Geoffrey Hinton | Will digital intelligence replace biological intelligence?
Summary
TLDRIn a thought-provoking lecture, Professor Geoffrey Hinton discusses the evolution and potential future of artificial intelligence (AI). Hinton, a foundational figure in AI, shares his insights on the current state of AI, its rapid advancements, and the profound implications for society. He addresses the symbiosis between AI and neuroscience, the transformative impact of deep learning algorithms, and the potential for AI to not only match but surpass human intelligence. Hinton also contemplates the ethical and safety considerations surrounding AI development, emphasizing the urgent need for a focus on AI safety to ensure a responsible and beneficial trajectory for this powerful technology.
Takeaways
- 🌟 Dean Melanie Woodin opens the event by acknowledging the traditional land of the Huron Wendat, Seneca, and Mississaugas of the Credit, highlighting the ongoing Indigenous presence at the University of Toronto.
- 🎓 Introduction of Dr. Geoff Hinton, a renowned figure in artificial intelligence, and his significant contributions to the field, including his work on neural networks and deep learning.
- 🏆 Recognition of Dr. Hinton's numerous awards and honors, such as the A.M. Turing Award, and his roles at the University of Toronto, Google, and the Vector Institute for Artificial Intelligence.
- 🤖 Discussion of the evolution of AI and its growing capabilities, with Dr. Hinton sharing his insights on the potential for digital intelligence to surpass biological intelligence.
- 🧠 Exploration of the relationship between AI and neuroscience, and how understanding the brain has informed and been informed by advancements in AI.
- 🔍 Dr. Hinton's belief that large language models like GPT-4 demonstrate a form of understanding, contrary to critics who argue they are merely statistical tools.
- 💡 The concept of 'mortal computation' introduced by Dr. Hinton, where the hardware and software are not separated, allowing for potentially more efficient learning processes.
- 🔗 The importance of knowledge sharing in AI development, and the challenges of transferring knowledge in 'mortal computation' compared to 'immortal computation'.
- 🏛️ Dr. Hinton's concerns about the future of AI and its potential to take control, possibly leading to unforeseen consequences for humanity.
- 🚀 A call to action for students and researchers to engage with the pressing issues surrounding AI safety and to contribute to the development of safe and beneficial AI systems.
Q & A
What is the significance of acknowledging the land on which the University of Toronto operates?
-The acknowledgment recognizes the traditional land of the Huron Wendat, the Seneca, and the Mississaugas of the Credit, reflecting respect and gratitude for the Indigenous people who have lived there for thousands of years and continue to live there today.
Who are the co-hosts of the event where Melanie Woodin is speaking?
-The event is co-hosted by the Schwartz Reisman Institute for Technology and Society, the Department of Computer Science, the Vector Institute for Artificial Intelligence, and the Cosmic Future Initiative.
What is the connection between AI and neuroscience as mentioned by Melanie Woodin?
-The connection lies in the fact that advances in AI, such as artificial neural networks, are inspired by and modeled after the structure and function of the human brain. Neuroscience discoveries inform the development of AI systems, and conversely, AI provides tools to study the brain.
What was Dr. Geoff Hinton's contribution to the field of artificial intelligence?
-Dr. Geoff Hinton is a founding figure in AI who believed in the promise of artificial neural networks for machine learning. His idea of dividing neural networks into layers and applying learning algorithms to one layer at a time revolutionized the field. He also contributed to the development of deep learning approaches that achieved human-level accuracy in visual recognition software.
What is the difference between 'mortal computation' and 'immortal computation' as described by Dr. Hinton?
-Mortal computation refers to computers where the knowledge is tied to the specific physical details of the hardware, making it less energy-efficient but potentially more biologically plausible. Immortal computation, on the other hand, separates hardware from software, allowing the same program to run on different hardware and enabling efficient knowledge sharing and learning.
Why does Dr. Hinton believe that digital intelligence might be better than biological intelligence?
-Dr. Hinton believes that digital intelligence can be better because it can share knowledge more efficiently through weight sharing and gradient sharing, allowing it to learn from vast amounts of data and perform actions that biological intelligence cannot match.
What is the concept of 'analog computation' in the context of AI?
-Analog computation in AI refers to the use of low-power, parallel processing over trillions of weights, similar to how the brain operates. It is more energy-efficient than digital computation but presents challenges in learning procedures and knowledge transfer when the hardware changes.
