DreamDiffusion - Thought to Image Generation | Paper Summary
Summary
TLDRDreamDiffusionは、脳から記録されたEEG信号を入力として受け取り、それに合致する高品質な画像を生成する新しい方法を提示します。従来のテキストプロンプトに代わり、EEGを使用することで、夢を視覚化したり、言語障害のある人が自己表現できるようになるかもしれません。このビデオでは、EEG信号からの画像生成に向けた研究、挑戦、そしてそれを実現するための技術的なアプローチについて説明しています。研究者たちは、Stable DiffusionとCLIPを利用し、EEG信号からのセマンティック表現を生成し、最終的にはそれを用いて関連する画像を生成する新しい手法を開発しました。
Takeaways
- 🧠 DreamDiffusionは、脳からのEEG信号を入力として受け取り、それに対応する高品質の画像を生成する新しい方法です。
- 💡 この技術は、テキストプロンプトを使用する従来のテキストから画像への生成モデル(例:Imagen、DALL-E 2、StableDiffusion)を一歩先に進めます。
- 🌌 夢を視覚化するだけでなく、言語障害のある人々が自己表現を助ける可能性があります。
- 🔍 EEG(脳波)信号は、頭皮に配置された電極を介して測定される人間の脳の電気活動の記録です。これは非侵襲的であり、高価な機器を必要としません。
- 🤖 研究者は、EEG信号からの埋め込み(エンベディング)を生成するためにエンコーダーを導入し、それをStable Diffusionに供給して画像を生成することを提案しました。
- 🚀 EEG信号はノイズが多く、高いバリアンスを持つため、高品質のエンベディングを生成するエンコーダーの作成は簡単ではありません。
- 🔧 研究者は、CLIPのテキストと画像の埋め込みに関連しない新しいエンコーダーからの埋め込みに直面した課題に取り組みました。
- 📊 大量のラベルなしEEGデータを使用してEEG信号エンコーダーをトレーニングする「マスクされた信号の事前トレーニング」という方法を提案しました。
- 💼 EEG埋め込みをCLIP埋め込みに似せるために、EEG-画像ペアの小さなデータセットを使用して、EEGエンコーダーとStable Diffusionを微調整しました。
- 🎨 DreamDiffusionは、同じEEG信号に対して印象的な画像サンプルを生成する能力を示しました。
- 📢 この技術は、将来、直感的な画像生成や非言語的コミュニケーションの新たな方法を提供する可能性があります。
Q & A
DreamDiffusionとは何ですか?
-DreamDiffusionは、脳から記録されたEEG(脳波)信号を入力として受け取り、それに合った高品質の画像を生成する新しい手法です。
DreamDiffusionが使用する信号の種類は何ですか?
-DreamDiffusionはEEG(脳波)信号を使用します。これは、脳の電気活動を記録したものです。
DreamDiffusionの目的は何ですか?
-DreamDiffusionの目的は、言語障害のある人が自己表現できるようにすることや、睡眠中の脳の活動を視覚化して夢を画像として捉えることなど、新しいコミュニケーション手段としての可能性を探ることです。
なぜEEG信号が選ばれたのですか?
-EEG信号は非侵襲的であり、高価な機器を必要とせず、容易にアクセスできるため、より高い利用可能性を持つモデルを実現するために選ばれました。
DreamDiffusionはどのようにしてEEG信号から画像を生成しますか?
-DreamDiffusionは、EEG信号からの埋め込み(エンコーディング)を生成するエンコーダーと、その埋め込みを使用して画像を生成するStable Diffusionを利用しています。
DreamDiffusion開発における主な課題は何ですか?
-主な課題は、EEG信号のノイズと高いバリアンスを処理すること、およびEEG信号からの埋め込みがCLIPのテキストと画像の埋め込みと異なる埋め込み空間にあることです。
研究者はこれらの課題をどのように克服しましたか?
-研究者は、大量のラベルなしEEGデータを使用してEEG信号エンコーダーを訓練する「マスクされた信号の事前学習」を採用し、EEGと画像のペアの小さなデータセットを使用してEEGエンコーダーとStable Diffusionを微調整しました。
DreamDiffusionの結果の例はどのようなものですか?
-データセット内のEEG信号に対応する実際の画像の左側に、同じEEG信号のDreamDiffusionによって生成された3つのサンプル画像が非常に印象的に表示されます。
DreamDiffusionはどのような用途が考えられますか?
