RCM Revenue Cycle Management Medical AI LLM Case Studies
Summary
TLDRDavid Hood, CEO of 42 Robots AI, discusses the transformative impact of AI, particularly in revenue cycle management for healthcare. He outlines the benefits of implementing AI, warns of common pitfalls, and emphasizes the importance of understanding AI capabilities and avoiding a one-size-fits-all approach. Hood shares insights on leveraging large language models for data processing efficiency and stresses the need for a strategic AI implementation roadmap, including assembling the right team with a deep understanding of AI integration.
Takeaways
- 😀 David Hood, CEO of 42 Robots AI, discusses the benefits of implementing AI in revenue cycle management for healthcare organizations.
- 🔍 The video script emphasizes the importance of understanding the capabilities of Large Language Models (LLMs) and their potential in healthcare, especially in data processing and analysis.
- 🚀 Hood highlights a positive feedback loop created by AI implementation, where initial AI use leads to increased understanding and value, helping organizations outcompete their rivals.
- ⏳ He mentions that modern AI tools, particularly LLMs, have significantly advanced, with GPT-4 being a turning point in 2023, and that multimodal capabilities are even more recent.
- 💡 The script points out that while LLMs are broadly applicable, they should be used as tools by traditional software, not as the central solution, to avoid common pitfalls.
- 🛑 Hood warns against relying solely on solutions like Microsoft Co-Pilot, as they may not leverage the full potential of LLMs and could leave significant value on the table.
- 🔧 The transcript details case studies where AI has been used to automate complex and unstructured data processing in healthcare, significantly reducing time and costs.
- 📉 The video stresses that the goal of AI implementation is not 100% automation but rather to improve efficiency and productivity, with an 80-90% automation rate being a realistic and valuable target.
- 👥 It is important to assemble a team with a deep understanding of AI, including how it works, how to leverage it effectively, and how to integrate it into the organization's systems.
- 🛠 The transcript differentiates between AI engineers who understand the practical application of LLMs and machine learning engineers who focus on the technical aspects of AI models.
- 🔄 Hood suggests starting with small, achievable AI projects to gain traction and then iterating and building on those successes to develop a comprehensive AI implementation roadmap.
Q & A
What is the main focus of the video by David Hood?
-The video focuses on AI usage in revenue cycle management within the healthcare and medical space, highlighting the benefits and potential pitfalls of implementing AI.
Who is David Hood and what is his role in the video?
-David Hood is the CEO of 42 Robots AI, and he discusses the company's expertise in helping organizations implement AI, particularly in revenue cycle management.
What does David Hood suggest is the starting point for implementing AI in a healthcare organization?
-David Hood suggests understanding the capabilities of large language models (LLMs) and their benefits in revenue cycle management as a starting point for AI implementation.
What are the potential benefits of using AI in revenue cycle management according to the video?
-The video suggests that AI can significantly improve efficiency, speed, and productivity in processing and analyzing large amounts of data in healthcare organizations.
What does David Hood warn against in terms of AI implementation?
-He warns against relying solely on solutions like Microsoft Co-Pilot, which he says only utilize a fraction of the capabilities of large language models and may not fully leverage AI's potential.
What is the importance of not seeking 100% automation with AI, as mentioned in the video?
-The video emphasizes that pursuing 100% automation can be unrealistic and less efficient; instead, achieving 80-90% automation can often be more valuable and manageable.
What is the significance of the 'positive feedback loop' mentioned by David Hood?
-The positive feedback loop refers to the cycle of improvement that occurs as an organization begins to use AI, gains understanding, and builds momentum, ultimately outperforming competitors.
What are the key skills required for an effective AI implementation team according to the video?
-The video outlines three key skills: understanding how AI works, knowing how to leverage AI effectively, and having the ability to integrate AI efficiently into the organization's systems.
Why is having a Chief AI Officer important for an organization implementing AI, as suggested in the video?
-A Chief AI Officer is crucial for understanding the organization's systems, ensuring AI is integrated effectively, and for guiding the AI implementation strategy to avoid common pitfalls.
What is the role of an AI engineer in the context of the video's discussion on AI implementation?
