Measures of Spread: Crash Course Statistics #4
Summary
TLDRIn this episode of Crash Course Statistics, Adriene Hill explores measures of spread, which describe how data is distributed around the central tendency. She explains concepts like range, interquartile range (IQR), variance, and standard deviation, using real-life examples like YouTube audience demographics and sports statistics. These measures help to understand data variability and assess the reliability of conclusions drawn from the mean or median. The video highlights how extreme values can skew results and emphasizes the importance of considering the spread of data for more accurate interpretations in various contexts.
Takeaways
- 😀 Measures of spread tell us how data is distributed around the 'middle' (mean or median) of a dataset, providing insight into how reliable the central tendency is.
- 😀 The Range is the simplest measure of spread, calculated by subtracting the smallest value from the largest, but it only considers the extremes and ignores the middle values.
- 😀 The InterQuartile Range (IQR) focuses on the spread of the middle 50% of data, providing a better sense of the 'core' audience, especially in the context of YouTube viewer ages.
- 😀 Variance considers the spread of all data points by calculating the average of squared deviations from the mean, giving a more comprehensive picture of data variability.
- 😀 Sample variance is adjusted by dividing by the number of samples minus 1 to eliminate bias, making it a better estimate for population variance.
- 😀 Extreme values (outliers) can greatly influence the mean and variance, as seen in the example of Muggle-born Quidditch players whose times skew the data.
- 😀 Standard deviation, the square root of variance, brings the units back to a more understandable level and tells us the average deviation from the mean.
- 😀 A smaller standard deviation indicates that data points are clustered closely around the mean, while a larger standard deviation suggests greater variability in the data.
- 😀 It's important to be aware of outliers as they can distort both the mean and the spread of data, potentially leading to misleading conclusions.
- 😀 Measures of spread are valuable not just for statisticians, but for everyone, as they give a more complete picture of data beyond the average and help us avoid misinterpretation.
Q & A
What is the difference between central tendency and measures of spread?
-Central tendency refers to the 'middle' of a dataset, usually measured by the mean or median, while measures of spread describe how data is distributed around that middle. They provide insights into how varied or clustered the data is.
What does the range of a dataset tell us?
-The range gives us the difference between the highest and lowest values in a dataset, showing the extent to which the data is spread out. However, it can be influenced heavily by outliers or extreme values.
How does the Interquartile Range (IQR) differ from the range?
-The IQR measures the spread of the middle 50% of the data, ignoring extreme values (outliers). It is calculated by finding the difference between the 75th percentile (Q3) and the 25th percentile (Q1), giving a more robust measure of spread compared to the range.
Why is the IQR useful in understanding a dataset's core audience?
-The IQR helps identify the central portion of a dataset by focusing on the middle 50%, excluding extreme values. This makes it particularly useful for understanding the core audience in applications like analyzing YouTube viewership, where the range might be influenced by very young or very old viewers.
What is variance and how is it calculated?
-Variance measures how much individual data points differ from the mean. It is calculated by subtracting the mean from each data point, squaring the result, summing those squared differences, and then dividing by the number of data points minus 1 (for sample variance).
Why is the variance of a sample divided by (n-1)?
-The variance of a sample is divided by (n-1) to correct for bias, ensuring that the sample variance is a better estimate of the population variance. This adjustment, known as Bessel's correction, compensates for the fact that a sample tends to underestimate the population variance.
What is the unit of variance, and why might this be a problem?
-The unit of variance is the square of the unit of the data, such as 'seconds squared' or 'wins squared'. This can be problematic because squared units are not directly interpretable in real-world terms, which is why variance is often followed by the calculation of standard deviation.
How does standard deviation differ from variance?
-Standard deviation is the square root of variance, which brings the units back to a more interpretable form (such as seconds or wins). It provides a clearer understanding of how much data points deviate from the mean, making it easier to interpret compared to variance.
What role do outliers play in measures of spread like variance and standard deviation?
-Outliers can significantly inflate measures of spread, particularly variance and standard deviation. Since both are sensitive to extreme values, even a few outliers can distort the overall understanding of the data's distribution.
