Cisco Artificial Intelligence and Machine Learning Data Center Networking Blueprint
Summary
TLDRNemanja Kamenica, a technical marketing engineer at Cisco, introduces the AI/ML data center networking blueprint, a comprehensive guide to optimizing networks for AI workloads. He highlights the importance of AI in various industries, from healthcare to retail, and explains the differentiation between training and inference AI clusters. The presentation delves into the technical requirements for these clusters, including high bandwidth and low latency, and showcases Cisco's Nexus 9000 switches as a solution. Through a detailed demo, Kamenica demonstrates congestion management techniques like PFC and ECN, crucial for maintaining a lossless network environment for AI clusters. The session concludes with resources Cisco provides to help customers implement these AI networking solutions.
Takeaways
- 📊 AI and ML workloads require specialized network configurations to handle their unique data traffic patterns efficiently.
- 💻 There are two main types of AI clusters: distributed training clusters for model training, which require high bandwidth and low latency, and product inference clusters for model deployment, which prioritize real-time responses and high availability.
- 📦 On-premises AI clusters offer full control and constant availability, whereas cloud-based solutions provide flexibility and scalability with cost considerations.
- 🛠 Key challenges in building AI clusters include managing rapidly doubling data volumes and models, necessitating scalable infrastructure to maintain performance and accuracy.
- 📚 Cisco's data center networking solutions, particularly the Nexus 9000 series, are designed to support AI/ML workloads with low latency, high throughput, and efficient node-to-node communication.
- 🔧 RDMA over Converged Ethernet (RoCEv2) is critical for efficient AI cluster operations, enabling direct memory access between nodes to reduce latency and increase throughput.
- 🚨 Network configurations for AI workloads must be non-blocking, lossless, and capable of handling congestion through mechanisms like Priority Flow Control (PFC) and Explicit Congestion Notification (ECN).
- 📡 Effective congestion management is crucial to prevent data loss and ensure continuous operation, especially in distributed AI training scenarios where data synchronization between nodes is constant.
- 📱 Cisco's Nexus Dashboard Fabric Controller and other tools offer advanced features for network visibility, congestion management, and QoS configuration, aiding in the deployment and management of AI clusters.
- 💻 Custom automation scripts and templates provided by Cisco can streamline the configuration of networks for AI/ML clusters, aligning with the automation of endpoint provisioning.
Q & A
What is the significance of AI/ML in modern data center networking according to Cisco's blueprint?
-The significance of AI/ML in modern data center networking lies in optimizing network configurations to support the unique demands of AI/ML workloads, such as high bandwidth, low latency, and lossless data transport, which are crucial for efficient model training and inference.
What are the two major types of AI clusters mentioned in the transcript?
-The two major types of AI clusters mentioned are the distributed training cluster, used for training AI models, and the inference cluster, used for applying the trained models to new data.
Why is high node-to-node communication important in a distributed training cluster?
-High node-to-node communication is crucial in a distributed training cluster because it enables the rapid exchange and processing of data samples between nodes, which is essential for updating and refining AI models efficiently.
null
-The key network requirements for a distributed training cluster include high bandwidth and low latency to facilitate quick data exchange and computation among nodes, which leads to faster model training times.
What does a product inference cluster require from the network?
-A product inference cluster requires the network to be real-time and highly available to handle numerous user requests simultaneously without significant delays.
What are the benefits and challenges of deploying AI clusters on-premises versus in the public cloud?
-Deploying AI clusters on-premises offers full control, data security, and constant availability but requires significant infrastructure and management. In contrast, public cloud deployment offers flexibility and scalability but can lead to increased costs and concerns over data security.
Why is network congestion management critical in AI/ML clusters?
-Network congestion management is critical in AI/ML clusters to avoid data loss and ensure efficient communication between nodes, which is essential for the accurate and timely training and inference of AI models.
What are Rocky V2 and RDMA, and why are they important for AI/ML workloads?
-Rocky V2 (RDMA over Converged Ethernet version 2) and RDMA (Remote Direct Memory Access) are technologies that provide low latency and high throughput data transport. They are crucial for AI/ML workloads to enable fast and efficient data transfer between nodes in the network.
How does Cisco's Nexus 9000 series support AI/ML data center requirements?
-Cisco's Nexus 9000 series supports AI/ML data center requirements by providing low latency, high bandwidth, and advanced features such as flow control and congestion management, which are essential for handling the demands of AI/ML workloads.
What role does quality of service (QoS) play in managing AI/ML network traffic?
-Quality of Service (QoS) plays a critical role in managing AI/ML network traffic by prioritizing different types of traffic, ensuring that important data, such as AI model updates, are transmitted quickly and reliably across the network.