-An AI engineer is responsible for calling the LLM APIs, solving real-world problems, and ensuring that AI solutions are not LLM-centric but rather use LLMs as tools within a broader software framework.
What advice does David Hood give for building an AI implementation roadmap for a revenue cycle management company?
-He suggests starting with assembling a team with the right skills, understanding the basics of AI, leveraging AI effectively, and integrating AI into the business systems, while also considering hiring fractional Chief AI officer services for guidance.
Outlines
🤖 Introduction to AI in Revenue Cycle Management
David Hood, CEO of 42 Robots AI, introduces the video's focus on AI usage in revenue cycle management within the healthcare and medical sectors. He emphasizes the significant benefits of implementing AI, particularly in data processing and analysis, which can greatly enhance efficiency and productivity. David also mentions the existence of several supplementary videos on topics like AI solution architecture and new capabilities of large language models (LLMs). He cautions about the potential pitfalls in AI implementation and suggests that there's a positive feedback loop in adopting AI, where initial successes lead to further integration and competitive advantage. The video aims to provide viewers with a basic understanding of AI's potential and a starting point for implementation.
📈 Leveraging AI for Data Processing and Analysis
The second paragraph delves into the transformative impact of AI, specifically large language models, on data processing and analysis within the medical and healthcare sectors. It highlights the underleveraged data and the costly manual labor often required for data processing. AI, and particularly modern LLMs, can drastically improve these processes by increasing speed, efficiency, and productivity. The speaker shares an example of a client case study where AI was used to process complex and unstructured data, reducing the time from months to a week for initial drafts. The video stresses that the solution should not be LLM-centric but rather use LLMs as tools within a broader software architecture. It also touches on the importance of having AI engineers who understand how to build and leverage LLMs effectively.
📑 Case Studies in AI Implementation for Revenue Cycle Management
Paragraph three presents further case studies illustrating the application of AI in revenue cycle management. It discusses the challenges of processing handwritten, unstructured, and variant healthcare faxes and how AI can be used to automate a significant portion of this manual work. The speaker clarifies that the goal is not 100% automation but rather achieving a substantial improvement in efficiency. They explain that attempting to automate everything can lead to increased complexity and diminishing returns, suggesting that focusing on 80-90% automation is more practical and valuable. The paragraph also emphasizes that the solution should be built around software that uses LLMs as tools, rather than the other way around, to avoid common pitfalls in AI implementation.
🛠 Building an AI Implementation Roadmap for Revenue Cycle Management
The final paragraph outlines the steps for building an AI implementation roadmap within a revenue cycle management company. It identifies the need for a team with three key skill sets: understanding how AI works, knowing how to leverage AI effectively, and having a systems understanding of how AI integrates into business processes. The speaker distinguishes between AI engineers who build AI tools and those who know how to use them effectively. They suggest starting with a fractional Chief AI officer to guide the hiring process and ensure the right AI talent is brought on board. The paragraph concludes by encouraging viewers to contact 42 Robots AI for assistance in developing a tailored AI implementation strategy, emphasizing the importance of acting quickly to gain a competitive edge.
Mindmap
Keywords
💡AI
💡Revenue Cycle Management
💡Large Language Models (LLMs)
💡AI Implementation Roadmap
💡Chief AI Officer
💡Automation
💡Data Processing
💡AI Engineering
💡Microsoft Co-pilot
💡Custom AI Solutions
💡Fractional Chief AI Officer
Highlights
David Hood, CEO of 42 Robots AI, discusses AI implementation in revenue cycle management for healthcare organizations.
Emphasizes the significant benefits of AI in medical and healthcare organizations, particularly in revenue cycle management.
The importance of understanding the capabilities of Large Language Models (LLMs) and their potential in business.
Warns of potential pitfalls and 'landmines' in AI implementation and how to avoid them for value addition.
Describes a positive feedback loop created by AI, where initial use builds organizational 'muscle' and understanding.
Advises on the competitive advantage gained by early and aggressive AI implementation in business processes.
The transformative impact of modern AI capabilities, especially those emerging within the last year.
Caution against relying solely on Microsoft Co-Pilot or similar tools, as they may not leverage the full potential of LLMs.
Details the enhanced data processing capabilities of AI, particularly in unstructured or variant data scenarios.