How can measures of spread help when interpreting averages in real life?
-Measures of spread, such as standard deviation, can provide context for averages, helping to determine how representative the average is. For example, a low standard deviation means that the data points are close to the average, while a high standard deviation suggests more variation, making the average less reliable.
Outlines
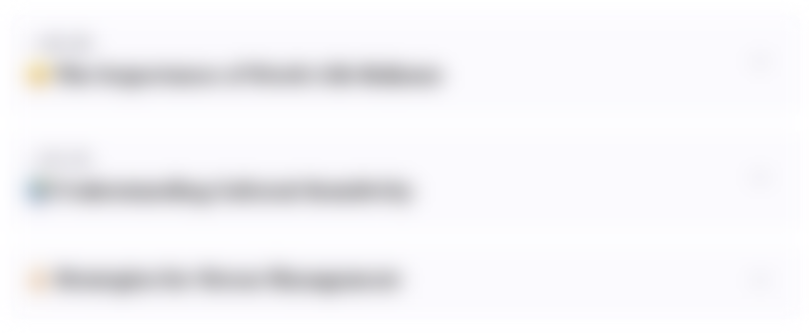
此内容仅限付费用户访问。 请升级后访问。
立即升级Mindmap
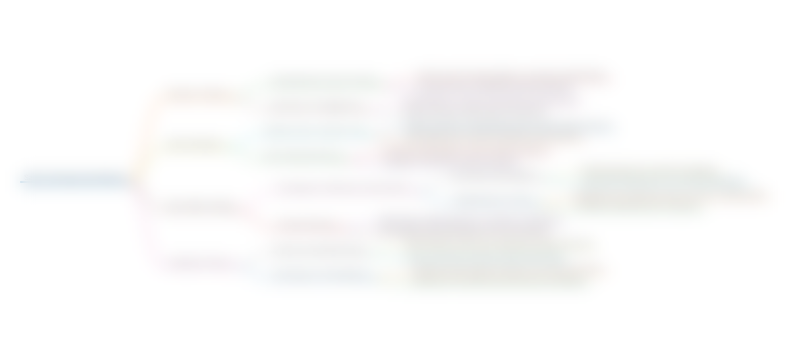
此内容仅限付费用户访问。 请升级后访问。
