The future of artificial intelligence in radiology: Prof. Dr. med. Mathias Goyen
Summary
TLDRThe speaker, a radiologist and professor, discusses the transformative impact of artificial intelligence (AI) in radiology. With the exponential growth of medical data, AI is essential for managing information overload and reducing medical errors. The talk highlights AI's role in personalized medicine, improving diagnostic accuracy, and enhancing patient care. The speaker advocates for embracing AI as a tool to augment radiologists' capabilities, not replace them, emphasizing the importance of a technology quotient (TQ) for future professionals.
Takeaways
- 📈 Rapid Data Growth: The healthcare industry is generating an enormous amount of data, doubling in a mere 73 days, highlighting the exponential growth of medical information.
- 📚 Overwhelming Information: With 5,600 medical journals producing 800,000 articles annually, the volume of medical literature is vast, and radiologists face significant challenges in keeping up with it.
- 🔍 Radiologist's Challenge: Radiologists are tasked with analyzing tens of thousands of images in a single shift, a task that has become increasingly demanding with the rise in data volume.
- 🚑 Medical Errors: Medical errors are alarmingly common, with an estimated 350,000 patients dying annually in Europe due to hospital-related errors, emphasizing the need for improved accuracy in diagnoses.
- 👩⚕️ Healthcare Worker Shortage: The global shortage of healthcare workers is a pressing issue, with a projected 14 million missing workers by 2035, largely due to retirements and a lack of new professionals entering the field.
- 🤖 AI in Healthcare: Artificial intelligence (AI) is being integrated into healthcare to assist with the management of data and to improve diagnostic accuracy and efficiency.
- 🛠️ AI Implementation Levels: AI is being implemented at various levels in healthcare, including individual imaging devices, departmental workflow optimization, and enterprise-level patient flow management.
- 👥 Personalized Medicine: The shift towards personalized medicine, based on individual genetic differences, is transforming healthcare from a 'one size fits all' approach to tailored treatments and diagnostics.
- 🔑 Unlocking Potential: AI's potential to highlight critical cases, such as pneumothorax, can significantly improve patient outcomes by ensuring timely diagnoses and prioritizing urgent cases.
- 🤝 Need for Partnerships: GE Healthcare recognizes the importance of partnerships for developing AI applications and ensuring their clinical relevance and utility.
- 🚀 Future Opportunities: The rise of digitization will lead to the disappearance of certain jobs while creating new ones, emphasizing the importance of adaptability and a high 'Technology Quotient' (TQ) for future success.
Q & A
What is the role of the speaker in the context of the script?
-The speaker is the Chief Medical Officer for GE Healthcare in Europe, a radiologist, and a professor at Hamburg University, responsible for overseeing medical affairs, medical education, and healthcare in Europe.
How rapidly is medical data doubling according to the speaker's presentation?
-The speaker mentions that in 2010, medical data doubled every 3.5 years, but by 2020, it was doubling in just 0.2 years, which equates to approximately every 73 days.
What is the significance of the number of articles published in medical journals in relation to the data explosion?
-The speaker points out that there are 5,600 medical journals publishing 800,000 articles annually, emphasizing the sheer volume of new information being generated in the medical field.
What is the comparison made by the speaker between the amount of information in a mammogram and a New York telephone book?
-The speaker illustrates the vast amount of data in medical imaging by stating that there is more information in a mammogram than in the telephone book of New York.
How has the workload of a radiologist changed over the past 15 years according to the script?
-The speaker notes that a radiologist in a 12-hour shift now looks at 50,000 images, compared to only 500 images 15 years ago, indicating a significant increase in data to be analyzed.
What is the estimated number of patients dying annually in Europe due to medical errors, as mentioned in the script?
-The speaker estimates that 350,000 patients die every year in Europe due to medical errors, comparing this number to the population of a city like Venice or Toulouse.
What is the current global shortage of healthcare workers, and what is the projected shortage by 2035?
