AI, Machine Learning, Deep Learning and Generative AI Explained
Summary
TLDRThis video script delves into the distinctions between artificial intelligence (AI), machine learning, and deep learning, clarifying misconceptions and exploring their interrelations. It highlights the evolution of AI from its early days to the rise of generative AI, including large language models and deepfakes. The script emphasizes the exponential growth in AI adoption due to foundation models, which have transformed our interaction with technology, generating new content and summarizing existing information for easier consumption.
Takeaways
- 🧠 Artificial Intelligence (AI) aims to simulate human intelligence with a computer, encompassing the ability to learn, infer, and reason.
- 📚 The concept of AI has been around since the early days of computer science, with early work in languages like Lisp and Prolog leading to the development of expert systems.
- 🤖 Machine Learning is a subset of AI where machines learn from data without being explicitly programmed, becoming adept at pattern recognition and prediction.
- 🔍 Machine Learning became more popularized in the 2010s, with its ability to spot outliers making it particularly useful in fields like cybersecurity.
- 🧠🔽 Deep Learning involves neural networks with multiple layers to simulate the human brain's operation, becoming a significant advancement in the 2010s.
- 🌐 Generative AI is the latest advancement, focusing on creating new content through technologies like large language models, audio models, and video models.
- 🏗️ Foundation Models, such as large language models, form the basis for technologies that predict and generate text, sentences, and even entire documents.
- 🎶 The script uses the analogy of music composition to explain how Generative AI can create new content from existing information.
- 🎭 Deepfakes are a part of Generative AI, capable of recreating voices and faces, with potential uses in entertainment and potential for abuse.
- 📈 The adoption of AI has accelerated with the rise of Machine Learning, Deep Learning, and Generative AI, shifting from a slow start to widespread application.
- 📊 Foundation Models have significantly altered the adoption curve of AI, leading to its integration and application across various industries.
Q & A
What is the primary goal of artificial intelligence (AI)?
-The primary goal of AI is to simulate with a computer something that would match or exceed human intelligence, which generally includes the ability to learn, infer, and reason.
How are machine learning and AI different?
-Machine learning is a subset of AI where the machine learns from data without being explicitly programmed, whereas AI is a broader field that includes any attempt to simulate human intelligence using computers.
What does the term 'deep learning' refer to in the context of AI?
-Deep learning refers to a subset of machine learning that uses neural networks with multiple layers to simulate the human brain's operations, allowing the system to learn and make decisions based on complex patterns in data.
What is a generative AI and how does it relate to foundation models?
-Generative AI is a type of AI that can generate new content, such as text, audio, or video. It is related to foundation models, which are underlying models like large language models that enable the generation of content by making predictions based on learned patterns.
Can you explain the concept of 'foundation models' in AI?
-Foundation models are the basic models that underpin advanced AI technologies. An example is a large language model that can predict and generate text based on the input it receives, serving as a foundation for technologies like chatbots and content generation.
How do deepfakes fit into the realm of AI?
-Deepfakes are a product of generative AI, specifically using models that can manipulate audio and visual data to create realistic but fake representations of individuals saying or doing things they never did.
What is the significance of the term 'generative' in the context of AI technologies?
-The term 'generative' in AI refers to the ability of these technologies to create new content rather than just processing or analyzing existing data. This includes generating text, music, images, or even videos.
How did the adoption of AI technologies evolve over time?
-The adoption of AI technologies started slowly with early AI research projects. It gained more traction with the rise of machine learning in the 2010s, and then skyrocketed with the advent of deep learning, foundation models, and generative AI.
What role does machine learning play in cybersecurity?
-In cybersecurity, machine learning is used to spot outliers and anomalies in data, helping to identify and prevent unauthorized or malicious activities by detecting patterns that deviate from the norm.
How does the script simplify complex AI concepts for a broader audience?
-The script simplifies AI concepts by using analogies, such as comparing generative AI to music composition, and by making generalizations to avoid overly technical explanations that could confuse non-experts.
