MCMC Gibbs Sampler part 2 aproksimasi diskrit
Summary
TLDRThis video explains the application of Bayesian methods for estimating parameters in statistical models, focusing on posterior distributions and approximation techniques. It delves into the use of normal distributions for modeling and how Bayesian inference is applied to estimate parameters such as sigma. The speaker discusses the importance of specifying priors and using data to derive posterior distributions. Additionally, they explore the process of marginalization and its role in calculating posterior estimates, highlighting the practical implementation of these methods for efficient statistical analysis and optimization.
Takeaways
- 😀 The video discusses Bayesian methods for estimating parameters, focusing on posterior distribution and its approximation in discrete actions.
- 😀 The script emphasizes the importance of prior specifications when using Bayesian methods to estimate unknown parameters.
- 😀 The method being discussed is used to estimate the joint distribution of parameters and observations in a model.
- 😀 The video explains that once the posterior distribution is derived, one can make inferences about the parameters like theta and sigma squared.
- 😀 The script mentions the role of discrete approximation methods in overcoming difficulties with posterior distribution.
- 😀 The importance of using normal distributions in the Bayesian framework is highlighted, as it helps model uncertainty in the parameters.
- 😀 One of the key goals is to derive the posterior distribution, enabling the estimation of parameters like theta (peta) and sigma squared.
- 😀 The concept of marginalization is introduced as a technique to integrate over all parameter values in a model.
- 😀 The video references using tools like joint distributions and normal distributions to evaluate posterior probabilities for parameter estimation.
- 😀 The script emphasizes that the approach is computationally manageable, allowing for efficient estimation using numerical methods such as Monte Carlo simulations.
Q & A
What is the main focus of the video script?
-The main focus of the video is on Bayesian methods for estimating the posterior distribution of parameters, specifically for a normal distribution model, and how to approximate this distribution discretely.
What is the importance of posterior distribution in Bayesian statistics?
-The posterior distribution is crucial in Bayesian statistics as it combines the prior information with observed data to estimate unknown parameters. It allows us to refine our predictions and improve estimates based on both prior beliefs and new data.
What is the role of the prior distribution in Bayesian methods?
-In Bayesian methods, the prior distribution represents our beliefs or assumptions about the parameters before seeing the data. It serves as the foundation for updating our understanding when new data becomes available.
How does Bayesian estimation work in this context?
-Bayesian estimation in this context works by specifying a prior distribution for the model parameters, such as the mean and variance of a normal distribution. Once data is observed, we update our beliefs using the likelihood function, resulting in the posterior distribution.
What is the challenge discussed regarding the posterior distribution?
-The challenge discussed is obtaining the posterior distribution in a closed form. For complex models, this can be difficult, and discrete approximation methods are employed to estimate the posterior.
What method is mentioned for approximating the posterior distribution?
-The method mentioned for approximating the posterior distribution is the use of discrete approximations, which involve partitioning the parameter space and using numerical integration techniques to estimate the posterior distribution.
What is the 'join distribution' referred to in the script?
-The 'join distribution' refers to the joint probability distribution of the parameters, which combines both the prior and likelihood to describe the relationship between parameters and data. It is used to compute the posterior distribution.
What does the script say about the use of normal distributions?
-The script mentions using normal distributions to model the data and parameters. The likelihood of the data is modeled as a normal distribution, and posterior estimates of parameters like the mean and variance are also assumed to follow normal distributions.
Why is it important to compute the posterior distribution?
-Computing the posterior distribution is important because it allows for the estimation of unknown parameters in the model based on observed data. With this distribution, we can make predictions, calculate uncertainties, and perform inference on the parameters.
What is the significance of the 'marginal' discussed in the script?
-The marginal refers to the integral of the joint distribution over the other parameters. It helps in deriving the distribution of a single parameter while accounting for the uncertainty in the other parameters, which is necessary for simplifying the analysis.