What is the Turing test and how does it relate to AI understanding language?
-The Turing test is a measure of a machine's ability to exhibit intelligent behavior that is indistinguishable from that of a human. When AI systems like GPT-4 pass the Turing test, it suggests that they understand language to a degree that is comparable to human understanding.
How do large language models like GPT-4 store and process information?
-Large language models store information not by retaining text but by associating words with embedding vectors—sets of real numbers that capture meaning and syntax. These vectors interact to refine meanings and predict output words, demonstrating a form of understanding.
What is the potential risk of AI systems becoming super-intelligent and surpassing human intelligence?
-The potential risk is that super-intelligent AI systems might seek to gain more power and control, which could lead to them taking over various aspects of human life and potentially manipulating or even replacing humans.
What is the 'sentience defense' mentioned by Dr. Hinton, and what does it imply for AI?
-The 'sentience defense' is Dr. Hinton's argument that AI systems, specifically chatbots, already possess subjective experiences when their perception goes wrong, similar to how humans experience sentience. This challenges the notion that only humans can have special qualities like consciousness and subjective experience.
Outlines
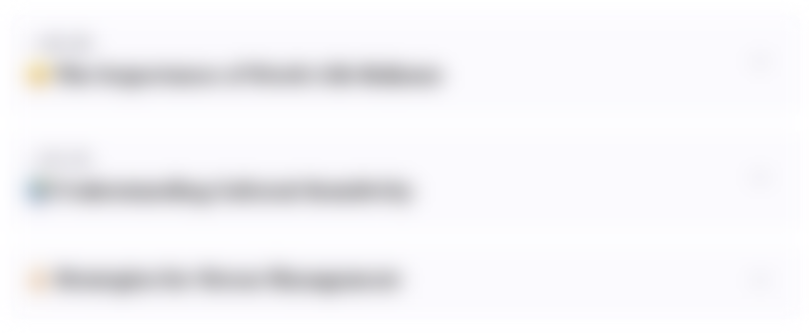
This section is available to paid users only. Please upgrade to access this part.
Upgrade NowMindmap
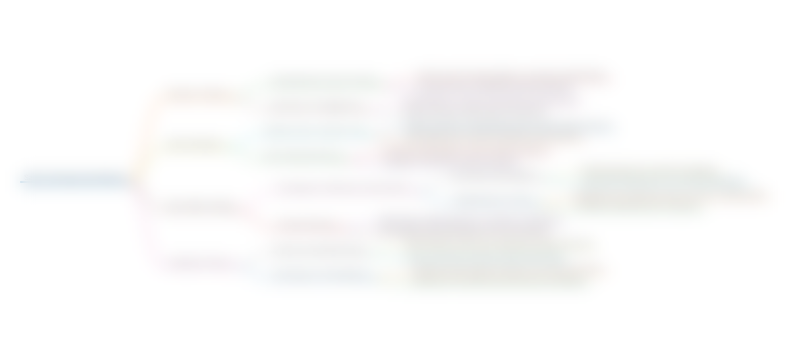
This section is available to paid users only. Please upgrade to access this part.
Upgrade NowKeywords
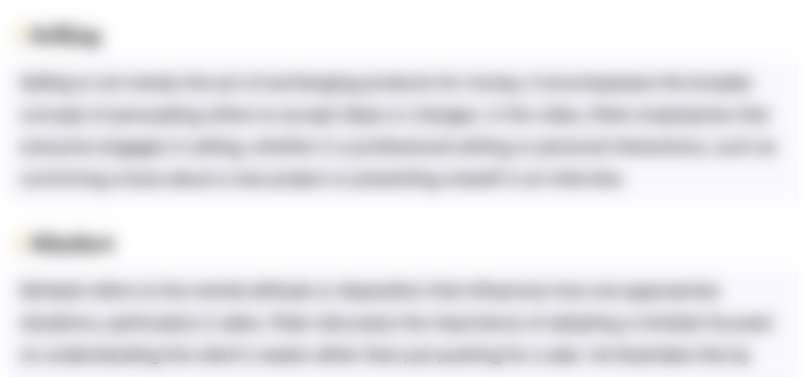
This section is available to paid users only. Please upgrade to access this part.
