我们采访了苹果芯片背后的男人——Johny Srouji!
Summary
TLDR在这段访谈中,苹果公司的Johnny Park讨论了iPhone 16和16 Pro系列的A18和A18 Pro芯片如何支持苹果智能技术。他强调了苹果智能技术对芯片设计的影响,以及8GB RAM在提升用户体验中的重要性。此外,他还提到了苹果在CPU核心数量、微架构设计和热管理方面的策略,以及苹果芯片在提升iPhone摄影能力方面的作用。
Takeaways
- 📱 iPhone 16和iPhone 16 Pro系列是首批搭载苹果智能技术的iPhone,A18和A18 Pro芯片在运行苹果智能技术方面发挥了重要作用。
- 🚀 A18和A18 Pro芯片在设计时就考虑了苹果智能技术,通过增强神经引擎、增加系统内存带宽和容量来提升整体性能。
- 💾 去年iPhone 15 Pro升级到8GB RAM,iPhone 16系列也继续这一配置,这不仅是为了支持苹果智能技术,也为了满足如高端游戏等其他应用的需求。
- 🎮 苹果智能技术是推动iPhone升级到8GB RAM的一个重要因素,但8GB RAM也将极大地促进其他应用的性能,包括高端游戏。
- 🤖 苹果的设计理念是构建统一的CPU架构,无论是手机、iPad还是Mac,以便于软件开发者能够针对单一架构进行开发。
- 🏋️♂️ 苹果的CPU设计注重单线程性能和能效,而不是简单地增加核心数量来追求峰值性能。
- 🔧 在决定CPU微架构时,苹果会根据产品需求、电池大小、电源系统和热环境等因素进行综合考虑。
- 🎮 苹果的GPU架构在iPhone和Mac之间保持一致,但会根据不同产品的特点进行实施上的差异化,以保持能效。
- 📹 iPhone 16系列在热管理方面进行了改进,以支持更强大的性能,同时保持了产品的轻薄设计。
- 🎥 苹果的图像信号处理器(ISP)和视频编码器的紧密集成,使得iPhone能够提供Dolby Vision 4K 120fps的视频拍摄能力。
Q & A
苹果A18和A18 Pro芯片如何支持运行苹果智能技术?
-A18和A18 Pro芯片通过持续改进神经引擎,增加系统内存带宽,并在整体性能上进行优化,从而支持苹果智能技术。这些芯片从设计之初就考虑了苹果智能技术的需求。
苹果智能技术对苹果硅芯片设计有何影响?
-苹果智能技术是苹果硅芯片设计中的一个重要考虑因素,特别是在决定内存容量和带宽时。苹果智能技术是推动iPhone 16 Pro系列升级到8GB RAM的关键因素之一。
为什么iPhone 16系列继续使用2个性能核心和4个效率核心的CPU配置?
-苹果的设计理念是提供最佳的用户体验,而不是追求特定的峰值性能。苹果的CPU在单线程性能和能效方面都是行业领先的,因此2个性能核心和4个效率核心的配置足以满足iPhone的需求。
苹果M4芯片相比A18系列在核心数量上有所增加,这是基于什么考虑?
-苹果M4芯片用于iPad等产品,这些设备有更大的散热空间和不同的电源供应,因此可以支持更高的频率和性能。苹果通过模拟和性能建模工具来决定不同产品的最佳核心配置。
苹果在CPU微架构设计上是如何做出决策的?
-苹果的决策基于提供最佳用户体验的原则,通过大量的数据和性能建模工具来确定最佳的CPU配置。苹果不会为了某个基准测试而设计产品,而是专注于整体的能效和性能。
苹果如何在iPhone上实现高端游戏的性能优化?
-苹果通过提供相似的GPU架构在不同设备上实现高性能,同时在实施层面进行调整,以适应不同的电源供应和散热限制。苹果的GPU设计注重能效,以确保在移动设备上也能提供持续的高性能。
苹果在iPhone 16系列中对散热管理做了哪些改进?
-苹果在iPhone 16系列中进行了多项散热改进,以提供更好的产品体验。这些改进包括使用新材料和优化组件布局,同时保持设备的轻薄设计。
苹果硅芯片在iPhone的视频拍摄能力中扮演了什么角色?
-苹果硅芯片中的图像信号处理器(ISP)和视频编码器在视频拍摄中发挥了关键作用。ISP负责处理图像的色彩和色调,而视频编码器则以高速度压缩视频,使得iPhone能够支持杜比视界4K 120帧每秒的视频录制。
苹果如何在不同设备上保持GPU架构的一致性,同时又满足各自的特点?
-苹果通过在不同设备上使用相似的GPU架构,同时在实施层面进行调整,如核心数量和频率,来保持一致性。这样的设计使得开发者可以更容易地将游戏和应用移植到不同的苹果设备上。
苹果如何决定在iPhone中使用特定的散热解决方案?
