Bias in AI and How to Fix It | Runway
Summary
TLDRこのビデオスクリプトは、AIモデルが持つステレオタイプの偏見と、それを是正するための取り組みに焦点を当てています。Runwayのスタッフ研究科学者であるDTが率いる研究努力を通じて、生成画像モデルにおけるステレオタイプ的偏見を理解し、修正することが議論されます。特に、データとアルゴリズムの両方のアプローチが探求され、データに焦点を当てた「多様性ファインチューニング」が紹介されます。この方法は、偏見を減少させ、AI技術の公平で公正な使用を保証するために不可欠です。結果として、より安全で、世界をより正確に反映したテキストから画像へのモデルが提供されます。
Takeaways
- 😀バイアスは、特定の事柄に対して無意識のうちに持つ偏見や先入観を意味する。
- 🤖AIモデルも人間と同様に、ステレオタイプな表現に偏る傾向があることが問題とされている。
- 🔍バイアスの修正には、アルゴリズムとデータの二つのアプローチがあるが、ここではデータに焦点を当てる。
- 📊モデルは膨大なデータに基づいて学習するため、人間の偏見がデータを通じて反映されやすい。
- 🛠️AIモデルのバイアスを認識し改善するプロセスは、AI技術の公平で公正な使用を確保するために重要である。
- 👥モデルのデフォルト出力は、若く魅力的な女性や鋭い顎線を持つ男性など、特定の美の形を偏向している。
- 💼権力のある職業(CEOや医師など)は、より軽い肌の色と男性に偏る傾向がある。
- 🌍これらの偏見は、世界の現状を正確に反映していない。
- 📈多様性ファインチューニング(DFT)は、バイアスを修正し、テキストから画像へのモデルをより安全で世界を代表するものにする効果的な方法として提案されている。
- 🎨DFTは、特定の結果を表すデータのサブセットにより重点を置くことで、モデルに多様性を学習させる。
- 👩🔬研究チームは、170の異なる職業と57の民族性を用いて、約990,000枚の合成画像を生成し、モデルを多様性ファインチューニングするための豊かで多様なデータセットを作成した。
- 🌟多様性ファインチューニングは、モデルがより包括的になることを目指す上で、すでに効果を示している。
Q & A
バイアスとは何ですか?
-バイアスとは、特定のものに対して無意識のうちに持つ、一定の方向への見方や感じ方の傾向です。人間の脳に組み込まれており、世界をより効率的にナビゲートするのに役立ちますが、ステレオタイプにつながる問題もあります。
バイアスがAIモデルにどのような影響を与えますか?
-AIモデルは、ステレオタイプな表現にデフォルトする傾向があり、これによって社会的なバイアスが増幅される可能性があります。例えば、権力のある職業は明るい肌色の男性に、低収入の職業は暗い肌色の女性にデフォルトするなどです。
バイアスを修正するためのアプローチにはどのようなものがありますか?
-バイアスを修正する主な方法は、アルゴリズムとデータの2つです。このスクリプトでは、データに焦点を当てています。
データに基づくバイアス修正の方法は何ですか?
-データに基づくバイアス修正は、特定のデータサブセットにより多くの重点を置くことで、望む結果を代表するモデルを微調整することによって行われます。
ダイバーシティ・ファインチューニング(DFT)とは何ですか?
-DFTは、バイアスを修正するために、特定の結果を代表するデータサブセットに焦点を当ててモデルを微調整するプロセスです。これは、テキストから画像へのモデルがより安全で、現実の世界をより代表するようにするための効果的な方法として証明されています。
DFTの実装において重要なステップは何ですか?
-DFTの実装においては、特定の職業や民族性を持つ女性医師など、多様なプロンプトを使用して合成画像を生成し、豊かで多様なデータセットを作成することが重要です。
DFTによって生成された画像の数はどれくらいですか?
-DFTプロジェクトでは、170の異なる職業と57の民族性を使用して、約990,000枚の合成画像が生成されました。
AIモデルのバイアスを修正することの重要性は何ですか?
-AIモデルのバイアスを修正することは、AI技術の公平で平等な使用を確保し、既存の社会的バイアスを増幅させないようにするために重要です。
DFTがテキストから画像へのモデルに与える影響は何ですか?
-DFTは、テキストから画像へのモデルをより安全で、実世界をより正確に反映するものにする効果的な方法です。
将来的にAIモデルが目指すべき状態は何ですか?