-The speaker states that the current global shortage of healthcare workers is seven million, with an estimated shortage of fourteen million by 2035.
What is the speaker's view on the role of disruptive technology in healthcare?
-The speaker believes that the best disruption is an innovation that works in the background without being obtrusive, rather than technology that disrupts the workflow of healthcare professionals.
What does the speaker mean by 'personalized medicine' and how does it differ from traditional evidence-based medicine?
-Personalized medicine refers to an approach that tailors diagnosis and treatment to the individual's unique genetic makeup, as opposed to traditional evidence-based medicine, which often follows a one-size-fits-all approach.
Can you explain the example of personalized medicine provided in the script involving Herceptin-positive breast cancer?
-The speaker uses the example of Herceptin-positive breast cancer, where a specific monoclonal antibody called trastuzumab (Herceptin) can extend life but has side effects. Personalized medicine ensures that only patients who are Herceptin positive receive the drug, avoiding unnecessary side effects for others.
What are the three levels of AI application in healthcare as described by the speaker?
-The speaker identifies three levels of AI application in healthcare: individual level (integrating AI into medical scanners), departmental level (using AI to streamline workflows in radiology departments), and enterprise level (using AI for patient flow management in hospitals or hospital networks).
What is the purpose of the 'Command Center' in a hospital as mentioned in the script?
-The 'Command Center' in a hospital uses predictive analytics to manage patient flow and experience, providing real-time data on bed availability and other resources to optimize hospital operations.
Why is the speaker emphasizing the importance of partnerships in developing AI applications for healthcare?
-The speaker emphasizes partnerships because they believe that the majority of smart people and innovative ideas are outside of GE. Partnerships are crucial for developing clinically useful applications and for gaining insights into the practical application and user experience of these technologies.
What does the speaker suggest is the key to thriving and surviving in the future job market, particularly in relation to AI and digitization?
-The speaker introduces the concept of 'Technology Quotient' (TQ), which measures one's openness to embracing new technologies. A high TQ indicates an ability to adapt to digitization and is key to thriving in the future job market.
Will AI replace doctors and radiologists according to the speaker's perspective?
-The speaker believes that AI will not replace doctors and radiologists but will instead augment their capabilities, similar to how autopilot in airplanes has not replaced human pilots but has enhanced their capabilities.
What is the speaker's final suggestion regarding the adoption of AI in healthcare?
-The speaker suggests that healthcare professionals should responsibly embrace AI instead of fearing it, as it offers tremendous opportunities to improve diagnostics, speed, and accuracy, and to humanize radiology.
Outlines
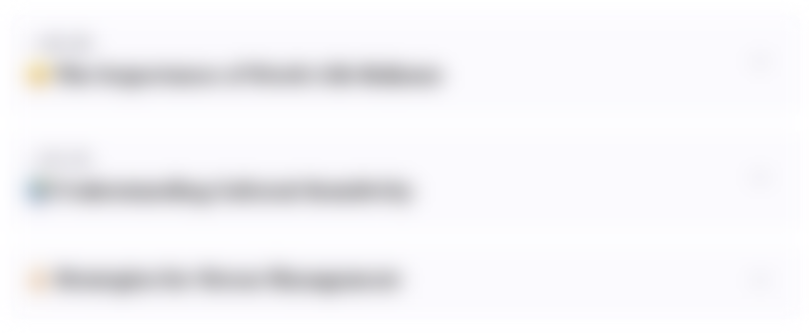
This section is available to paid users only. Please upgrade to access this part.