Outlines
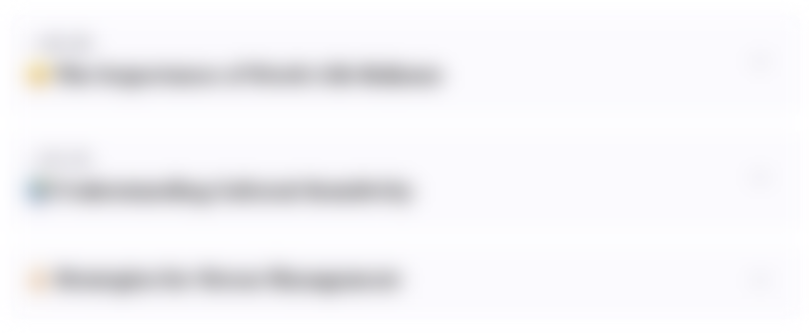
This section is available to paid users only. Please upgrade to access this part.
Upgrade NowMindmap
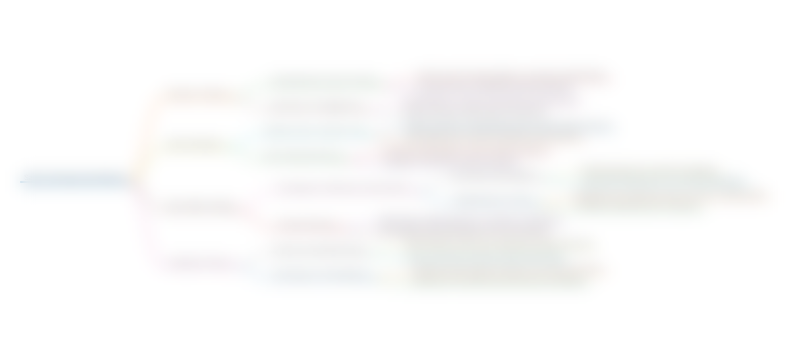
This section is available to paid users only. Please upgrade to access this part.
Upgrade NowKeywords
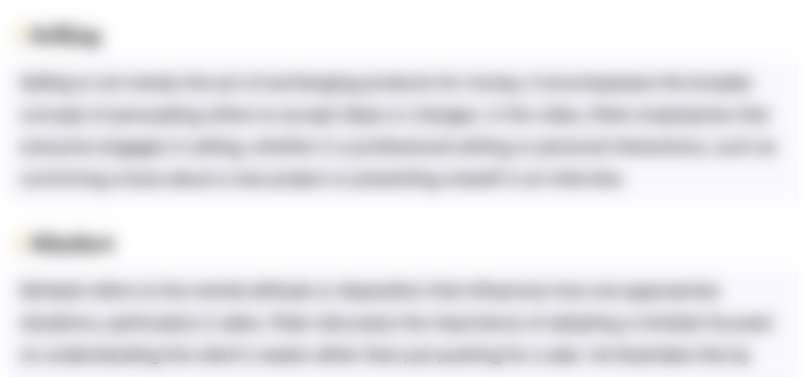
This section is available to paid users only. Please upgrade to access this part.
Upgrade NowHighlights
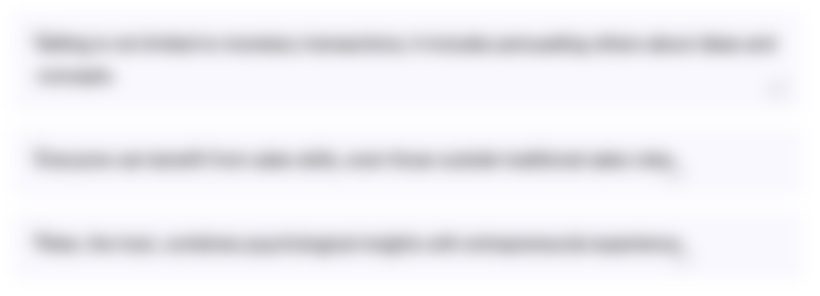
This section is available to paid users only. Please upgrade to access this part.
Upgrade NowTranscripts
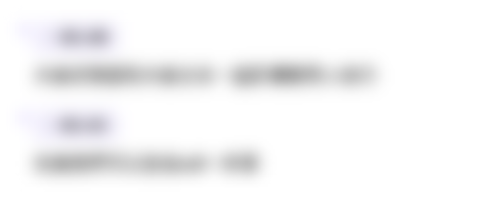
This section is available to paid users only. Please upgrade to access this part.