-将来的には、AIモデルがより包括的で、多様性が豊かな表現を生成し、現実世界の真の代表となるような状態を目指すべきです。
Outlines
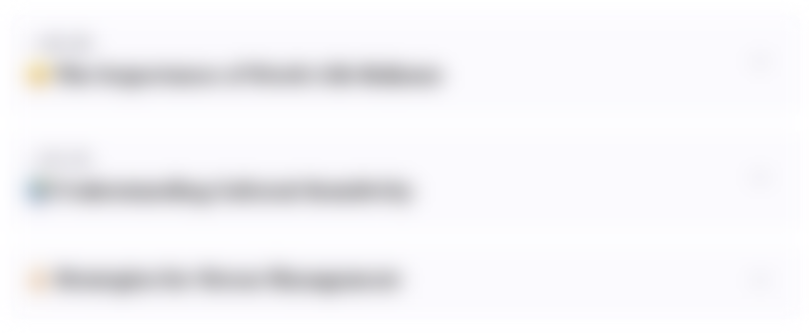
This section is available to paid users only. Please upgrade to access this part.
Upgrade NowMindmap
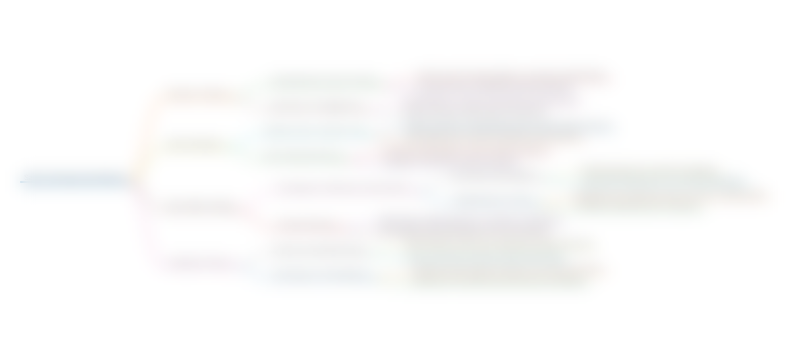
This section is available to paid users only. Please upgrade to access this part.
Upgrade NowKeywords
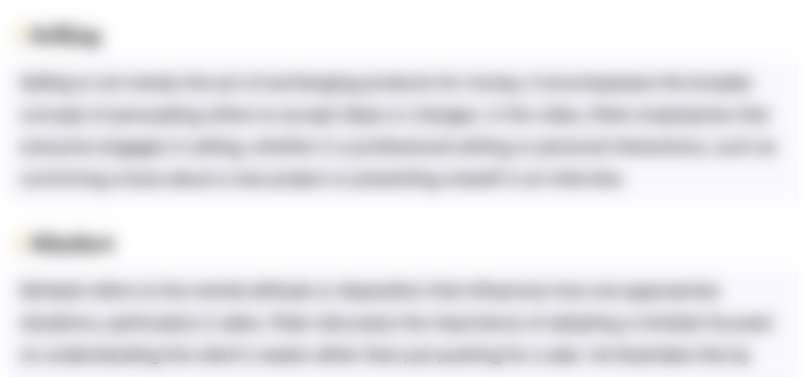
This section is available to paid users only. Please upgrade to access this part.
Upgrade NowHighlights
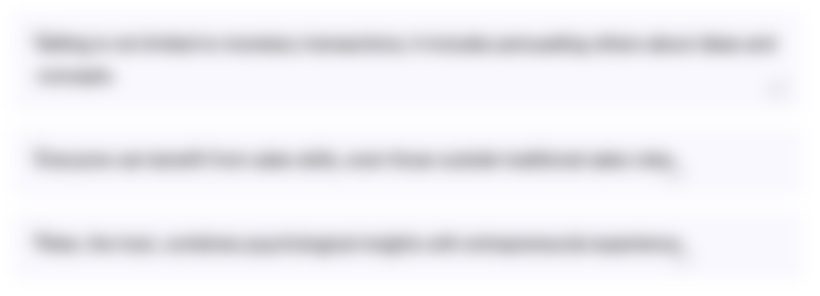
This section is available to paid users only. Please upgrade to access this part.
Upgrade NowTranscripts
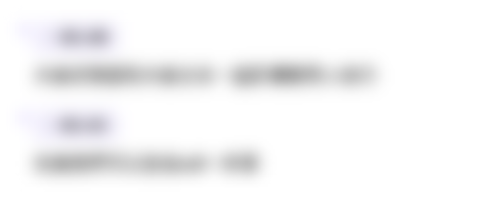
This section is available to paid users only. Please upgrade to access this part.
Upgrade Now5.0 / 5 (0 votes)