Interpretation HD 720p
Summary
TLDRThis lecture focuses on the critical role of interpretation in the data analysis process. It emphasizes that interpretation is continuous and iterative, influencing all stages of analysis. Key principles discussed include revisiting the original question, considering the direction, magnitude, and uncertainty of results, and evaluating the totality of evidence from primary and secondary models. The lecture also highlights the importance of integrating existing knowledge and considering the implications for decision-making, taking into account stakeholder values and goals. Ultimately, effective interpretation ensures that analysis leads to meaningful insights and informed decisions.
Takeaways
- 😀 Interpretation in data analysis is a continuous process that happens throughout the entire analysis, not just at the end.
- 😀 The process of interpreting results is iterative and requires revisiting earlier stages like exploratory data analysis and formal modeling.
- 😀 Revisit the original question to ensure your analysis remains focused and aligned with the goals of the study.
- 😀 Interpretation should account for directionality, magnitude, and uncertainty in your results.
- 😀 Directionality refers to the nature of the relationship (positive, negative, or neutral) between variables, especially in causal or inferential questions.
- 😀 Magnitude is the size of the effect or association. Assess whether it is meaningful and relevant in the context of the analysis.
- 😀 Uncertainty is an important aspect of interpretation; use continuous measures like confidence intervals instead of binary significance tests.
- 😀 Secondary models and sensitivity analyses provide additional context for interpreting results, and should be evaluated using the same factors of directionality, magnitude, and uncertainty.
- 😀 The totality of evidence—combining primary and secondary models—should be considered when interpreting results and comparing them to existing literature or knowledge.
- 😀 The implications of your findings should inform decisions, guiding next steps or actions. Different stakeholders may interpret the evidence based on their unique values and priorities.
Q & A
What is the role of interpretation in the data analysis process?
-Interpretation in data analysis is a continuous and iterative process that happens throughout the entire analysis. It is vital for understanding the results and aligning them with the expectations set during the initial stages of the analysis.
Why is revisiting the original question important during interpretation?
-Revisiting the original question helps ensure that the analysis stays focused on the right problem and that any potential divergence from the original question is recognized. This step helps avoid misinterpretation and ensures that the results align with the question being asked.
What are the three key aspects of primary results that should be considered during interpretation?
-The three key aspects to consider when interpreting primary results are: directionality (positive, negative, or zero association), magnitude (size or significance of the effect), and uncertainty (confidence in the result's accuracy and reliability).
How should uncertainty be handled during interpretation?
-Uncertainty should be considered through continuous measures such as confidence intervals, rather than binary measures like statistical significance. This helps assess the range of possible values for the result and the degree of confidence in the findings.
What is the importance of considering secondary models in the interpretation process?
-Secondary models provide additional evidence that can help validate or challenge the primary model's results. By comparing directionality, magnitude, and uncertainty across different models, you can better understand the robustness and reliability of your findings.
What is meant by the 'totality of the evidence' in data interpretation?
-The 'totality of the evidence' refers to all the information generated from both primary and secondary models, as well as any pre-existing knowledge from literature or other sources. This total evidence should be evaluated together to draw more accurate and informed conclusions.
How do you ensure that the results are not biased during interpretation?
-To avoid bias, it's important to evaluate the sampling process used in the analysis. Ensure that the sample accurately represents the population you're studying, and consider potential biases in the selection of data or the sampling methods.
What are the potential implications of findings in data analysis?
-The implications of findings could inform decisions or actions to be taken based on the results. For example, in causal or inferential studies, the findings might lead to designing new studies, interventions, or controlled trials, depending on the outcome of the analysis.
How should the directionality of results be interpreted?
-Directionality refers to the type of association between variables—whether it's positive, negative, or zero. It's important to compare this with your expectations. If the direction of the result differs from what you expected, it could indicate a misalignment in your modeling approach or a lack of a real association.
Why is it important to consider both primary and secondary models during interpretation?
-Considering both primary and secondary models allows you to evaluate the consistency and robustness of your results. If different models produce similar findings, this strengthens your confidence in the conclusions. If they diverge, it may signal issues such as model misspecification or confounding factors.
