Data Science Life Cycle | Life Cycle Of A Data Science Project | Data Science Tutorial | Simplilearn
Summary
TLDRIn this session on data science, Mohan introduces the life cycle of a data science project, starting with the concept study to understand the business problem and available data. He then discusses data preparation, including data gathering, integration, and cleaning. Mohan explains model planning and building, highlighting various algorithms and exploratory data analysis techniques. The session covers training and testing models, deploying them, and communicating results to stakeholders. Finally, he summarizes the process, emphasizing the importance of presenting and operationalizing the findings to solve business problems effectively.
Takeaways
- 📚 The first step in a data science project is the concept study, which involves understanding the business problem and available data, and meeting with stakeholders.
- 🔍 Data preparation, also known as data munching or manipulation, is crucial for transforming raw data into a usable format for analysis.
- 🔧 Data scientists explore and clean the data, handling issues like missing values, null values, and improper data types.
- 📈 Data integration, transformation, reduction, and cleaning are all part of the data preparation process to ensure data quality for analysis.
- ⚖️ Handling missing values can involve removing records, filling them with mean or median values, or using more complex methods depending on the dataset's size and importance.
- 📊 Exploratory data analysis (EDA) uses visualization techniques like histograms and scatter plots to understand data patterns and relationships.
- 🤖 Model planning involves selecting the right statistical or machine learning model based on the problem, such as regression for continuous outcomes or classification for categorical outcomes.
- 🛠️ Model building is the execution phase where the chosen algorithm is trained with the cleaned data to create a predictive model.
- 📉 Testing the model with a separate dataset ensures its accuracy and reliability before deployment.
- 🛑 If the model fails to meet accuracy expectations during testing, it may need to be retrained or a different algorithm may be required.
- 📑 Communicating results effectively to stakeholders and operationalizing the model to solve the initial business problem is the final step in the data science lifecycle.
Q & A
What is the first step in the life cycle of a data science project?
-The first step is the concept study, which involves understanding the business problem, meeting with stakeholders, and assessing the available data.
Why is it important to meet with stakeholders during the concept study phase?
-Meeting with stakeholders helps to understand the business model, clarify the end goal, and determine the budget, which are all crucial for the project's success.
What are some examples of data issues that might be encountered during data preparation?
-Examples include missing values, null values, improper data types, and data redundancy from multiple sources.
What is the purpose of data munching or data manipulation in the data preparation phase?
-Data munching or manipulation is necessary to transform raw data into a usable format for analysis, addressing issues like data gaps, structure inconsistencies, and irrelevant columns.
How can data scientists handle missing values in a dataset?
-They can handle missing values by removing records with missing data if the percentage is small, or by imputing values using the mean, median, or mode of the dataset.
Why is it essential to split data into training and test sets during model preparation?
-Splitting data ensures that the model is tested on unseen data, providing a more accurate measure of its performance and preventing overfitting.
What is exploratory data analysis, and why is it important?
-Exploratory data analysis is the initial examination of data to discover patterns and understand the data types and distributions. It's important for identifying data issues and guiding the choice of models.
What are some common tools used for model planning and building in data science?
-Common tools include R, Python with libraries like pandas or numpy, MATLAB, and SAS, each offering capabilities for statistical analysis, machine learning, and data visualization.
Can you explain how linear regression works in the context of model building?
-Linear regression works by finding the best-fit straight line that represents the relationship between an independent variable and a dependent variable. The model training process determines the slope (m) and y-intercept (c) for the given data.
What is the final step in the data science project life cycle after obtaining results?
-The final step is operationalizing the results, which involves communicating the findings to stakeholders, getting their acceptance, and putting the model into practice to solve the stated problem.
