What is the difference between parametric and nonparametric hypothesis testing?
Summary
TLDRThis video explains the difference between parametric and non-parametric hypothesis testing, helping users understand when to use each test. Parametric tests, like the T-test or Pearson correlation, are used when data is normally distributed and offer more statistical power. Non-parametric tests, such as the Mann-Whitney U test or Spearman's correlation, are used when data does not meet these assumptions, relying on ranks rather than raw data. The video also highlights how online tools, like DataTab, can assist in choosing the right test and calculating results efficiently.
Takeaways
- 📊 To choose between parametric and non-parametric hypothesis testing, one must first check if the data is normally distributed.
- 🧮 If data is normally distributed, parametric tests like T-Test, ANOVA, or Pearson correlation are used.
- 🔍 For non-normally distributed data, non-parametric tests such as Mann-Whitney U Test or Spearman's correlation are appropriate.
- 📉 Non-parametric tests make fewer assumptions than parametric tests, which is why they are used when data does not meet the assumptions of parametric tests.
- 💪 Parametric tests are generally more powerful than non-parametric tests, meaning they are more likely to detect a difference if one exists.
- 🔢 The structural difference between parametric and non-parametric tests is that parametric tests use raw data while non-parametric tests use the ranks of the data.
- 📈 Spearman's rank correlation is a non-parametric counterpart to Pearson correlation and uses ranks instead of raw data.
- 🧐 The T-Test for independent samples checks for mean differences between groups, while the Mann-Whitney U Test checks for rank sum differences.
- 📋 When conducting hypothesis testing, the choice of test depends on the number of variables and whether the samples are independent or dependent.
- 🛠️ If the assumptions for parametric tests are not met, one can use the non-parametric counterpart, and tools like DataTab can assist in choosing the right test.
- 📈 DataTab is a tool that can calculate both parametric and non-parametric tests, and it helps users determine the appropriate test based on their data.
Q & A
What is the main difference between parametric and non-parametric hypothesis tests?
-The main difference lies in the assumptions about the data. Parametric tests assume that the data follows a normal distribution, while non-parametric tests do not require this assumption. Instead, they use rankings of the data.
When should parametric tests be used?
-Parametric tests should be used when the data is normally distributed and other test assumptions, such as homogeneity of variances, are met. They are more powerful, meaning they can detect differences with smaller samples or smaller differences between groups.
What are some examples of parametric tests?
-Examples of parametric tests include the T-test, Analysis of Variance (ANOVA), and Pearson correlation.
When should non-parametric tests be used?
-Non-parametric tests should be used when the data does not meet the assumptions of normality or when the sample size is too small to reliably test for normality.
What are some examples of non-parametric tests?
-Examples of non-parametric tests include the Mann-Whitney U test (non-parametric counterpart to the T-test), Spearman’s rank correlation (counterpart to Pearson correlation), and the Kruskal-Wallis test (counterpart to ANOVA).
Why are parametric tests considered more powerful than non-parametric tests?
-Parametric tests are more powerful because they can detect smaller differences between groups with smaller sample sizes. This is due to their stronger assumptions about the data distribution.
What is the structural difference between Pearson correlation and Spearman’s rank correlation?
-Pearson correlation uses raw data to measure the linear relationship between two variables, while Spearman’s rank correlation uses the ranks of the data instead of raw values, making it suitable for non-normally distributed data.
How is the Mann-Whitney U test different from the T-test for independent samples?
-The T-test checks whether the means of two groups are significantly different, while the Mann-Whitney U test checks whether there is a significant difference in the rank sums between two groups.
What does it mean to calculate a 'rank sum' in non-parametric tests?
-In non-parametric tests like the Mann-Whitney U test, rank sums are calculated by assigning a rank to each value in the dataset and then summing the ranks within each group. These rank sums are then compared to determine if there’s a significant difference between the groups.
How can users easily choose between parametric and non-parametric tests when analyzing data?
-Users can first check whether the assumptions for a parametric test (such as normality) are met. If these assumptions are violated, they can switch to a non-parametric counterpart. Tools like DataTab simplify this process by automatically suggesting the appropriate test based on the data and assumptions.
