How Microsoft Copilot for Microsoft 365 works
Summary
TLDRMicrosoft 365 Copilot nutzt große Sprachmodelle, um die Arbeitsweise zu revolutionieren. Es interagiert mit Organisationsdaten und kann in Word vollständige Dokumente, in Outlook E-Mail-Antworten, in PowerPoint Präsentationen und in Teams Besprechungszusammenfassungen erstellen. Es beruht auf öffentlichen Daten und respektiert Datenschutz und Zugriffsrechte. Die Integration in Microsoft 365-Apps ermöglicht eine effiziente und sichere Zusammenarbeit.
Takeaways
- 🤖 Microsoft 365 Copilot ist ein Werkzeug, das durch die Nutzung großer Sprachmodelle die Arbeitsweise transformiert.
- 📝 In Word kann Copilot mithilfe vorhandener Dateien eine vollständige Geschäftsvorlage erstellen.
- 💌 In Outlook kann Copilot basierend auf ausgewähltem Inhalt automatisch E-Mail-Antworten verfassen.
- 📑 In PowerPoint kann man mit einem Klick aus geschriebenem Inhalt eine ansprechende Präsentation erstellen.
- 📋 In Teams kann Copilot Besprechungszusammenfassungen mit erörterten Maßnahmen generieren.
- 🗂 Mit Business Chat in Microsoft Teams kann man sich auf etwas aufholen, das man möglicherweise verpasst hat, indem es Informationen aus mehreren Quellen zusammenfasst.
- 🧠 Große Sprachmodelle (LLMs) werden mit riesigen Mengen an öffentlichen Daten trainiert, um Sprache, Kontext und Bedeutung zu erlernen.
- 🗣️ Interaktionen mit LLMs erfolgen über sogenannte Prompts, die typischerweise eine Aussage oder Frage darstellen.
- 🔒 Der Copilot-System respektiert die Zugriffsberechtigungen des Benutzers für jeglichen Inhalt und Graph-Informationen, die es abruft.
- 🔄 Microsoft 365 Copilot verwendet eine eigene Standardaufforderung mit verantwortungsbewussten Interaktionsregeln.
- 🗃️ Copilot kann in Microsoft 365-Apps helfen, Zeit zu sparen, indem es Inhalte generiert, basierend auf den vom Benutzer ausgewählten Dokumenten.
Q & A
Was ist Microsoft 365 Copilot?
-Microsoft 365 Copilot ist ein Werkzeug, das die Arbeitsweise transformiert, indem es große Sprachmodelle nutzt, die mit den Daten Ihrer Organisation interagieren.
Wie kann Copilot in Word verwendet werden?
-In Word kann Copilot einen völlig neuen Dokumenten, wie einen Geschäftsvorschlag, basierend auf Inhalten aus Ihren vorhandenen Dateien, erstellen.
Was kann Copilot in Outlook tun?
-In Outlook kann Copilot basierend auf dem gewählten Inhalt E-Mail-Antworten für Sie verfassen.
Wie transformiert Copilot geschriebenen Inhalt in PowerPoint in Präsentationen?
-Mit einem Klick auf eine Schaltfläche kann Copilot in PowerPoint geschriebenen Inhalt in eine visuell ansprechende Präsentation verwandeln.
Was kann Copilot in Teams leisten?
-In Teams kann Copilot Besprechungszusammenfassungen erstellen, in denen auch die besprochenen Maßnahmen zur Nachbearbeitung aufgeführt sind.
Wie hilft Copilot bei der Nutzung von Business Chat in Microsoft Teams?
-Copilot hilft bei der Nutzung von Business Chat in Microsoft Teams, indem er Informationen aus mehreren Quellen zusammenführt, um Sie auf dem Laufenden zu halten, auch wenn Sie etwas verpasst haben.
Woher erhalten große Sprachmodelle ihr Wissen?
-Große Sprachmodelle werden auf riesigen Mengen an öffentlich zugänglichen Daten trainiert, wie Büchern, Artikeln und Websites, um Sprache, Kontext und Bedeutung zu lernen.
Was ist ein Prompt und wie wird er verwendet?
