Statistics Terminology and Definitions| Statistics Tutorial | MarinStatsLectures
Summary
TLDRThis educational video delves into the fundamentals of statistics, essential for research across various disciplines. It outlines the process of formulating a research question, translating it into a statistical test, and analyzing data to make inferences about a population. The video distinguishes between descriptive and inferential statistics, explaining concepts like estimation, hypothesis testing, and prediction. Key terminology such as units, variables, population, sample, parameters, and statistics are defined. The script also touches on external and internal validity, crucial for generalizing findings and ensuring study accuracy. It uses a hypothetical study on the link between exercise and depression among university students to illustrate these concepts.
Takeaways
- 📚 Statistics serves as the foundation for most research across various disciplines, involving the analysis of data to answer research questions.
- 📊 Descriptive statistics involve summarizing data through plots or numeric summaries like mean, median, and standard deviation.
- 🔍 Inferential statistics aim to make inferences about a population using a sample, which includes estimation, hypothesis testing, and prediction.
- 🧐 Estimation in statistics is about determining the average or mean of a population and expressing it with a confidence interval.
- ❓ Hypothesis testing involves posing questions that can be answered by comparing sample statistics to a population parameter.
- 🔮 Prediction uses statistical models to forecast outcomes for individuals within a population based on the sample data.
- 🔑 Key vocabulary includes 'unit' (subject), 'variable', 'population', 'sample', 'parameter', and 'statistic', which are crucial for understanding statistical concepts.
- 🌐 External validity assesses whether the findings from a sample can be generalized to a broader population.
- 🔄 Internal validity evaluates whether the sample estimate is accurate and free from bias or confounding factors.
- 📈 The script provides a concrete example of studying the relationship between exercise and depression risk among university students, illustrating the application of statistical concepts.
Q & A
What is the primary purpose of statistics in research?
-Statistics serves as the backbone of most research, helping to define a research question, translate it into a statistical statement, design studies, collect and summarize data, and generalize findings back to the population of interest.
What are the two main categories of statistics?
-Statistics can be divided into descriptive and inferential statistics. Descriptive statistics involve summarizing data using plots or numeric summaries, while inferential statistics attempt to infer information about a population from a sample.
What is the difference between estimation, hypothesis testing, and prediction in inferential statistics?
-Estimation in inferential statistics involves calculating an average or mean for a population and providing a confidence interval. Hypothesis testing involves questions that compare groups, such as whether taller CEOs earn more. Prediction uses a model to estimate an outcome for an individual, like a CEO's salary.
What is meant by a 'unit' or 'subject' in statistics?
-A 'unit' or 'subject' refers to the entities on which data is collected. These could be people, objects, or any other entities depending on the study.
How are variables defined in the context of statistics?
-Variables are recorded characteristics for the units or individuals in a study. They are typically organized in rows for individuals and in columns for different characteristics in a data table.
What is the population in a study, and why is it often difficult to study?
-The population is the group of interest for a study. It is often difficult to study an entire population due to its size, cost, time constraints, or because it may not be possible to access all members.
What is a sample and why is it used in research?
-A sample is a subset of the population used in a study. It is used because studying the entire population can be impractical, so a sample is taken to represent the population for the purpose of the study.
What is the difference between a parameter and a statistic?
-A parameter is a quantity or characteristic of the entire population, while a statistic is an estimate of that parameter derived from a sample. For example, the population mean is a parameter, and the sample mean is a statistic.
What does external validity refer to in a study?
-External validity refers to the ability to generalize the findings from a sample to an external population. It asks whether the estimates obtained from the sample can represent an external population.
What is internal validity and why is it important?
-Internal validity is the degree to which a study's findings are free from bias and can be trusted as an accurate representation of the sample. It is important to ensure that the study's results are reliable and not influenced by confounding factors.
Can you provide an example of how the concepts of population, sample, parameter, and statistic are applied in a real-world study?
-In a study investigating whether regular exercise decreases the risk of depression among university students, the population would be all university students, the sample could be 5000 students from a particular university, the parameter might be the true difference in depression rates between exercising and non-exercising students, and the statistic would be the observed difference in depression rates in the sample.