-DreamDiffusionは、夢の視覚化、言語障害がある人の自己表現支援、創造性の向上など、さまざまな用途が考えられます。
DreamDiffusionを使用するにはどのような機器が必要ですか?
-EEG信号を取得するためのポータブルな商業製品を含む、脳波を記録するための機器が必要です。
Outlines
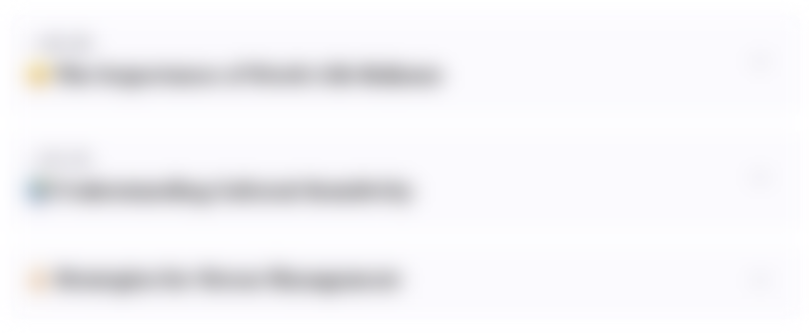
This section is available to paid users only. Please upgrade to access this part.
Upgrade NowMindmap
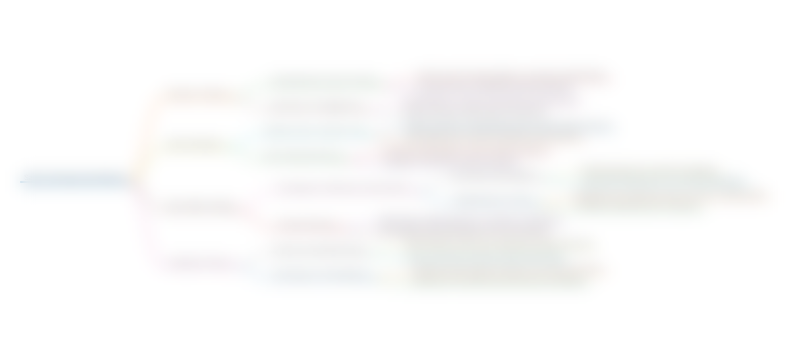
This section is available to paid users only. Please upgrade to access this part.
Upgrade NowKeywords
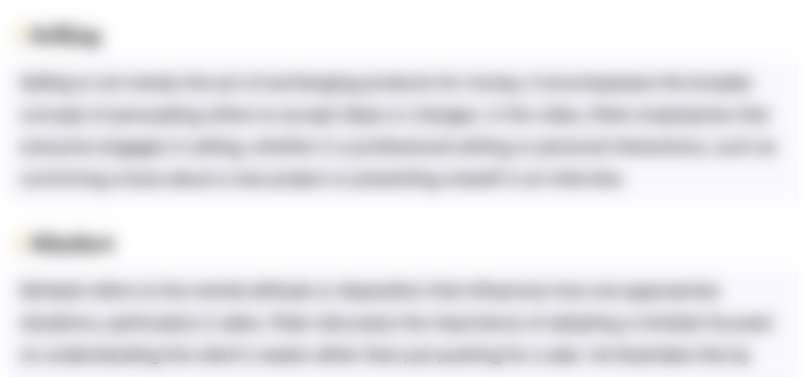
This section is available to paid users only. Please upgrade to access this part.
Upgrade NowHighlights
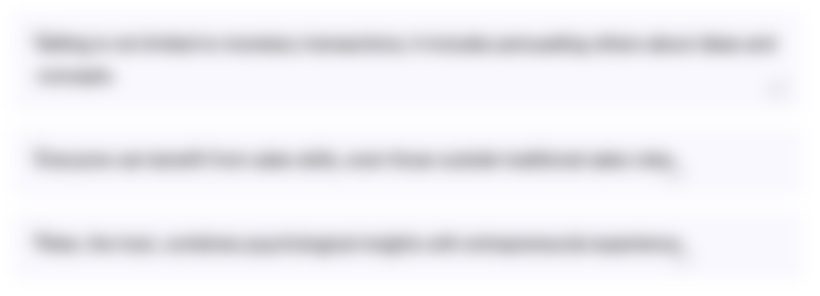
This section is available to paid users only. Please upgrade to access this part.