Outlines
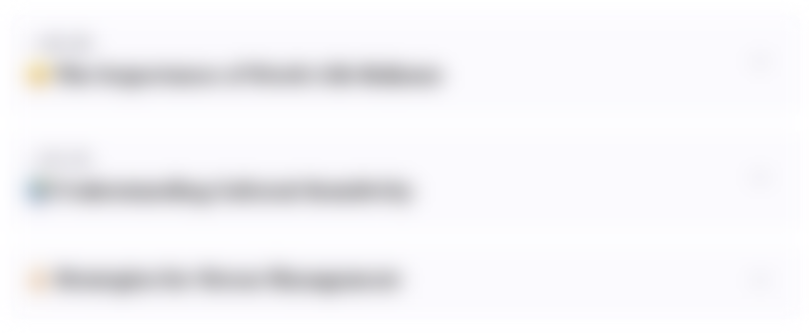
此内容仅限付费用户访问。 请升级后访问。
立即升级Mindmap
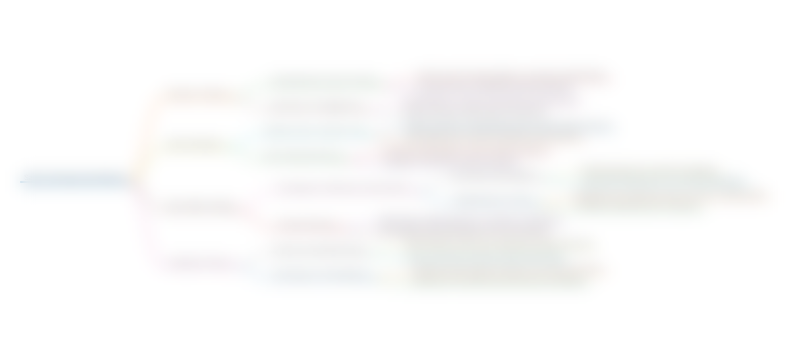
此内容仅限付费用户访问。 请升级后访问。
立即升级Keywords
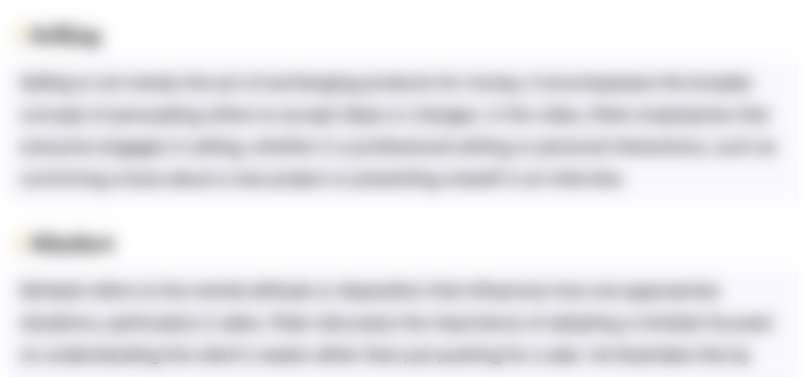
此内容仅限付费用户访问。 请升级后访问。
立即升级Highlights
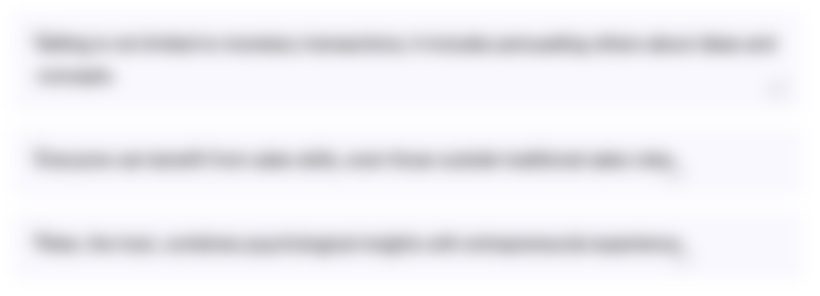
此内容仅限付费用户访问。 请升级后访问。