-Ein Prompt ist normalerweise eine Aussage oder Frage, die Sie an das Sprachmodell richten. Das Modell generiert eine Antwort basierend auf seiner Schulung und dem Verständnis des Kontextes, den es aus der Art und Weise Ihres Prompts erhält.
Wie wird die Privatsphäre geschützt und wie werden Daten sicher gehalten, wenn Copilot verwendet wird?
-Copilot respektiert die Zugriffsberechtigungen pro Benutzer auf jeglichen Inhalt und Graph-Informationen, die es abruft. Dies ist wichtig, weil Copilot nur basierend auf Informationen generiert, auf die Sie ausdrücklich Zugriff haben.
Wie funktioniert der Orchestrierungsprozess von Copilot?
-Der Copilot-Orchestrierungsprozess kombiniert den Standardprompt mit Ihrem Prompt, der zusätzlichen Informationen, die es gesammelt hat, um einen langen Prompt zu bilden, den er an das Sprachmodell sendet, um eine Antwort zu generieren.
Wie hilft Copilot dabei, Zeit zu sparen, indem er Inhalte in den Apps generiert, die Sie verwendet?
-Copilot kann beispielsweise in Word einen Entwurf eines Vorschlags erstellen, indem er den Inhalt verwendet, an dem Sie gearbeitet haben, und relevante Informationen aus zusätzlichen Dokumenten, auf die Sie Zugriff haben, hinzufügt.
Outlines
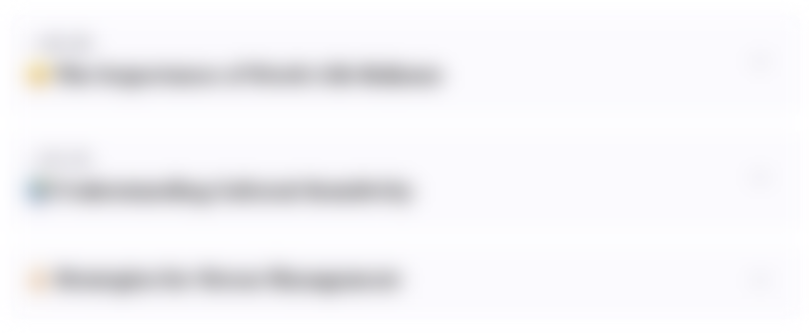
This section is available to paid users only. Please upgrade to access this part.
Upgrade NowMindmap
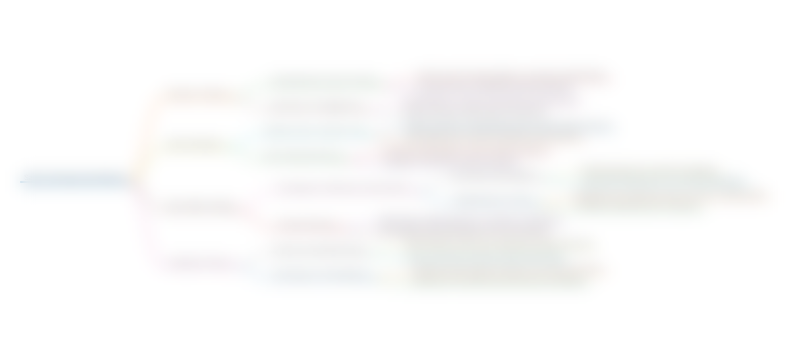
This section is available to paid users only. Please upgrade to access this part.
Upgrade NowKeywords
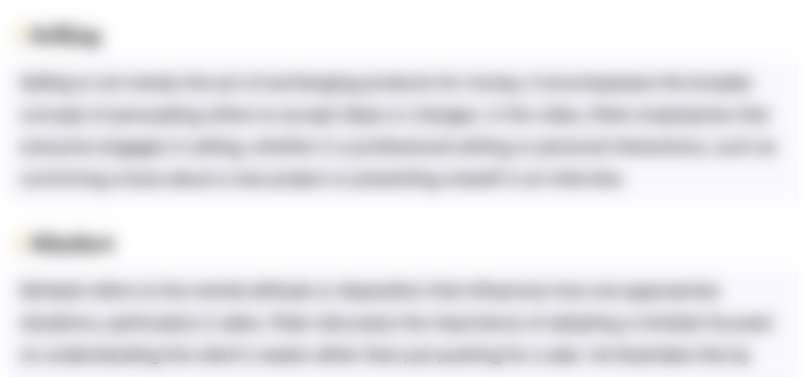
This section is available to paid users only. Please upgrade to access this part.