立即升级Keywords
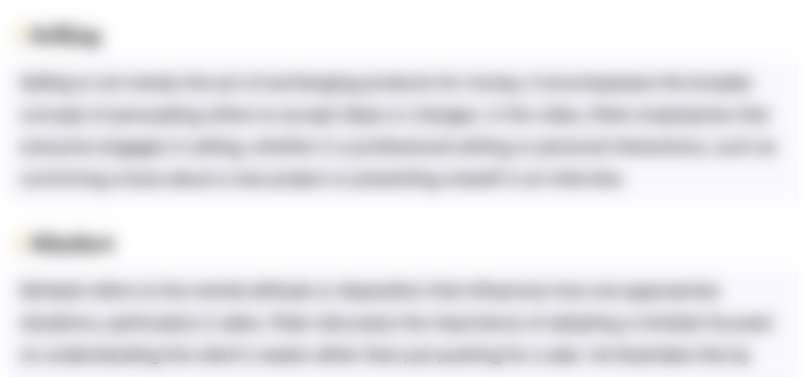
此内容仅限付费用户访问。 请升级后访问。
立即升级Highlights
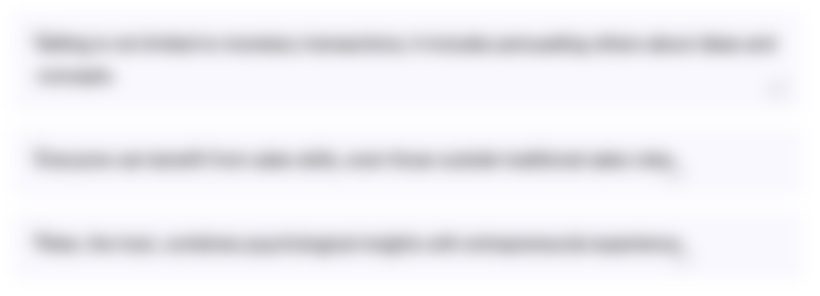
此内容仅限付费用户访问。 请升级后访问。
立即升级Transcripts
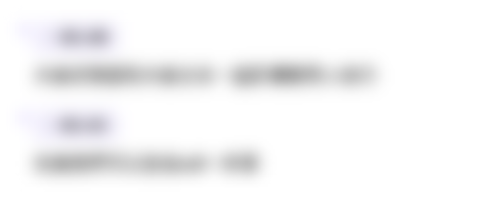
此内容仅限付费用户访问。 请升级后访问。
立即升级浏览更多相关视频
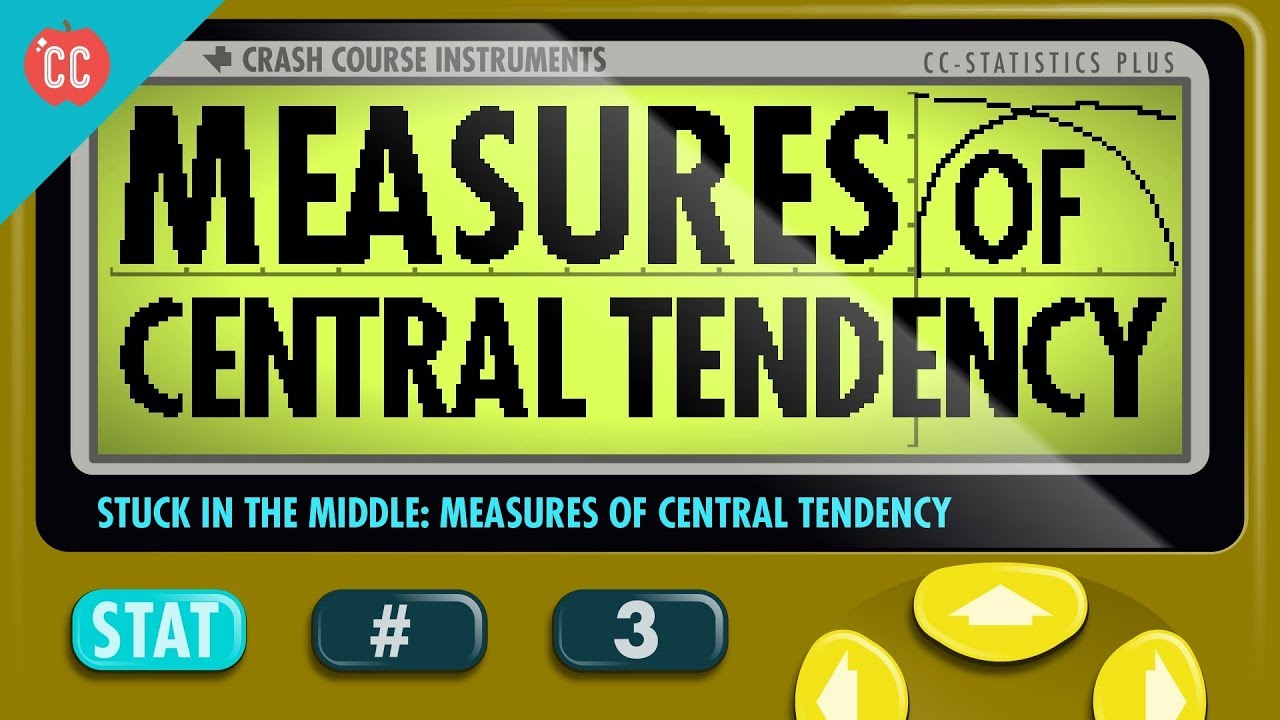