立即升级Transcripts
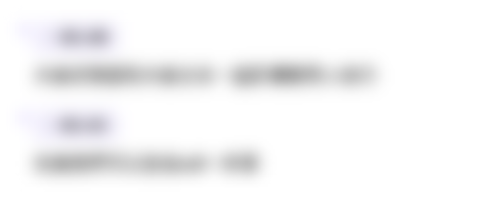
此内容仅限付费用户访问。 请升级后访问。
立即升级浏览更多相关视频
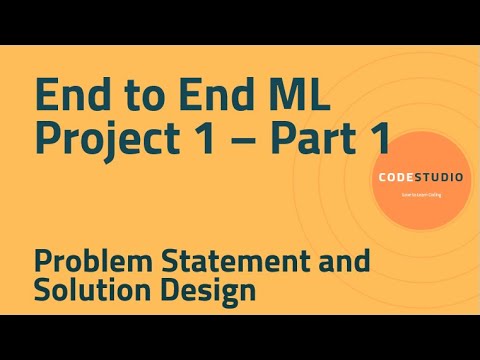
End to End ML Project 1 - P1 - Problem Statement and Solution Design

Lec-1: Introduction to Data Science & ML | Roadmap to Learn Data Science & ML
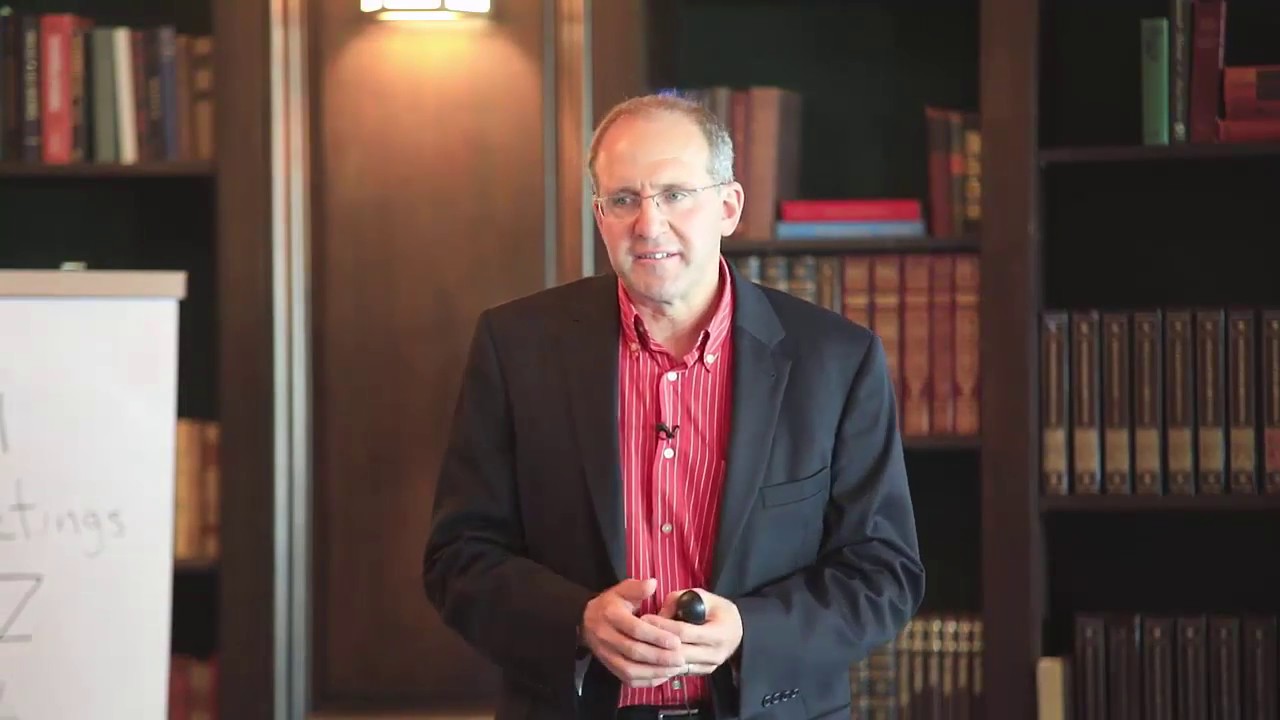