Upgrade NowBrowse More Related Video

Inside the World's Largest AI Supercluster xAI Colossus

Streamlined AI Development with NVIDIA DGX Cloud
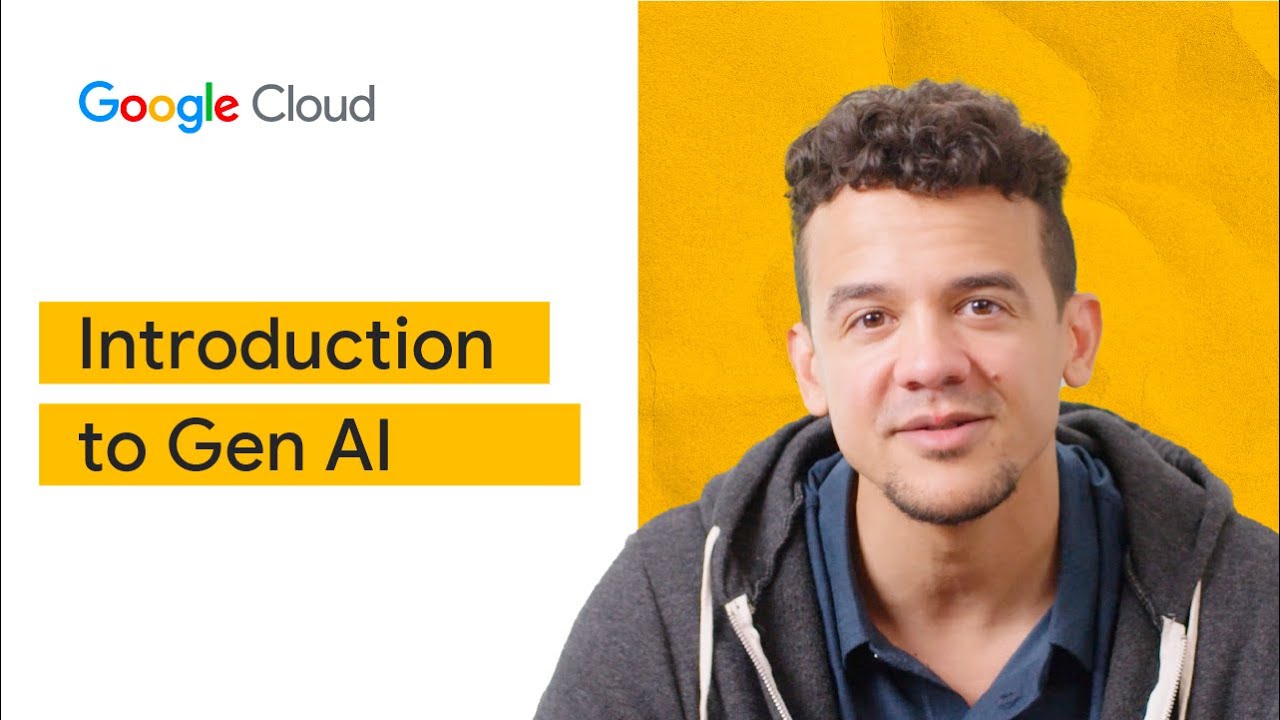
Introduction to Generative AI

