Intro to Data Science: What is Data Science?
Summary
TLDRThe video script offers an introduction to data science, emphasizing the iterative process of asking questions and using data to find answers. It highlights the importance of data collection, curation, and cleaning, as well as the critical role of database engineering. The script also underscores the significance of visualization, analysis, and machine learning in building predictive models. It stresses the collaborative nature of data science, the need for 'pie-shaped' experts with both domain knowledge and data science skills, and the importance of reproducibility in ensuring reliable scientific results.
Takeaways
- 🔍 Data Science is fundamentally about asking questions that can be answered with data and involves a feedback cycle to refine those questions and answers.
- 🗂️ A significant part of data science is data collection, curation, storage, cleaning, and processing, which are critical for handling real-world messy data.
- 👷♂️ Database engineering and management are essential for preparing data for analysis, including identifying outliers and handling missing data.
- 📊 Visualization is a key aspect of data science, helping to communicate findings and insights effectively to various stakeholders.
- 🤖 Machine learning is an exciting area within data science that involves developing predictive models from data, but it requires a solid foundation in data preparation.
- 🔁 Feedback loops are integral at every stage of the data science process, from data collection to analysis and modeling, allowing for continuous improvement and adjustment.
- 🚀 Data science is dynamic and not a static architecture; it is driven by expert human teams who are engaged in asking and answering questions with data.
- 💡 The importance of investing in analytics and modeling alongside data engineering is emphasized, warning against the risk of being 'up data creek without a data paddle' without it.
- 👥 Data science is a collaborative art that involves teams with a mix of domain expertise and data science skills, moving towards 'pie-shaped' experts with multiple depths of knowledge.
- 🔄 Reproducibility is a critical aspect of data science, ensuring that processes and findings can be reliably reproduced by others, which is essential for scientific integrity.
- 🌐 The script highlights the importance of communication in data science, noting that great ideas must be effectively communicated to be truly impactful.
Q & A
What is the primary focus of data science according to the transcript?
-The primary focus of data science, as mentioned in the transcript, is about asking questions that can be answered with data and establishing a feedback cycle.
Why is data collection, curation, and cleaning considered crucial in data science?
-Data collection, curation, and cleaning are crucial in data science because real-world data is often messy, and these processes are essential to ensure the data is usable and accurate for analysis and modeling.
What role does database engineering and management play in data science?
-Database engineering and management play a significant role in data science as they are responsible for the storage, processing, and cleaning of data, which are foundational steps before any analysis or modeling can take place.
What are the downstream activities that follow data curation and cleaning in the data science process?
-Downstream activities following data curation and cleaning include visualization, analysis, and modeling. These activities involve using the cleaned data to build predictive models and make data-driven decisions.
How does the transcript describe the importance of visualization in data science?
-The transcript describes visualization as a critical aspect of data science because it helps in communication. Being able to visualize and communicate findings is essential for the feedback cycle and for ensuring that insights are understood and acted upon.
What is the significance of feedback loops in the data science process as described in the transcript?
-Feedback loops are significant in the data science process as they allow for continuous improvement and adaptation. They can lead to modifications in data collection methods, storage practices, or even the questions being asked, based on insights gained from analysis and visualization.
What is the role of machine learning in the context of data science as per the transcript?
-Machine learning plays a role in data science by developing models from data for predictions and insights. It is an exciting field that requires a solid foundation of cleaned and curated data to be effective.
Why is it important to invest in analysis, modeling, and visualization along with data engineering?
-Investing in analysis, modeling, and visualization is important because without these, one might end up with a vast amount of data but without the means to derive actionable insights from it, leading to the metaphorical situation of 'up data creek without a data paddle'.
What does the transcript suggest about the future of data scientists in teams?
-The transcript suggests that in the future, data scientists will not be isolated but will be integrated into teams, contributing their data science expertise alongside their domain expertise, becoming what is referred to as 'pie-shaped' experts.
