Introduction to Pure Storage FlashBlade with Par Botes
Summary
TLDRThe presentation covers the development of FlashBlade, a scalable storage solution designed for growing datasets in fields like media, analytics, and technical computing. It highlights the challenges of handling large amounts of metadata, data reduction, and energy efficiency. The solution's design allows for flexibility in storage interfaces, with an emphasis on simplicity, performance, and scalability. The product targets industries with emerging use cases like machine learning, genomics, and financial modeling, offering high-speed, energy-efficient storage for complex data interactions. The session also discusses customer use cases and the strategic vision for future development.
Takeaways
- 😀 Flash Blade is a scalable storage product designed for modern data sets, including scientific data, log data, and more, that have different requirements than traditional storage systems.
- 😀 The product features an NFS interface at launch, with plans for an object interface later in the year, allowing for flexibility in how data is accessed.
- 😀 Flash Blade was designed to handle large datasets that are growing exponentially and require fast, concurrent access by machines, not humans.
- 😀 A major focus during development was creating a storage system that could start small and scale out easily, maintaining performance as data volumes grow.
- 😀 The system is optimized for simplicity, offering easy upgrades, minimal setup, and a self-balancing system with instant capacity availability.
- 😀 The initial Flash Blade design offers blades with 1 terabyte of storage, scaling up to 52 terabytes per blade, with six times the storage capacity compared to earlier designs.
- 😀 The system delivers impressive throughput of about 1 gigabyte per second per blade, demonstrating solid performance in real-world workloads.
- 😀 Real customer data shows a compression rate of around 3:1 to 3.5:1, indicating effective data reduction, though some datasets may not compress well.
- 😀 Flash Blade's energy efficiency is a key feature, with a full-load 52-terabyte blade consuming only 8950 watts, making it highly energy-efficient for large-scale data processing.
- 😀 Flash Blade targets industries like rich media, data analytics, and technical computing, with use cases in movie production, genomics, financial modeling, and more.
- 😀 The system has been successfully deployed in sectors such as genomics, machine learning, and gaming, showing its versatility across industries with large, complex datasets.
Q & A
What is Flash Blade and what makes it unique in the storage world?
-Flash Blade is a scale-out storage product designed to handle growing and diverse data sets. It's not a traditional filer but a storage system with multiple interface methods, making it versatile. Its design allows for expansion, scalability, and high performance while keeping it simple to operate.
What are the key types of data that Flash Blade is built to handle?
-Flash Blade is designed to manage data from sources like scientific data, analytics, sensor data, backups, imaging, and transcoding. These are born-digital datasets that grow rapidly, are machine-generated, and have different storage and access requirements than traditional data.
Why was Flash Blade designed with an object interface in addition to NFS?
-Flash Blade initially launched with an NFS interface but plans to add an object interface to cater to the growing demands of object storage. This approach allows Flash Blade to handle the rapid growth of metadata and unstructured data in diverse industries.
How does Flash Blade compare to Flash Array in terms of use cases?
-Flash Blade is designed for newer types of data, such as machine-generated and unstructured data, which is different from the traditional databases and virtual machines Flash Array is optimized for. Flash Blade serves industries dealing with large amounts of analytics, sensor data, and imaging, among others.
What were the main design goals for Flash Blade?
-The key goals for Flash Blade were to create a system that is scalable, fast, and simple. The system needs to accommodate large datasets, perform at high speeds, and be easy to upgrade, set up, and operate without the complexity of traditional storage systems.
How does Flash Blade ensure high performance and energy efficiency?
-Flash Blade achieves high performance through a design that allows for fast throughput and high IOPS. It is also highly energy-efficient, consuming only 8950 watts at full load, making it an efficient choice for large-scale storage environments.
What is the data reduction rate in Flash Blade, and how does it vary across different use cases?
-Flash Blade's data reduction rate varies based on the type of data. In real-world scenarios, the data reduction rate is typically around 3:1 to 3.5:1, but some data sets, especially those without compressible data, may not see any reduction at all.
How does Flash Blade handle large amounts of metadata?
-Flash Blade's design is tailored to cope with large amounts of metadata pressure. The system's architecture is engineered to handle these demands, particularly in industries like genomics and machine learning, where large-scale metadata management is crucial.
What industries are currently using Flash Blade, and what types of use cases are being addressed?
-Flash Blade is being used in industries like rich media, data analytics, and technical computing. Use cases include movie production, special effects, real-time transcoding, genomics, financial modeling, machine learning, and simulations in various fields.
Why is Flash Blade considered a launchpad for future innovations?
-Flash Blade is seen as a launchpad because it addresses the most complex and demanding workloads in various industries. By proving its capabilities in challenging areas like genomics and machine learning, it lays the groundwork for further development and new applications in storage solutions.