Outlines
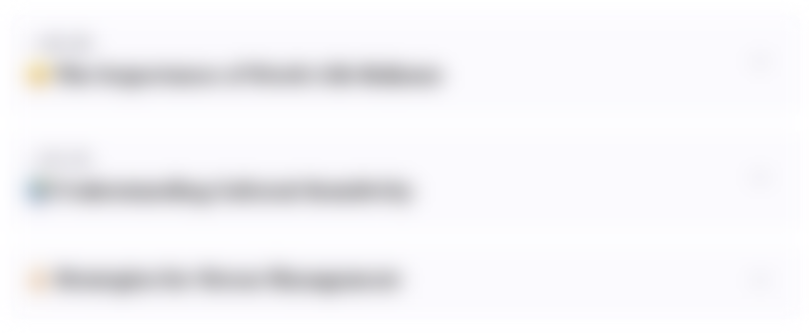
此内容仅限付费用户访问。 请升级后访问。
立即升级Mindmap
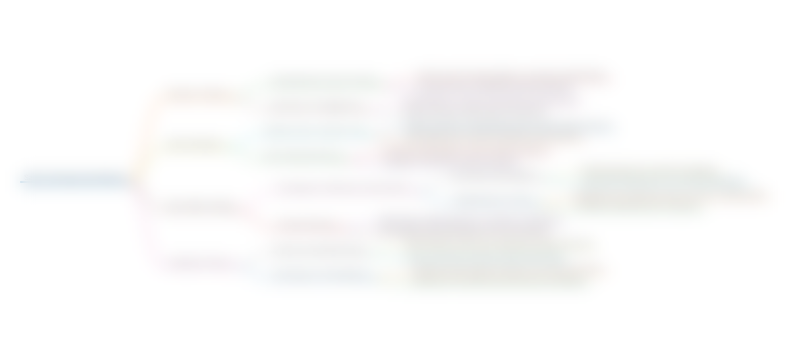
此内容仅限付费用户访问。 请升级后访问。
立即升级Keywords
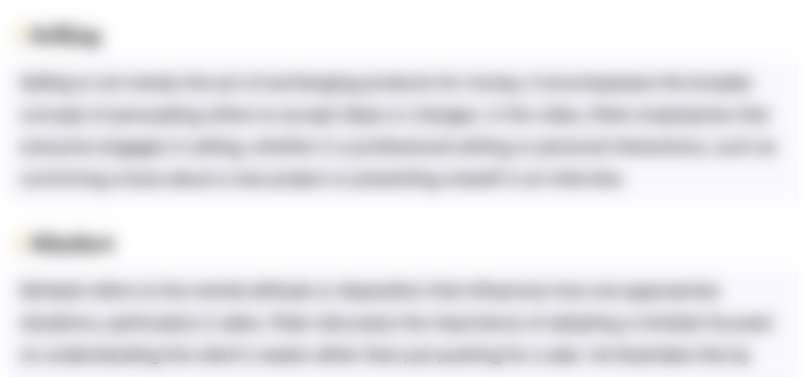
此内容仅限付费用户访问。 请升级后访问。
立即升级Highlights
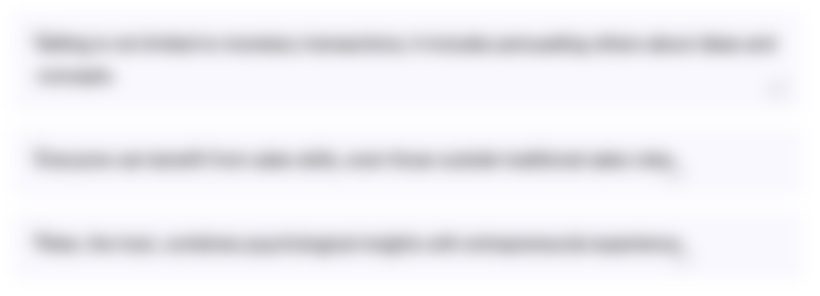
此内容仅限付费用户访问。 请升级后访问。
立即升级Transcripts
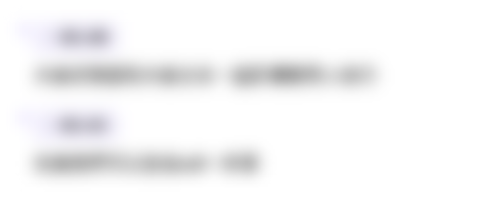
此内容仅限付费用户访问。 请升级后访问。
立即升级5.0 / 5 (0 votes)