Understanding Statistical Inference - statistics help
Summary
TLDRDr. Nic introduces statistical inference as a method to draw conclusions about a population from a sample. He differentiates it from descriptive statistics, emphasizing its ability to extend insights beyond the sample. The video covers the importance of sample representativeness, the inherent uncertainty in sampling, and the impact of sampling methods on inference accuracy. It also touches on the concepts of sampling error, confidence intervals, and hypothesis testing, all crucial for understanding statistical inference.
Takeaways
- 📊 Descriptive statistics summarize data using graphs and summary values like mean and interquartile range, helping identify relationships and patterns but not drawing conclusions beyond the data.
- 🔍 Inferential statistics allow making conclusions about the population from which a sample is drawn, extending insights beyond the sample itself.
- 🔑 The definition of inference is drawing conclusions about population parameters based on a sample, highlighting the importance of the sample as a representation of the population.
- 🍎 Examples of populations and samples include all apples in an orchard versus a sample of 100 apples, illustrating how samples are used to infer about the whole.
- 📈 There are three main ideas underlying inference: the sample as a good representation of the population, the uncertainty in how well the sample represents the population, and the importance of the way the sample is taken.
- 🤔 The expectation that a sample will represent the population is reasonable but not guaranteed due to sampling error and the fact that no sample is a perfect representation.
- 📊 Through simulation and probability theory, we can estimate what the population is likely to be like based on sample information, addressing the uncertainty of representation.
- 📉 A simulation can show the likelihood of certain outcomes in samples, such as the probability of a sample proportion deviating significantly from the population proportion.
- 📐 The margin of error, typically around 3% with a sample size of 1000, is used to create confidence intervals, providing a range where the population parameter is likely to fall.
- 🧐 In inferential statistics, hypothesis testing and p-values are used to evaluate claims about the population based on sample data.
- 🔄 The method of sampling is crucial for representativeness, with random sampling being ideal but often challenging and costly, especially with human populations.
Q & A
What are the two main focuses of statistical analysis?
-The two main focuses of statistical analysis are descriptive statistics and inferential statistics.
What do descriptive statistics do?
-Descriptive statistics summarize data using graphs and summary values, such as the mean and interquartile range. They help identify relationships and patterns but do not draw conclusions beyond the data.
How does inferential statistical analysis differ from descriptive statistics?
-Inferential statistical analysis allows us to make conclusions beyond the data we have to the population from which it was drawn, whereas descriptive statistics only summarize the existing data.
What is a definition of statistical inference?
-Statistical inference is the process of drawing conclusions about population parameters based on a sample taken from the population.
What are some examples of populations and samples?
-Examples include: the population of all apples in an orchard with a sample of 100 apples; the population of all chocolate bars produced on a machine with a sample of 30 bars; the population of all voters in New Zealand with a sample of 1000 people surveyed online.
What are the three main ideas underlying inference?
-The three main ideas are: 1) A sample is likely to be a good representation of the population. 2) There is an element of uncertainty in how well the sample represents the population. 3) The way the sample is taken matters.
Why is there uncertainty in how well a sample represents a population?
-There is uncertainty because a sample will never be a perfect representation of the population, leading to sampling error. Different samples from the same population can yield different results.
How can we estimate the likelihood of a sample proportion being close to the population proportion?
-We can estimate this using probability theory or simulations. For example, by simulating multiple samples from a population and analyzing the distribution of the sample proportions.
What is the margin of error, and how is it related to sample size?
-The margin of error indicates the range within which the sample proportion is expected to differ from the population proportion. For a sample size of 1000, the margin of error is typically about 3%.
Why does the way the sample is taken matter?
-The sample must be representative of the population, which is best achieved when each member of the population has an equal chance of being selected. This reduces non-sampling error.