Case study: Accelerating healthcare outcome and process metrics processing from months to weeks using AI.
The inefficiency of manual data processing and how AI can offer a 100x to 10,000x improvement in productivity.
Differentiating between AI Engineers who understand LLMs and those who effectively leverage them in practical applications.
The misconception of aiming for 100% automation with LLMs and the practicality of achieving 80-90% efficiency.
Highlighting the importance of building an AI implementation roadmap for a revenue cycle management company.
The necessity of assembling a team with the right skills to understand, leverage, and integrate AI within an organization.
The role of a Chief AI Officer in understanding the organization's systems and the impact of AI integration.
Encourages contacting 42 Robots AI for assistance in building an AI implementation roadmap and gaining a competitive edge.
Transcripts
hi this video is about AI usage case
studies with revenue cycle management
companies in the me in the healthcare
medical space my name is David hood and
I'm CEO of 42 robots Ai and we help
organizations Implement AI especially
revenue cycle management that's where we
have a lot of experience in the medical
space so there's links to several videos
that I'll put below that are all
relevant to and and supplement this
content here we got the chief AI officer
video bad AI solution architecture and
custom AI solution and llm new
capabilities recommend you check those
out uh before after you watch this video
so first of all there are real
significant benefits today uh to
implementing AI at any medical or
Healthcare organization especially
revenue cycle management um and I'll
explain to you that in a second based
upon the capabilities of llms some of
which don't really get talked about that
that much uh but there's definitely some
landmines to navigating this that you
want to keep in mind and I'll sprinkle
those throughout here uh and that should
help you avoid some of the biggest
pitfalls and move forward with things
that add the most value I'd say that
there's a positive feedback loop here
because you know you can't expect to be
like all right 3 months from now we're
going to use AI in all the places it's
going to be perfect in everything and
and I don't think anybody necessarily
reasonably expects that uh but as you
start to use AI with an organization as
an organization you start build some of
that muscle you'll get some
understanding you'll get some political
biy in and it starts to create a
positive feedback loop to add more and
more value to the organization which
helps you beat out your competitors uh a
lot of the latest capabilities some of
which are weren't even available until
within the last year um are uh not being
used by most companies uh at the at the
same time there's a lot of companies
that understand that this needs to be
implemented and if you're competitors do
some of the stuff we're going to talk
about here uh in this video before you
do it then they will leave you in the
dust and the other the opposite is true
if you get to this sooner and faster and
you implement some of these AI Solutions
very aggressively then uh you'll start
to to uh get ahead of them and it'll be
hard for them to even see what you're
doing so part of the objectives of this
video is to help you gain understanding
of what's possible and and at least a