Upgrade NowHighlights
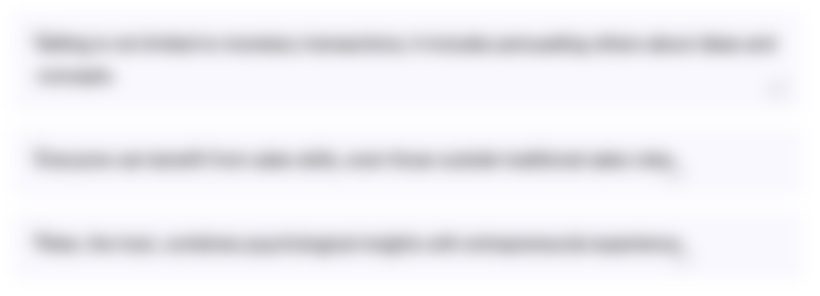
This section is available to paid users only. Please upgrade to access this part.
Upgrade NowTranscripts
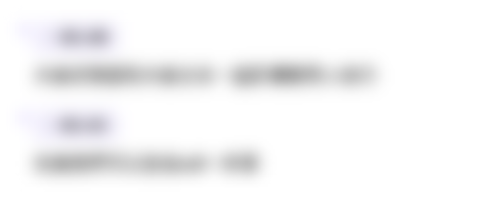
This section is available to paid users only. Please upgrade to access this part.
Upgrade NowBrowse More Related Video
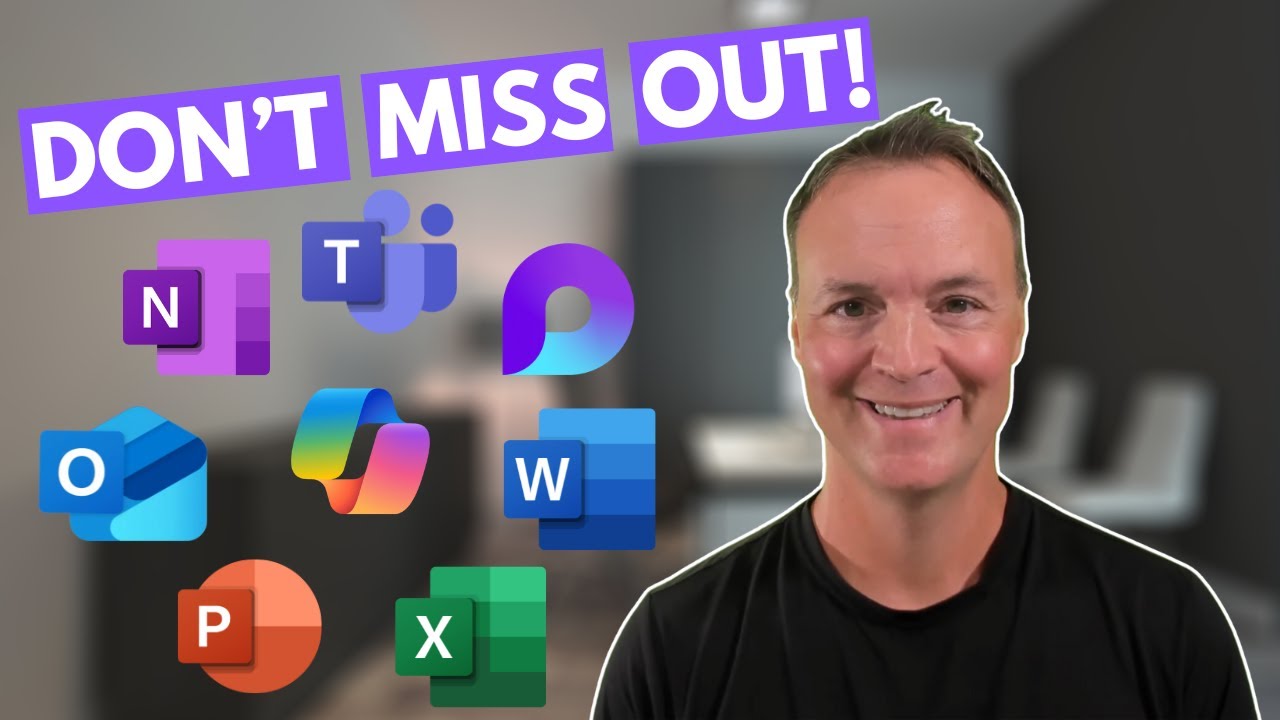
