Correlation Doesn't Equal Causation: Crash Course Statistics #8
Summary
TLDRIn this Crash Course Statistics episode, Adriene Hill explores data relationships, focusing on how one variable can predict another. She introduces scatter plots as a tool to visualize these relationships, highlighting their versatility in identifying both linear and nonlinear connections. Hill discusses the significance of regression lines in describing relationships and introduces the concept of correlation, explaining how it measures the direction and strength of the relationship between two variables. The episode emphasizes that correlation does not imply causation, warning against the common mistake of equating the two. It concludes by stressing the importance of understanding data relationships for prediction and reflection.
Takeaways
- 📊 Scatter plots are essential tools for visualizing relationships between two continuous variables, allowing us to observe patterns and clusters in data.
- 🔍 The regression line, introduced by Karl Pearson, is a method to describe the relationship between variables by fitting the line closest to all data points.
- ↗️ The slope (m) of the regression line indicates the change in the dependent variable (y) for each unit increase in the independent variable (x), providing a predictive measure.
- 🔢 The correlation coefficient (r) measures the strength and direction of the linear relationship between two variables, ranging from -1 (perfect negative correlation) to 1 (perfect positive correlation).
- 🔄 Positive correlation implies that as one variable increases, the other also tends to increase, while negative correlation suggests an inverse relationship.
- 📉 Correlation does not imply causation; just because two variables are correlated does not mean one causes the other to occur.
- 🌡️ The R-squared (r^2) value represents the proportion of the variance in the dependent variable that is predictable from the independent variable, with values ranging from 0 to 1.
- 🚫 It's crucial to be cautious of spurious correlations, which can occur by chance or due to a third, unobserved variable influencing both variables in question.
- 👀 Always examine scatter plots to understand the nature of the relationship between variables, as different datasets can have the same correlation coefficient but very different relationships.
- 🌟 Understanding correlations helps in making predictions and understanding past events, but it's important to consider the context and not jump to conclusions based solely on correlation.
Q & A
What is the main topic of discussion in this Crash Course Statistics video?
-The main topic of discussion is data relationships, specifically how one variable can be used to predict another, and the use of scatter plots to visualize these relationships.
What is a scatter plot and why is it useful in statistics?
-A scatter plot is a type of plot that displays data points on horizontal and vertical axes, typically with one variable on the x-axis and another on the y-axis. It is useful in statistics for visualizing the relationship between two continuous variables and identifying patterns, clusters, or trends.
What does the term 'bivariate data' refer to in the context of this video?
-Bivariate data refers to data that involves relationships between two continuous variables, which is the simplest form of data relationship discussed in the video.
How does the video illustrate the concept of linear relationships using the example of father and son heights?
-The video uses the example of father and son heights to illustrate linear relationships by fitting a regression line through the data points, which represents the average change in son's height for each unit increase in father's height.
What is the significance of the regression line in the context of this video?
-The regression line is significant because it is the line that is as close as possible to all the data points, representing the best fit for the data. It helps in predicting one variable based on the value of another and understanding the strength and direction of the relationship between variables.
What is the formula for a line in the context of a regression line, and what do the variables represent?
-The formula for a line in the context of a regression line is y = mx + b, where 'm' represents the slope (the change in y for each unit change in x), 'x' is the independent variable, 'y' is the dependent variable, and 'b' is the y-intercept (the value of y when x is 0).
How does the video explain the concept of correlation and its importance?
-The video explains that correlation measures the way two variables move together, indicating both the direction and the strength of their relationship. It is important because it provides insight into the nature of the relationship between variables, whether they move in the same direction (positive correlation) or opposite directions (negative correlation).
What is the correlation coefficient 'r' and what does it represent?
-The correlation coefficient 'r' is a measure that quantifies the strength and direction of the linear relationship between two variables. It ranges from -1 to 1, where 1 indicates a perfect positive correlation, -1 indicates a perfect negative correlation, and 0 indicates no linear correlation.
What is the difference between correlation and causation as discussed in the video?
-The video emphasizes that correlation does not equal causation. While correlation indicates a relationship between two variables, causation implies that one variable causes the other to change. The video warns against interpreting correlations as causations without considering other factors.
How does the video use the 'Cool-Cage Act' as an example to illustrate the misunderstanding of correlation?
