Step-by-Step Guide to Building a RAG LLM App with LLamA2 and LLaMAindex
Summary
TLDRIn this video, Krishak guides viewers through building a retrieval-augmented generation (RAG) system using open-source models like Llama 2. He covers the step-by-step process, from installing necessary libraries like PyPDF and Transformers to creating embeddings, indexing documents, and querying with Llama 2 via Hugging Face. The tutorial also introduces techniques like quantization for optimizing models in Google Colab, integrating LangChain, and using Hugging Face for embeddings. Krishak plans to explore more models like Mistral and Falcon in future videos, offering valuable insights for developers working with RAG systems.
Please replace the link and try again.
Please replace the link and try again.
Outlines
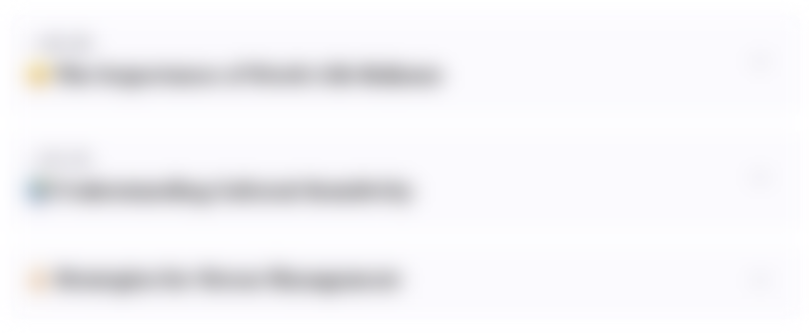
This section is available to paid users only. Please upgrade to access this part.
Upgrade NowMindmap
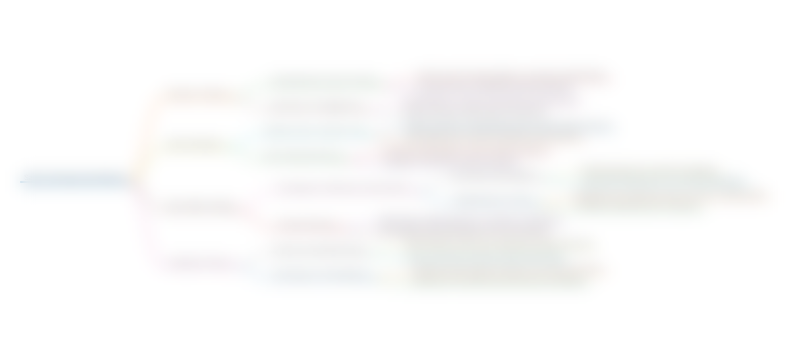
This section is available to paid users only. Please upgrade to access this part.
Upgrade NowKeywords
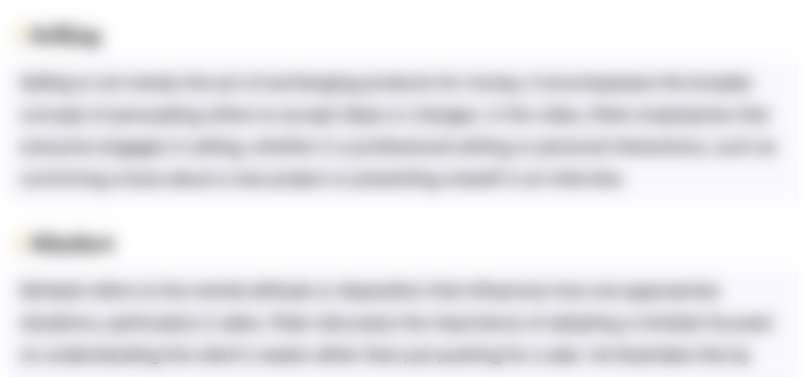
This section is available to paid users only. Please upgrade to access this part.
Upgrade NowHighlights
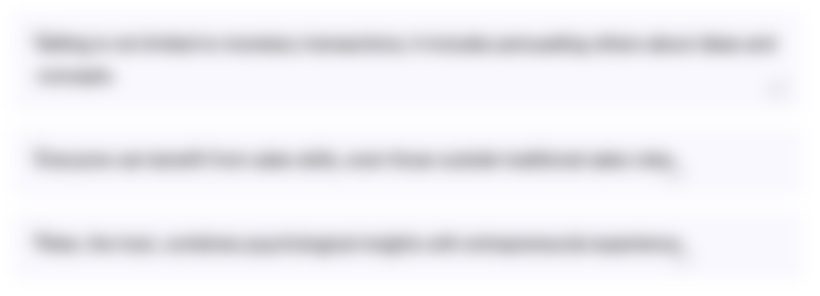
This section is available to paid users only. Please upgrade to access this part.
