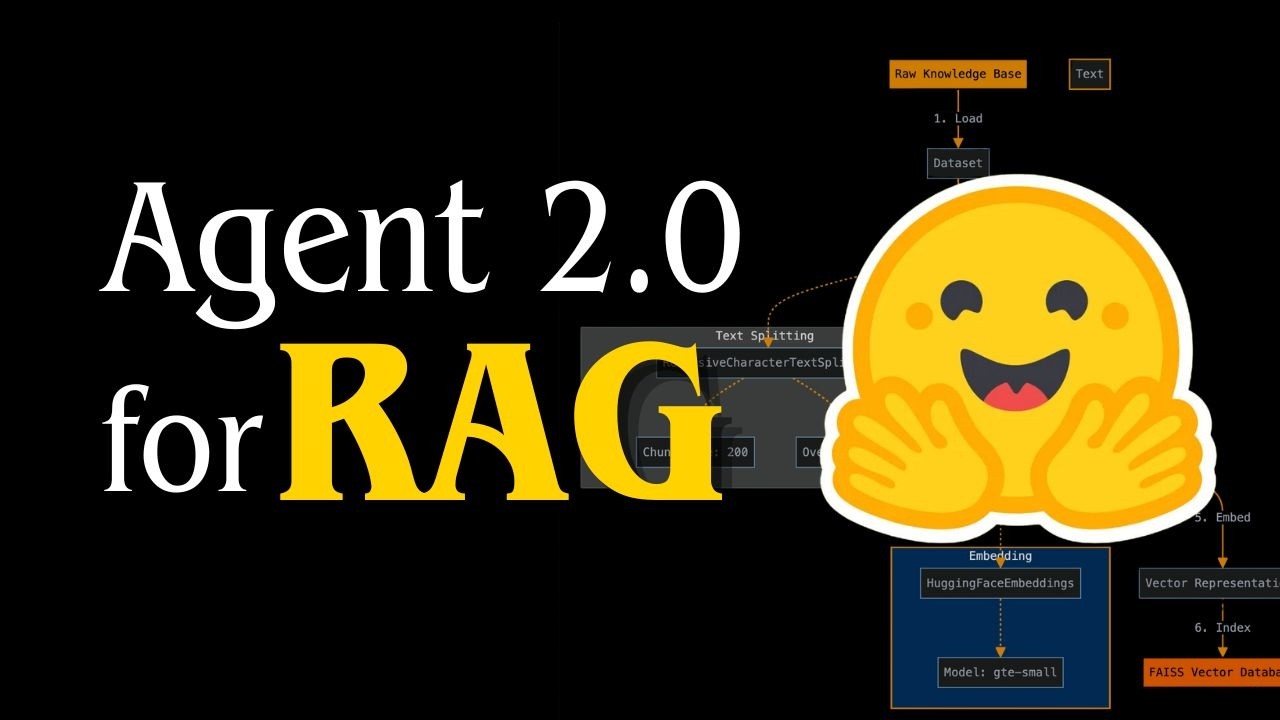
Agentic RAG: Make Chatting with Docs Smarter
5.0 / 5 (0 votes)