-The 'Cool-Cage Act' is a satirical example used in the video to show how correlations can be misinterpreted. The act proposes to reduce drownings by limiting air conditioning sales and preventing Nicolas Cage from starring in movies, based on the observed correlations with drownings. The video points out that these correlations are likely due to a third variable (heat) and do not imply causation.
What is the significance of R^2 in the context of this video, and how does it relate to prediction?
-R^2, or the coefficient of determination, represents the proportion of the variance in one variable that is predictable from the other variable. It is always between 0 and 1, and a higher R^2 value indicates a better fit of the model and a more accurate prediction of one variable based on the other.
Outlines
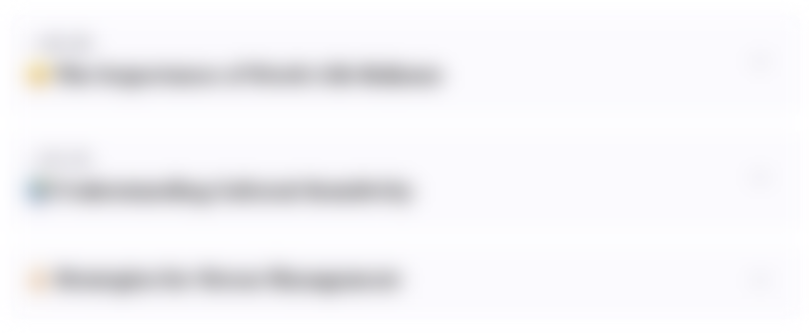
Dieser Bereich ist nur für Premium-Benutzer verfügbar. Bitte führen Sie ein Upgrade durch, um auf diesen Abschnitt zuzugreifen.
Upgrade durchführenMindmap
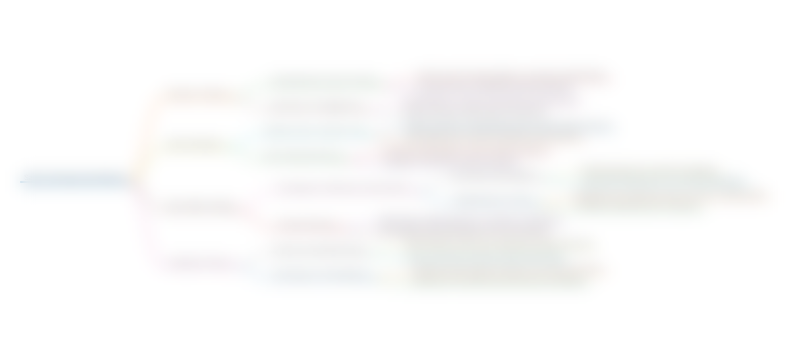
Dieser Bereich ist nur für Premium-Benutzer verfügbar. Bitte führen Sie ein Upgrade durch, um auf diesen Abschnitt zuzugreifen.
Upgrade durchführenKeywords
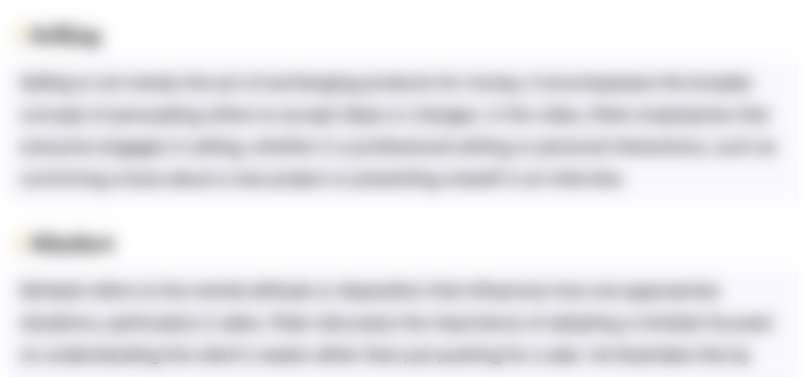
Dieser Bereich ist nur für Premium-Benutzer verfügbar. Bitte führen Sie ein Upgrade durch, um auf diesen Abschnitt zuzugreifen.