Outlines
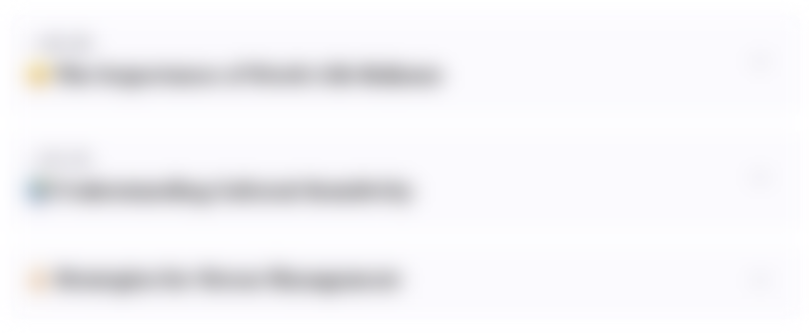
此内容仅限付费用户访问。 请升级后访问。
立即升级Mindmap
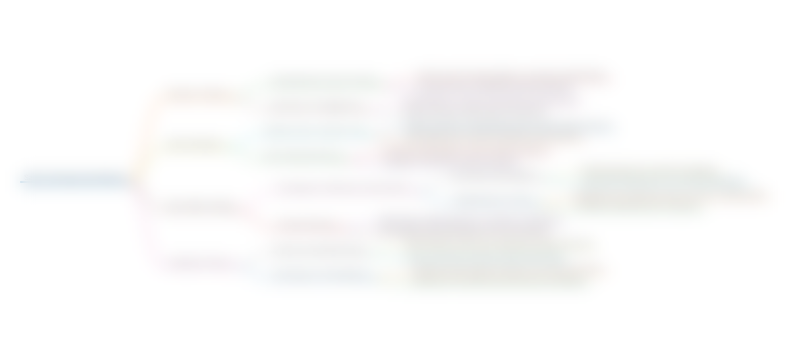
此内容仅限付费用户访问。 请升级后访问。
立即升级Keywords
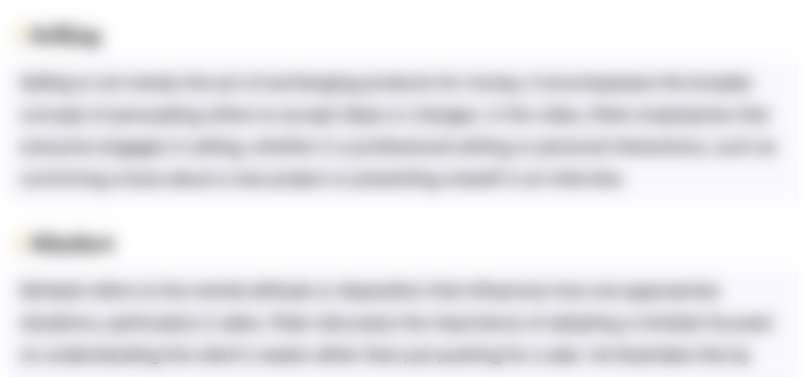
此内容仅限付费用户访问。 请升级后访问。
立即升级Highlights
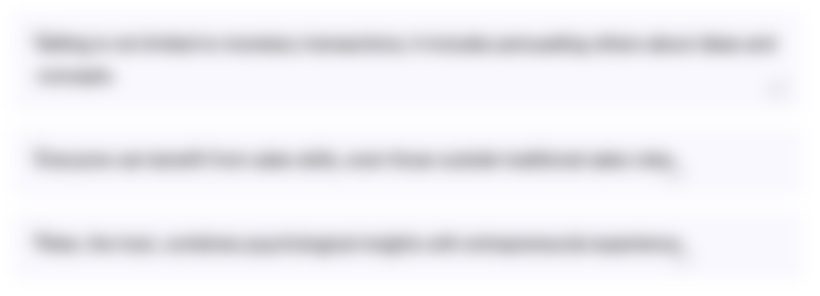
此内容仅限付费用户访问。 请升级后访问。
立即升级Transcripts