Don't Miss Out on Microsoft 365 Copilot Features
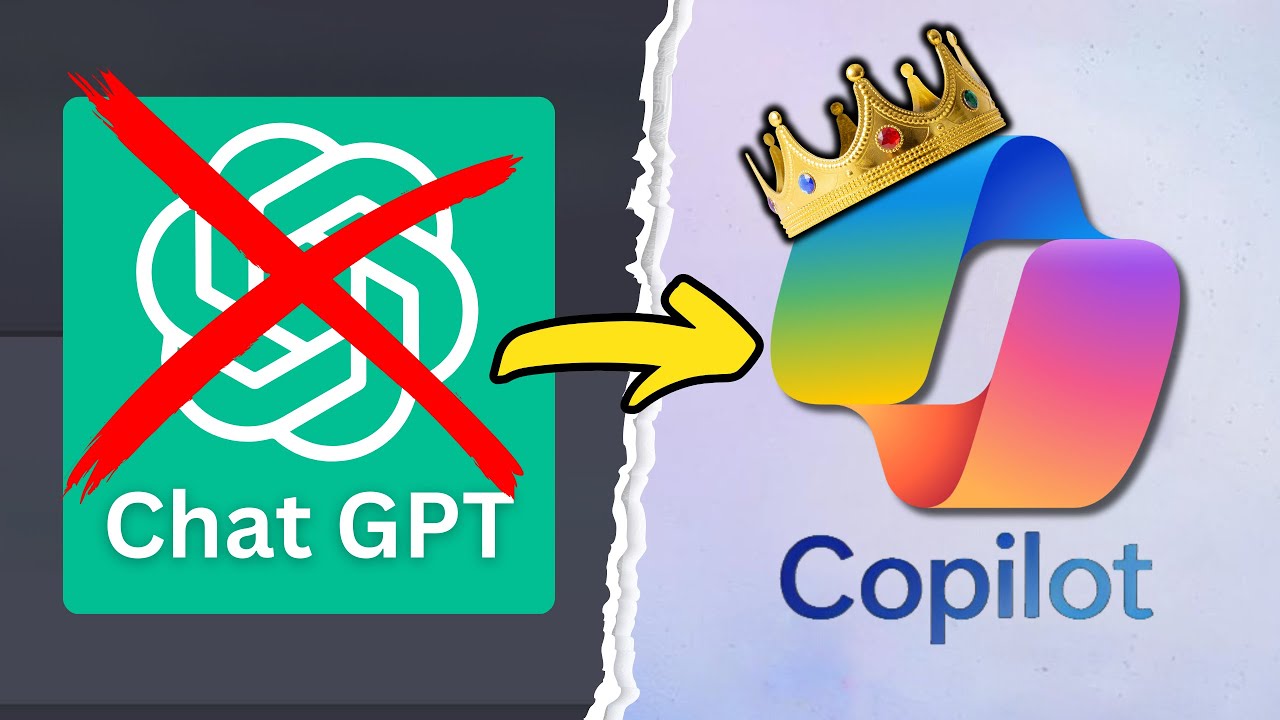
Besser als ChatGPT? - Microsoft Copilot Pro im Test
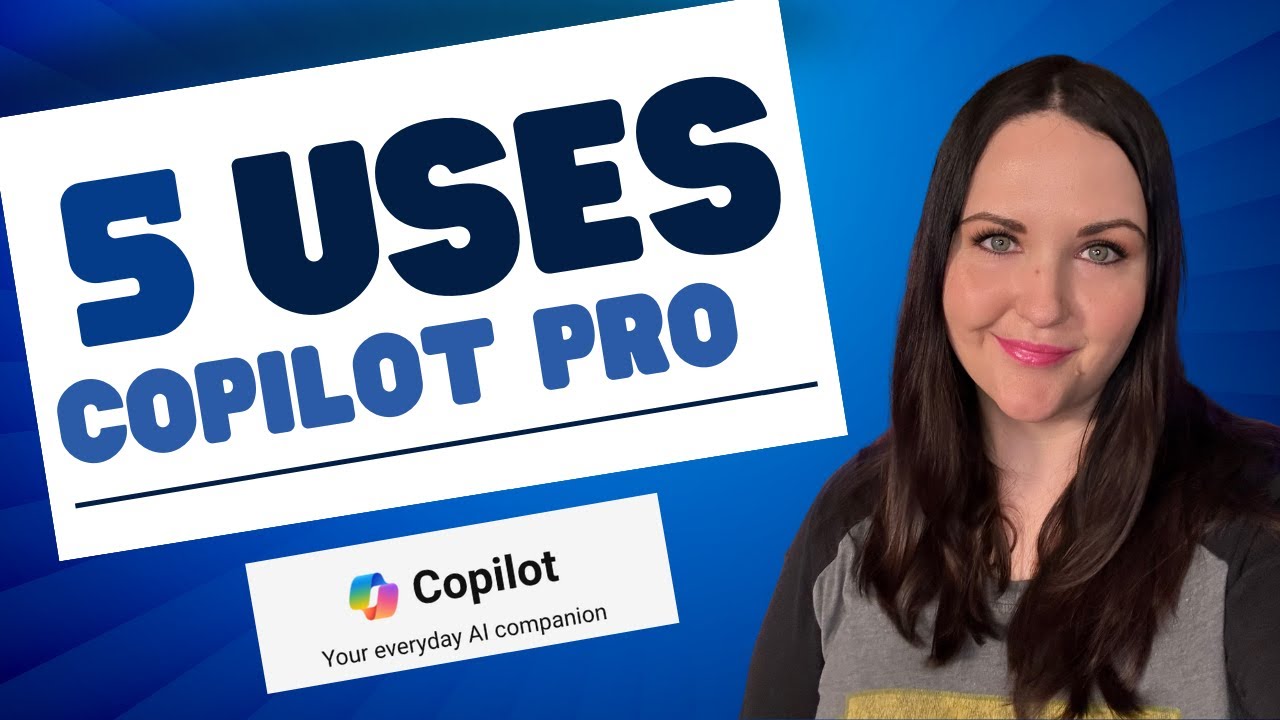
