Pemikiran dan Prediksi Analitik Data (Data Analytic Thinking and Prediction)
Summary
TLDRThis video provides an in-depth introduction to data science and predictive analytics, focusing on its connection with management accounting. Key topics include problem definition, data collection, data models, and the importance of decision trees. The video explores algorithms like Gini impurity, the process of refining decision trees, model validation techniques, and how to evaluate models using tools like ROC curves and confusion matrices. Practical examples, such as an investment scenario, demonstrate how data science can optimize decision-making and create value in business processes. The presenter emphasizes the role of data science in shaping strategies and improving organizational outcomes.
Takeaways
- π Data science is about using data analysis to make predictions and guide decision-making processes.
- π The integration of data science with management accounting can help businesses optimize product design and manufacturing decisions.
- π Understanding and defining the problem is the first step in selecting the relevant data for analysis.
- π Algorithms and models, such as decision trees, are essential for processing large datasets and making predictions.
- π Gini impurity is a key metric used to measure the purity of data in decision trees, helping in classification tasks.
- π Pruning decision trees is important to prevent overfitting and improve the generalization of the model.
- π Model validation techniques include cross-validation, maximum likelihood estimation, and testing models on sample data.
- π Effective model selection is based on its performance in real-world scenarios, with an emphasis on minimizing bias and variance.
- π Evaluating models involves metrics like likelihood values, feature evaluation, ROC curves, and confusion matrices to measure prediction accuracy.
- π Real-world case studies, like predicting loan defaults, show how different probabilities affect investment decisions and the accuracy of predictions.
- π Implementing data science models requires careful consideration of data accuracy, historical data relevance, and proper decision thresholds for successful outcomes.
Q & A
What is the main focus of the video transcript?
-The main focus of the transcript is explaining the role of data science in predictive analytics, specifically in business decision-making, with an emphasis on understanding data models, algorithms, and evaluation methods.
What are the three key areas where data science intersects in predictive analytics?
-The three key areas are computer science and data skills, mathematics and statistics, and substantive expertise in specific domains such as accounting and management.
How does data science aid in decision-making for businesses?
-Data science helps businesses make informed decisions by predicting future outcomes based on historical data, allowing organizations to strategize effectively and optimize operations.
What is the Gini impurity and how is it used in decision trees?
-The Gini impurity is a measure of how mixed the classes are in a dataset. It is used in decision trees to evaluate the 'purity' of data at each node, helping to decide the best split based on class distribution.
What is the process of pruning a decision tree?
-Pruning a decision tree involves removing branches that do not contribute significantly to the model's predictive power. This helps reduce complexity and overfitting, making the model more generalizable.
What is the importance of model validation in data science?
-Model validation ensures that a model performs well on unseen data. It helps in evaluating the modelβs accuracy and robustness by testing it on data that was not used during training, thus preventing overfitting.
What are the three techniques mentioned for validating models?
-The three techniques mentioned for validating models are cross-validation, maximum likelihood estimation, and testing the pruned decision tree model on a sample.
How does adjusting the cutoff probability affect financial predictions, such as loan repayments?
-Adjusting the cutoff probability impacts the classification of loans as 'default' or 'repayment.' A higher cutoff reduces the number of defaults but may also lead to more loan repayments being misclassified, affecting the overall financial outcome.
What are some challenges when implementing data science models in business?
-Challenges include ensuring data accuracy, dealing with incomplete or outdated data, and selecting the appropriate cutoff values for predictions. The model must be capable of handling diverse data conditions for successful implementation.
Why is it important to choose a model based on validation data rather than training data?
-Choosing a model based on validation data helps avoid overfitting, ensuring the model generalizes well to new, unseen data. It ensures that the modelβs performance is not just due to memorizing the training data but truly reflects its ability to predict real-world outcomes.