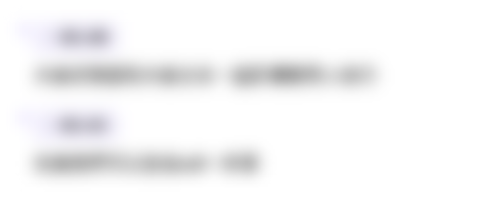
此内容仅限付费用户访问。 请升级后访问。
立即升级浏览更多相关视频

Methods - 0 - Introduction
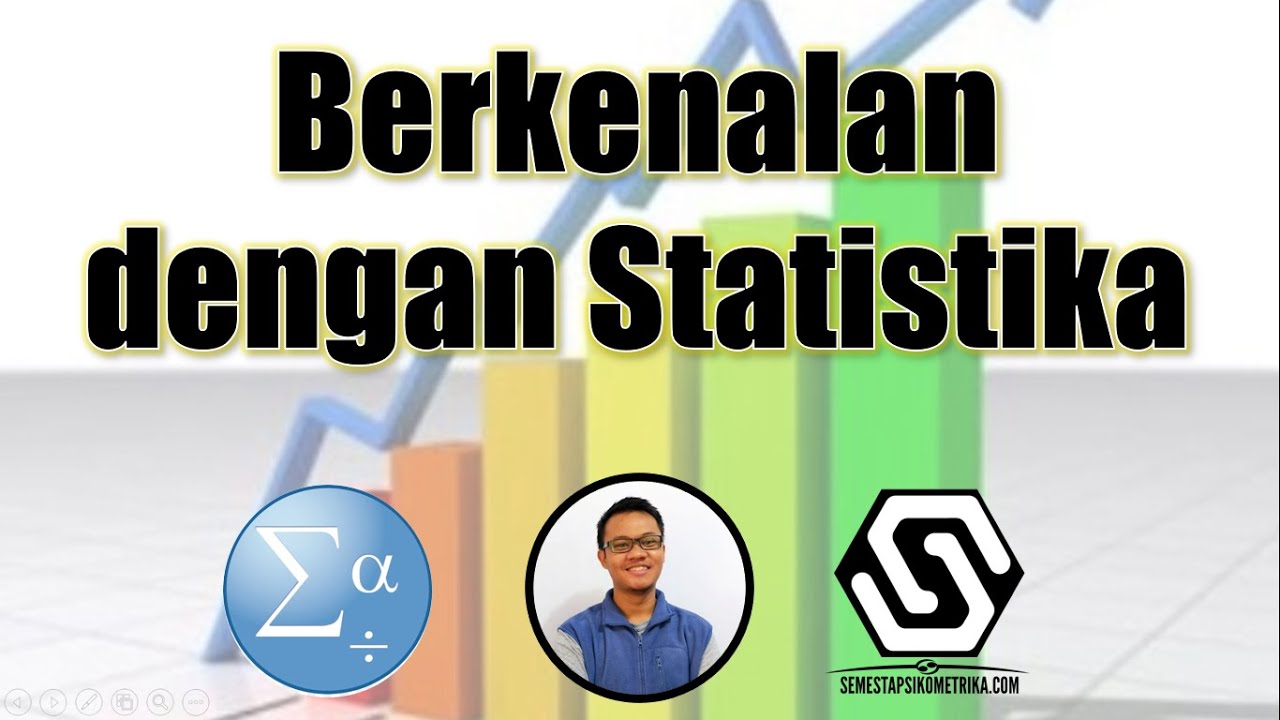
Berkenalan dengan Statistika
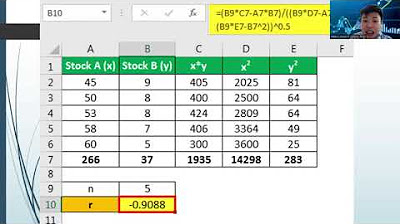
Statistics Lecture 5 Test of Relationship

Корреляция горизонта и разлома по сейсмическому кубу. Построение карты с учётом разлома.