What is the purpose of the video script in addressing frequently asked questions about AI?
-The purpose of the video script is to clear up myths and misconceptions around AI, machine learning, and deep learning by addressing common questions and providing straightforward explanations of these technologies.
Outlines
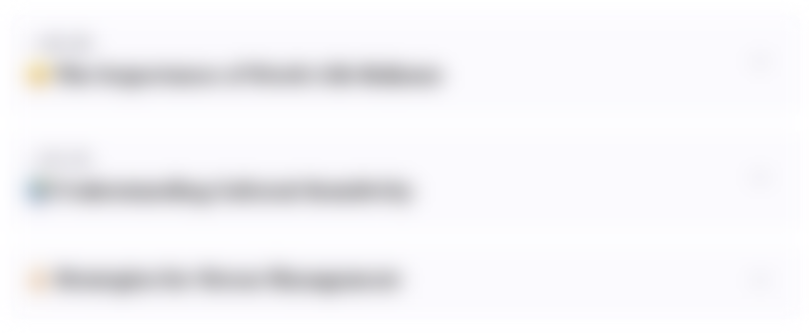
This section is available to paid users only. Please upgrade to access this part.
Upgrade NowMindmap
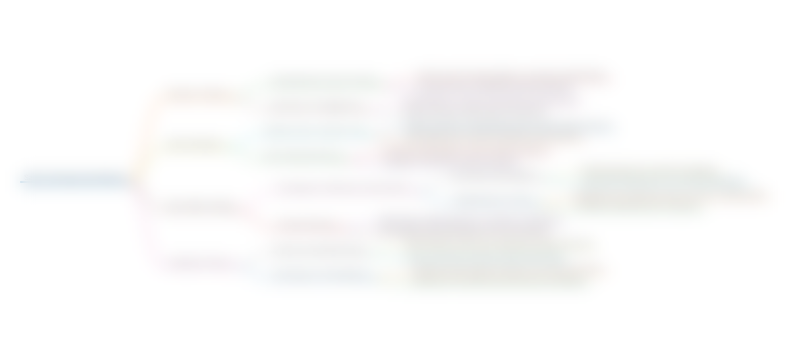
This section is available to paid users only. Please upgrade to access this part.
Upgrade NowKeywords
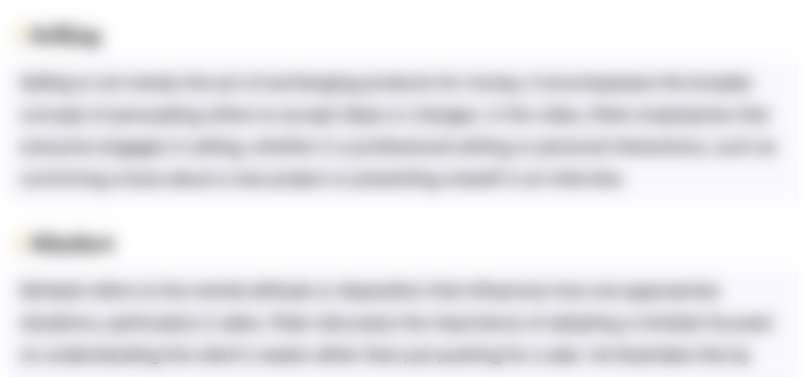
This section is available to paid users only. Please upgrade to access this part.
Upgrade NowHighlights
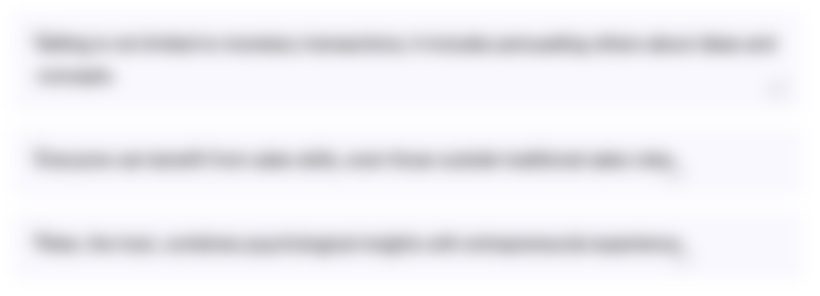
This section is available to paid users only. Please upgrade to access this part.