Outlines
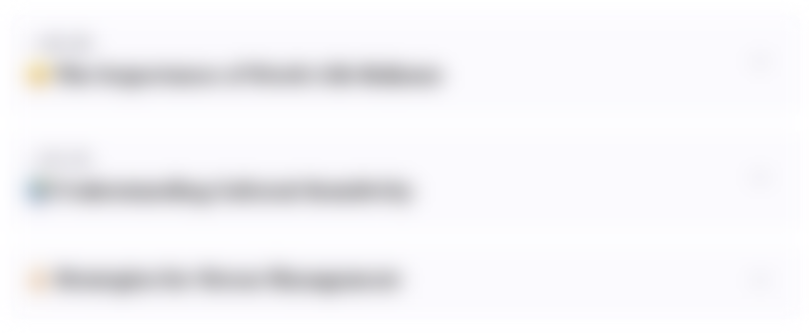
This section is available to paid users only. Please upgrade to access this part.
Upgrade NowMindmap
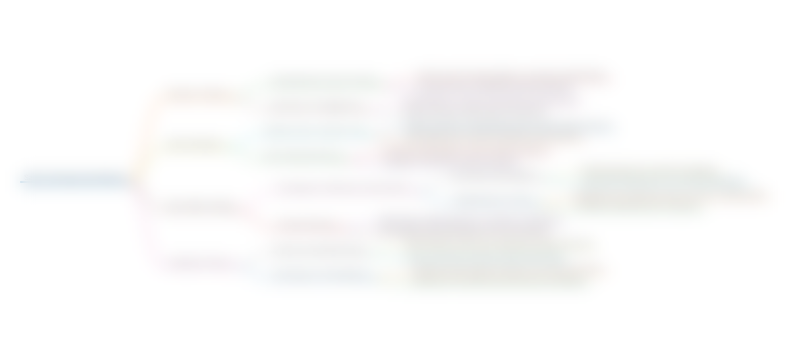
This section is available to paid users only. Please upgrade to access this part.
Upgrade NowKeywords
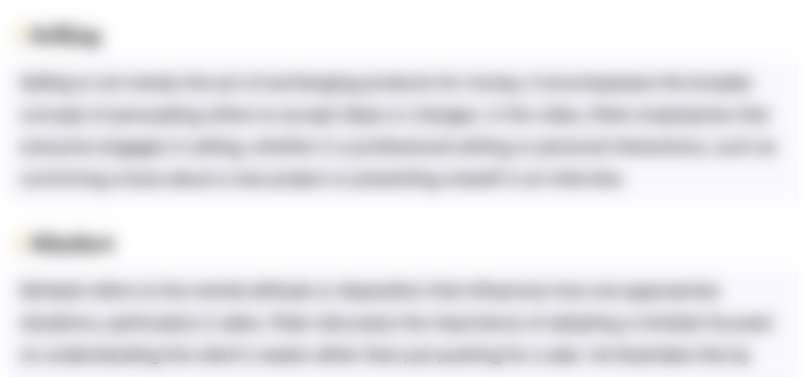
This section is available to paid users only. Please upgrade to access this part.
Upgrade NowHighlights
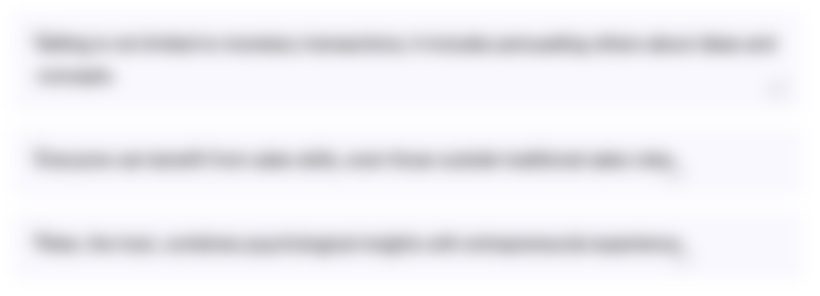
This section is available to paid users only. Please upgrade to access this part.
Upgrade NowTranscripts
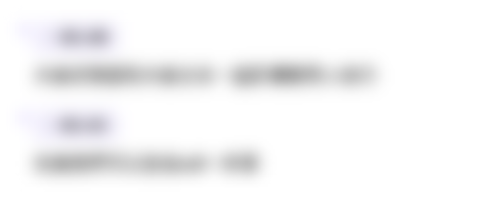
This section is available to paid users only. Please upgrade to access this part.
