MCMC Gibbs Sampler part 1 pendahuluan
Summary
TLDRThis lecture discusses posterior approximation using chipset techniques, focusing on parameter estimation. It revisits prior models and how to estimate parameters when certain conditions are met, such as assuming a normal distribution. The lecture addresses the limitations of these assumptions and explores alternative methods, including using approximation techniques and distribution assembly to estimate parameters. The aim is to provide strategies for situations where standard conditions aren't met and to optimize posterior estimation effectively.
Takeaways
- 😀 The lecture discusses posterior approximation using chipsets.
- 😀 Previous lessons focused on models and a single parameter with given variations.
- 😀 The parameter discussed is symbolized and was previously analyzed with a normal distribution and variance.
- 😀 The prior used in earlier lessons resulted in a normal distribution with a standard deviation variance.
- 😀 Sometimes we aim to explore conditions where dependencies between parameters are relaxed.
- 😀 The lecture emphasizes how to handle joint parameter estimation.
- 😀 Estimations are made using the distribution of normal parameters and sigma.
- 😀 The process is easy when certain conditions, such as variance, are met.
- 😀 The challenge arises when these conditions do not hold, and the lecture addresses this scenario.
- 😀 Two methods for parameter estimation are discussed: approximation and assembling distributions for posterior analysis.
Q & A
What is the focus of the lecture discussed in the script?
-The lecture focuses on posterior approximation using Bayesian methods, specifically for a single parameter model where the variance is provided.
What was covered in the previous video before this lecture?
-The previous video discussed various models, specifically focusing on a single parameter model with a given variance.
How is the parameter for this model represented in the script?
-The parameter is represented with a symbol (likely referring to a Bayesian parameter), and it is noted that this model works with a known variance.
What does the script mention about the prior in the model?
-The script mentions using a prior distribution where the variance is fixed, leading to a normal distribution with a defined variance.
What is the significance of using a normal distribution in this context?
-The normal distribution is significant because it allows for the calculation of estimations and posterior distributions in Bayesian analysis, especially with a known variance.
Why might one want to use a joint distribution in this context?
-Using a joint distribution is useful when multiple parameters or factors interact, and the goal is to estimate or understand their combined effects.
What problem is the lecture aiming to address?
-The lecture aims to address the issue of estimating parameters or handling posterior distributions when certain conditions (such as variance) do not hold or are not fully met.
What are the two methods discussed for handling posterior estimation?
-The two methods discussed are using approximation techniques (specifically using a 'scrub' approximation) and assembling the distribution from a combination of other techniques.
What is the importance of posterior distribution in Bayesian analysis?
-The posterior distribution is crucial in Bayesian analysis because it represents updated beliefs about parameters after observing data, and it forms the basis for parameter estimation.
What does the script suggest when traditional conditions are not met in parameter estimation?
-The script suggests using approximation methods or joint distribution techniques to handle situations where traditional conditions for estimation (like fixed variance) are not met.
Outlines
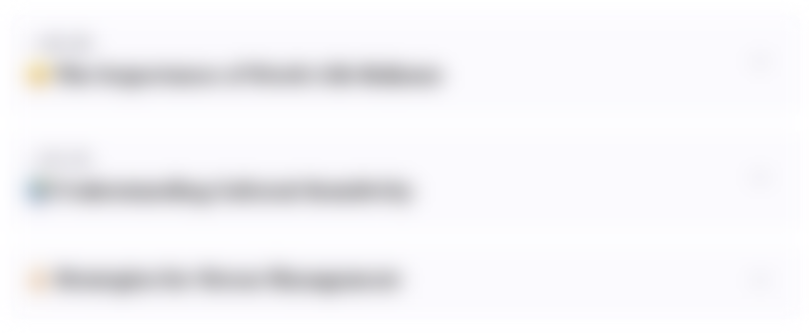
This section is available to paid users only. Please upgrade to access this part.
Upgrade NowMindmap
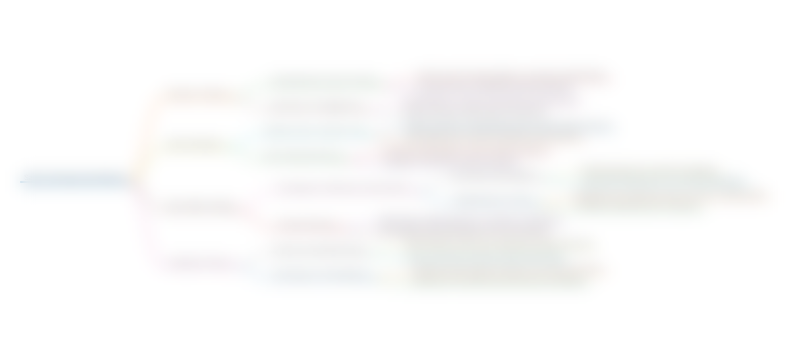
This section is available to paid users only. Please upgrade to access this part.
Upgrade NowKeywords
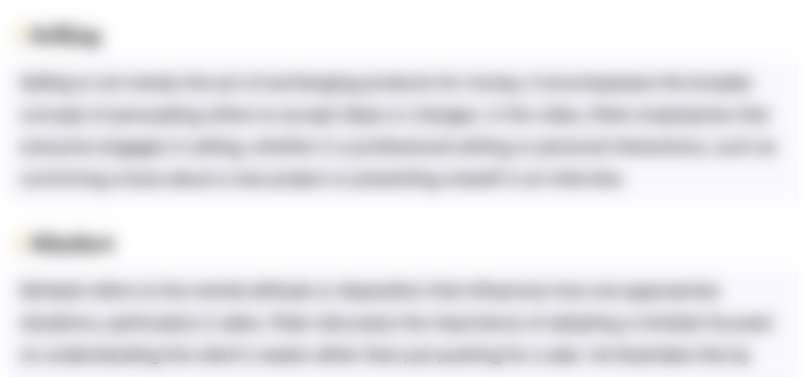
This section is available to paid users only. Please upgrade to access this part.
Upgrade NowHighlights
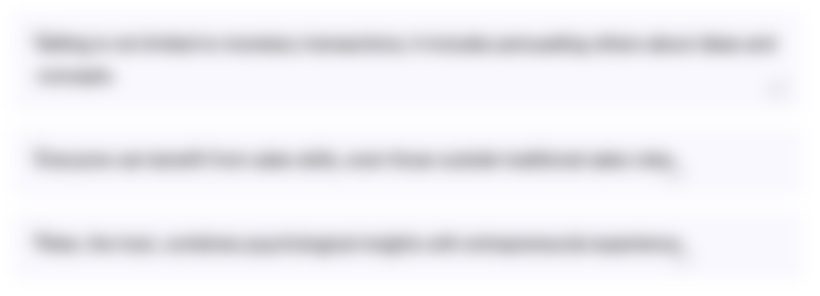
This section is available to paid users only. Please upgrade to access this part.
Upgrade NowTranscripts
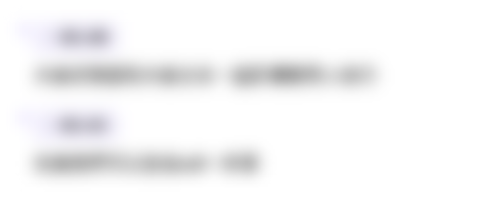
This section is available to paid users only. Please upgrade to access this part.
Upgrade Now5.0 / 5 (0 votes)