-苹果在决定散热解决方案时,会考虑多种因素,包括材料、设备厚度、电池空间和其他组件的布局。苹果的目标是提供最佳的产品体验,而不是单纯追求某个方面的性能。
Outlines
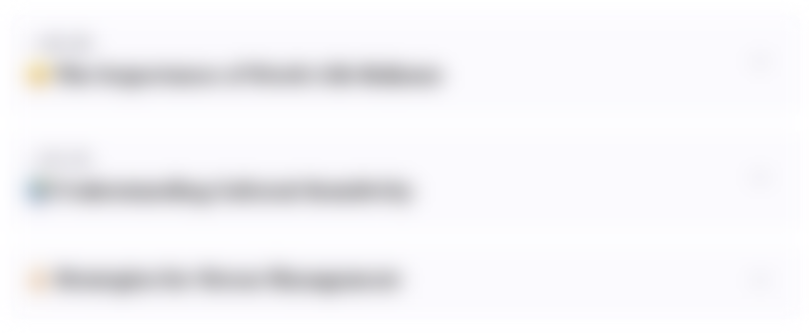
Dieser Bereich ist nur für Premium-Benutzer verfügbar. Bitte führen Sie ein Upgrade durch, um auf diesen Abschnitt zuzugreifen.
Upgrade durchführenMindmap
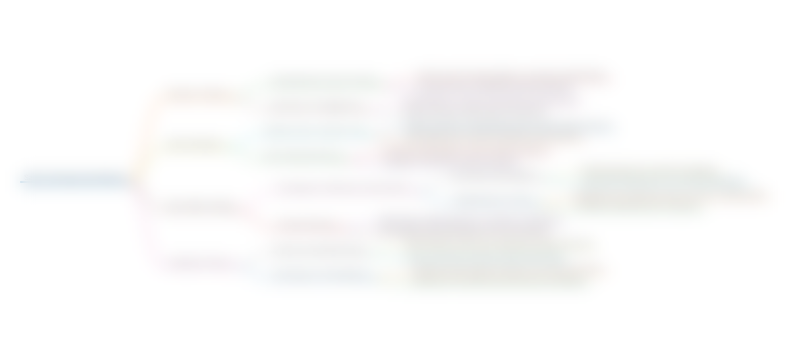
Dieser Bereich ist nur für Premium-Benutzer verfügbar. Bitte führen Sie ein Upgrade durch, um auf diesen Abschnitt zuzugreifen.
Upgrade durchführenKeywords
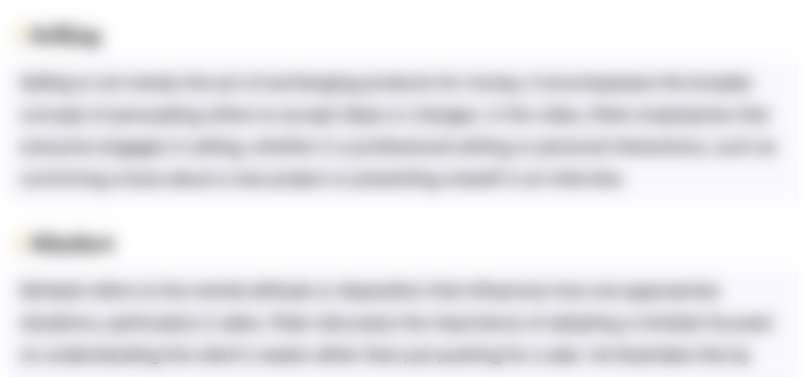
Dieser Bereich ist nur für Premium-Benutzer verfügbar. Bitte führen Sie ein Upgrade durch, um auf diesen Abschnitt zuzugreifen.
Upgrade durchführenHighlights
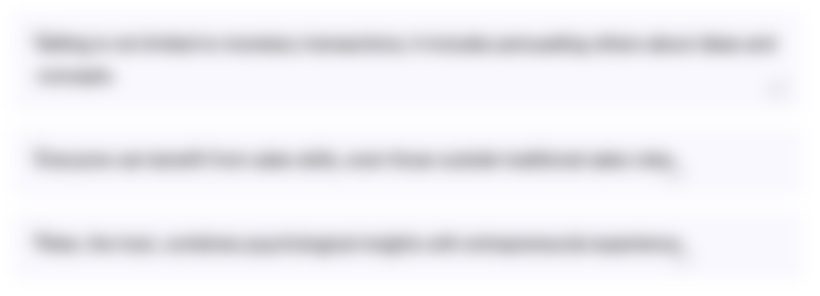
Dieser Bereich ist nur für Premium-Benutzer verfügbar. Bitte führen Sie ein Upgrade durch, um auf diesen Abschnitt zuzugreifen.
Upgrade durchführenTranscripts
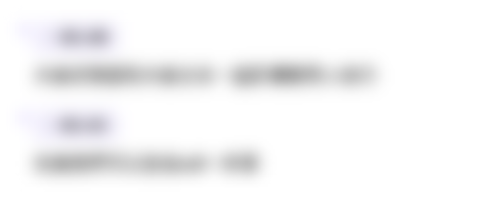
Dieser Bereich ist nur für Premium-Benutzer verfügbar. Bitte führen Sie ein Upgrade durch, um auf diesen Abschnitt zuzugreifen.
Upgrade durchführenWeitere ähnliche Videos ansehen
5.0 / 5 (0 votes)