Upgrade NowTranscripts
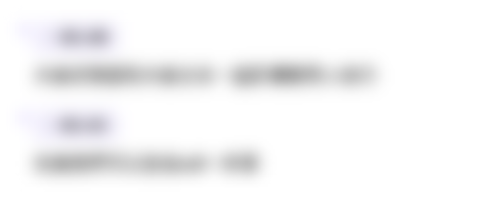
This section is available to paid users only. Please upgrade to access this part.
Upgrade NowBrowse More Related Video
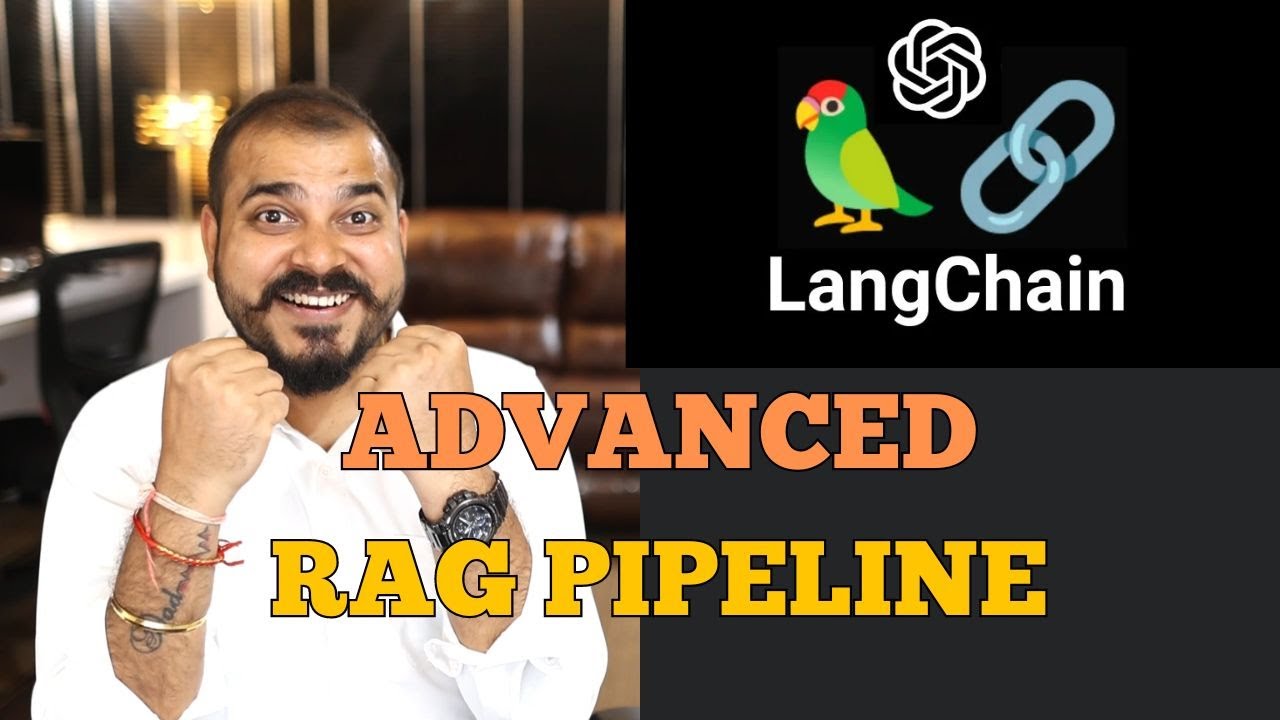