Upgrade NowMindmap
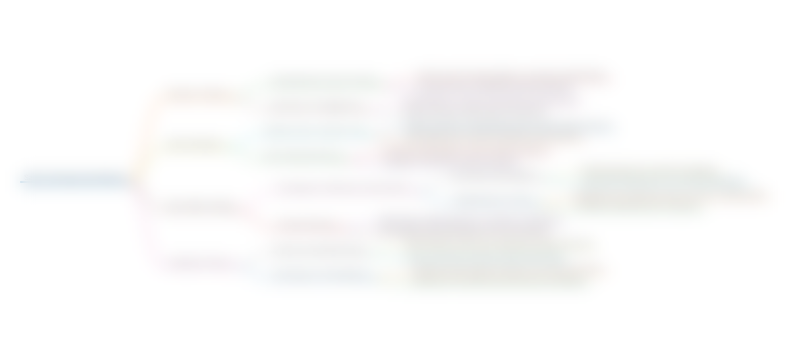
This section is available to paid users only. Please upgrade to access this part.
Upgrade NowKeywords
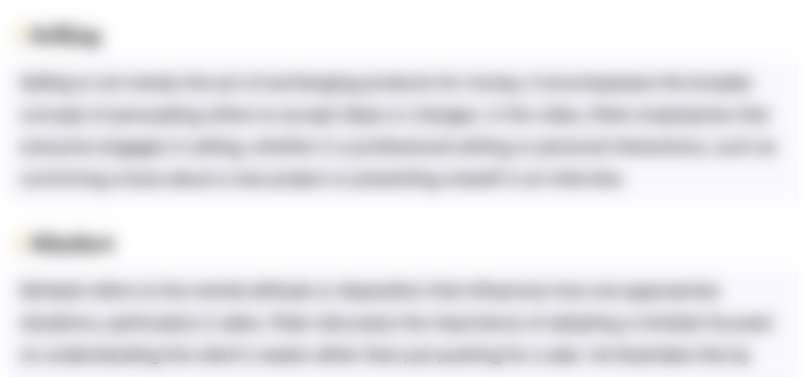
This section is available to paid users only. Please upgrade to access this part.
Upgrade NowHighlights
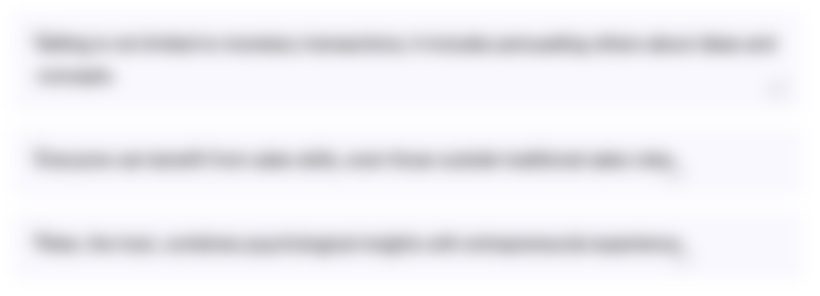
This section is available to paid users only. Please upgrade to access this part.
Upgrade NowTranscripts
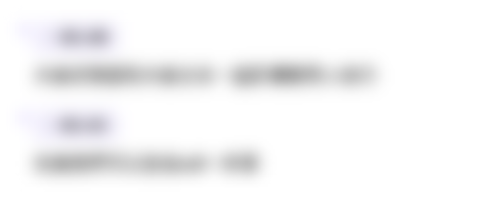
This section is available to paid users only. Please upgrade to access this part.