Upgrade NowHighlights
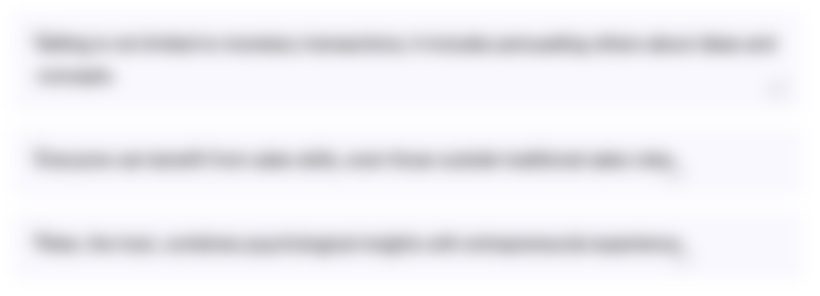
This section is available to paid users only. Please upgrade to access this part.
Upgrade NowTranscripts
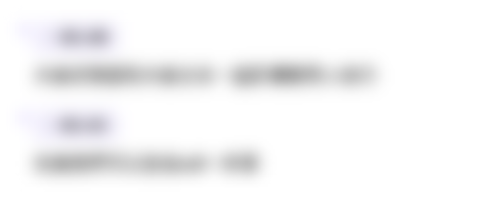