Outlines
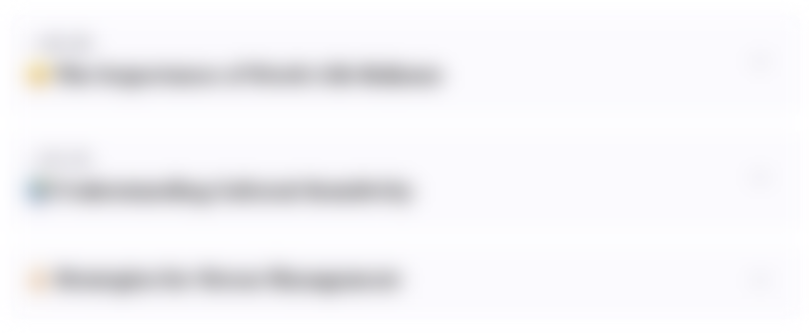
此内容仅限付费用户访问。 请升级后访问。
立即升级Mindmap
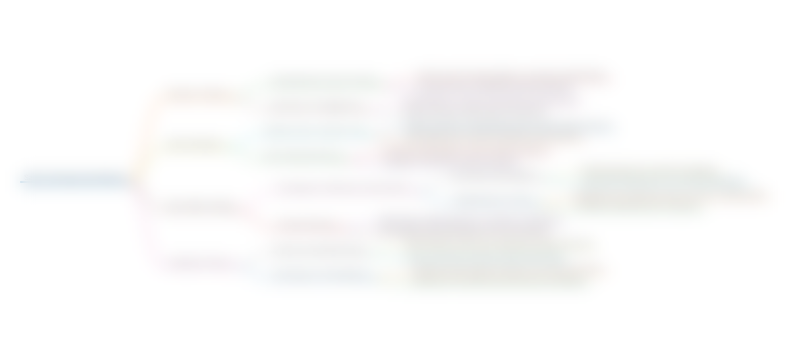
此内容仅限付费用户访问。 请升级后访问。
立即升级Keywords
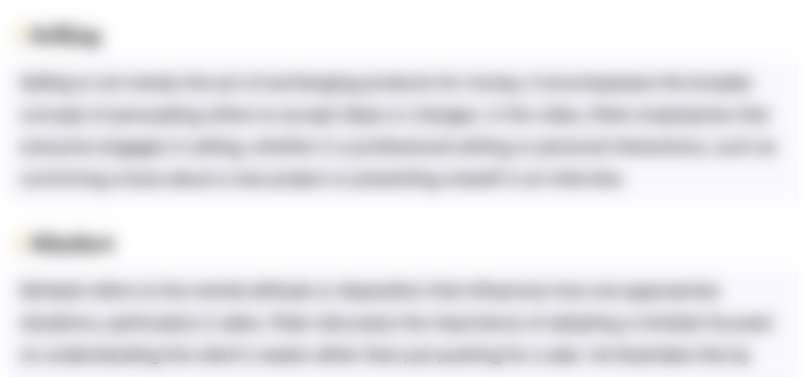
此内容仅限付费用户访问。 请升级后访问。
立即升级Highlights
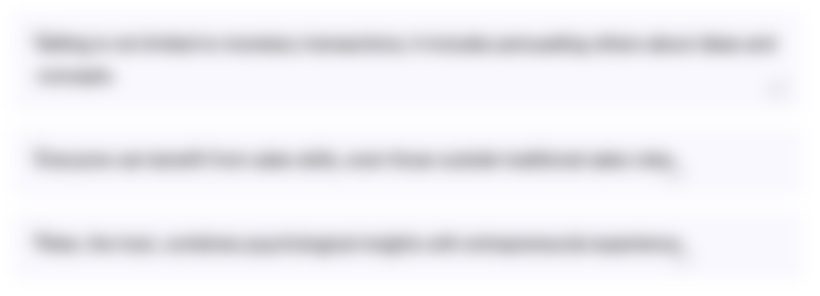
此内容仅限付费用户访问。 请升级后访问。
立即升级Transcripts
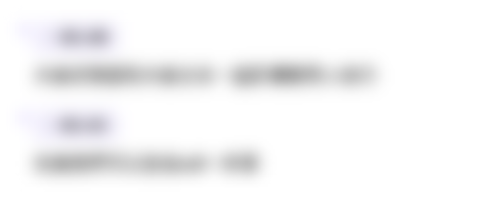
此内容仅限付费用户访问。 请升级后访问。
立即升级5.0 / 5 (0 votes)