What is inferential statistics? Explained in 6 simple Steps.
Summary
TLDRThis video explains inferential statistics, which helps us draw conclusions about a population based on sample data. It covers key concepts like hypothesis testing, P values, and statistical significance. The process involves formulating a hypothesis, conducting tests to check the null hypothesis, and interpreting results based on P values. Viewers also learn about Type 1 and Type 2 errors, and how statistical tools can help identify the right tests for data analysis. The video provides practical guidance for interpreting hypothesis test results and using software for analysis, with a teaser for the next video on parametric vs. non-parametric tests.
Takeaways
- 😀 Inferential statistics allows us to make conclusions about a population based on sample data.
- 😀 The population refers to the entire group of interest, while a sample is a smaller subset chosen from the population.
- 😀 Hypothesis testing is a method for testing claims about a population parameter using sample data.
- 😀 The null hypothesis assumes no effect or difference in the population, while the alternative hypothesis suggests there is an effect.
- 😀 To test a hypothesis, we first assume the null hypothesis is true and calculate the probability of obtaining the sample result.
- 😀 The P-value represents the probability of getting sample data that deviates as much as or more than our sample under the null hypothesis.
- 😀 A small P-value (usually < 0.05) indicates that the sample data is inconsistent with the null hypothesis, leading to its rejection.
- 😀 Statistical significance occurs when the P-value is below a threshold (e.g., 0.05), meaning the result is unlikely to have happened by chance.
- 😀 Type I error happens when a true null hypothesis is wrongly rejected, while Type II error occurs when a false null hypothesis is not rejected.
- 😀 Hypothesis tests can be conducted using tools like Data.net, which suggest appropriate tests and help interpret the results.
Q & A
What is the main purpose of inferential statistics?
-Inferential statistics allows us to make conclusions or inferences about a population based on data from a sample.
What is the difference between a population and a sample in statistical analysis?
-The population refers to the entire group of interest, while the sample is a smaller, selected subset of that population used to draw conclusions about the whole group.
Can you explain the concept of hypothesis testing?
-Hypothesis testing is a method used to assess a claim or assumption about a population based on sample data. It involves testing the null hypothesis and determining if there’s enough evidence to reject it in favor of an alternative hypothesis.
What is the null hypothesis and why is it important?
-The null hypothesis is the assumption that there is no effect or difference in the population. It is important because hypothesis testing starts with assuming the null hypothesis is true and tests whether the data suggests otherwise.
How is the P-value used in hypothesis testing?
-The P-value represents the probability of obtaining a sample result as extreme as or more extreme than the observed result, assuming the null hypothesis is true. A low P-value indicates strong evidence against the null hypothesis.
What does a small P-value indicate in statistical tests?
-A small P-value (typically less than 0.05) suggests that the sample data is inconsistent with the null hypothesis, leading us to reject the null hypothesis and support the alternative hypothesis.
What is statistical significance and how is it determined?
-Statistical significance occurs when the P-value is below a predetermined threshold (usually 0.05). It indicates that the observed result is unlikely to have occurred by chance alone, and there is enough evidence to reject the null hypothesis.
What are Type I and Type II errors in hypothesis testing?
-A Type I error occurs when a true null hypothesis is incorrectly rejected, meaning we falsely conclude there is an effect. A Type II error occurs when a false null hypothesis is not rejected, meaning we fail to detect a real effect.
Why is it important to select the correct hypothesis test?
-Selecting the correct hypothesis test ensures that the results are valid and reliable. The type of data and the specific research question determine which test is appropriate for drawing accurate conclusions.
How does data analysis software help in hypothesis testing?
-Data analysis software, like the one mentioned in the video, helps by suggesting the correct hypothesis test based on the data, calculating test statistics, and interpreting the results, including the P-value and statistical significance.