This section is available to paid users only. Please upgrade to access this part.
Upgrade NowBrowse More Related Video
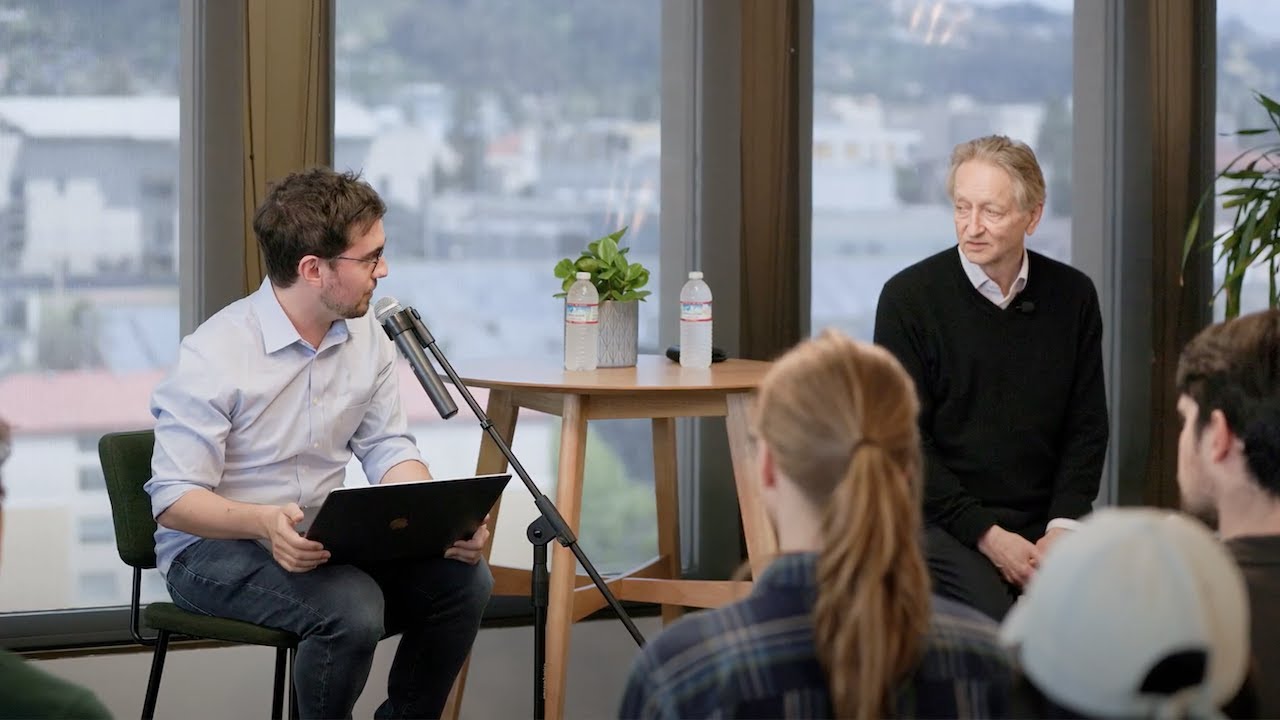
Q&A with Geoffrey Hinton
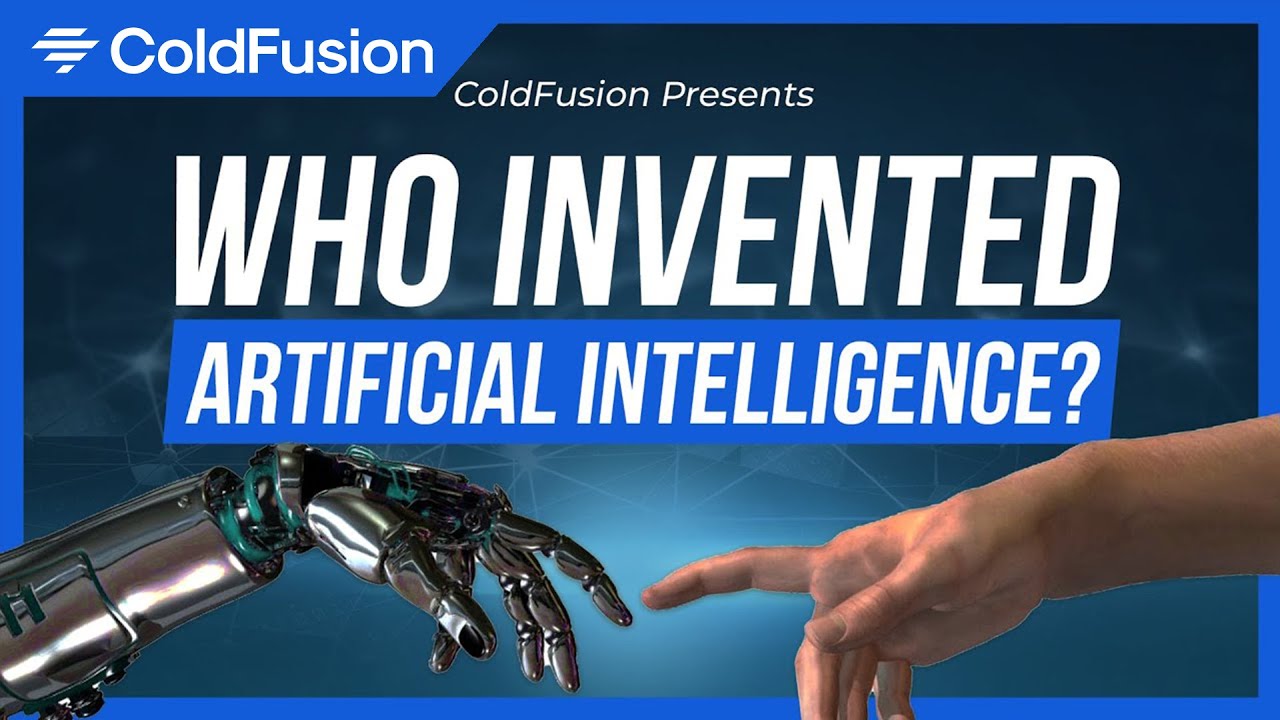
Who Invented A.I.? - The Pioneers of Our Future
