The implications of AI on a Center of Excellence
Summary
TLDRThe video script delves into the rapid rise of AI, especially ChatGPT, and its potential to revolutionize various industries. It emphasizes the importance of prompt engineering, data quality, and architectural considerations for organizations to harness AI's full potential. The speaker uses analogies to illustrate the early stages of AI development and warns against underestimating its future capabilities. The script also highlights the need for enhanced skills, such as prompt engineering, data governance, and business analysis, to leverage AI effectively. It encourages establishing centers of excellence to foster innovation, collaboration, and best practices within organizations, enabling them to identify AI's sweet spots and maximize its benefits.
Takeaways
- π GPT (Generative Pre-trained Transformer) models like ChatGPT have brought AI capabilities to the forefront, generating human-like text based on prompts.
- π We are still in the early stages of AI capabilities, like driving a Formula One car compared to a regular car.
- π§Ύ Prompts need to be more explicit and detailed to get better results from GPT models. Prompt engineering is a new skill to develop.
- π§© GPT models excel at solving puzzles and reasoning based on provided information, rather than using general knowledge.
- π¨βπ» AI highlights the importance of skills we should already have, like good business analysis, data quality, and documentation standards.
- π΅οΈββοΈ AI forces us to be more disciplined in areas like governance, change control, and metadata management due to added risks.
- π©βπ« AI creates a need for new skills like prompt engineering, but also emphasizes improving existing skills like data governance and architecture.
- π AI can be a catalyst for getting buy-in from executives to fund projects and improve areas we've neglected in the past.
- π Centers of Excellence (CoEs) should act as innovation hubs, bringing together people passionate about AI and exploring its use cases.
- π― Organizations should focus on finding the sweet spots where AI can provide significant ROI, easy adoption, and consistent results.
Q & A
What is the main topic discussed in the transcript?
-The main topic discussed is the impact of AI and specifically GPT (Generative Pre-trained Transformer) on businesses, and the skills and processes organizations need to adopt to leverage AI effectively.
Why did ChatGPT gain such rapid adoption with 100 million users in 2 months?
-ChatGPT gained rapid adoption because it was easy to use (just type in a prompt) and the results were staggeringly good, allowing users to generate text like song lyrics and content that seemed beyond current capabilities.
What analogy is used to describe the current stage of GPT development?
-The analogy of a car is used. ChatGPT is like a high-performance Porsche GT3 on a track, which gives a glimpse of the capabilities of a Formula 1 car, but doesn't represent the full potential of AI, which is still in its very early stages like a flip phone compared to an iPhone.
What are some key skills organizations need to develop to leverage GPT effectively?
-Organizations need to develop skills in prompt engineering (crafting effective prompts), data quality, business analysis, following industry standards for documentation, understanding system architecture, data governance, and change management.
Why is prompt engineering important for GPT?
-Prompt engineering is important because longer, more explicit prompts are needed to get better results from GPT. Prompts can be thought of as code that needs to be monitored, refined, and managed for dependencies, just like traditional code.
What are some use cases where GPT excels?
-GPT is good at solving puzzles and generating text when given all the puzzle pieces (context and data). It performs well in areas like generating code, interpreting legal documents, and writing lyrics because it has ingested large amounts of relevant data.
What is the role of a Center of Excellence (CoE) in the context of AI adoption?
-A CoE acts as an innovation hub, bringing together passionate individuals and teams to collaborate on AI initiatives, find use cases with strong ROI and ease of adoption, and ensure consistent and reliable results.
How does AI highlight the importance of existing best practices?
-AI punishes mediocrity and exposes weaknesses in areas like data quality, business analysis, documentation, and architecture. It forces organizations to adopt disciplines they may have neglected in the past due to the increased risks and potential impact of AI on business operations.
What are some key pillars or skills needed for a strong AI Center of Excellence?
-Key pillars or skills include vision, leadership, governance, change control, methodology, standards, metadata management, architecture, security, change management, project management, tooling, and innovation.
How can AI be used as a catalyst for organizational change?
-AI can be used as a catalyst for organizational change by highlighting the need for improvements in areas like documentation, architecture, and data governance. It provides an opportunity to get buy-in and funding from executives to address these issues, which were previously overlooked or underfunded.