Upgrade NowBrowse More Related Video
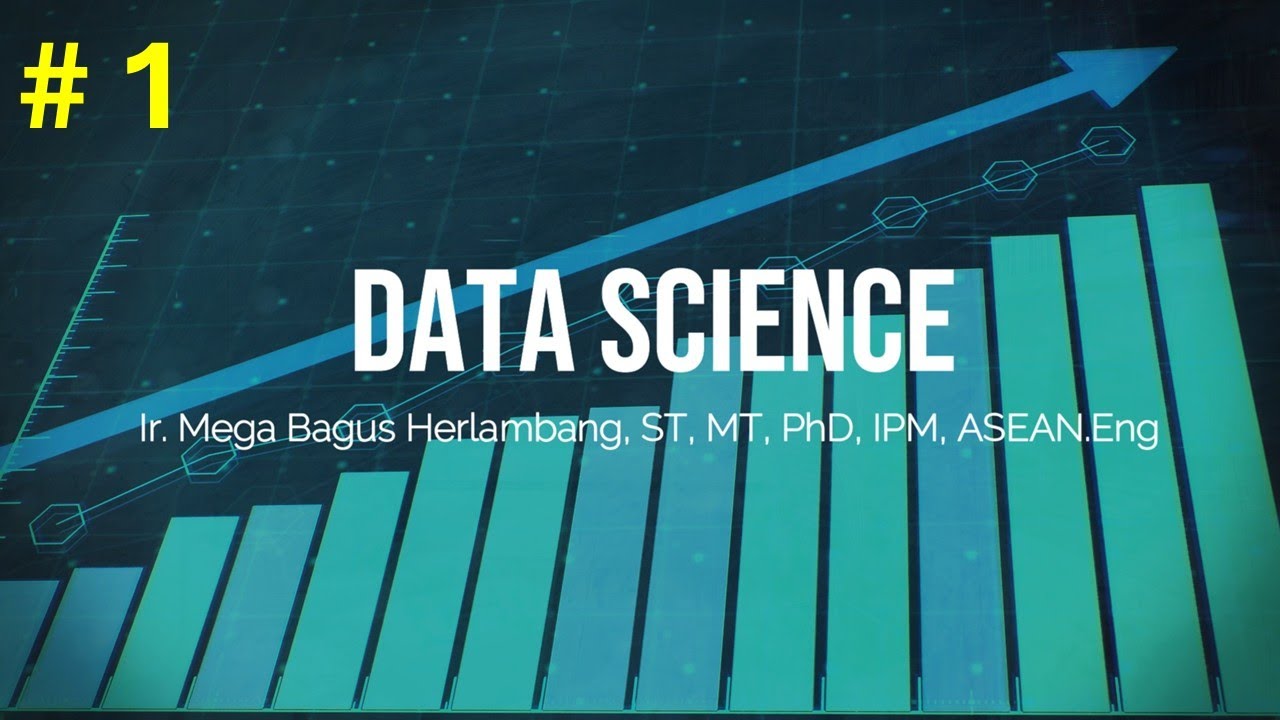
Pertemuan 1 - Data Science
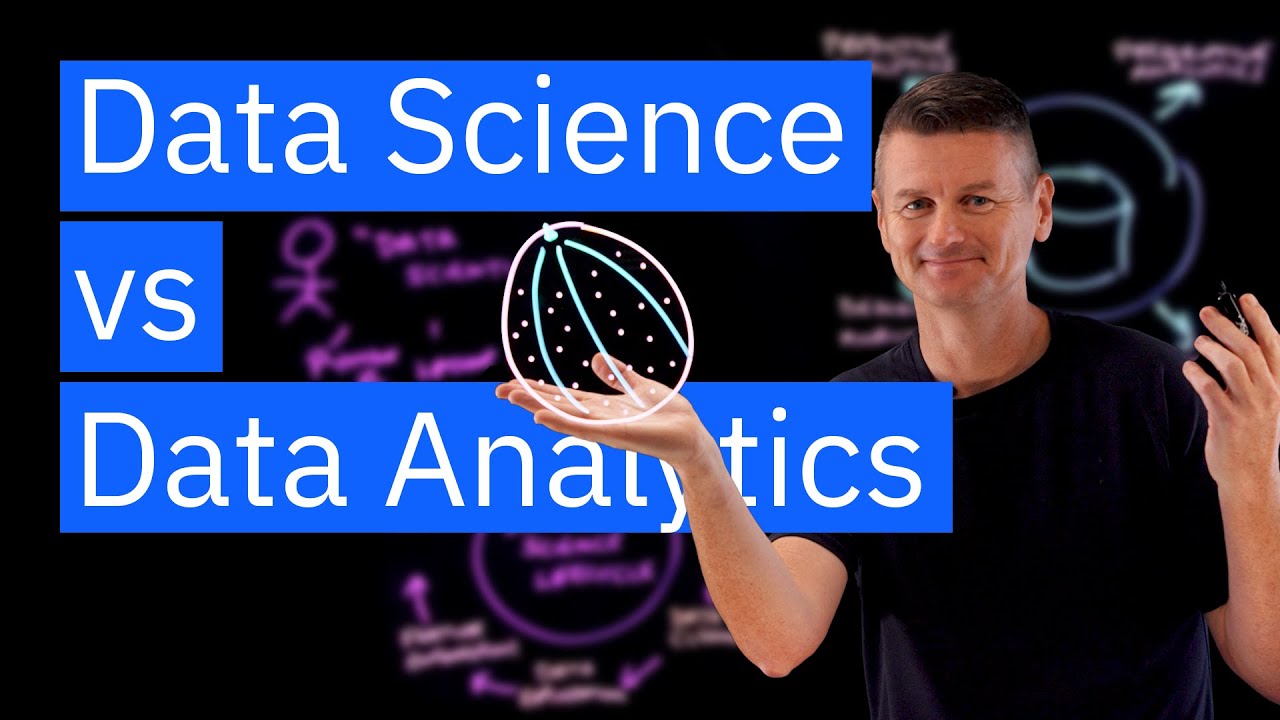
Data Analytics vs Data Science