Upgrade durchführenHighlights
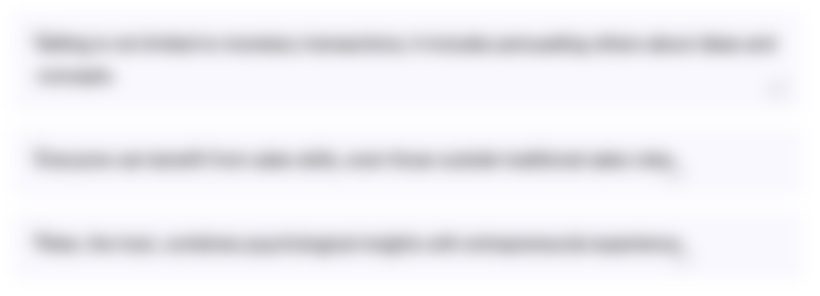
Dieser Bereich ist nur für Premium-Benutzer verfügbar. Bitte führen Sie ein Upgrade durch, um auf diesen Abschnitt zuzugreifen.
Upgrade durchführenTranscripts
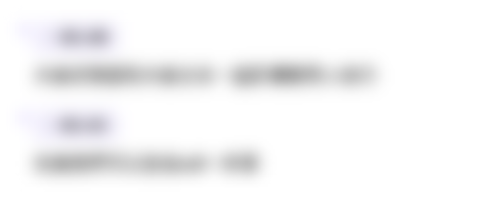