Power of Data Modeling
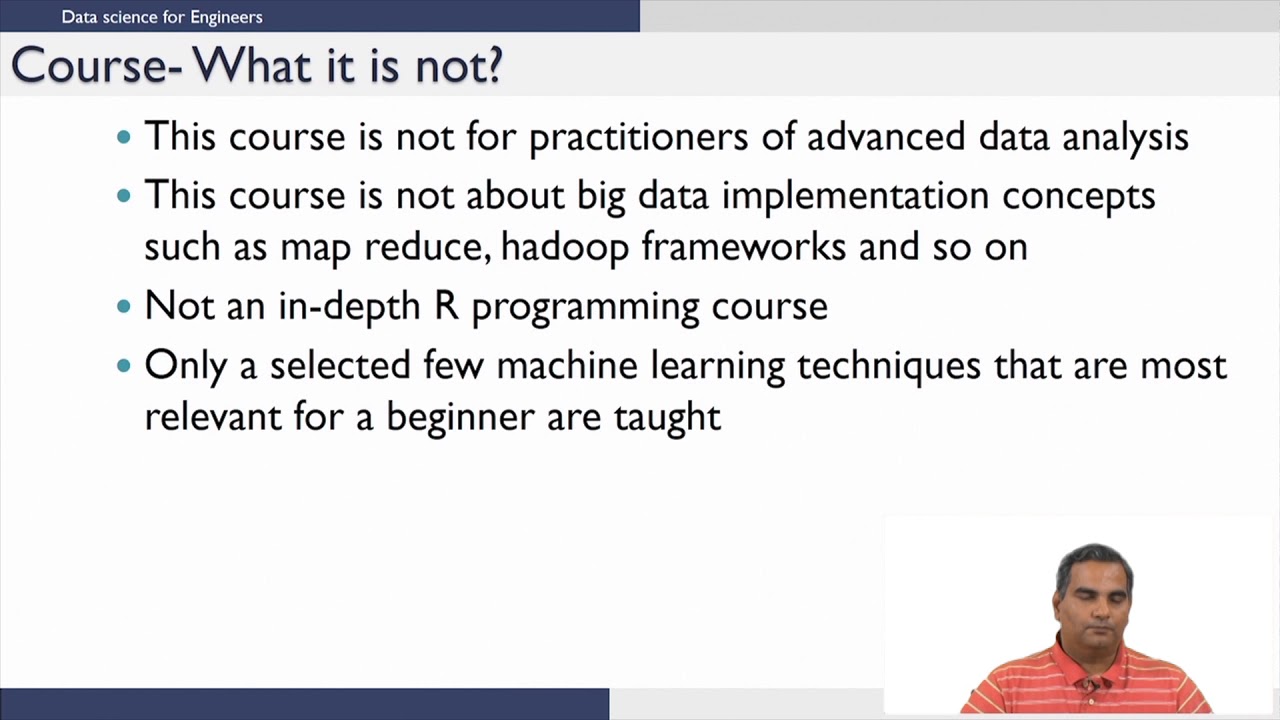
Data science for engineers Course philosophy and expectation
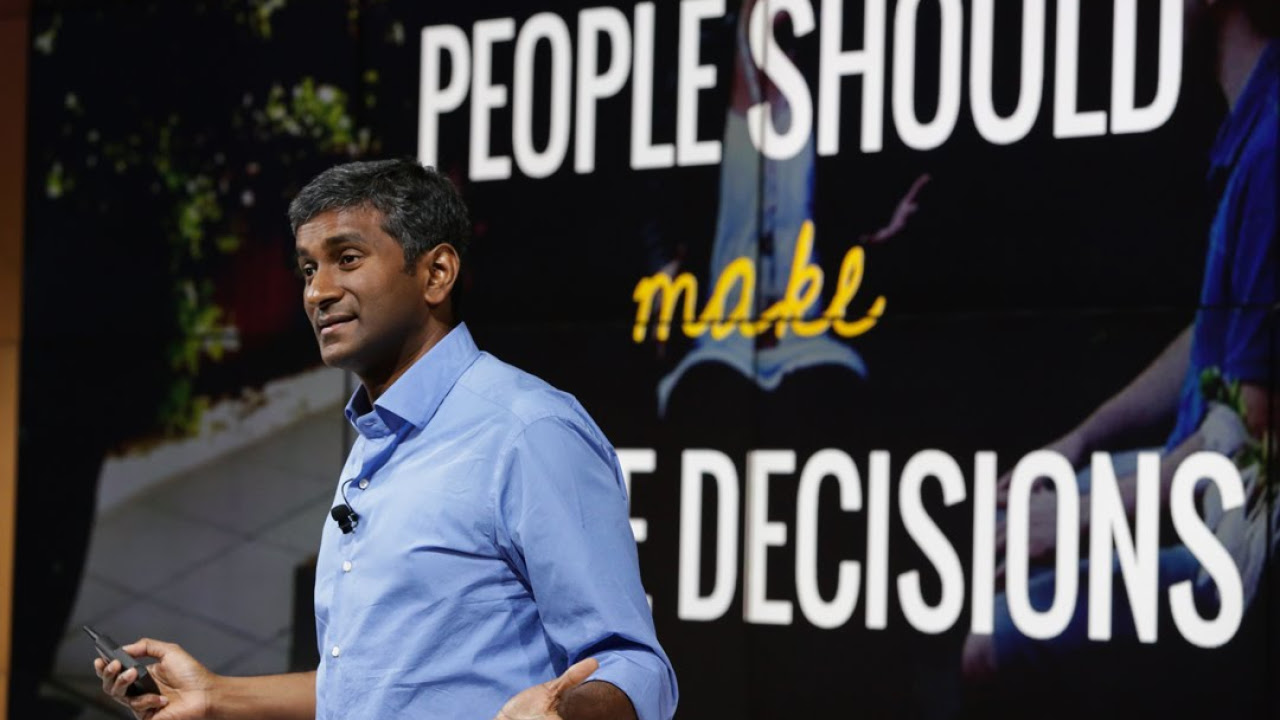
HR meets science at Google with Prasad Setty

EXPLORATORY ANALYSIS OF GEOLOCATIONAL DATA (MINI PROJECT)
5.0 / 5 (0 votes)