Upgrade NowBrowse More Related Video

The Exciting, Perilous Journey Toward AGI | Ilya Sutskever | TED
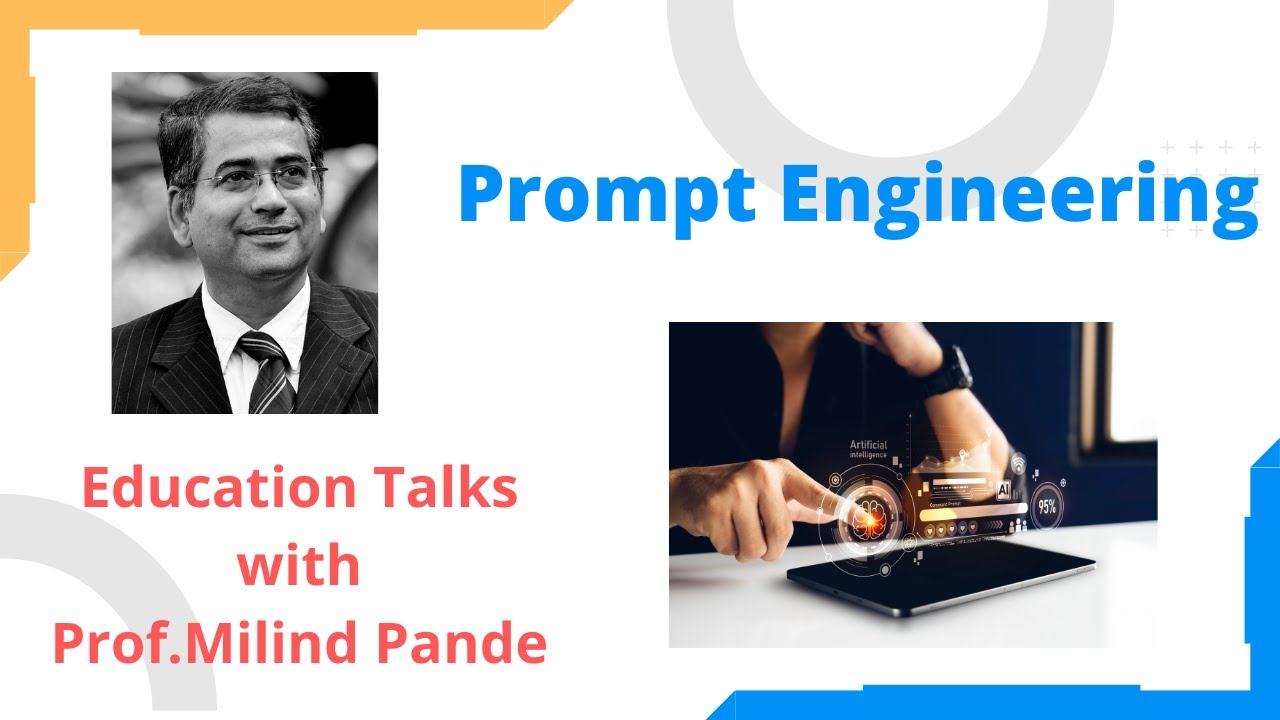
What is Prompt Engineering in Marathi? |Prompt Engineering| Prof. Milind Pande