Upgrade NowTranscripts
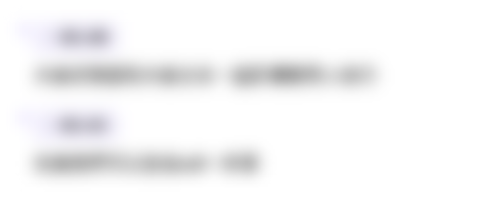
This section is available to paid users only. Please upgrade to access this part.
Upgrade NowBrowse More Related Video

Tile-Based Map Generation using Wave Function Collapse in 'Caves of Qud'
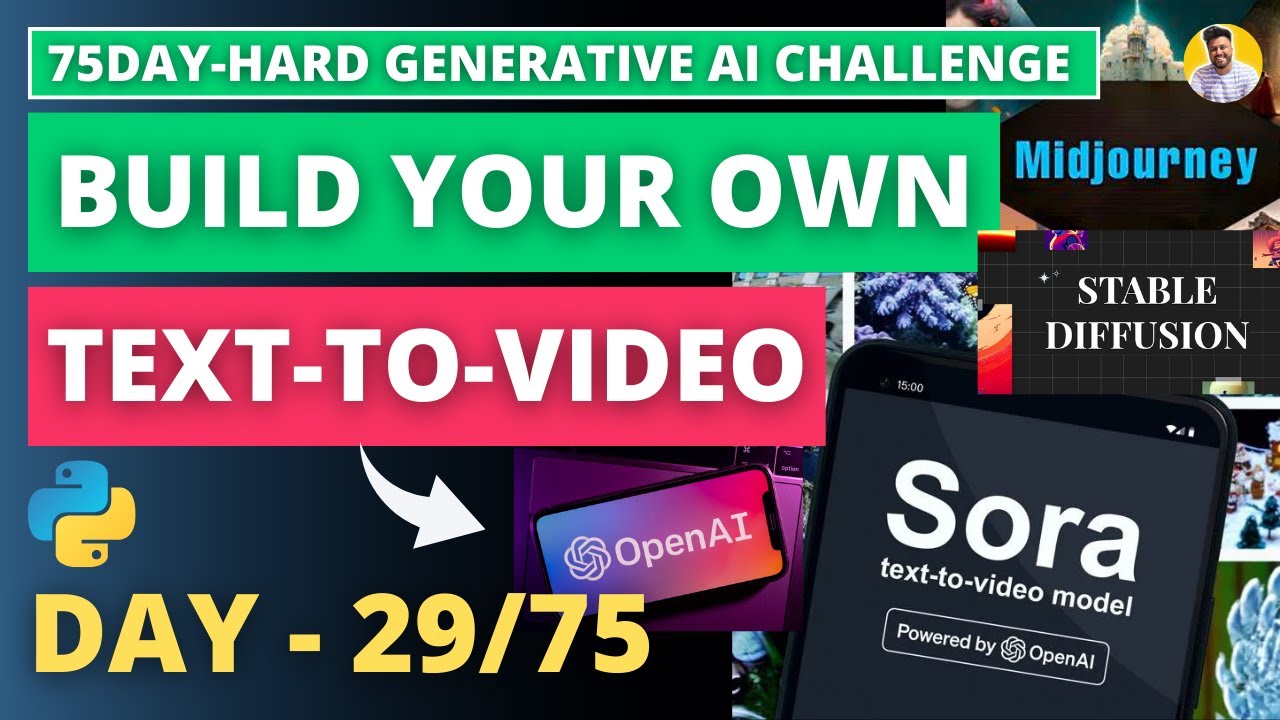
Day 29/75 Build Text-to-Video AI with LLM [Explained] OpenAI SORA Stable Diffusion VideoPoet Runway

【無料で使える動画生成AI】Stable Video Diffusionってどうなの?PikaやGen-2と比較してみた
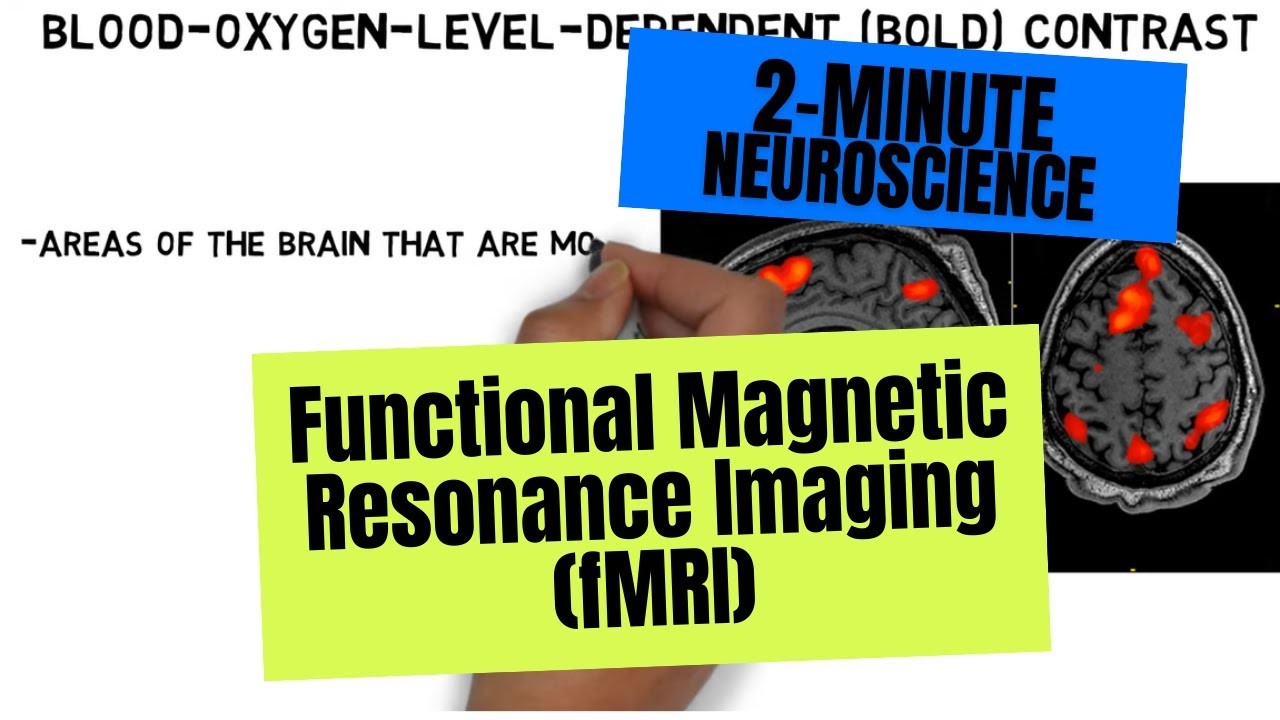