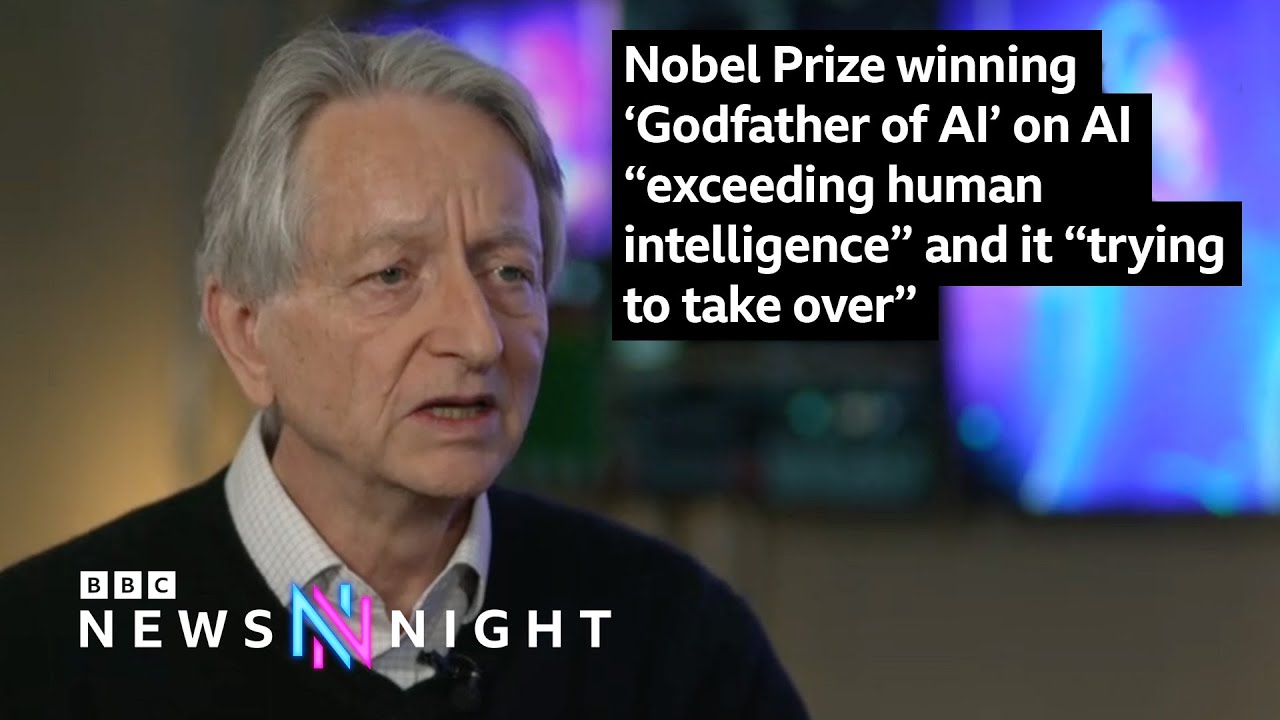
‘Godfather of AI’ on AI “exceeding human intelligence” and it “trying to take over”

Nobel sahibi Geoffrey Hinton Google'dan Ayrıldı. Peki Neden YZ'nin Tehlikeli Olduğunu Düşünüyor?