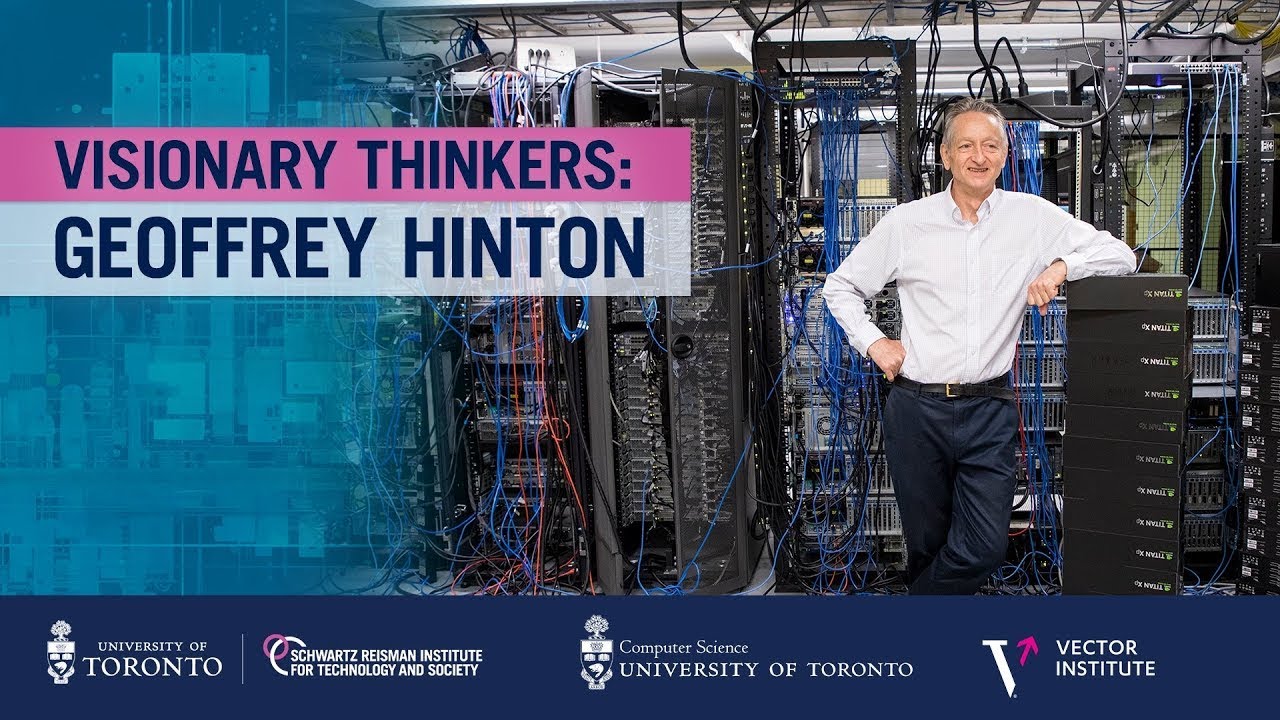
Geoffrey Hinton | Will digital intelligence replace biological intelligence?

What is Artificial Intelligence? with Mike Wooldridge
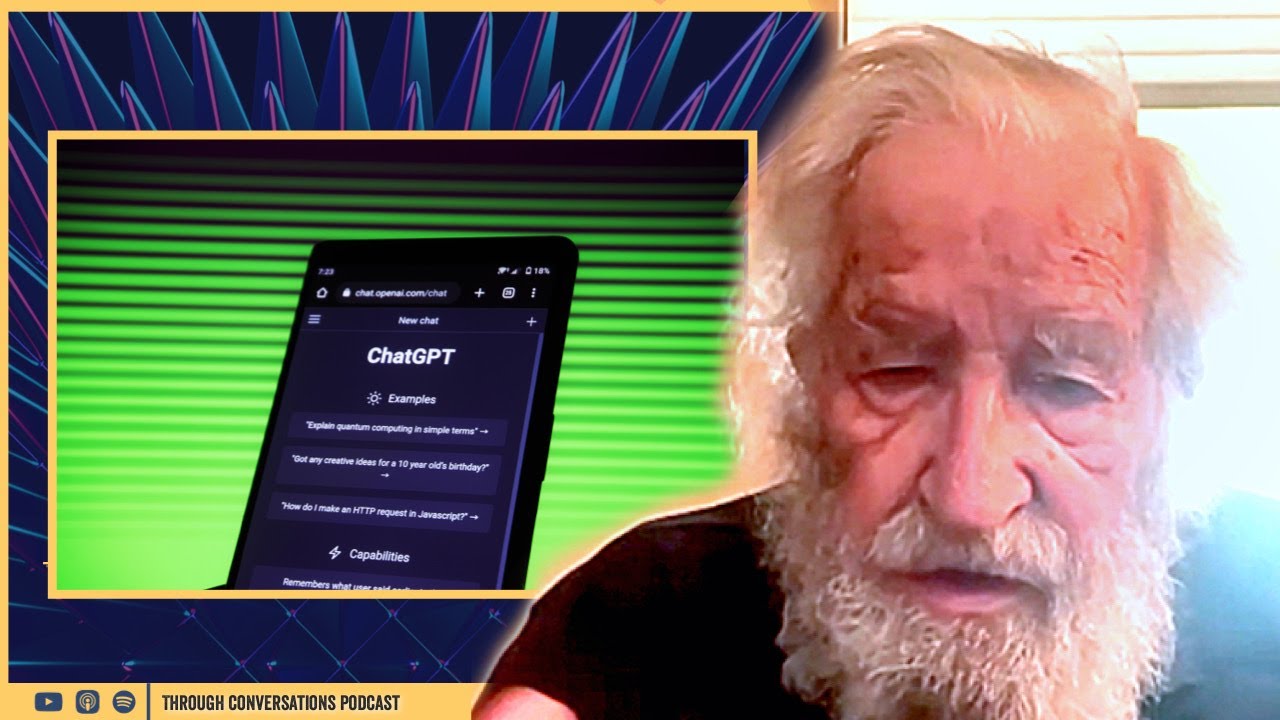
Noam Chomsky on Artificial Intelligence, ChatGPT.