basic starting point for how how to get
going on uh implementing AI at your
revenue cycle C uh management company
um put you in a better position to do
that and also to even give you just like
the really basic seed for building a
road map so let's talk about kind of the
new capabilities of that that come out
that have come out recently um because
of the modern large language model so
first of all I'd say the clock really
really doesn't start
for the modern tools until gp4 came out
in I think believe it it's April of
2023 um so you know some people might
say I've been an AI engineer for 10
years well that doesn't really mean a
whole lot because uh prior to GPT 4 or
if you really want gpt3 um there wasn't
a lot uh just the tools were different
it's it's like saying um uh I used to I
I've driven race cars therefore I'm an
expert at flying planes it's it's about
that much of a difference um well
there's certainly some overlap in skills
it's it's very different additionally
multimodal is even more recent um in
terms terms of uh the large language
model is actually being able to look at
images and um understand them and this
is useful in some use cases the the app
the capabilities um are super broad for
large language models um it the modern
llms are so broadly applicable that just
about every business and and about every
business process and just about every
business in the world can gain value
from large language models caution
though Microsoft co-pilot or chat GPT
Enterprise or whatever if that's your
solution I I've heard a lot of people
say oh our solution is we got Microsoft
co-pilot we're doing Ai No you are
leaving 99 plus% of the capabilities on
the table if you're doing that it is not
a solution for uh for actually
leveraging LMS I mean it is a solution
it gets some of the value but it does it
doesn't get the majority of the value um
but one of the key things that comes out
of this is that uh data processing has
been super leveled up uh good AI
Engineers who have experience processing
large amounts of unstructured or widely
Andor widely variant data can do a whole
lot with data to speed up and um make it
less expensive and and make people more
productive in the in the spaces where
there's lots of data that needs to be
processed which is huge in revenue cycle
management and Medical in
general so let's go a little bit deeper
into that so really what you have is you
have two major areas uh that are kind of
two sides of the same coin where there's
tons of data that is either
underleveraged
um which means that basically you have
this valuable data that you could do
stuff with that you're not or it's very
expensively leveraged oftentimes this
means you have very expensive manual
labor processing the data um and so in
both of these cases AI specifically
large language models the modern
Premiere you know Frontier models can
dramatically improve these processes and
some cases I I think especially when it
comes to like data processing and and
data analysis and data processing means
a bunch of different things you can get
a 100x 10,000x the value the the
productivity the efficiency the speed
that you could get from a human uh let's
say even a very experienced data person
this is not a knock on humans this is
just something that that a large
language models are really really good
at especially when you have an AI
engineer that knows how to build these