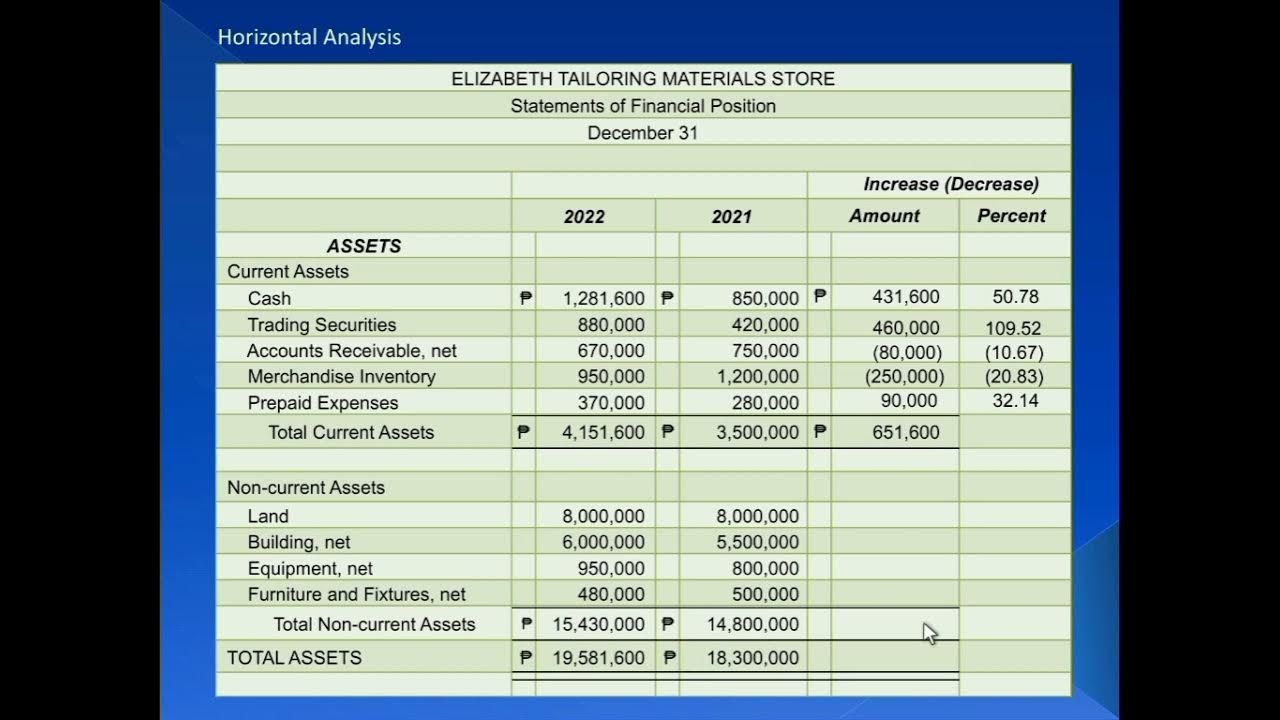
Part 1: Financial Statements Analysis (Intro, Horizontal Analysis and Vertical Analysis)
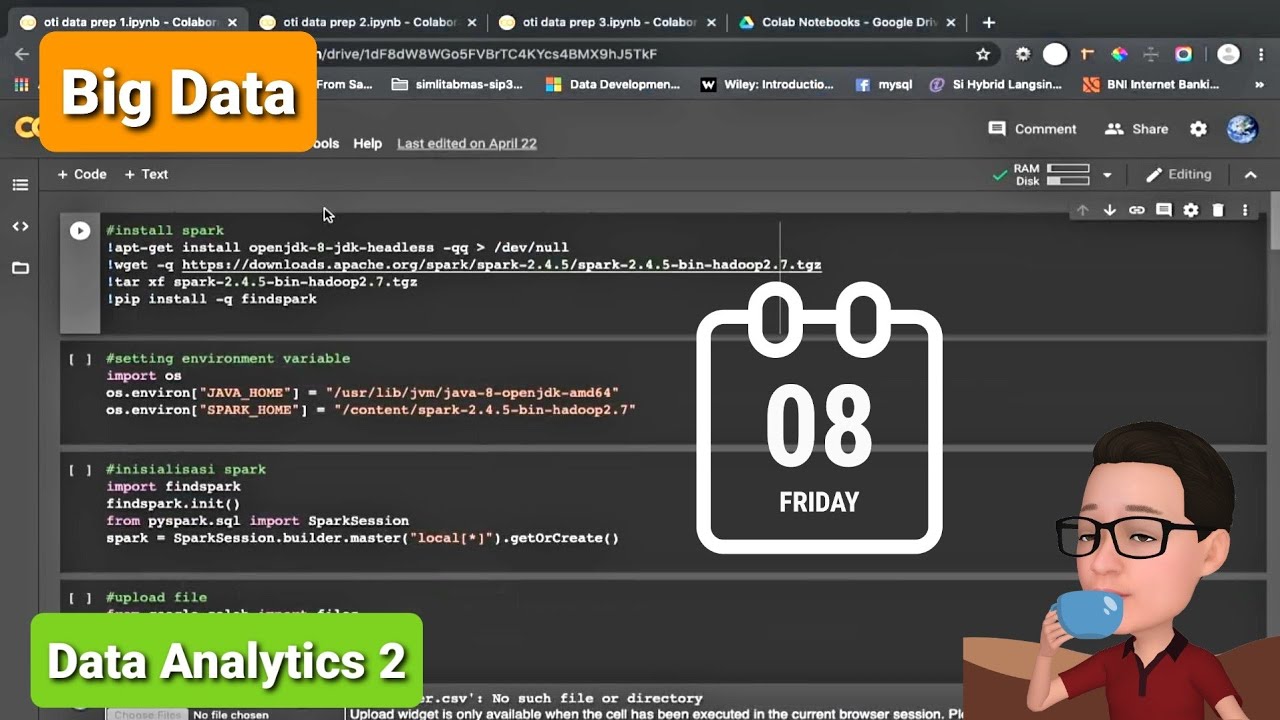
Big Data Analytics 02 | Data Preparation | Kuliah Online Big Data Pertemuan 11
5.0 / 5 (0 votes)