5-Langchain Series-Advanced RAG Q&A Chatbot With Chain And Retrievers Using Langchain
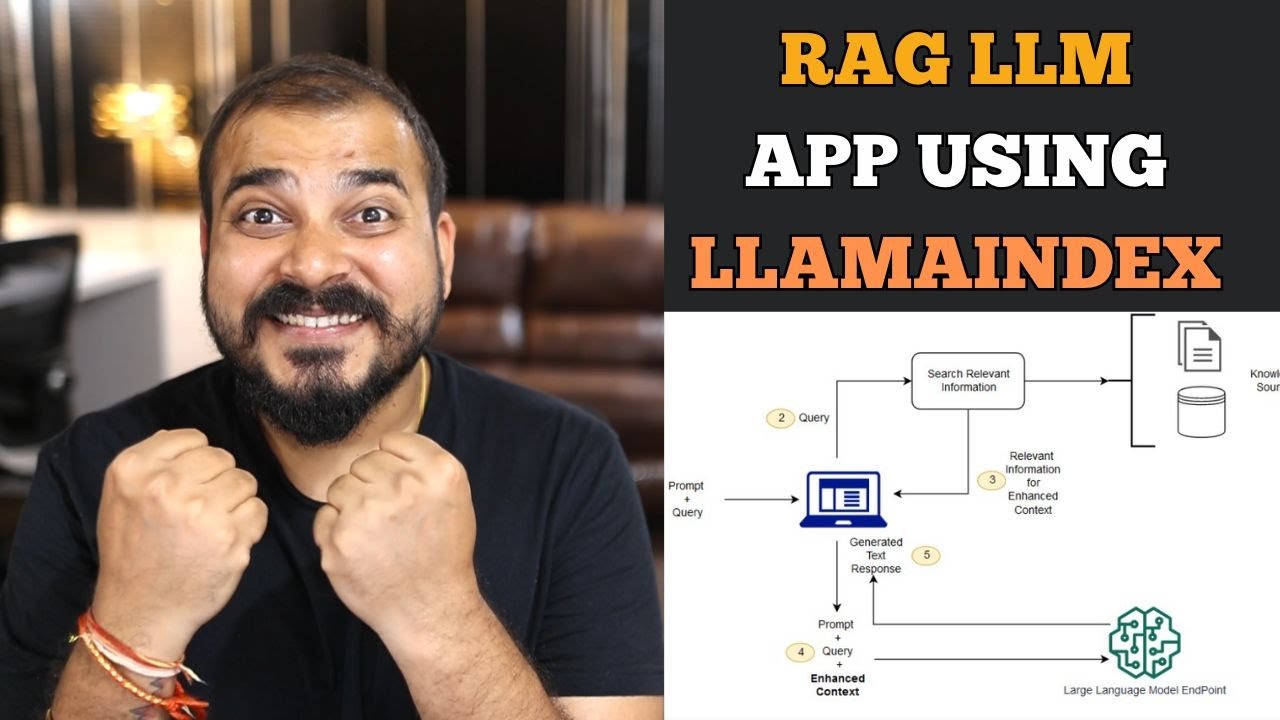
End to end RAG LLM App Using Llamaindex and OpenAI- Indexing and Querying Multiple pdf's
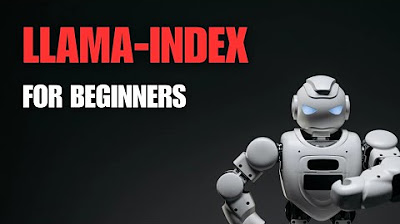
Llama-index for beginners tutorial