What OmniVista Network Advisor, AIOps system, can do for your network infrastructure?
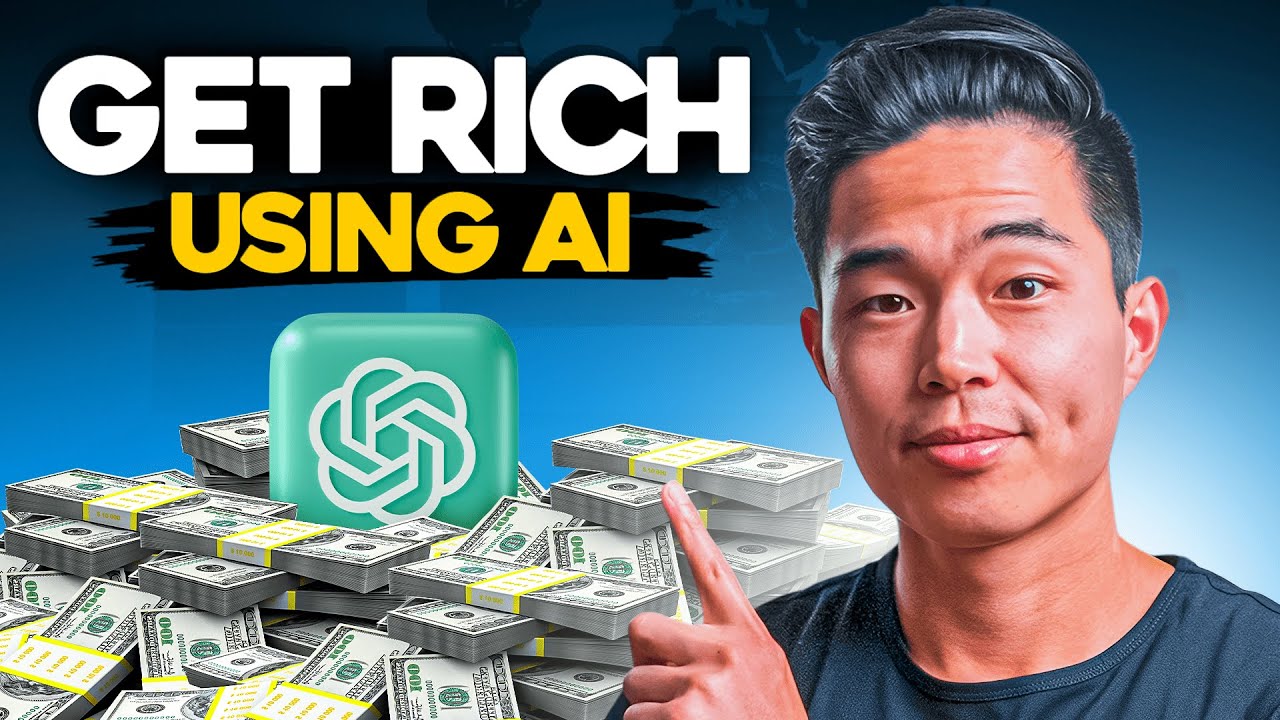
5 Genius Ways to Make Money From Home (Using AI)
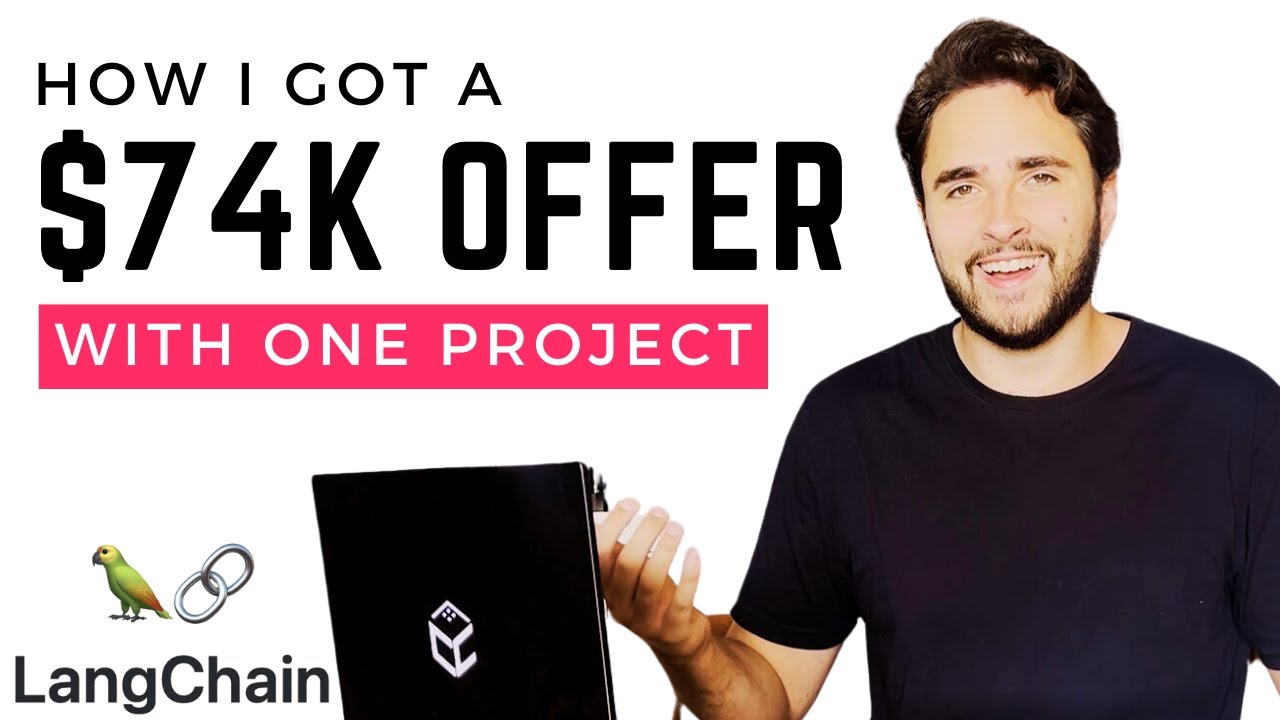
How I Became a Self-taught AI Engineer
5.0 / 5 (0 votes)