Types of Business Analytics (Descriptive, Predictive, and Prescriptive)

How is Big Data Analytics Used in Business and Accounting?-Summer 2020 Prof. Ruoxin Wu & Tumah Deen
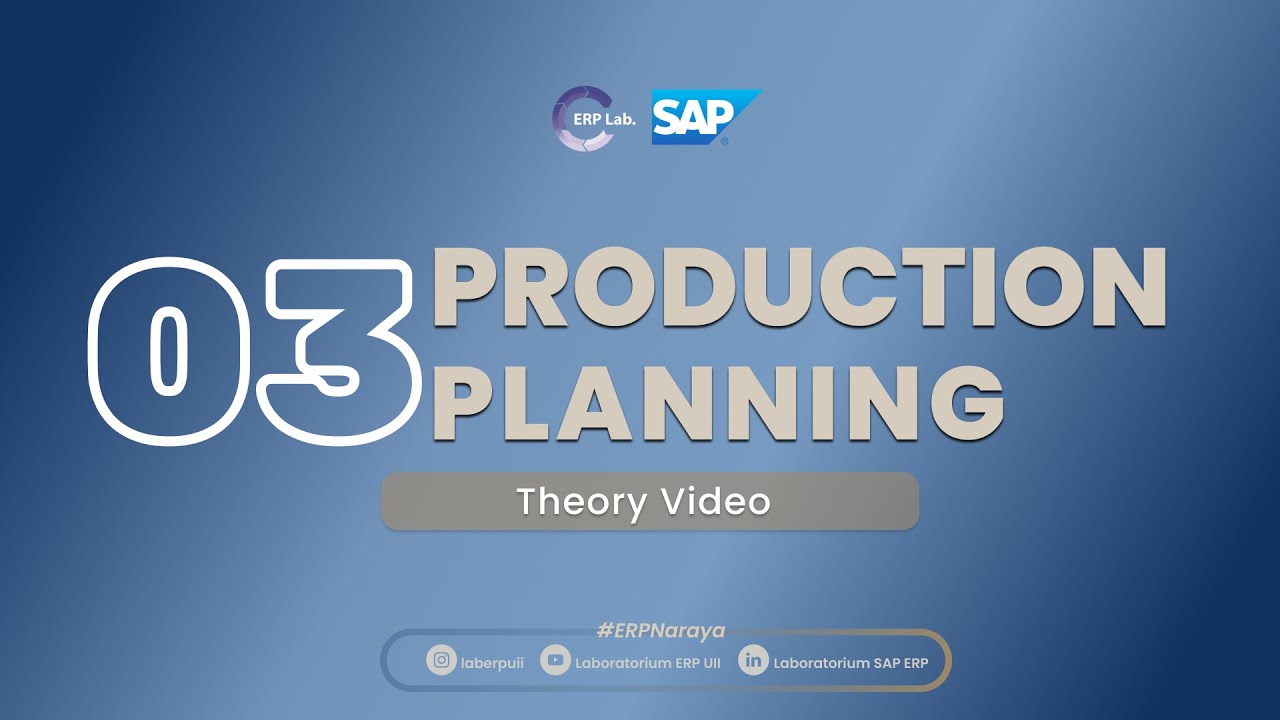
03. Production Planning - Theory
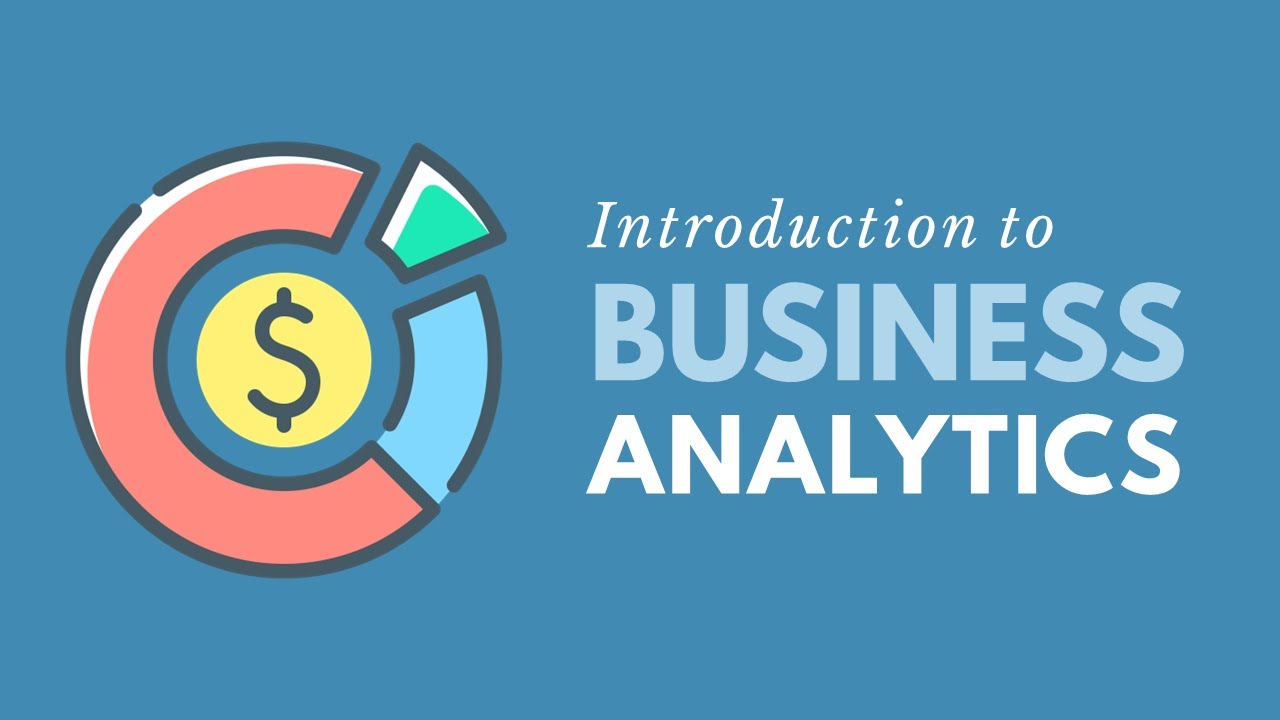
Introduction to Business Analytics (Updated Edition)
5.0 / 5 (0 votes)