Dieser Bereich ist nur für Premium-Benutzer verfügbar. Bitte führen Sie ein Upgrade durch, um auf diesen Abschnitt zuzugreifen.
Upgrade durchführenWeitere ähnliche Videos ansehen
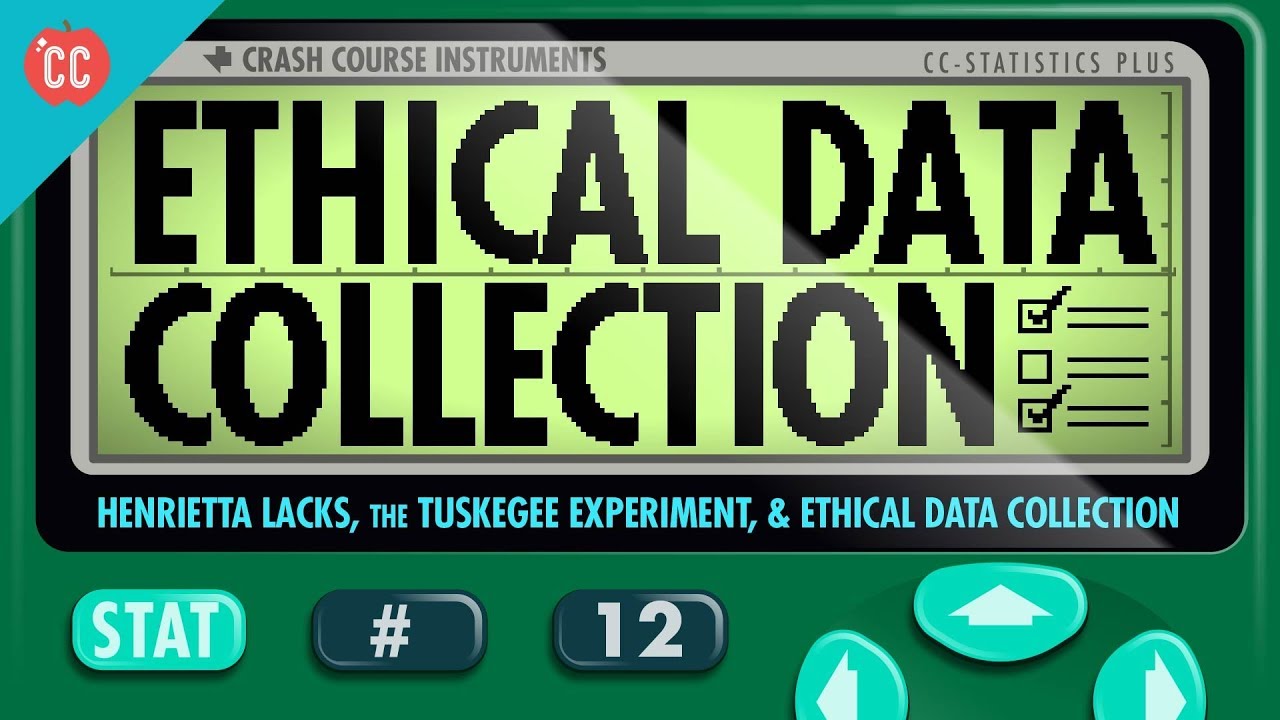