correctly again if you're using like
Microsoft co-pilot there's a little bit
of a value that you can get from this
but you're missing most of it which
brings me to real AI engineering I think
there's a lot of hand waving going on
with regards to AI engineering there's a
lot of people saying that they're AI
Engineers when they have no real world
experience or um or they're they don't
really have practical experience maybe
they think they're doing AI engineering
but they're building with like a bad AI
architecture again check out that video
or they're they've done a lot of hand
waving and they just put AI engineering
on there we can help kind of filter
through that nonsense and I I'll talk
about that uh in in a minute so let's
get into some of the the case studies a
little bit of details as to what we were
doing so for one client we did
Healthcare outcome and process metrics
so this is a revenue cycle management
SAS company the data was very large
comple complex and unstructured and even
hard for humans so some of the data that
they gave us that was supposed to be
good we figured out oh actually the
humans did some of it wrong too so it
was not easy to process um it would come
out this data would come out on a yearly
basis and their clients customers needed
it needed them to process it to put it
into their SASS and it usually took them
months and sometimes there's like a few
Key Resources a few key people that if
they were out it could take an
additional few months mean these are
very skilled humans these are highly
paid full-time salary people with lots
of experience in this specific stuff so
they're very expensive um and basically
with our solution we're able to make
that this can be months faster instead
of it taking 2 3 4 months it can take
about a week for them to get like a
first version draft of these to where
it's basically about 80% automated uh
maybe as much as 90% but 80% is kind of
the minimum bar and um this dramatically
improves things for them because they
get the the drafts it's highly accurate
for most of the different types of uh
inputs that they have there are a few
that it that some some of the more
complex ones uh more longtail ones that
it doesn't do well but that's fine
you're not really looking to fully
automate and I'll talk about that in a
second with the second case study why
this is actually good you wanting to
fully automate 100% automate things with
with LMS is not really a reasonable goal
in most
scenarios and I want to point out here
this is super critical the solution is
not llm Centric and that might sound
weird it's like well but you're using
llms they're they're key to the solution
yes um but the the solution itself does
not put the llm at the center the model
is not the solution the solution is not
the model and this is part of again if
you watch the bad architecture or the
how to build custom AI Solutions you
you'll understand this on a deeper level
but long story short the llms need to be
used as tools by classic code uh just
basically software versus the other way
around where uh most people are building
things LM Centric they have like a model
and then the model basically does
everything it it picks things it uses
tools they train everything everything
tries to get shoved in that model
basically and this is going to give you
a lot of trouble and you're going to
have you're going to really struggle to