Outlines
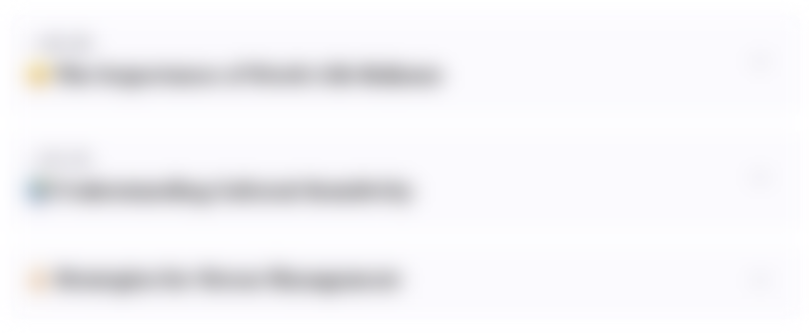
هذا القسم متوفر فقط للمشتركين. يرجى الترقية للوصول إلى هذه الميزة.
قم بالترقية الآنMindmap
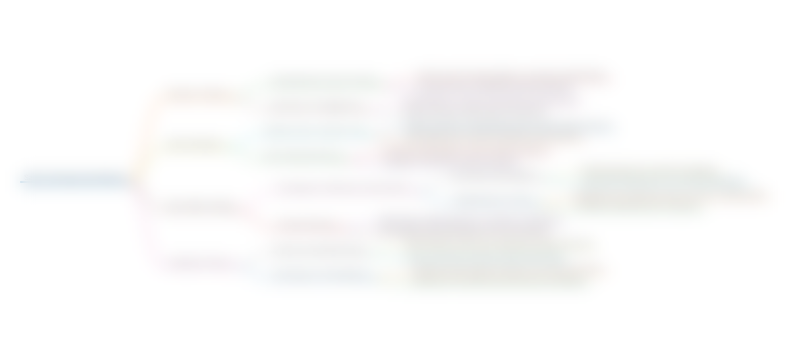
هذا القسم متوفر فقط للمشتركين. يرجى الترقية للوصول إلى هذه الميزة.
قم بالترقية الآنKeywords
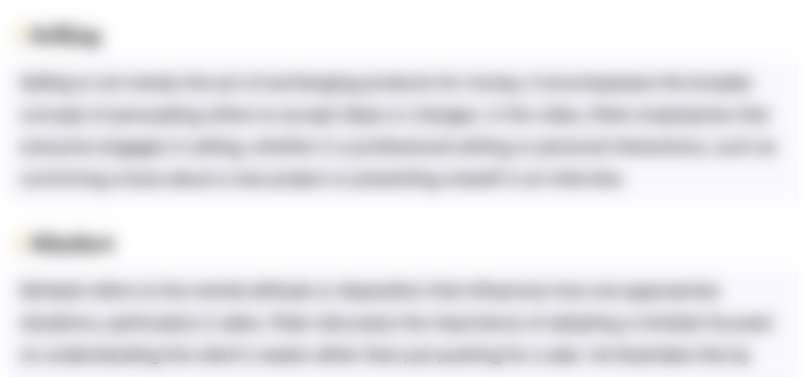
هذا القسم متوفر فقط للمشتركين. يرجى الترقية للوصول إلى هذه الميزة.
قم بالترقية الآنHighlights
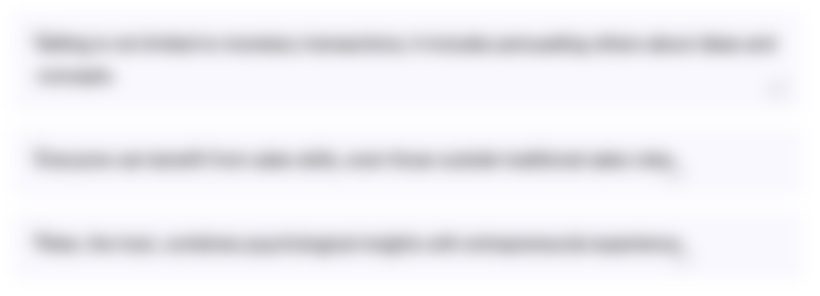
هذا القسم متوفر فقط للمشتركين. يرجى الترقية للوصول إلى هذه الميزة.
قم بالترقية الآنTranscripts
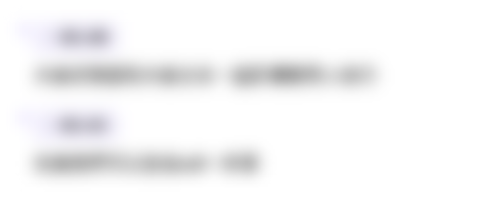
هذا القسم متوفر فقط للمشتركين. يرجى الترقية للوصول إلى هذه الميزة.
قم بالترقية الآنتصفح المزيد من مقاطع الفيديو ذات الصلة
5.0 / 5 (0 votes)