What is the importance of reproducibility in data science as highlighted in the transcript?
-Reproducibility in data science is important to ensure that the processes and results can be independently verified by others, which is crucial for maintaining the reliability and integrity of scientific findings.
How does the transcript define the term 'data-driven inquiry'?
-The transcript defines 'data-driven inquiry' as the science of asking questions that can be answered with data, emphasizing the iterative and collaborative nature of the data science process.
Outlines
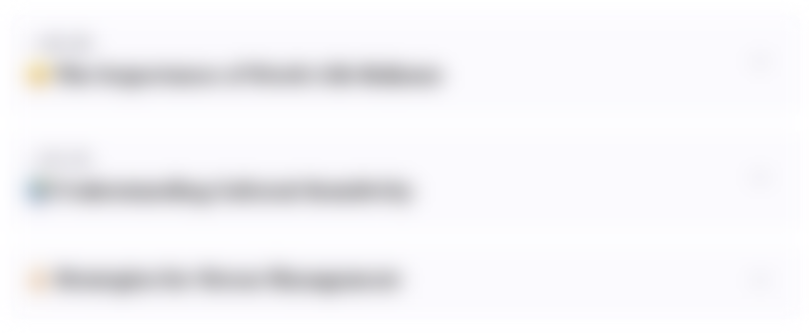
此内容仅限付费用户访问。 请升级后访问。
立即升级Mindmap
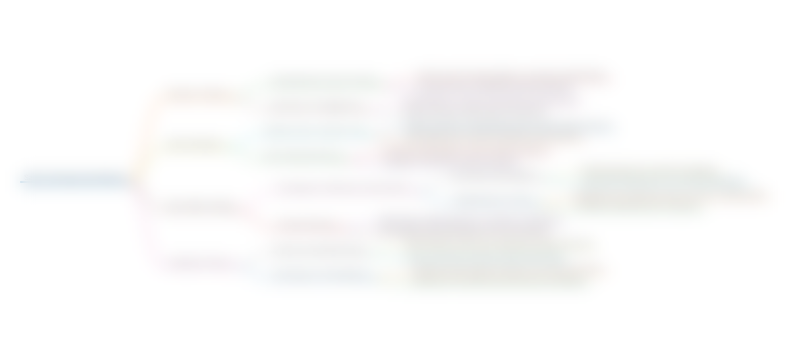
此内容仅限付费用户访问。 请升级后访问。
立即升级Keywords
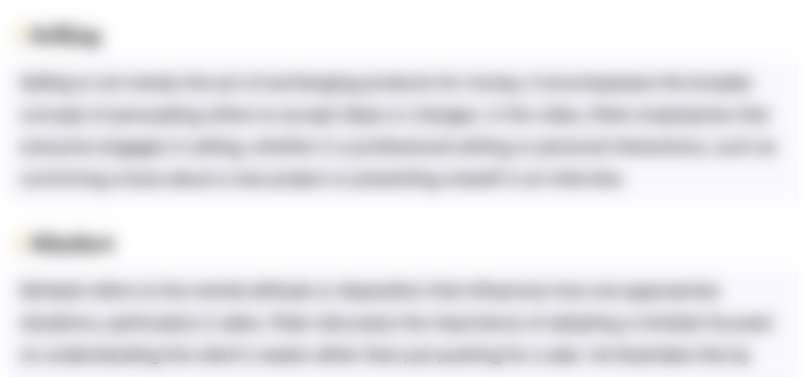
此内容仅限付费用户访问。 请升级后访问。
立即升级Highlights