Upgrade NowTranscripts
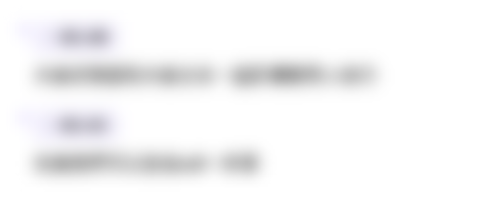
This section is available to paid users only. Please upgrade to access this part.
Upgrade NowBrowse More Related Video
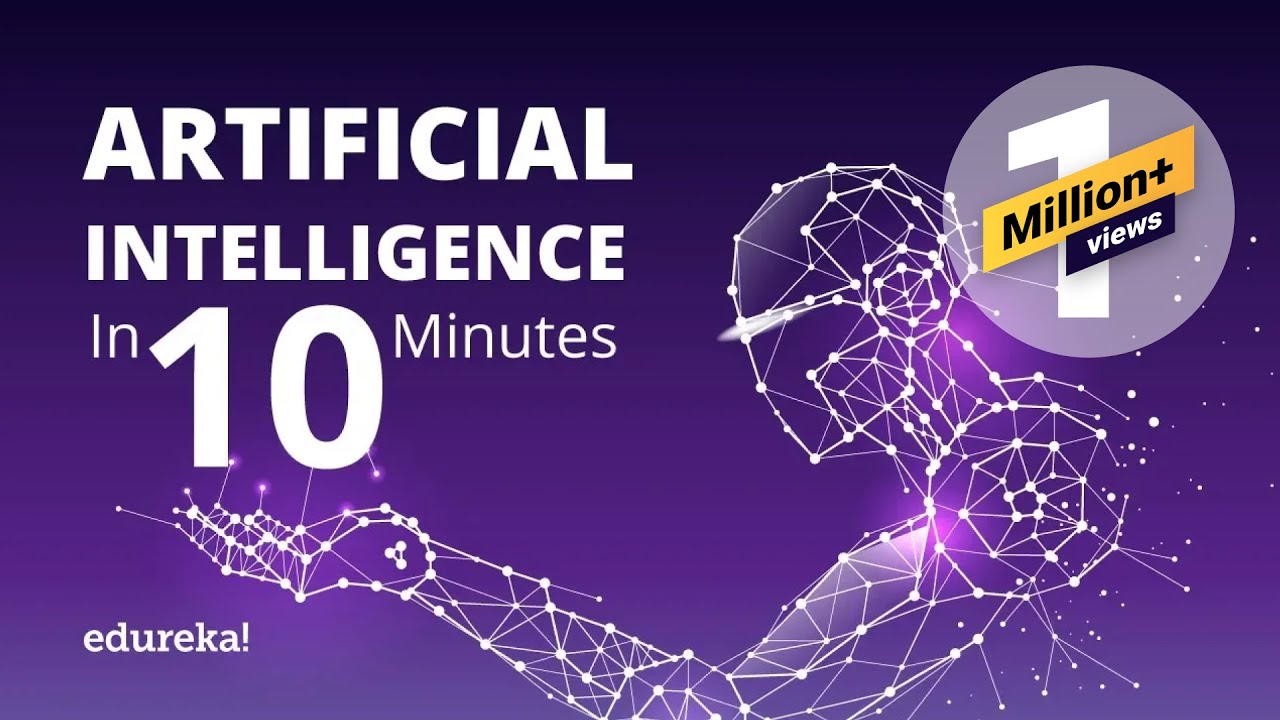
What Is Artificial Intelligence? | Artificial Intelligence (AI) In 10 Minutes | Edureka
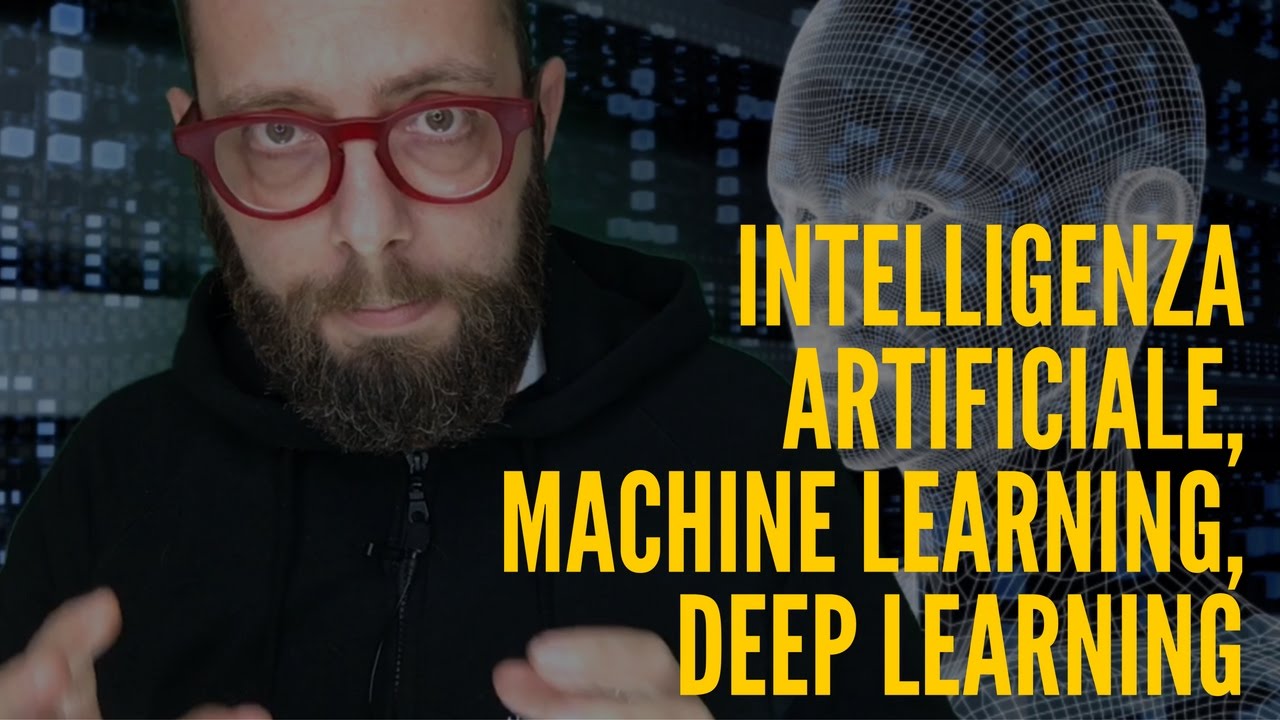
35. Che differenza c'è tra