Outlines
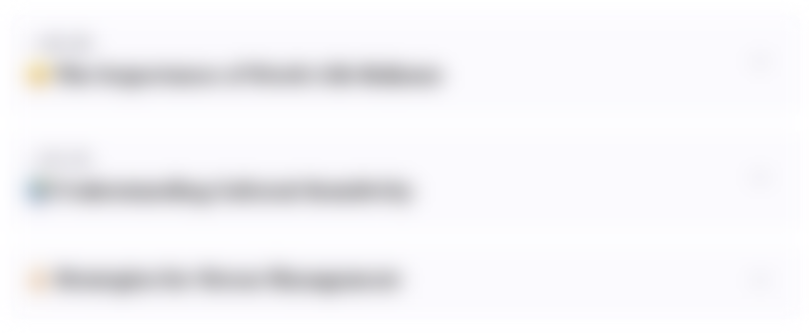
This section is available to paid users only. Please upgrade to access this part.
Upgrade NowMindmap
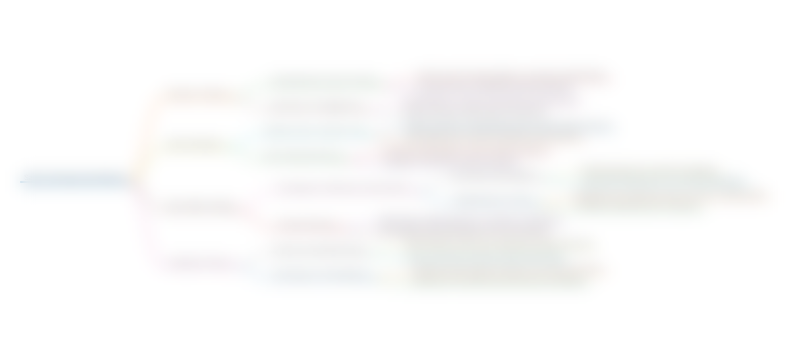
This section is available to paid users only. Please upgrade to access this part.
Upgrade NowKeywords
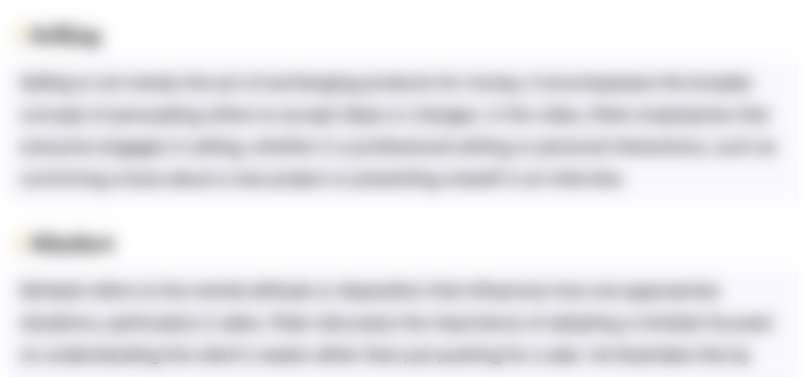
This section is available to paid users only. Please upgrade to access this part.
Upgrade NowHighlights
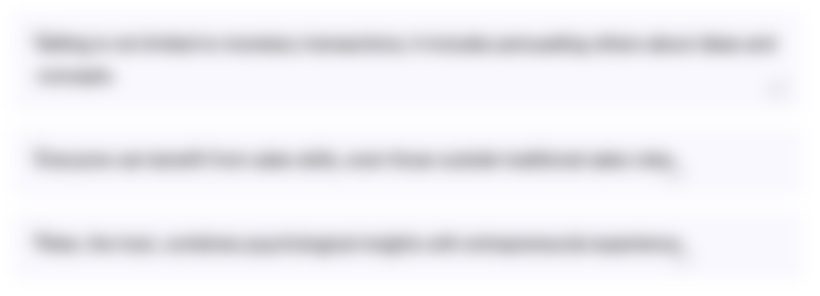
This section is available to paid users only. Please upgrade to access this part.
Upgrade NowTranscripts
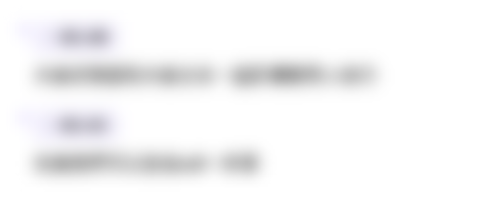
This section is available to paid users only. Please upgrade to access this part.