Outlines
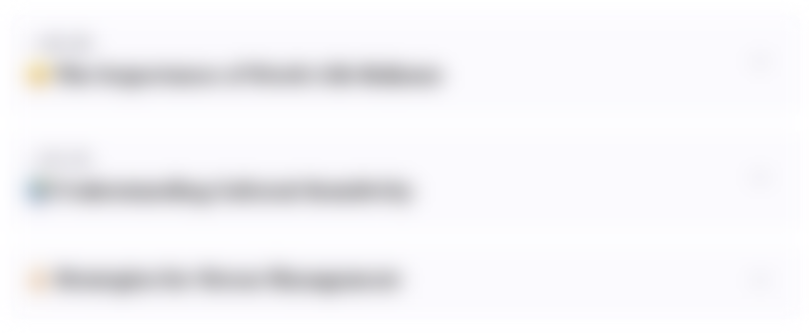
此内容仅限付费用户访问。 请升级后访问。
立即升级Mindmap
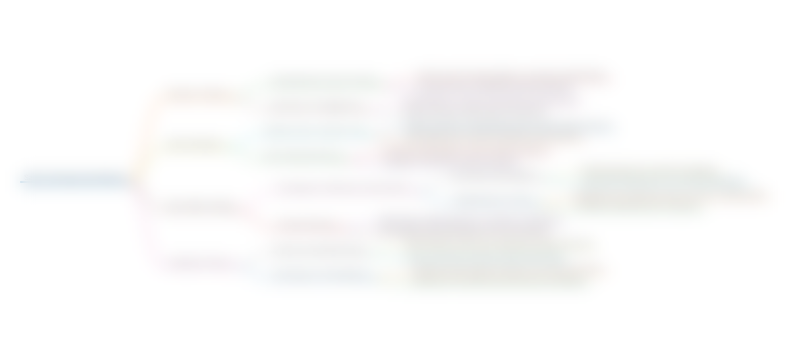
此内容仅限付费用户访问。 请升级后访问。
立即升级Keywords
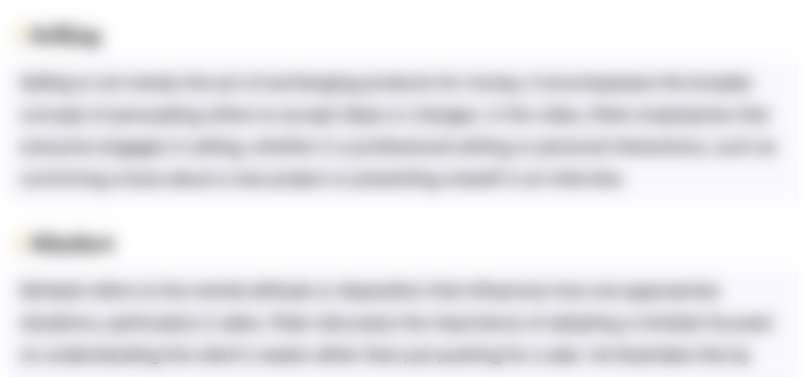
此内容仅限付费用户访问。 请升级后访问。
立即升级Highlights
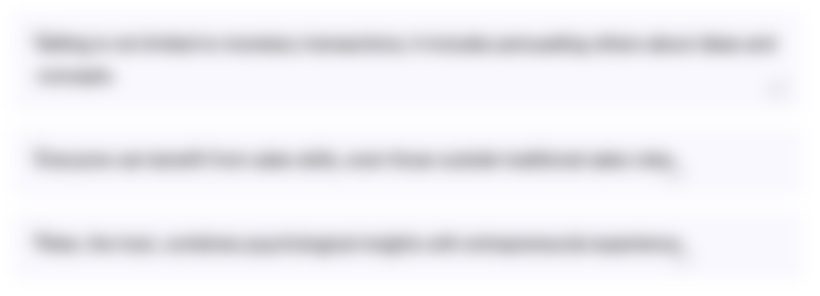
此内容仅限付费用户访问。 请升级后访问。
立即升级Transcripts
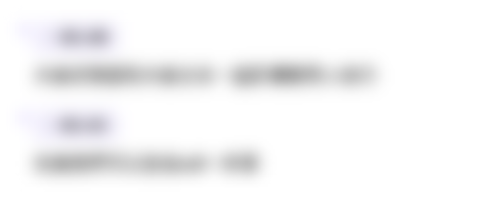
此内容仅限付费用户访问。 请升级后访问。
立即升级浏览更多相关视频
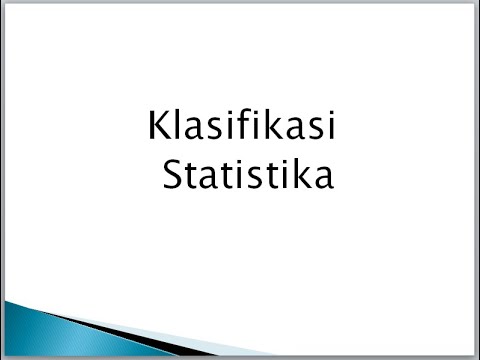
Statistik 2: pembagian statistika

KUPAS TUNTAS: Apakah Perbedaan Statistik Inferensial dengan Statistik Deskriptif ?