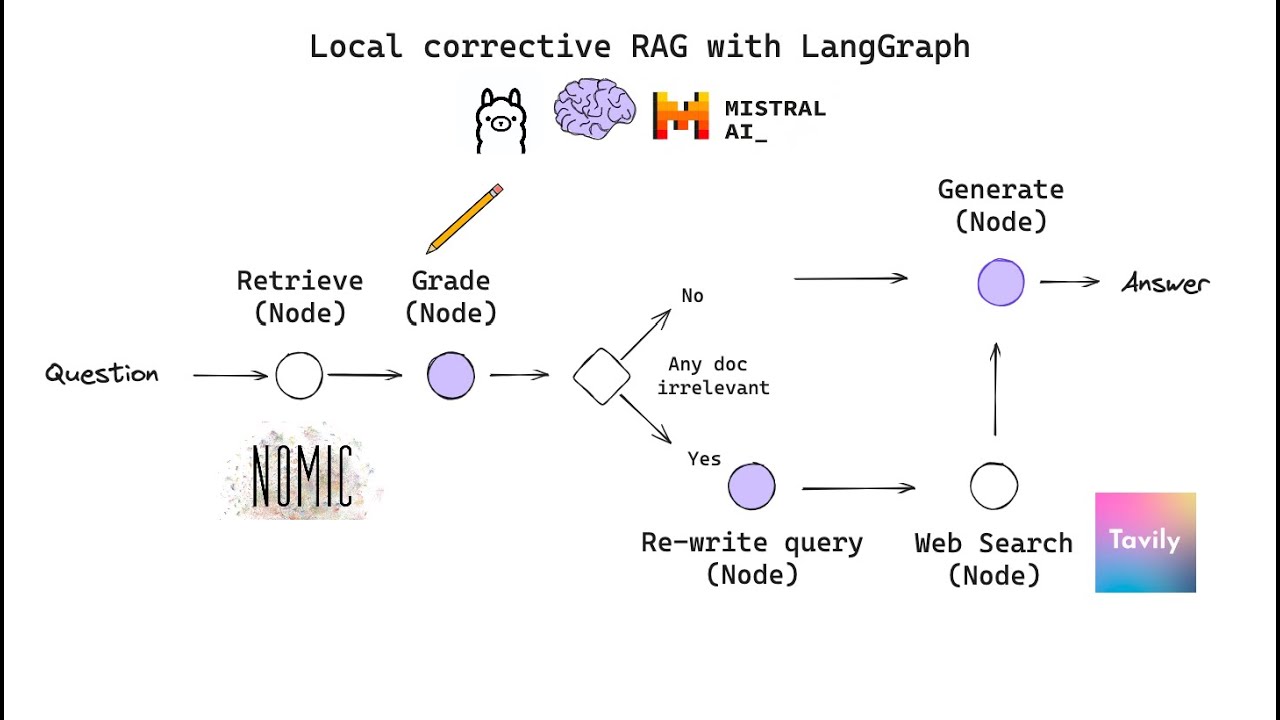
Building Corrective RAG from scratch with open-source, local LLMs
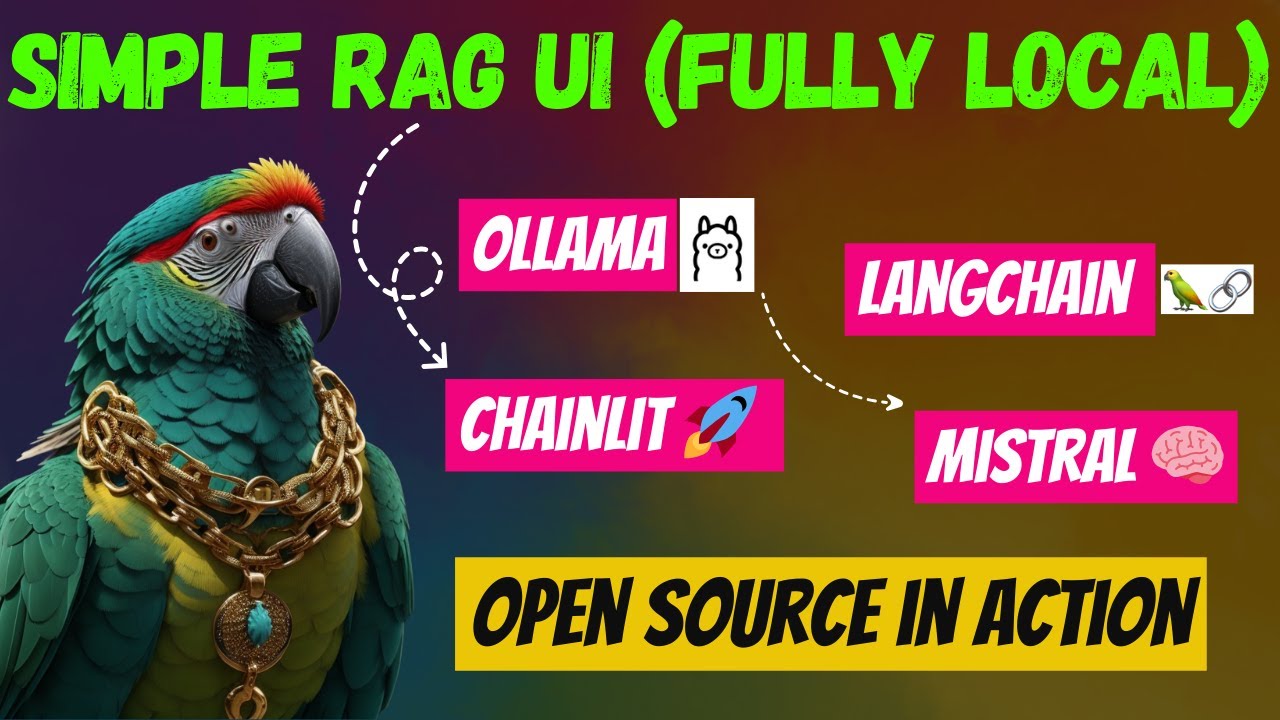
Chat With Documents Using ChainLit, LangChain, Ollama & Mistral 🧠

Unstructured” Open-Source ETL for LLMs
5.0 / 5 (0 votes)