Intelligenza Artificiale, Machine Learning e Deep learning? #36
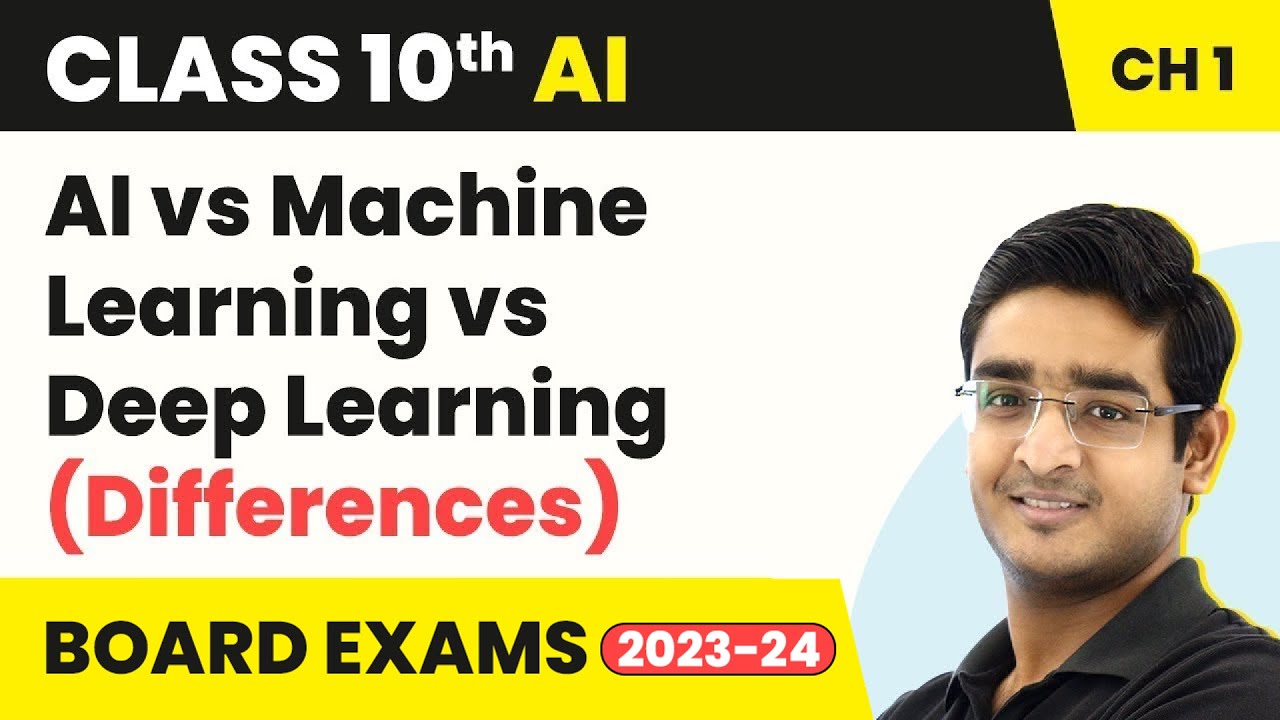
Artificial Intelligence Class 10 Ch 1 |AI vs Machine Learning vs Deep Learning (Differences) 2022-23