[PART 1] KONSEP DASAR STATISTIKA INFERENSIA
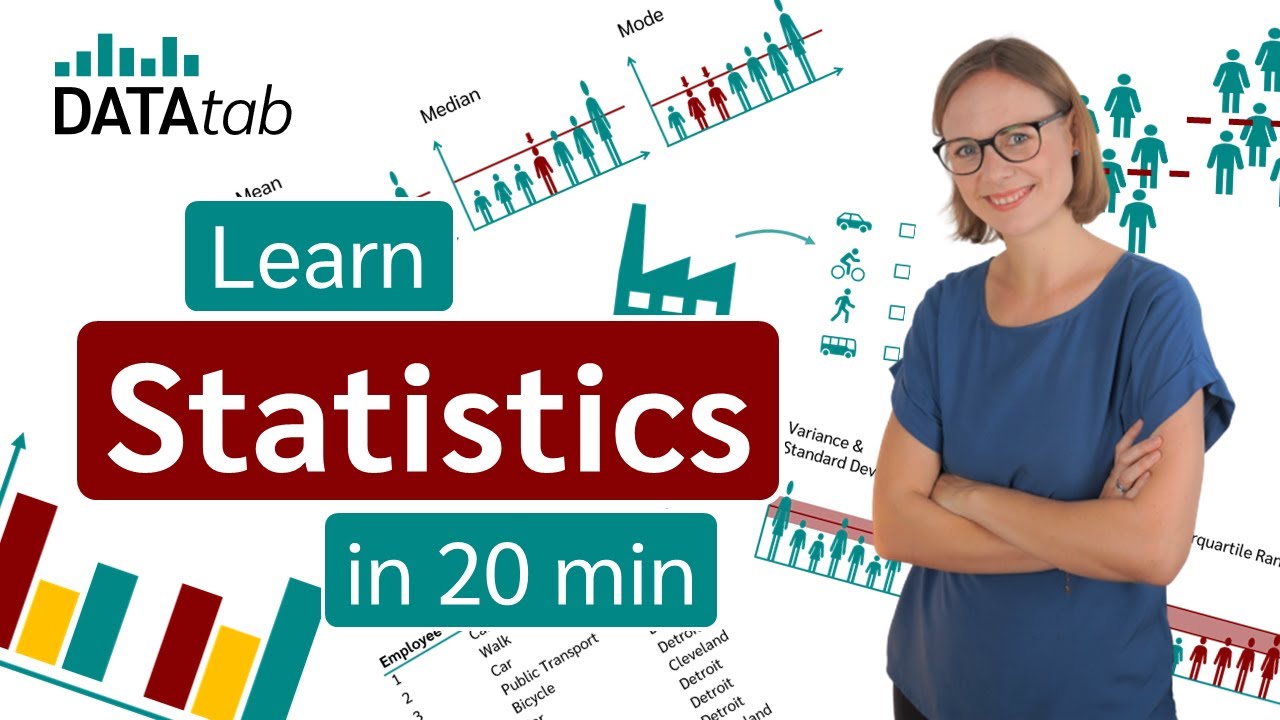
What is Statistics? A Beginner's Guide to Statistics (Data Analytics)!

video 5.1. inferential statistics logic
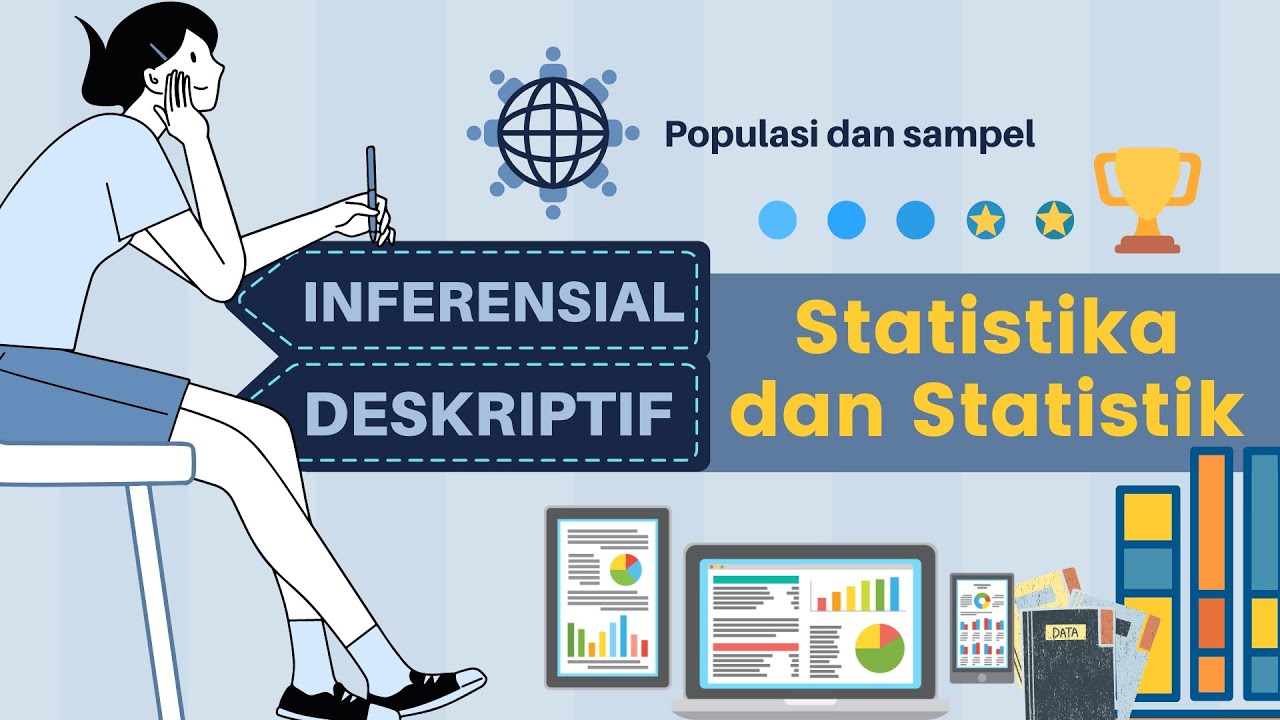
PERBEDAAN STATISTIK DESKRIPTIF DAN INFERENSIAL
5.0 / 5 (0 votes)