Outlines
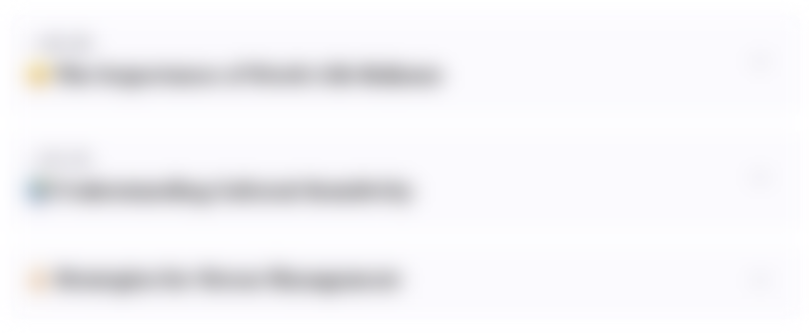
此内容仅限付费用户访问。 请升级后访问。
立即升级Mindmap
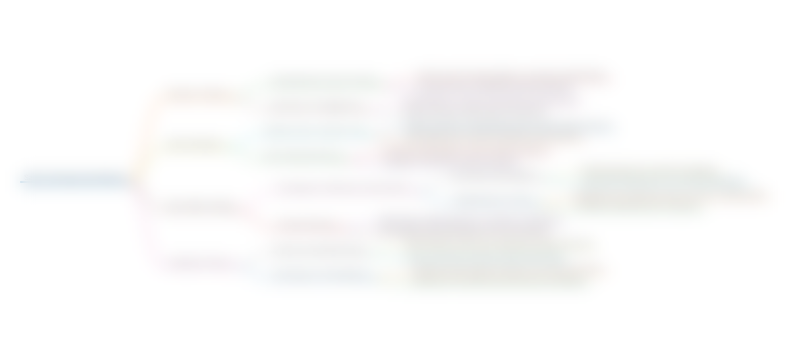
此内容仅限付费用户访问。 请升级后访问。
立即升级Keywords
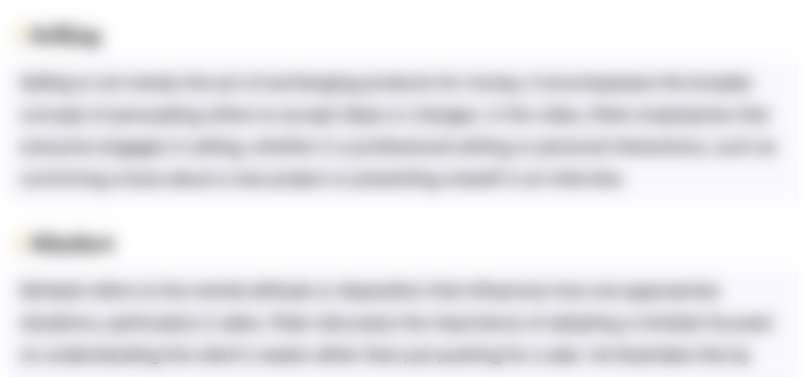
此内容仅限付费用户访问。 请升级后访问。
立即升级Highlights
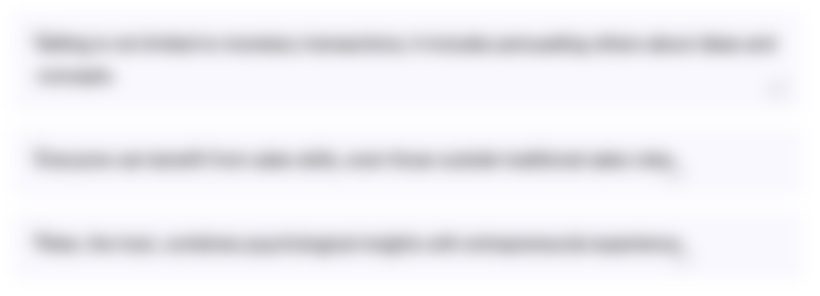
此内容仅限付费用户访问。 请升级后访问。
立即升级Transcripts