solve more complex problems um
especially on a very customized basis
and and to automate to a very high
degree custom if you're liking this
video please like if you have any
questions or thoughts or your own
experiences please feel free to leave a
comment below as well um this is this is
a case study where the the inputs are
handwritten unstructured and variant
healthc care faxes um again another
revenue cycle management SAS company you
know sometimes the the writing was
unclear or even if it's not handwritten
sometimes like the the typing is like a
skew or um the structure of the document
is really different to where sometimes
they put this over here sometimes they
put it over here and it really there was
not a whole lot of consisten these are
coming from a wide variety of different
sources um and then not only is the
formatting and the structure
inconsistent but the the the way that
the input is put in there can be
different so for example a simple
version of this would be the date
there's a bunch of different ways to
write a date and if you're trying to
write like a script for that there's a
bunch of different variants that's a
much more simple version of it but a
more complex one might be like a
diagnosis there's a bunch of different
ways to write a diagnosis there's a lot
more variance in the outputs in and in
terms of how that data is labeled um but
also what the what the the actual field
or or category or or content of that
field looks like um I I want to point
out again the objective here is not 100%
automation so this is stuff that is
getting 100% manually processed so
imagine if we could put it through a
system in it only 50% of them are
automated that's still a huge
Improvement on this because it means
that for 50% of the documents you don't
need the manual inputs also just a
bigger Point here and again I I think I
covered this pretty detail in the
customing solutions video where you
shouldn't be looking for 100% automation
Solutions in most scenarios the reason
for this is that
going back to llms are so broadly
applicable that uh on top of the fact
that once you get to like 80 90% there
becomes a point where every percentage
Point becomes exponentially harder so
going from 90% to 95% is probably harder
or more challenging than going from like
uh 0 to 50% or 0 to 80% or something
like that and then going from 95% to 97%
etc etc etc and um um part of this has
to do with especially if you're doing
llm Centric this is almost impossible in
a lot of scenarios but even with a
really good structure to the solution um
as you build try to build more and more
of the edge cases and more and more of
the challenging things it can actually
degrade some of the more basic
fundamental things that you do and so
you have to build much many more
exceptions the complexity of the
solution grows and it and as the
complexity grows the the the overall it
just becomes exponentially harder with
software and this is not true for every
case but in general this makes sense so
another way to put this is in terms of
from an efficiency standpoint it's
better in the same amount of time you
could probably get 10 80 to 90%
Solutions than you could get one like
99% solution okay in terms of automation
so which one has more value and almost
always the first one now there might be
some scenarios where the 99% to Plus
Solution does add more value it's like a
key process and automating it just is