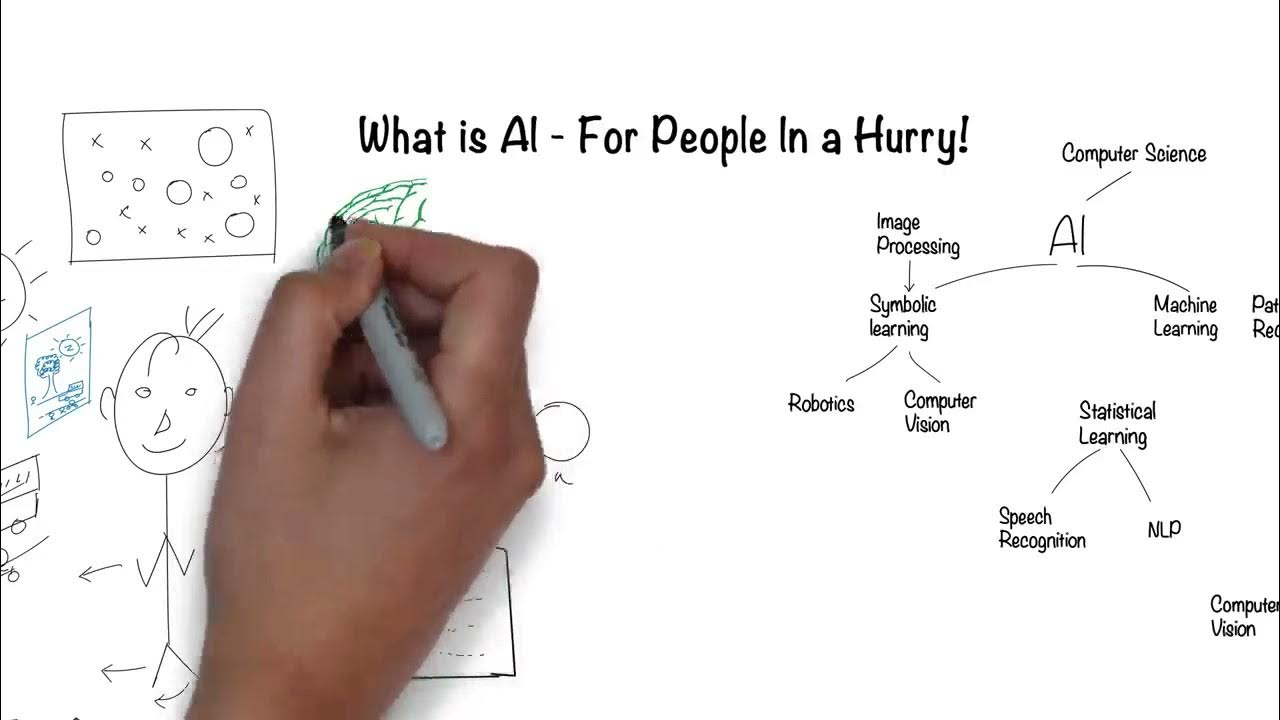
Artificial Intelligence (AI) for People in a Hurry
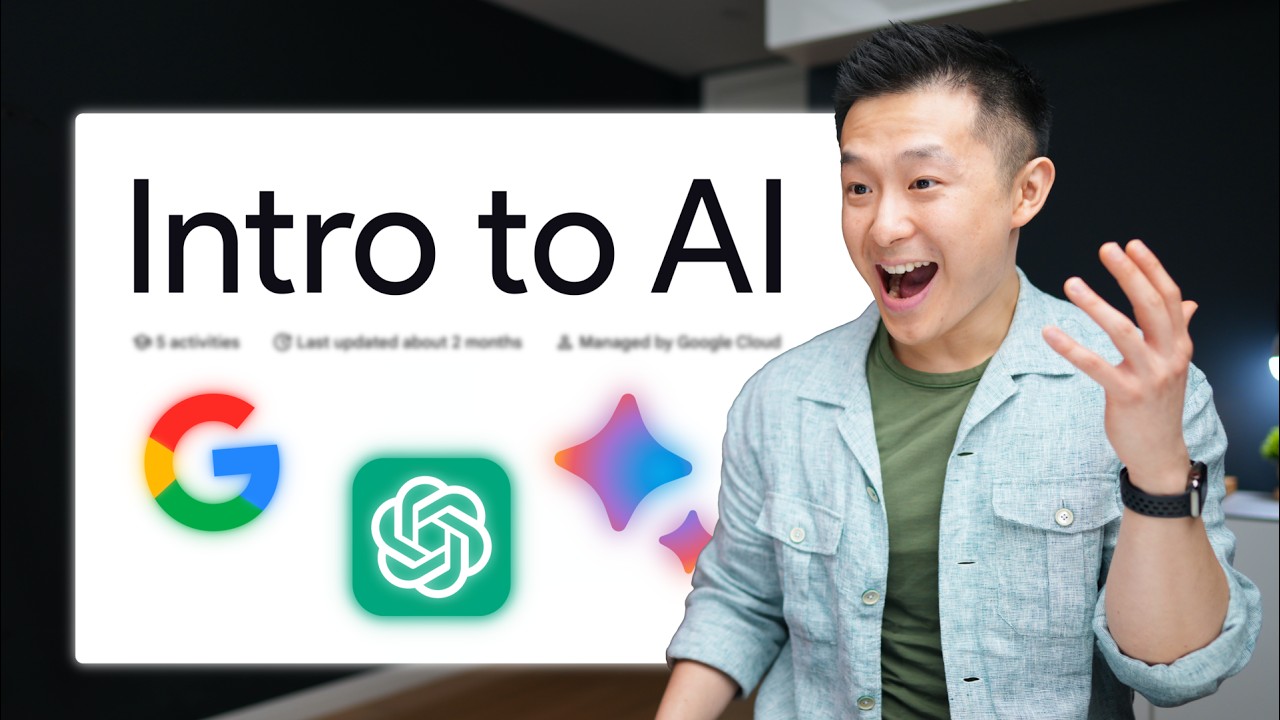
Google’s AI Course for Beginners (in 10 minutes)!