Upgrade NowBrowse More Related Video

Demystifying AI for your Organization - Amanda Teschko
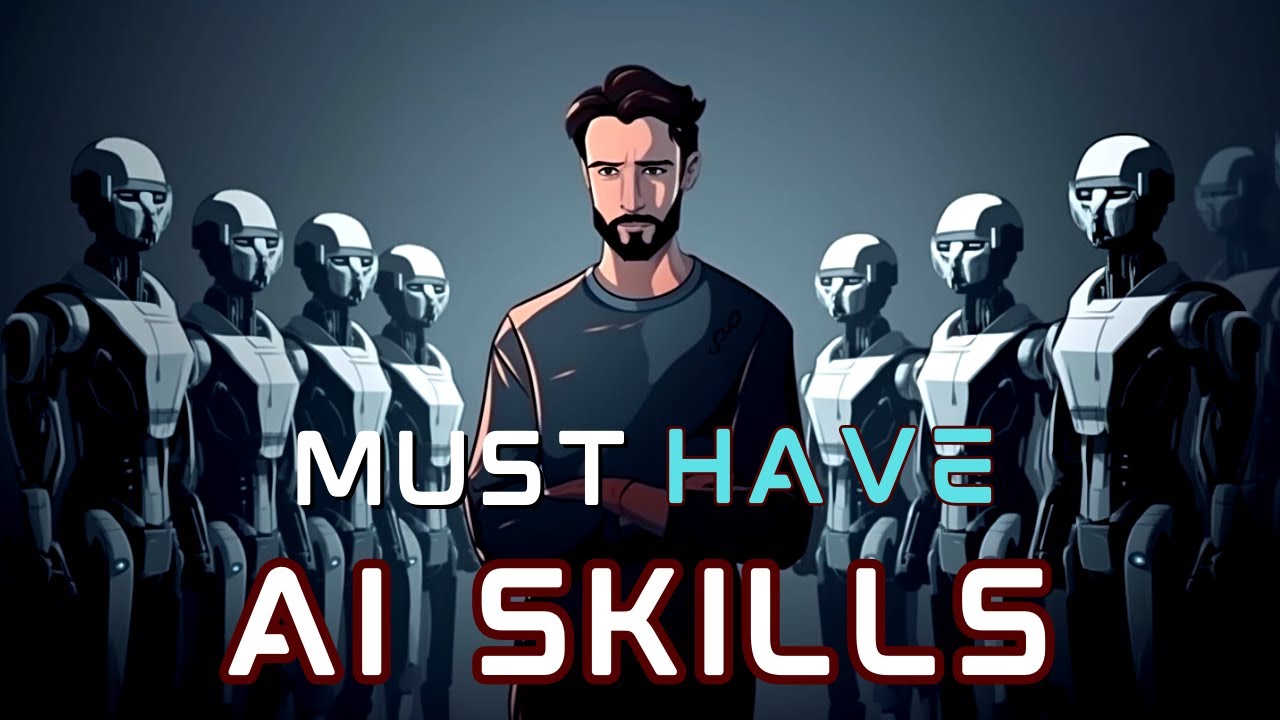
The 9 AI Skills You Need NOW to Stay Ahead of 97% of People