just huge for the company maybe that's
the case where it is worth it to try to
go for that really high level thing but
I think that's the exception and not the
rule at least in with the clients that
we've talk
to so I'll also briefly put in here a
just a general idea on how to build your
AI implementation roadmap as a revenue
cycle management company and then if you
really want us to help you with this
because there's obviously more nuance
and I can give in this quick video
please contact us you can give us a call
or click the link below and we'll help
you do this U and give you a lot more a
lot better of of ani implementation road
map so first you need to assemble the
team and there's three main skills that
you need how AI Works how AI can be
leveraged and how to integrate AI
efficiently into your organization okay
so let's cover each one of these
individually so how AI works most people
think that this is um an a machine
learning engineer and that is true but
you don't have to be a machine learning
engineer to get the fundamentals of how
AI Works down um a good AI engineer has
this and so this is isn't necessarily
understanding all the ins and outs of
how a large language model works at a
very computer science fundamental level
it's more about like understanding um
the the the basics and and the key the
some a handful of the key ideas so for
example understanding how large language
models turn the semantic meaning of
words into math that's a key
understanding and so somebody who has
some math experience with like um linear
algebra and linear programming and stuff
like that can be effective in
understanding this so some amount of
math understanding but at our company
some of our AI Engineers have machine
learning official machine learning
experience and some of them don't and
some of the ones that some of our best
ones don't have that experience but they
understand that it's next token
prediction they understand that there's
Randomness in there and some of the key
ideas there and then a lot of people
think conflate one and two they think oh
it's the same thing how does the LM work
a machine learning engineer understands
how to leverage llms effectively nope
um and let's
actually let's call these llms how llms
work and then how llms can be
effectively leveraged this is you know
is a little bit of a rough um metaphor
but it works generally there are two
different skill sets between the person
who drives the car and the person who
builds the car and that you can maybe
have somebody who understands both
really well um but it's two different
skills so think of the llms as a tool
and the person who knows how to use
these tools versus the person who knows
how to build these tools to to be really
good two different skill sets
unfortunately these get conflated a lot
and so pretty much all the time
resources and money in in the AI
industry are going towards item one here
and they're thinking that's all that you
really need and I'm telling you it's not
for sure definitely 100% uh that item
two here could actually end up being
more important especially if llms become
commoditized um and it's also definitely
where there's a big amount of
underserved population here
so uh this is where an AI engineer
really should Shine
versus a um I'm sorry yeah an AI
engineer versus a machine learning
engineering these are two different
skill sets um they need to have