The Top 5 Super Powers of Copilot Pro
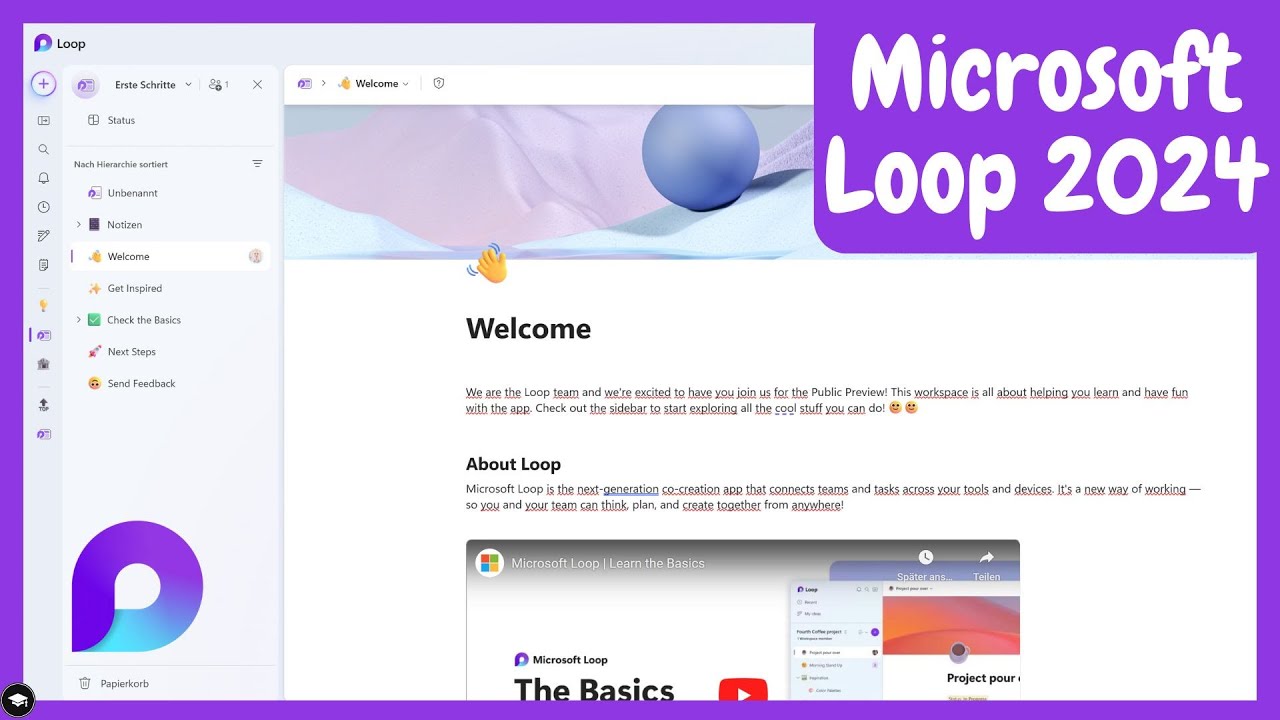
So nutzt du Microsoft Loop – ein Überblick der Funktionen und Möglichkeiten