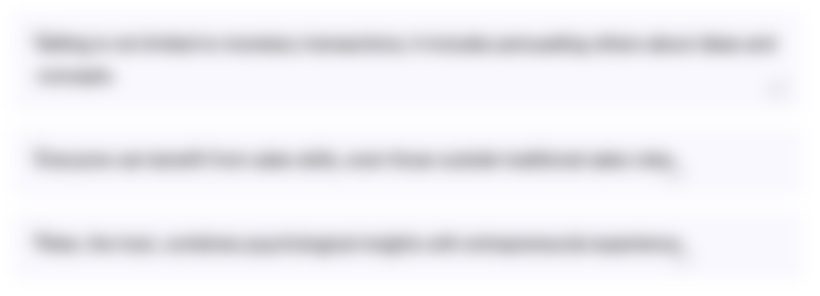
此内容仅限付费用户访问。 请升级后访问。
立即升级Transcripts
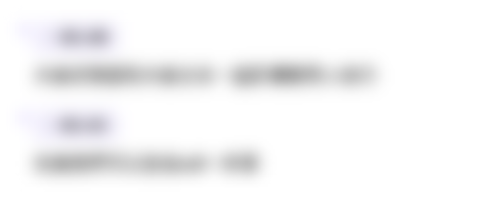
此内容仅限付费用户访问。 请升级后访问。
立即升级浏览更多相关视频
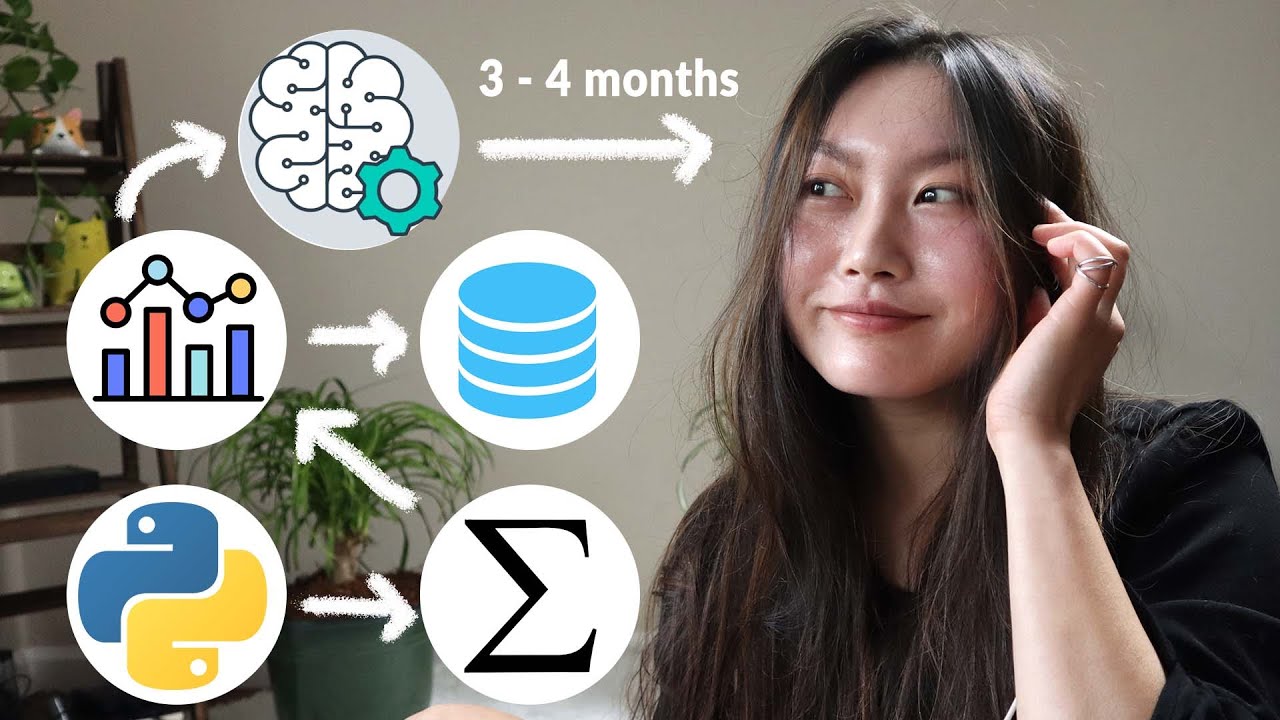
How I Would Learn Data Science in 2022
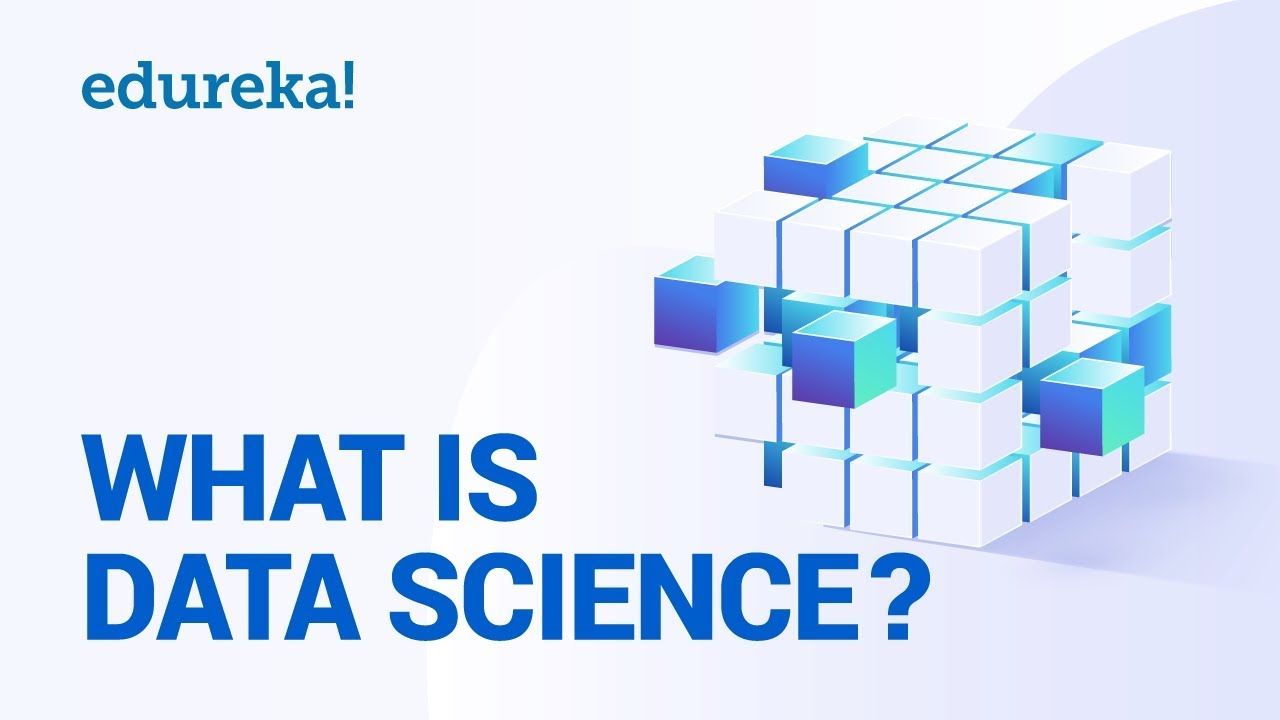