Henrietta Lacks, the Tuskegee Experiment, and Ethical Data Collection: Crash Course Statistics #12

Measures of Spread: Crash Course Statistics #4
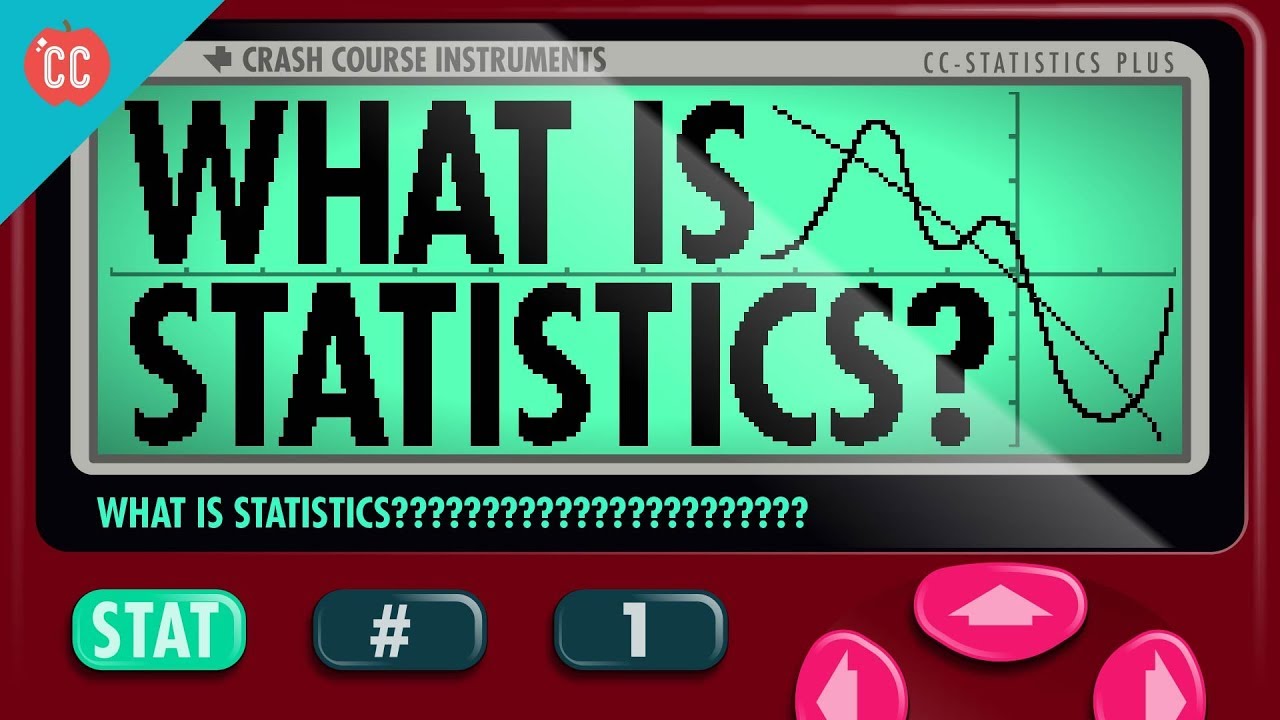
What Is Statistics: Crash Course Statistics #1
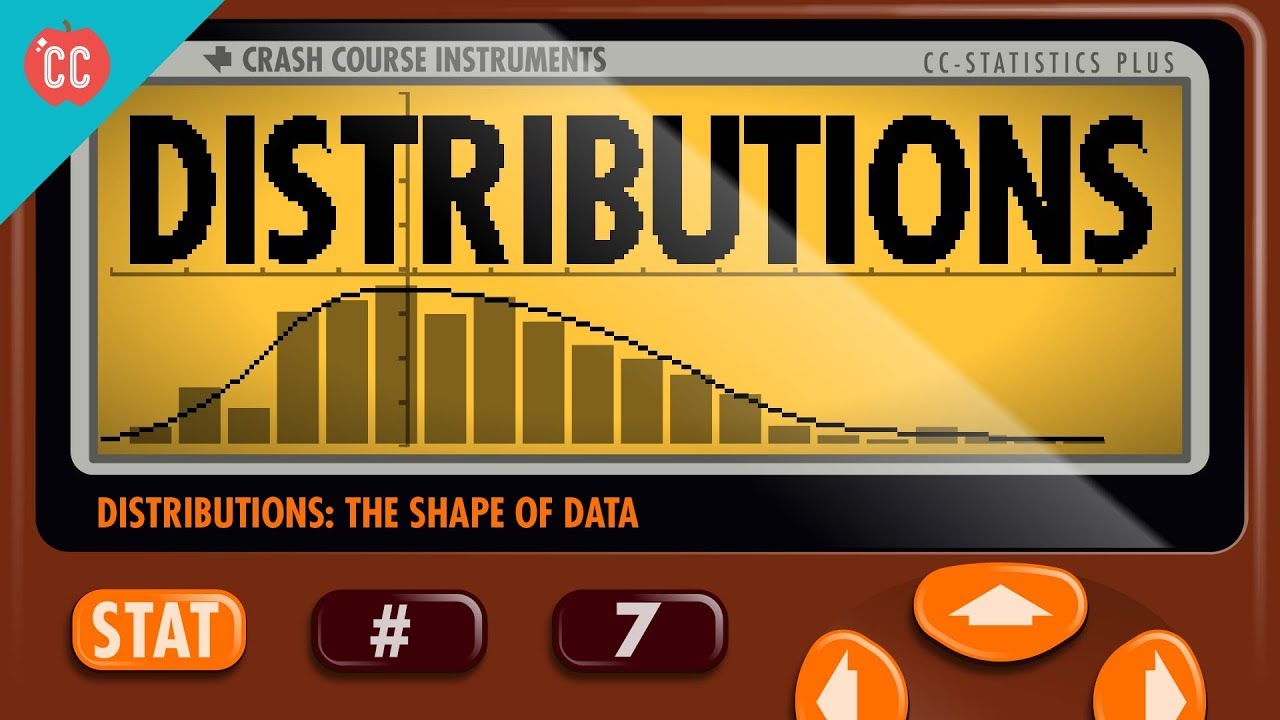