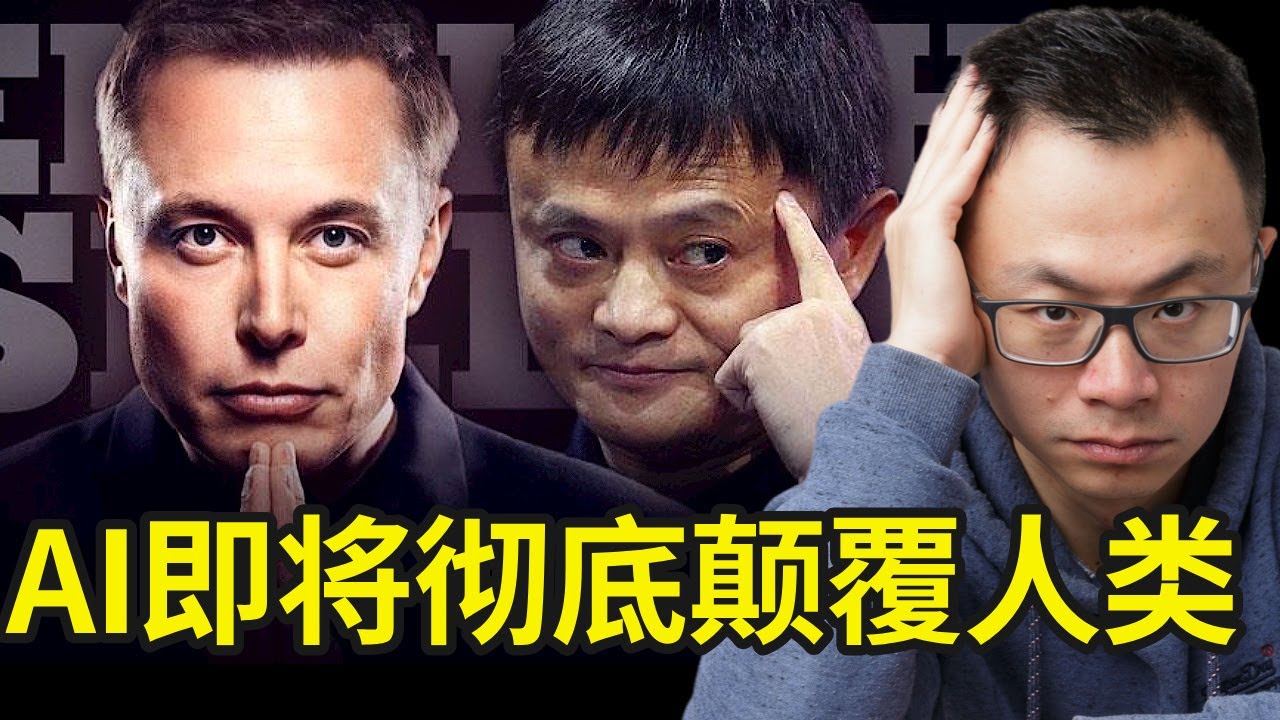
细思极恐!这个视频,你一定要看完!事关你的未来所有财富,事业和人生...最近马斯克,马云,扎克伯格纷纷放弃最赚钱的生意,全力冲刺这个赛道。未来10年,最好赚的钱,只会在这里!
5.0 / 5 (0 votes)