Mean, Median, and Mode: Measures of Central Tendency: Crash Course Statistics #3
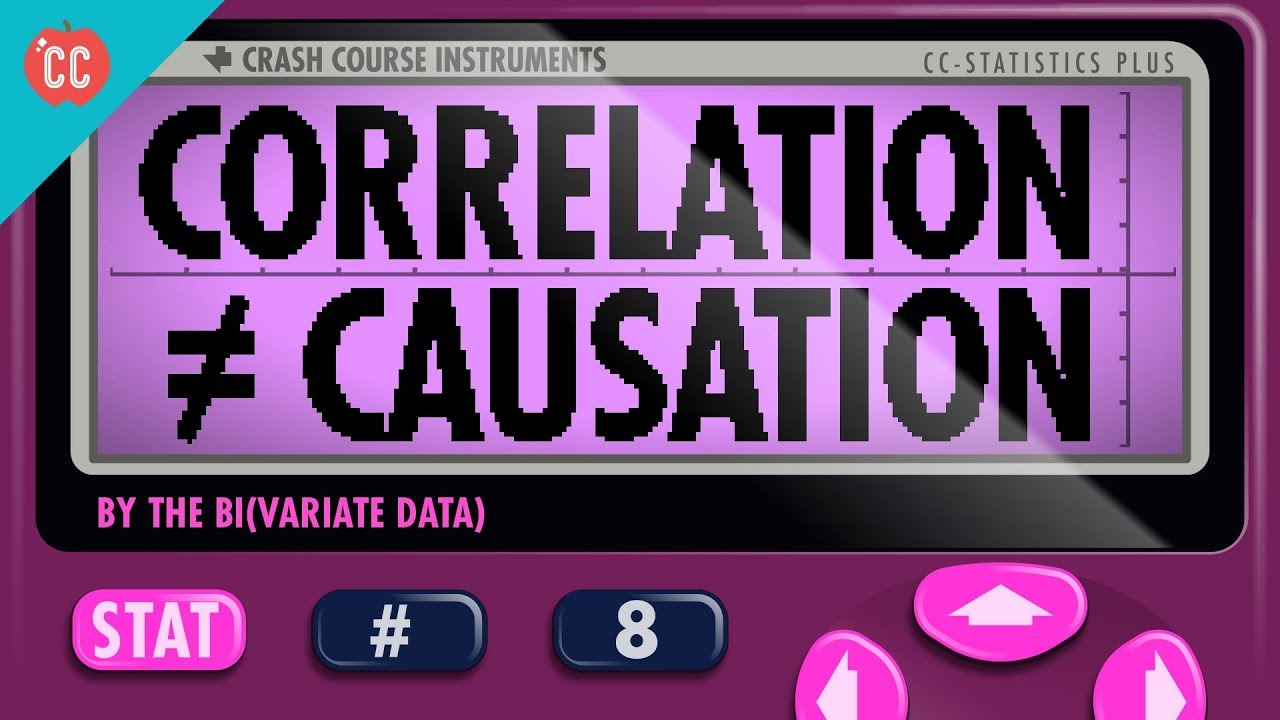
Correlation Doesn't Equal Causation: Crash Course Statistics #8

ANOVA: Crash Course Statistics #33
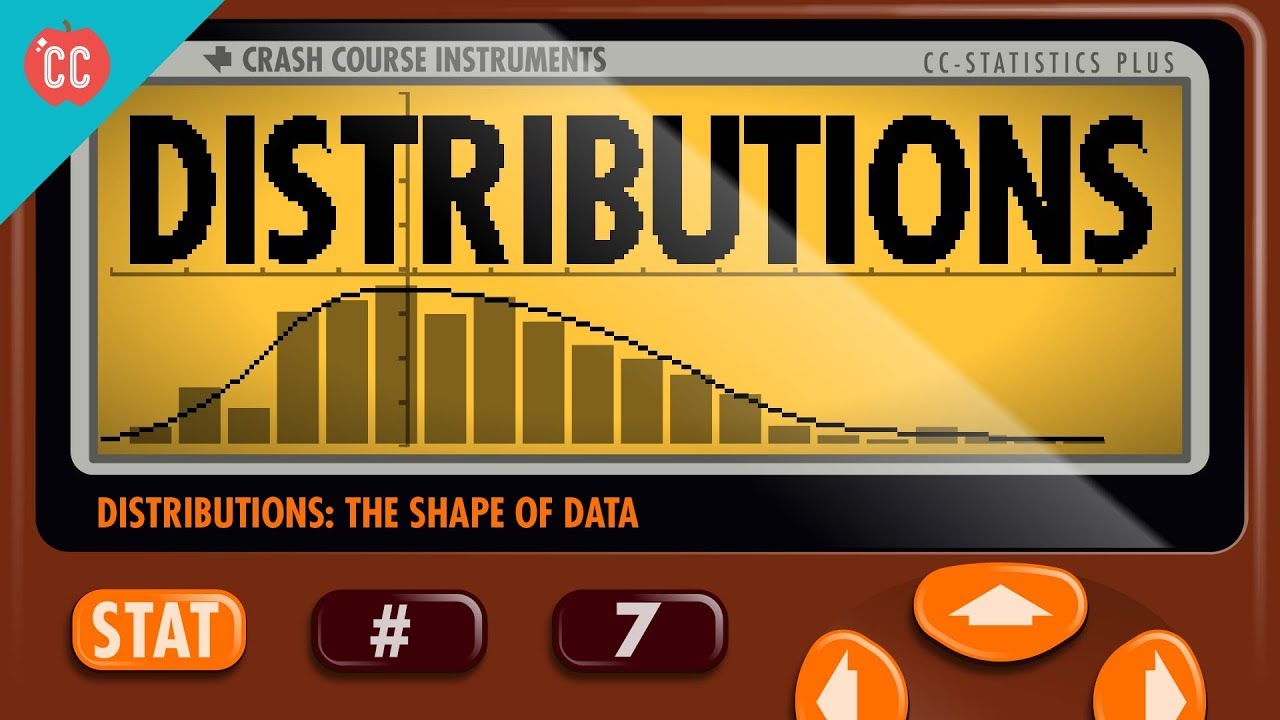