Outlines
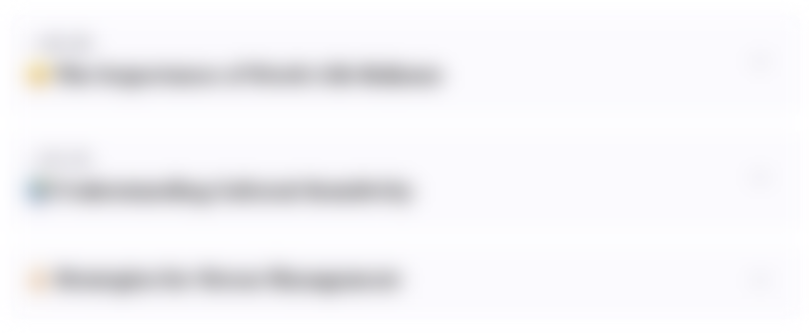
此内容仅限付费用户访问。 请升级后访问。
立即升级Mindmap
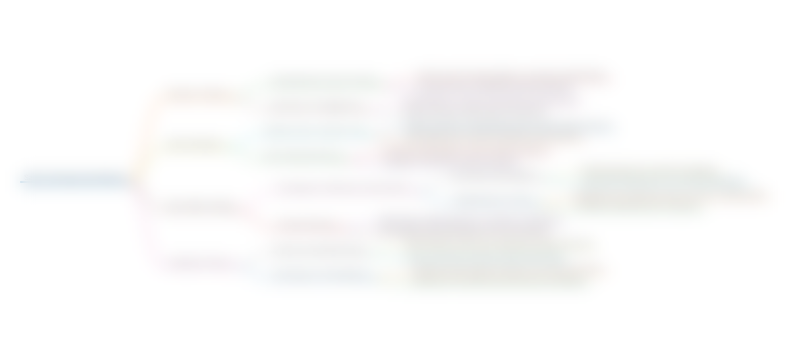
此内容仅限付费用户访问。 请升级后访问。
立即升级Keywords
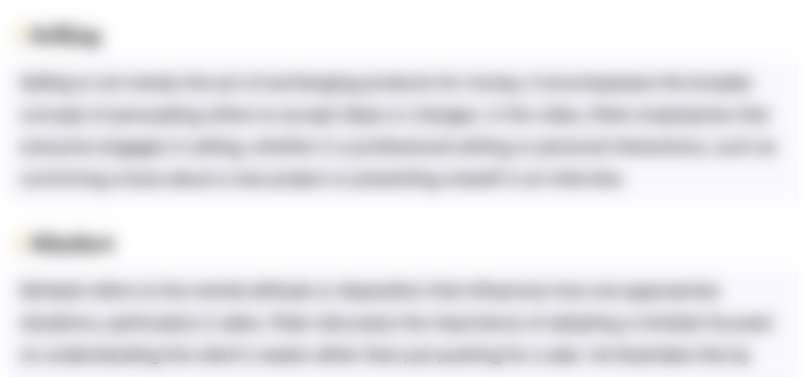
此内容仅限付费用户访问。 请升级后访问。
立即升级Highlights
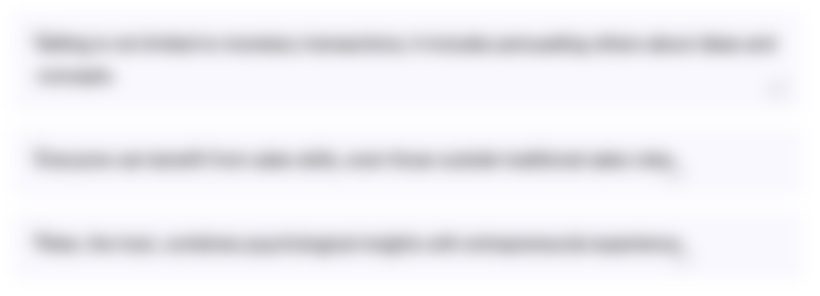
此内容仅限付费用户访问。 请升级后访问。
立即升级Transcripts
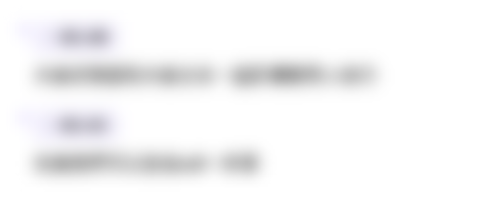
此内容仅限付费用户访问。 请升级后访问。
立即升级5.0 / 5 (0 votes)