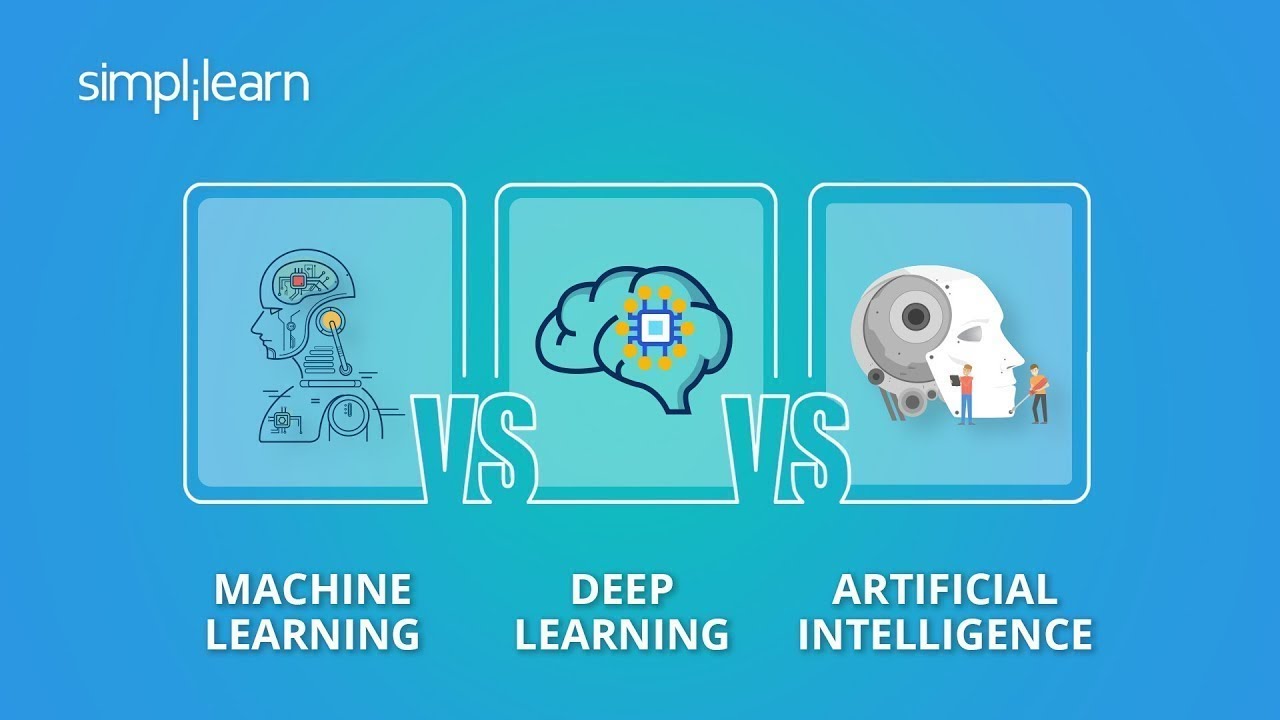
Machine Learning vs Deep Learning vs Artificial Intelligence | ML vs DL vs AI | Simplilearn
5.0 / 5 (0 votes)