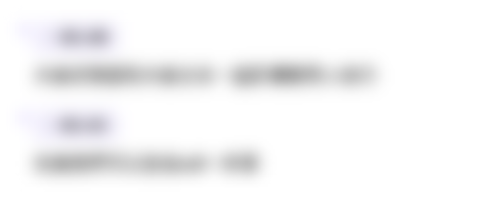
此内容仅限付费用户访问。 请升级后访问。
立即升级浏览更多相关视频

Understanding Confidence Intervals: Statistics Help

Statistical Inference: Introduction and Terminology (in Hindi)
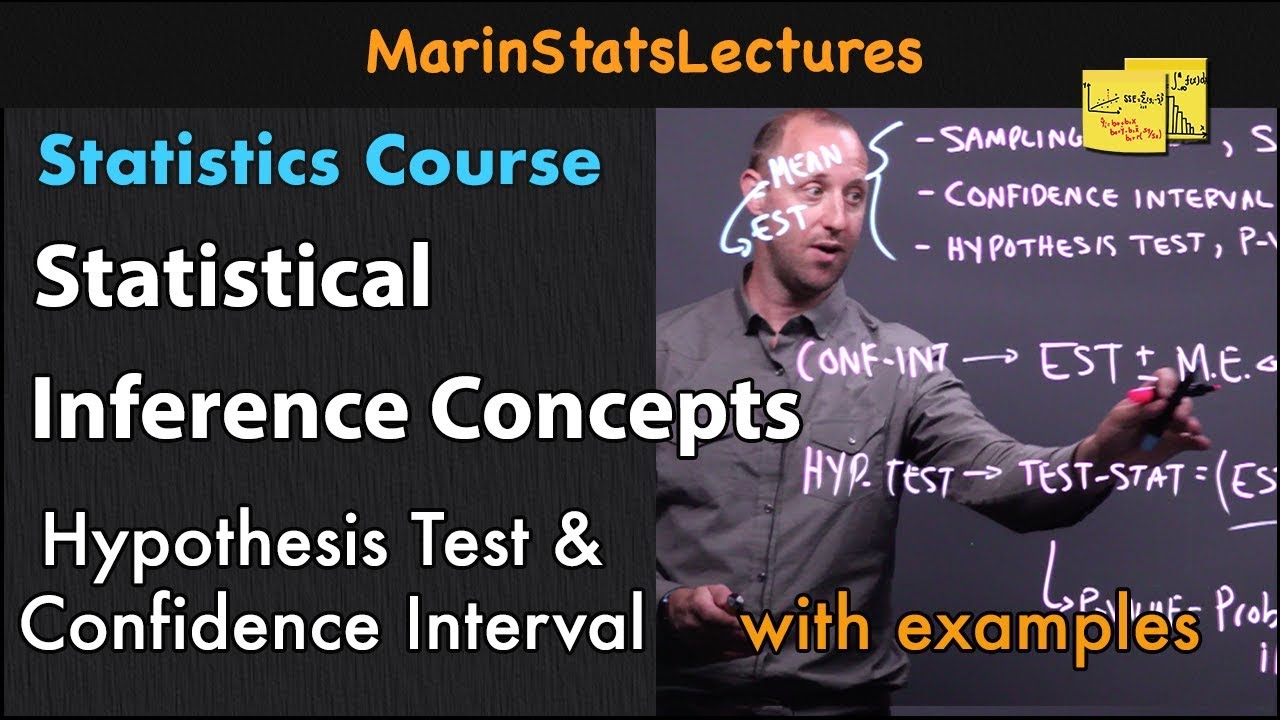
Statistical Inference Definition with Example | Statistics Tutorial #18 | MarinStatsLectures