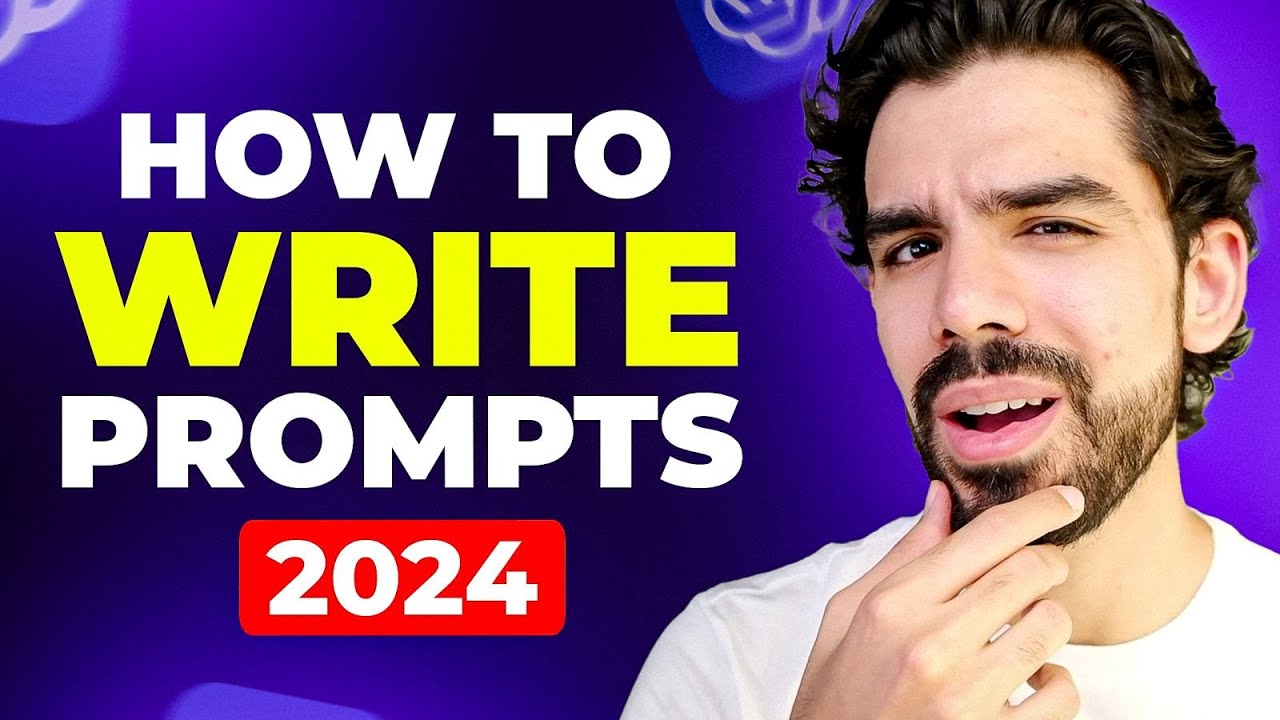
ChatGPT Tutorial - Prompt Engineering Tutorial for Beginners
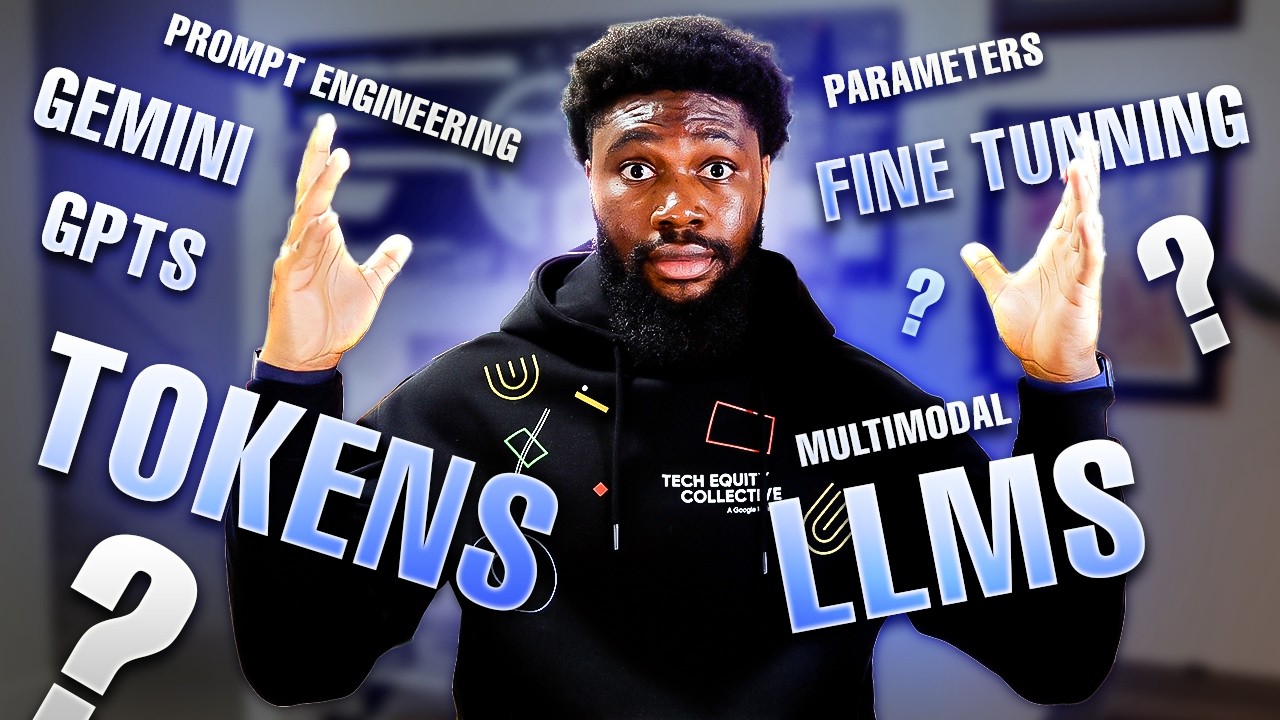
Simplifying Generative AI : Explaining Tokens, Parameters, Context Windows and more.