2-Minute Neuroscience: Functional Magnetic Resonance Imaging (fMRI)
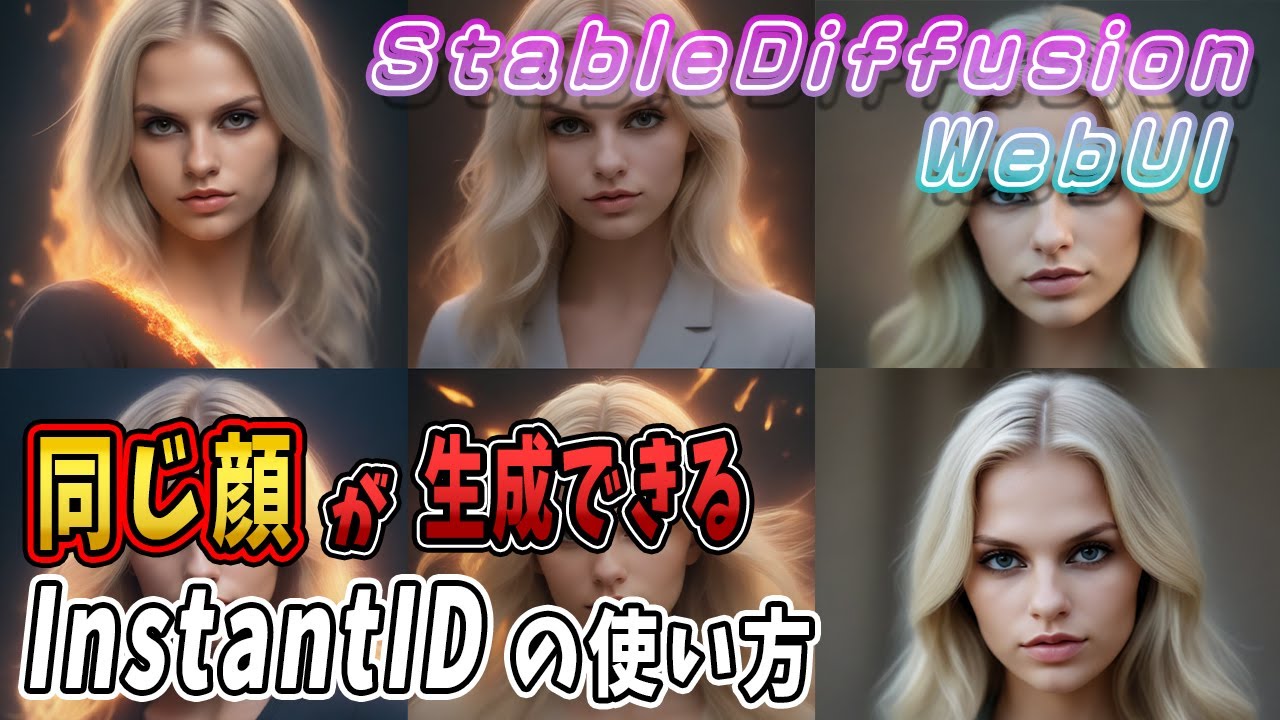
Instant IDでLoRAが不要になる?【Stable Diffusion WebUIでInstant IDを使い同じ顔の人物を生成する方法】
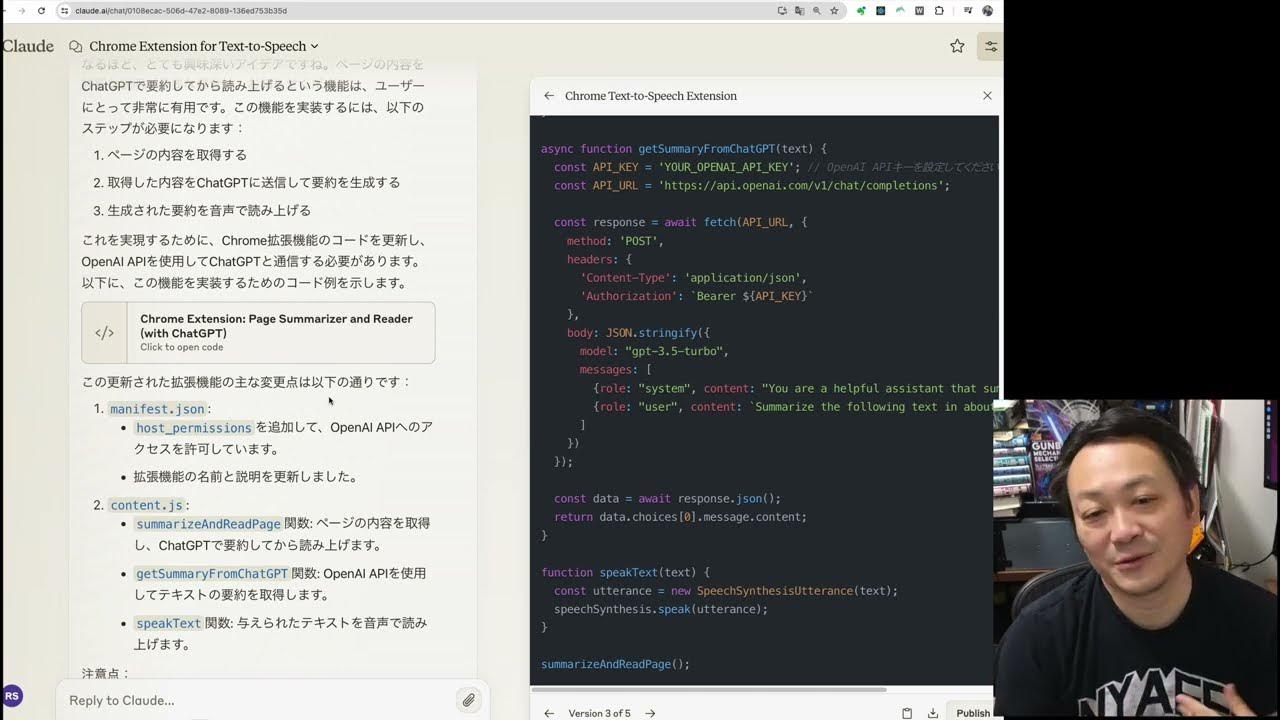
ハッカー魂 超絶便利なWeb要約読み上げツール
5.0 / 5 (0 votes)