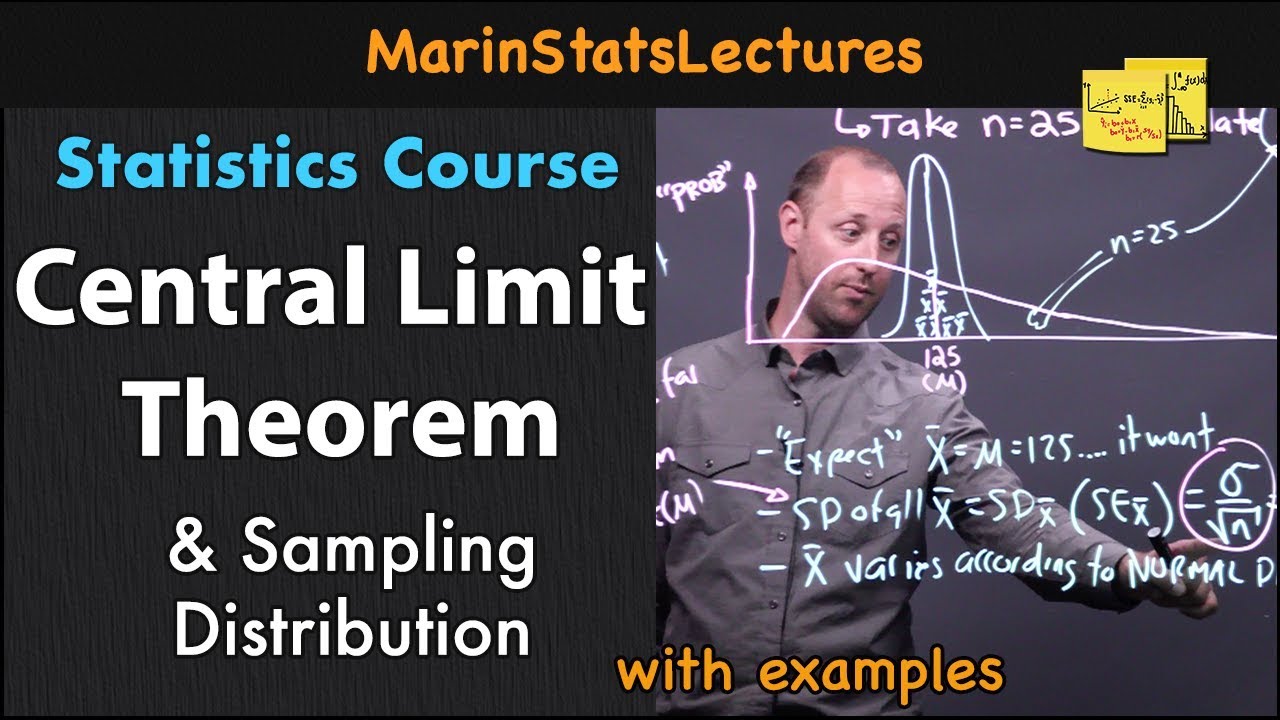
Central Limit Theorem & Sampling Distribution Concepts | Statistics Tutorial | MarinStatsLectures
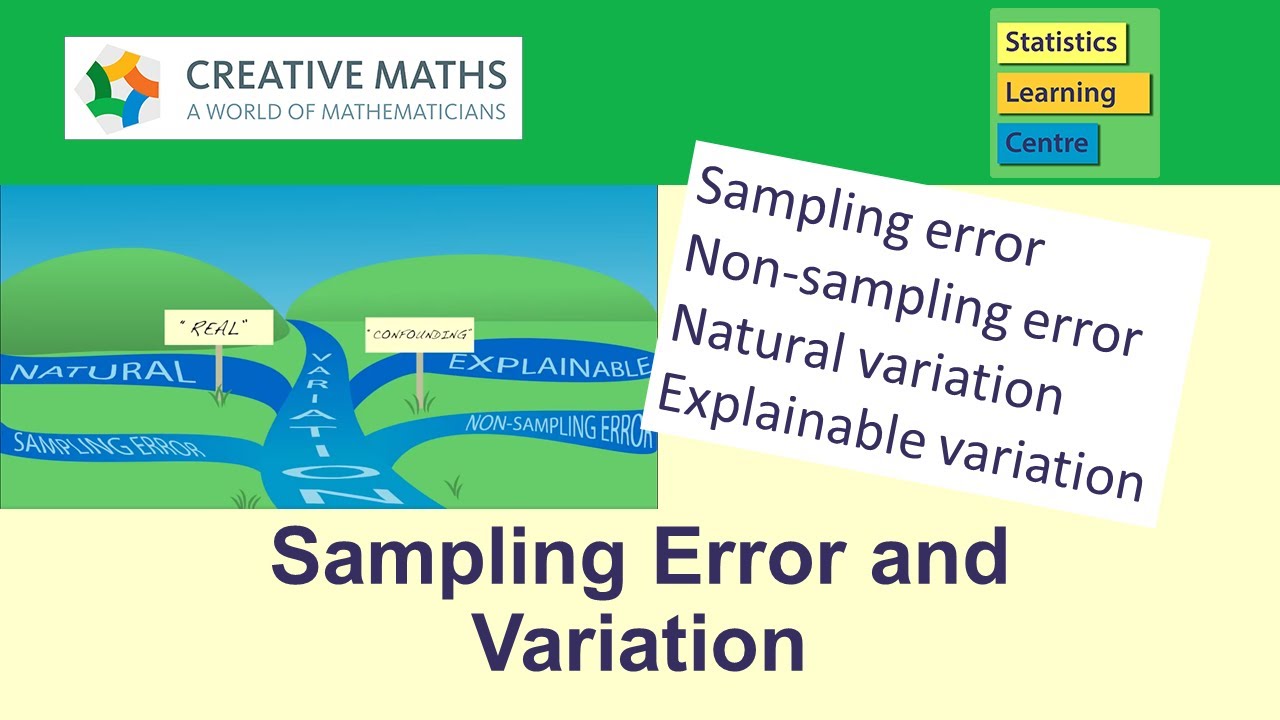
Sampling error and variation in statistics and data science

Sampling distributions
5.0 / 5 (0 votes)