The Shape of Data: Distributions: Crash Course Statistics #7
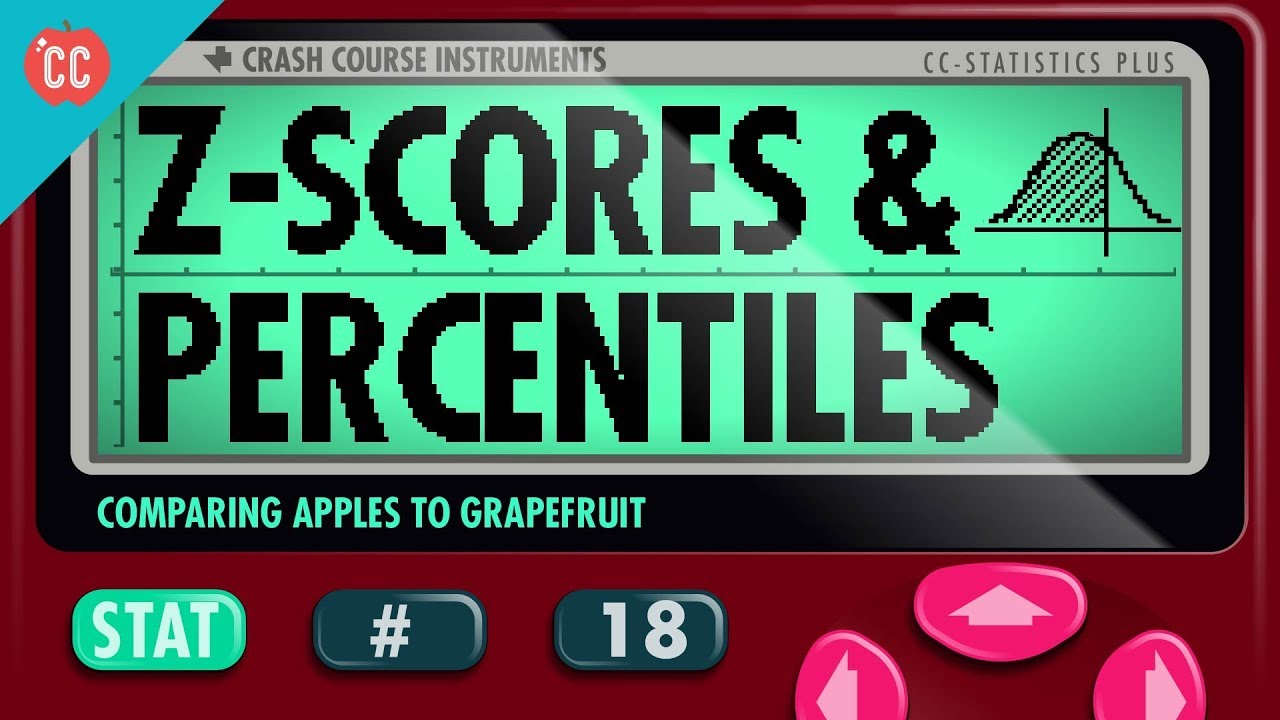
Z-Scores and Percentiles: Crash Course Statistics #18
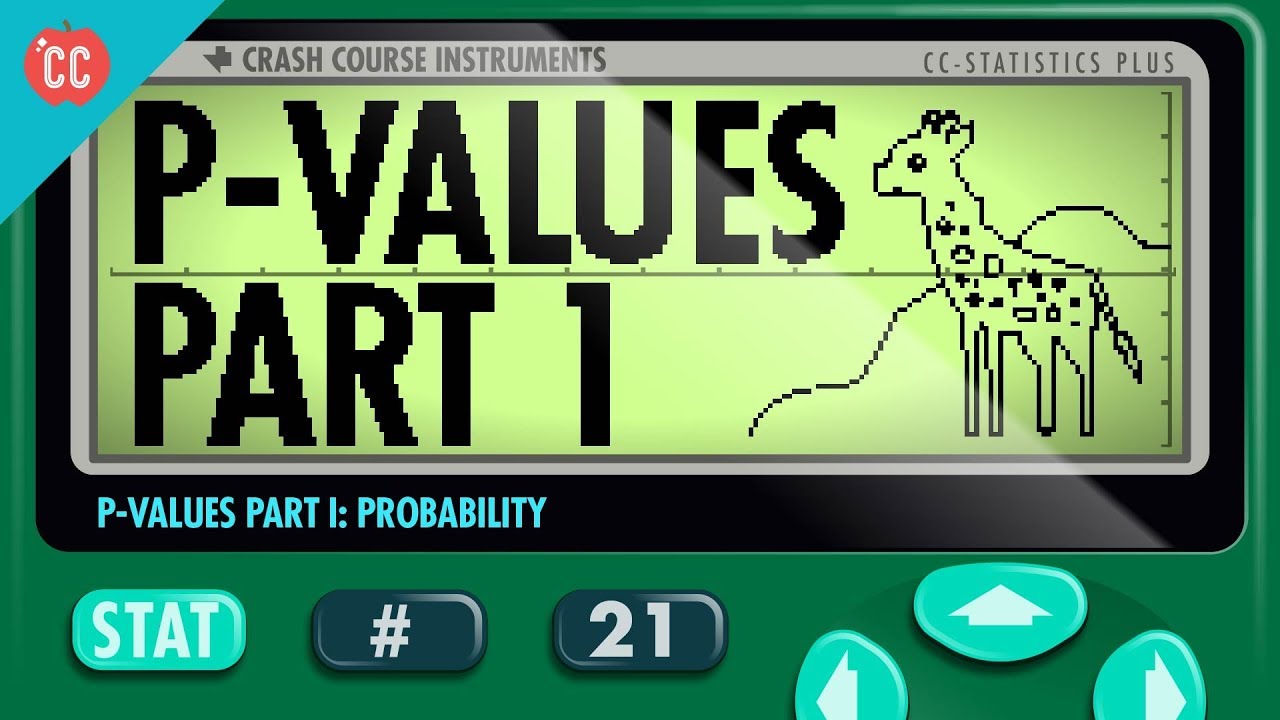
How P-Values Help Us Test Hypotheses: Crash Course Statistics #21
5.0 / 5 (0 votes)