The Shape of Data: Distributions: Crash Course Statistics #7
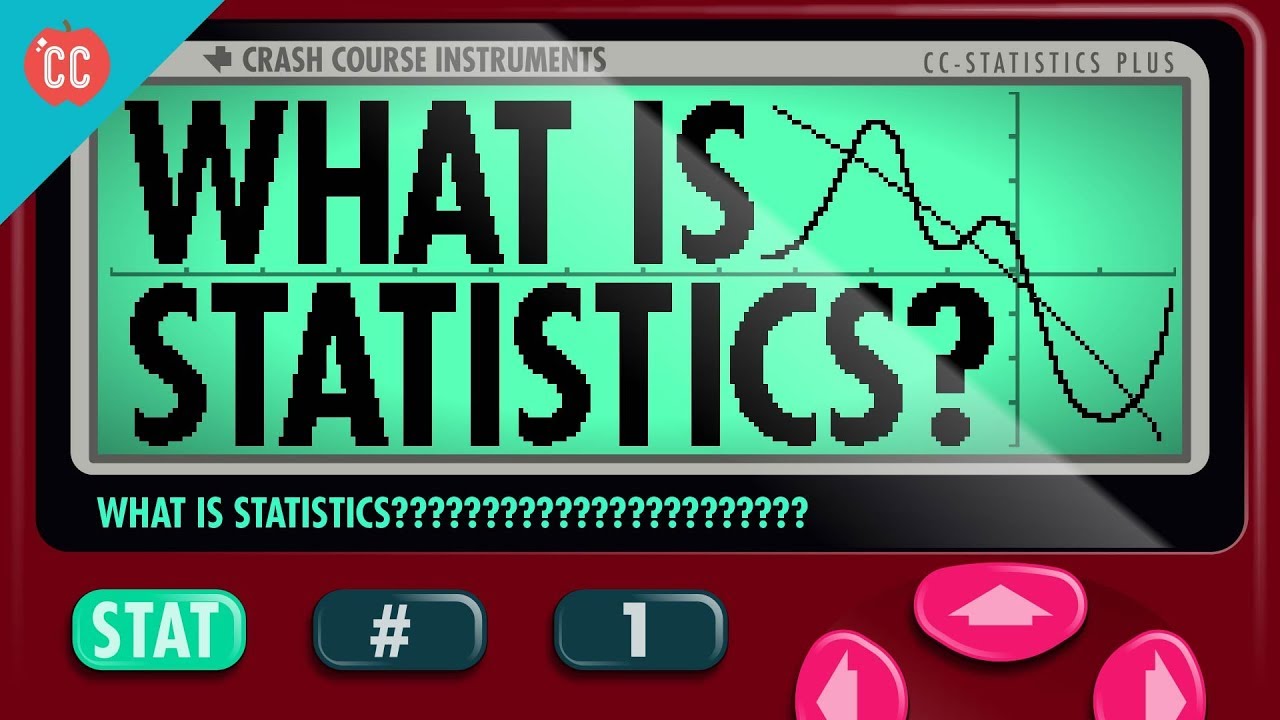
What Is Statistics: Crash Course Statistics #1
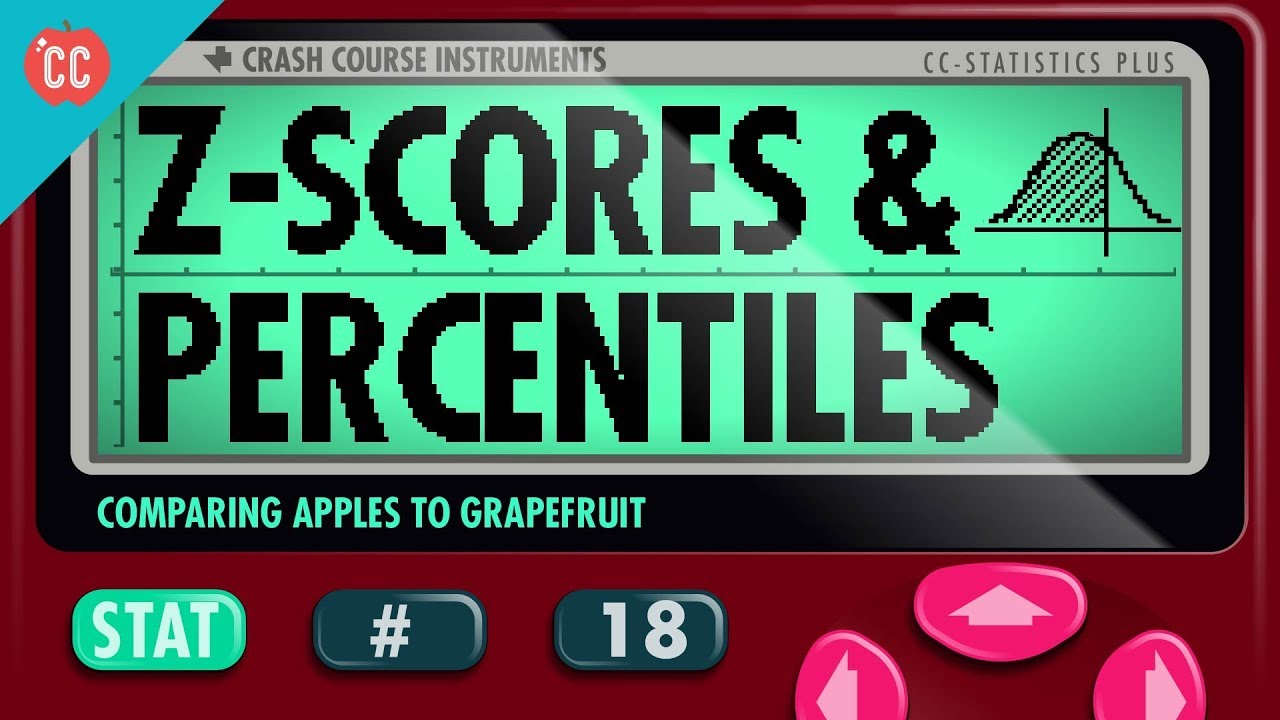
Z-Scores and Percentiles: Crash Course Statistics #18
5.0 / 5 (0 votes)