Data Science in 8 Minutes | Data Science for Beginners | What is Data Science? | Edureka
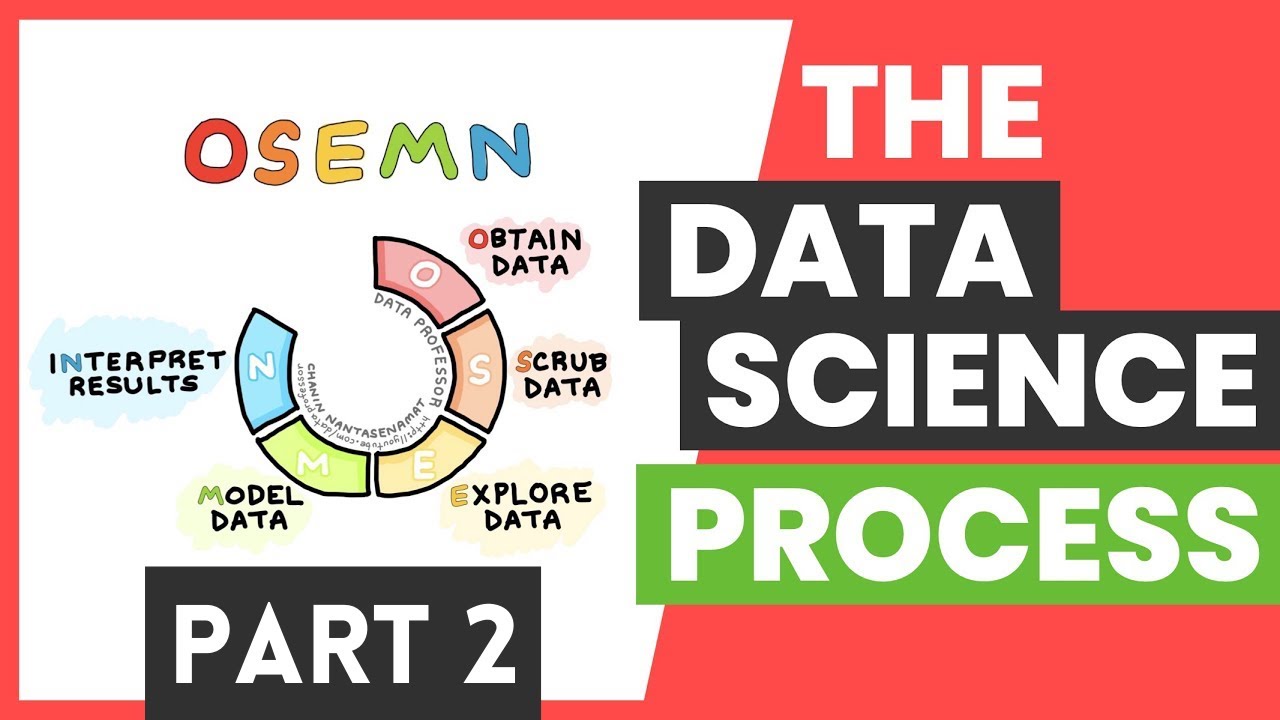
The Data Science Process - A Visual Guide (Part 2)
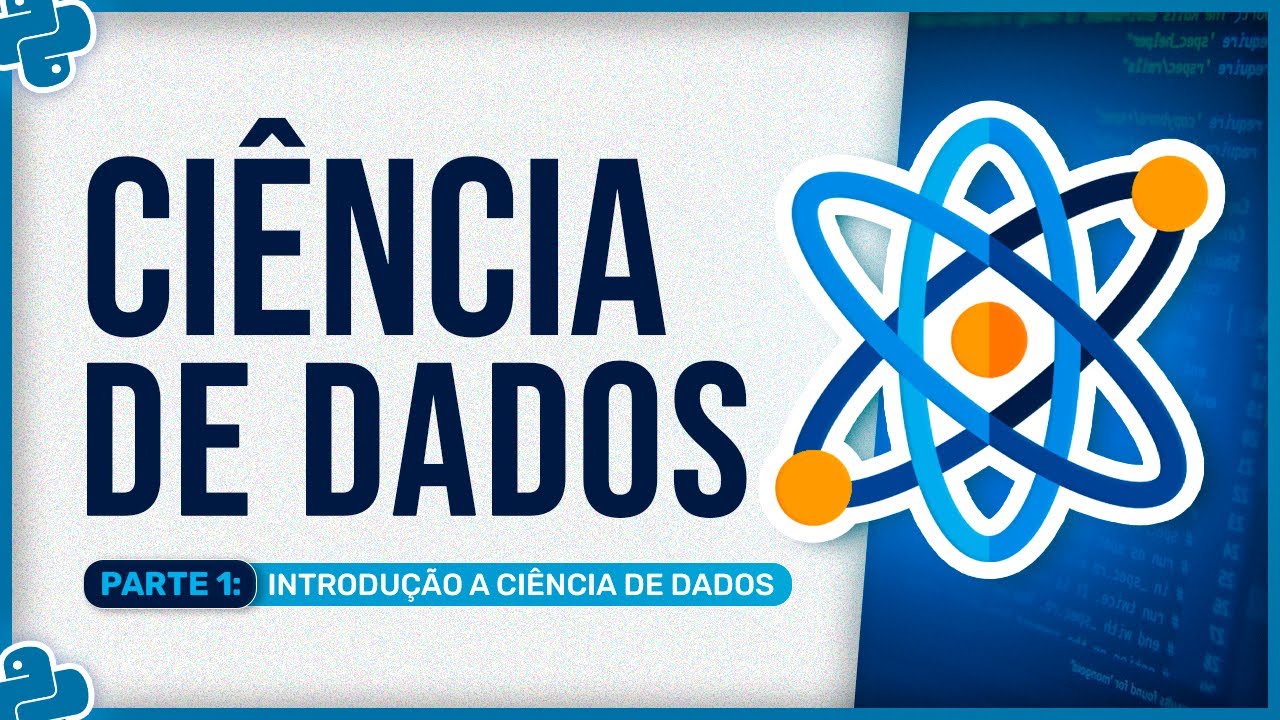