experience actually calling the llm apis
actually solving real world problems
probably with python um there's some
other languages but usually it's with
python to solve problems in a way that
is not LM Centric okay and then this
third item this one's a little bit
harder and more challenging and some
people might have part of this this is
more of a systems understanding both
broadly how are these llms going to
affect your business systems as a whole
but also an understanding of your
specific Business Systems and this is
where uh this third item is where a
chief AI officer comes into play a chief
AI officer really should have one and
two pretty well maybe they're a previous
AI engineer they've done AI engineering
work and then some amount of especially
like systems thinking is really helpful
or you know if if they don't understand
your your organization they come in and
that's part of their job is to really
understand the organization how if I'm
going to be doing stuff over here how
does it affect this other part of the
business and vice
versa um so this is a really important
position Chief a officer if you don't
believe me watch the video on Chief a
officer I'll put a link to that below um
it is super important for all sorts of
reasons and you could you also want to
if you need to get this up and running
really quickly consider Hiring Our
fractional Chief AI officer services
this will really help you get AI
Engineers hired because you don't want
to just go out and start hiring ai ai
Engineers because I bet if you don't
have a CH Chief AI officer who if you
have nobody in there who actually has AI
engineering experience how will you know
how to tell the difference between the
posers and the real PE the real deal you
won't and these people are very
expensive because there's not very many
AI Engineers there's not very many
people who have these one two three
skills we do at our organization
generally have these in in a variety of
different different capacities so once
you have a chief AI officer or a
fractional one you hire an AI engineer
or you Outsource which we can also
provide and then you go you try and you
try and you try and because the first
few times maybe it won't work or you try
for something with super low hang fruit
to just get a win even if it's um it's a
quick win to get some Buy in um and then
you iterate from there um and you start
building the organizational muscle okay
give us a call if that if you're still
with me give give us a call click the
link below and I I I we can we can
definitely help you navigate through
this and get get this going as soon as
possible so you can start getting value
from llms at your organization before
your competitors do thank you very much
have a great day bye
浏览更多相关视频

The State of Data & AI - Trevor Jones
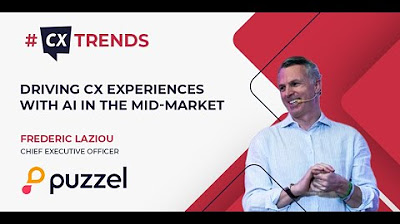
AI in the Mid-Market: Driving Better Customer Service Experiences - #CXTrends24
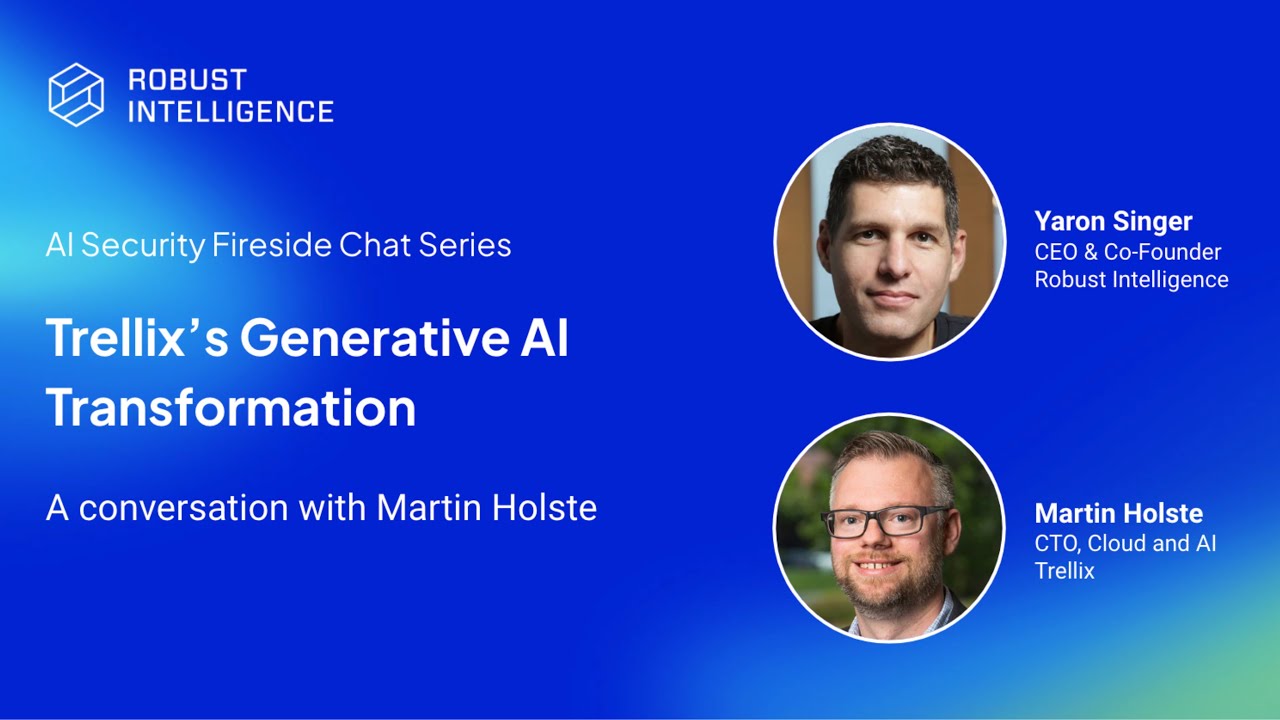
AI Security Fireside Series: Trellix's Generative AI Transformation
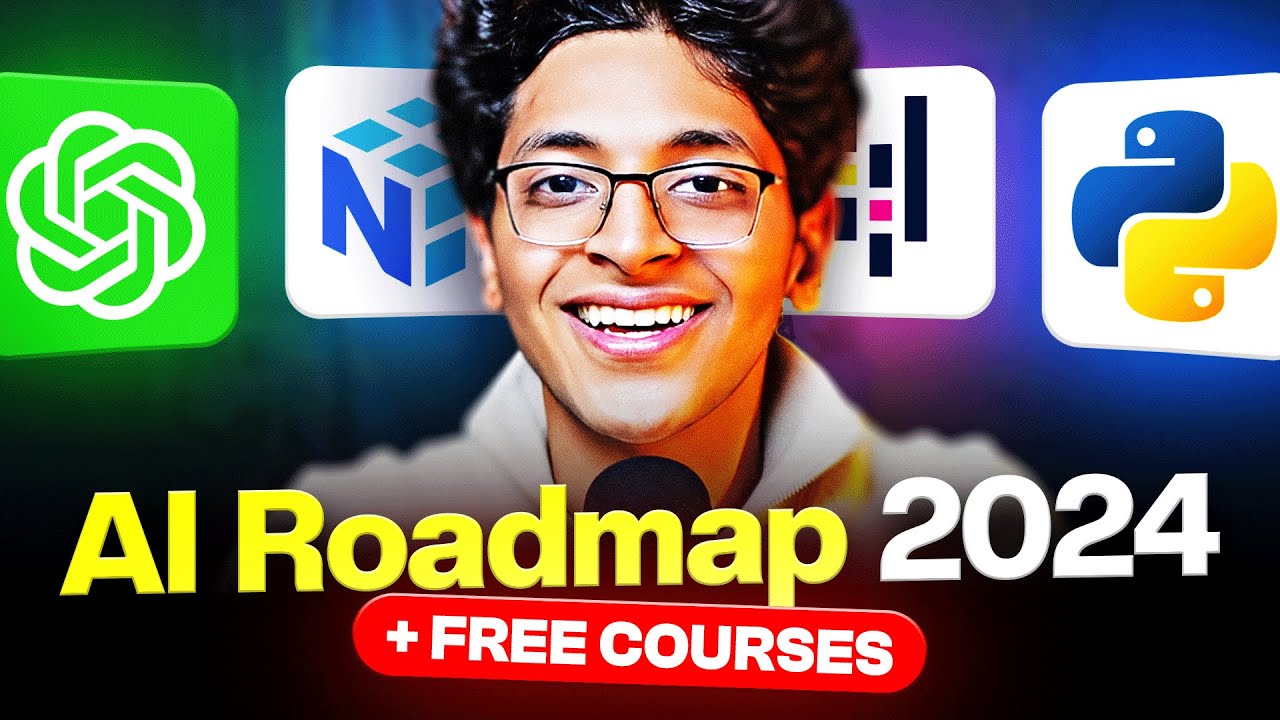
How I'd Learn AI in 2024 (If I Could Start Over) | Machine Learning Roadmap
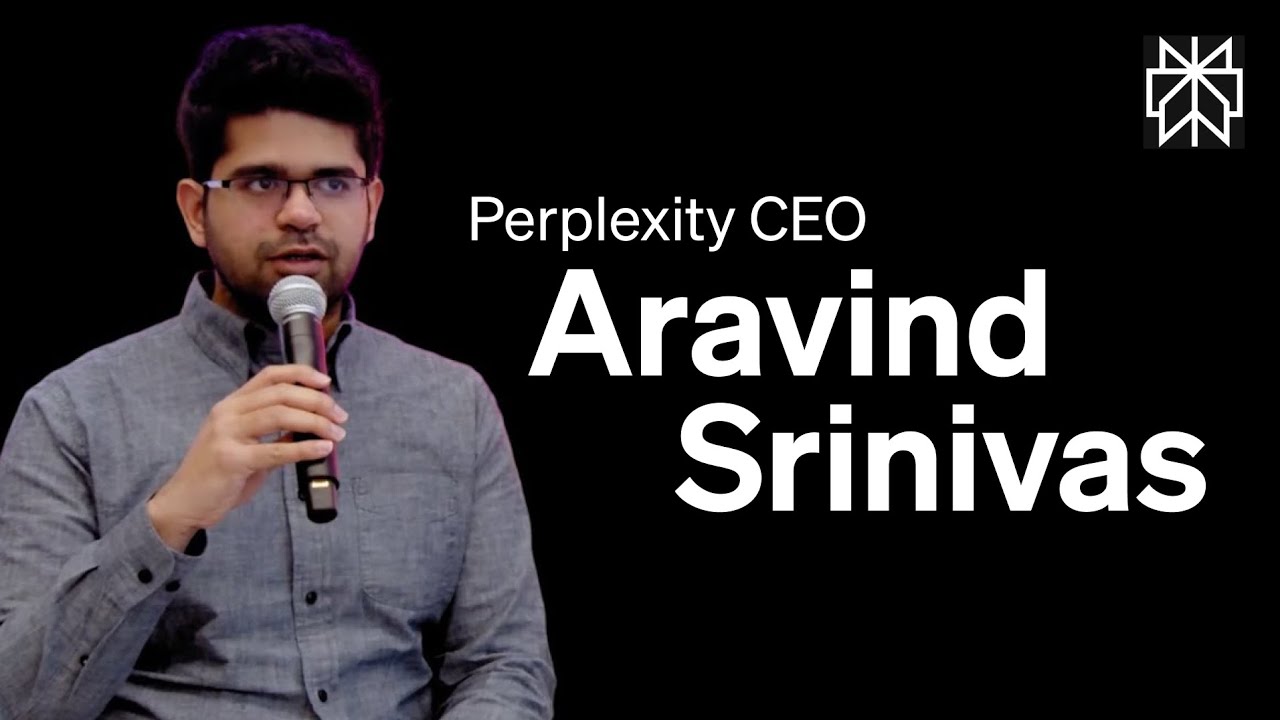
Aravind Srinivas (Perplexity) and David Singleton (Stripe) fireside chat
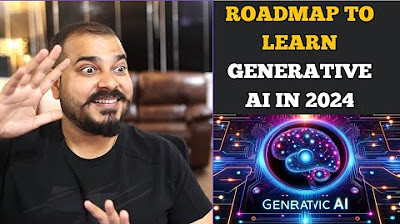
Roadmap to Learn Generative AI(LLM's) In 2024 With Free Videos And Materials- Krish Naik
5.0 / 5 (0 votes)