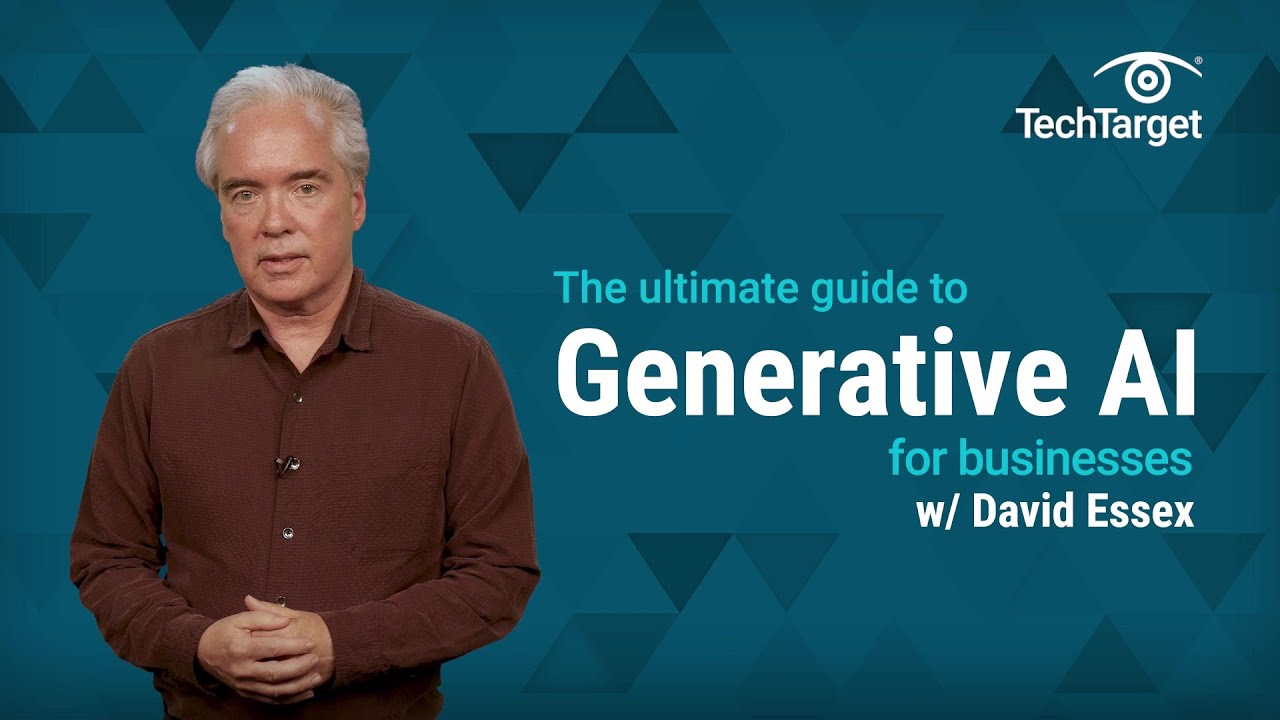
Ultimate Guide to Generative AI for Businesses

Unlock potential of Generative AI by Conquering the Spooky Mountain | Nikhil Bhojwani | TEDxBoston
5.0 / 5 (0 votes)