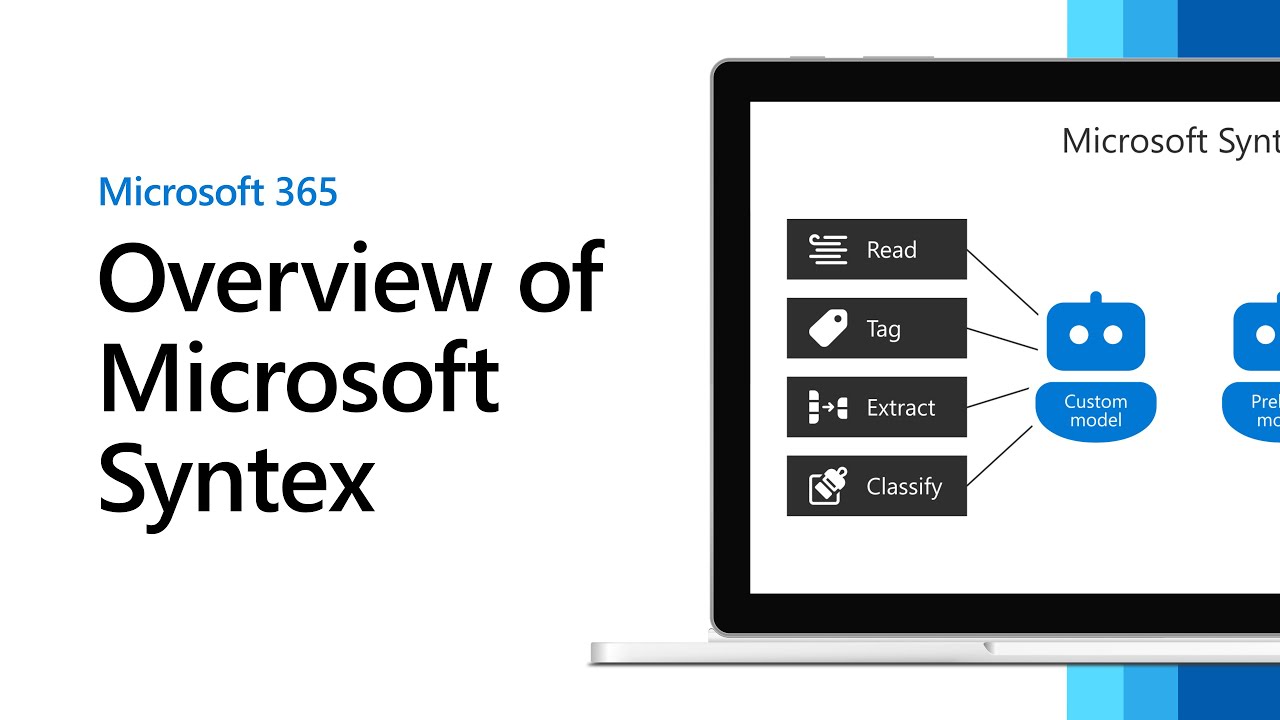
Overview of Microsoft Syntex
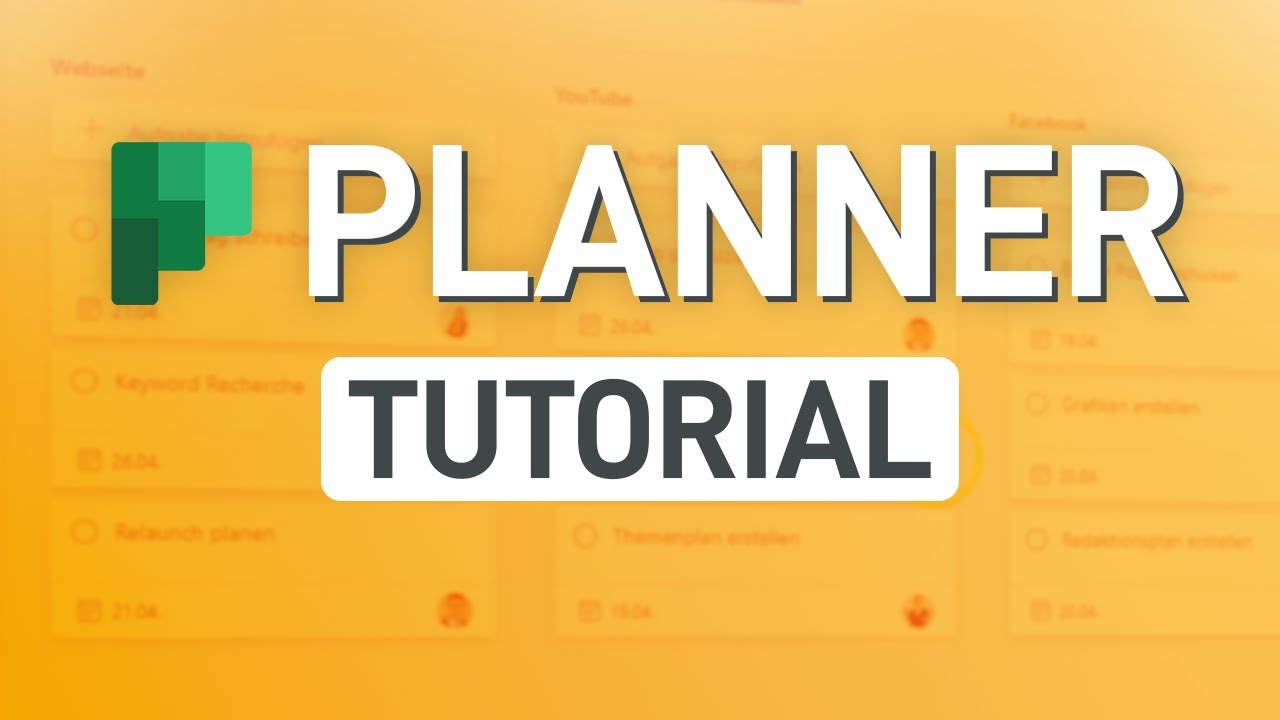
✅ Microsoft Planner Tutorial (alle wichtigen Funktionen erklärt!)
5.0 / 5 (0 votes)