Curso Básico de Ciência de Dados - Aula 1 - Introdução a Ciência de Dados
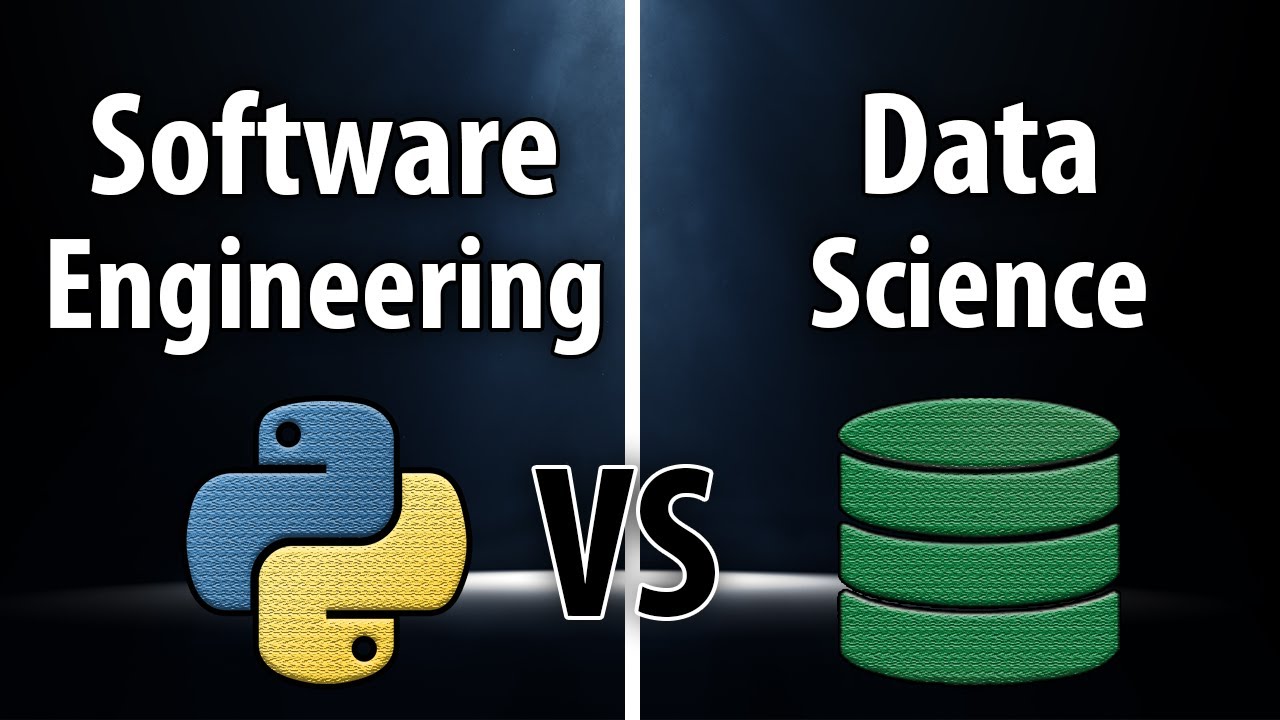
Software Engineering vs Data Science - How To Choose Between Them
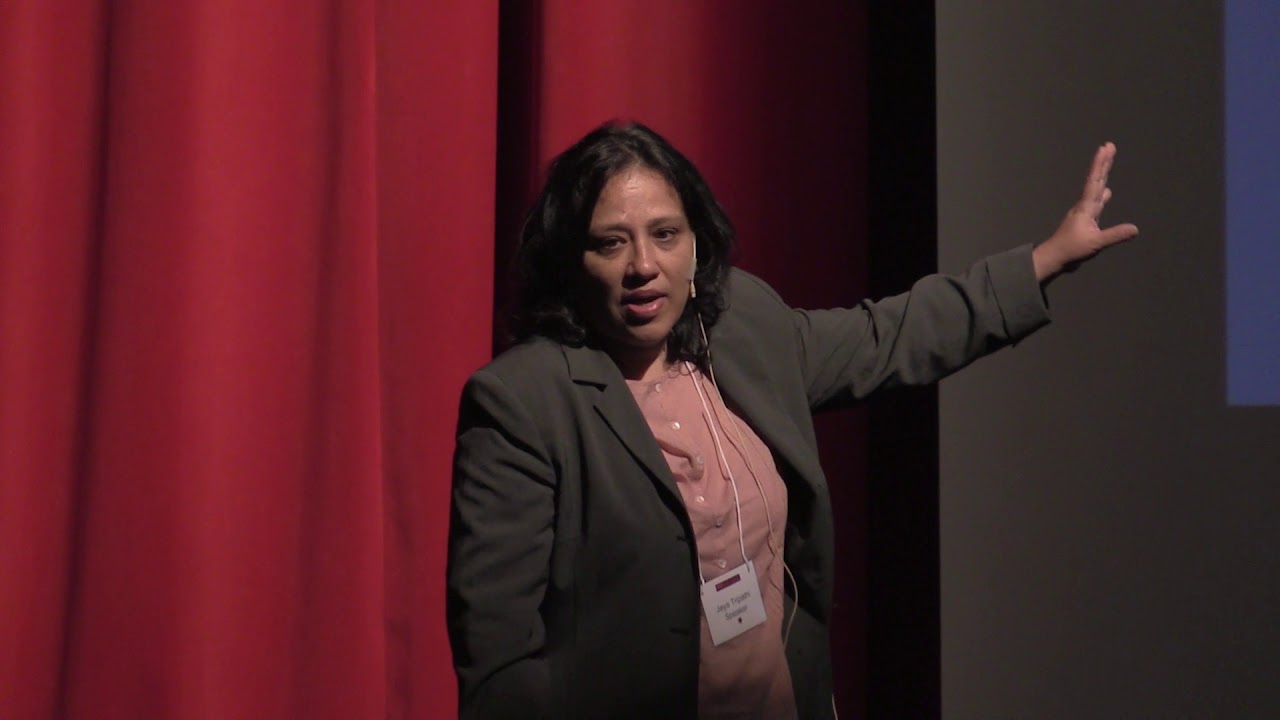
Truth in Data Science | Jaya Tripathi | TEDxYouth@BHS
5.0 / 5 (0 votes)