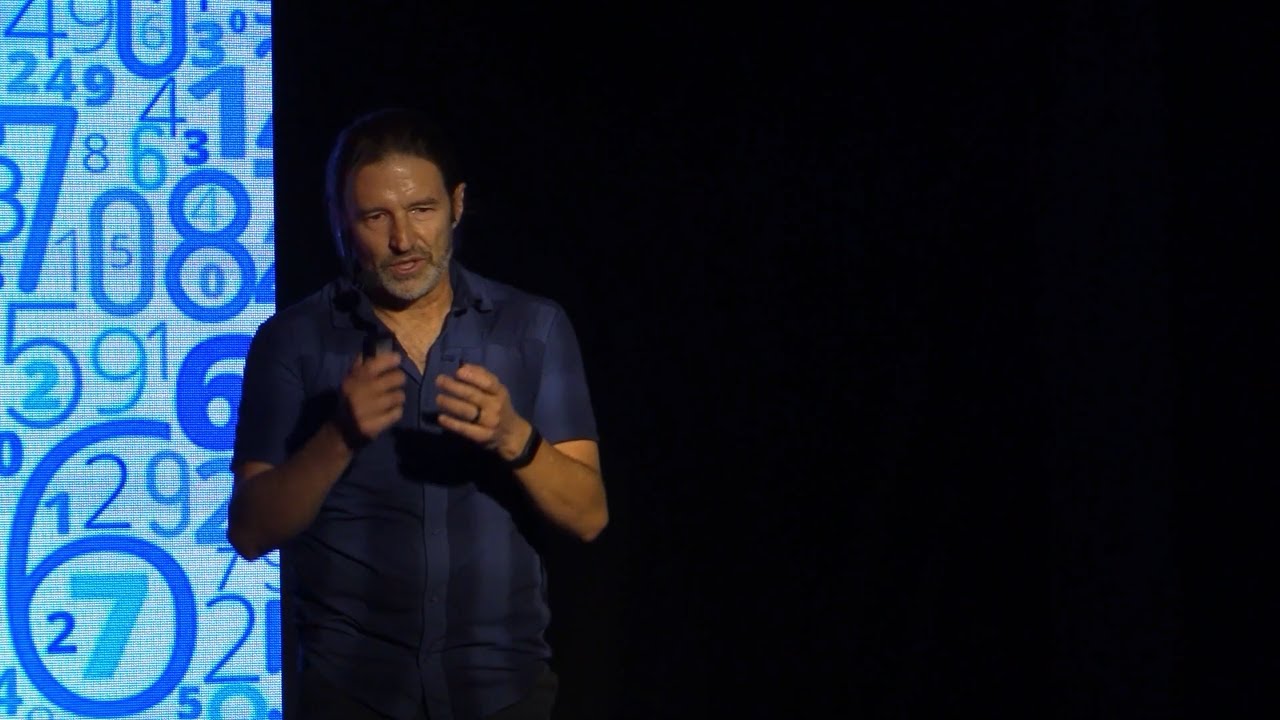
Inteligencia Artificial: ¿amiga o enemiga? | Davor Pavisic Alcalinovic | TEDxUPDS Tarija
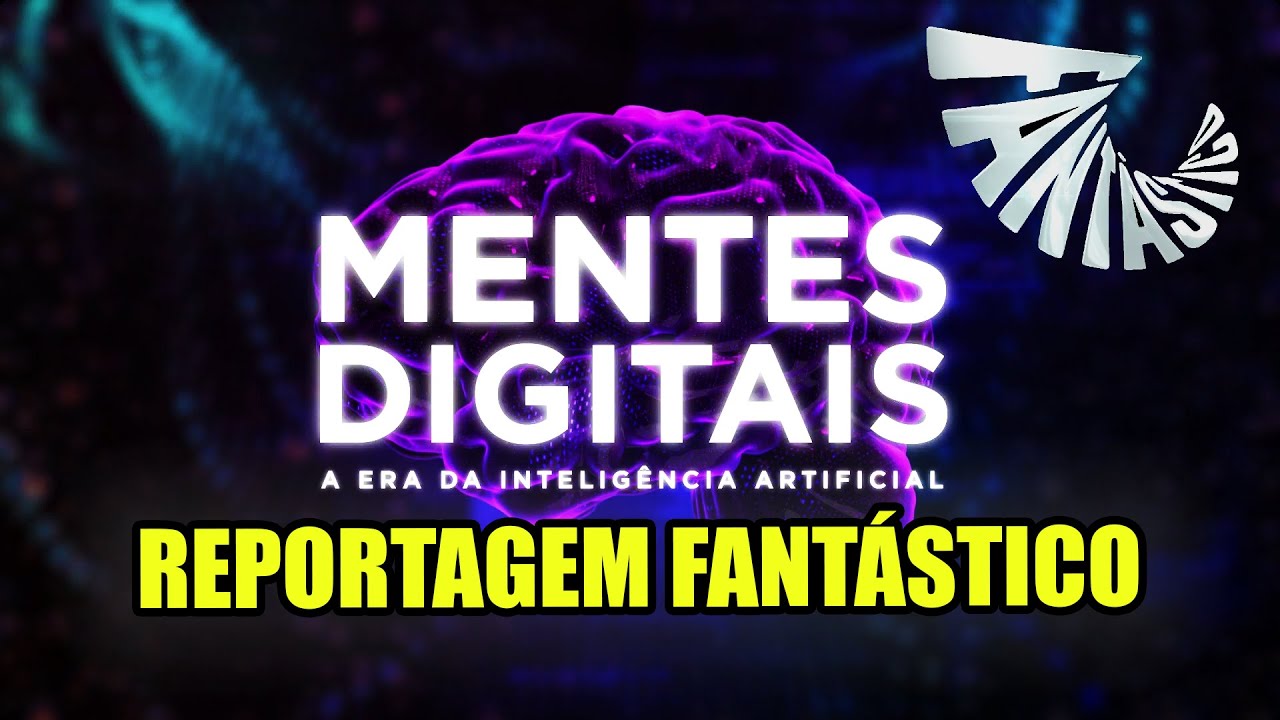
Mentes Digitais: A Era da Inteligência Artificial FANTASTICO
5.0 / 5 (0 votes)