サッカーの上達にデータを生かす
Summary
TLDRこのスクリプトでは、データがサッカーの向上にどのように役立つかについて語られています。経験や直感に限界があり、客観的なデータの活用が効果的かつ効率的な改善につながると示されています。ゲーム分析、GPSデータ、視覚スキル、目運動解析など、さまざまな研究を通じて、技術の進歩がスポーツにおけるデータ収集を容易にし、それらをトレーニングや戦略の改善に活用する重要性が強調されています。
Takeaways
- 😀 スポーツ界では、経験と直感は重要な要素ですが、経験と直感だけでは限界があり、オブジェクトデータの活用はより効果的かつ効率的な改善につながる。
- 🏆 サッカーの試合分析は、試合でのプレイを改善するために最も重要な活動であり、試合の質的な分析と、GPSや画像処理技術を用いた定量的な分析を行う。
- 📊 GPSトラッカーやビデオカメラを用いて選手やボールの動きデータを自動で取得し、試合の分析に利用することができる。
- 🔍 日本とドイツの攻撃パターンを比較した研究で、攻撃の成功率に差は見られず、攻撃パターンはほぼ同じであることが明らかになった。
- 🎯 ゴールキーパーの守備能力を評価する研究では、ロジスティック回帰分析を用いて、各シュートの難易度と失敗確率を算出した。
- 🏃♂️ GPSデータを用いて選手の持久力や体力を測定し、トレーニング計画を作成することができる。
- 🤔 サッカー選手の視覚スキルを評価する研究では、脳波、筋電位(EMG)、反応時間データを用いて、状況を評価する速さの違いを発見した。
- 👀 目の動きを測定する研究では、上級者と中級者の選手が異なる視点を重視していることが明らかになり、視覚スキルのトレーニングが必要であることが示された。
- 📈 技術の発展により、より多様な情報を収集することが容易になり、スポーツ活動でのデータの活用が重要になる。
- 💭 経験と直感を大切にしつつ、定量的なデータを効果的に適用する理解が必要であり、スポーツの向上にはデータの収集と分析が不可欠である。
Q & A
中谷先生はどのような研究センターに所属していますか?
-中谷先生は筑波大学サッカーコーチング研究センターに所属しています。
スポーツにおける経験と直感にはどのような限界がありますか?
-経験と直感はスポーツの進歩に重要な要因ですが、それだけでは限界があり、客観的なデータをうまく活用することでより効果的かつ効率的な改善が可能になります。
サッカーの試合分析において何を分析する予定ですか?
-試合分析では、どのようなプレイがうまくいくか、どのようなプレイが問題を引き起こすか、特定の状況がなぜ起こるのか、それらを改善するために何ができるかを分析します。
GPSや画像処理技術を利用して得られるデータをどのような分析に使いますか?
-GPSや画像処理技術を利用することで、選手やボールの動きに関する位置関連データや動きに関する情報を自動的に取得し、ゲーム分析に活用します。
日本とドイツの攻撃パターンの違いはどのように調査されましたか?
-攻撃シーンを3つのパターンに分類し、それぞれの攻撃でペナルティエリアへの侵入やシュートの有無、シュートの成功率などを記録して分析しました。
ゴールキーパーの守備能力を評価する指標は何ですか?
-ゴールキーパーの守備能力を評価する指標には、平均ゴール数やシュート成功率などが含まれますが、各シュートの難易度を考慮する必要があります。
ロジスティック回帰分析とはどのような分析手法ですか?
-ロジスティック回帰分析は、データから失敗する可能性や難易度を予測するための統計技術です。各シュートの成功や失敗、それに関連する要因を記録し、これらのデータを分析して回帰方程式を作成します。
GPSデータを使用して選手の持久力や体力を測定する方法はどのようなものですか?
-選手がGPSトラッカーを装着することで、心拍数や総移動距離、高強度の運動時間などを自動的に取得し、トレーニング計画を作成することができます。
視覚スキルの違いはどのように調査されましたか?
-視覚スキルの違いを調査するために、脳波データ、筋電位(EMG)データ、反応時間データを取得し、サッカーのパスシナリオに基づく選択反応タスクを行いました。
目線のデータはどのようにして分析されますか?
-目線のデータは、目線追跡システムを装着して取得し、視線が向いている場所や視線の動きを分析することで、選手が実際に何を見ているかを客観的に把握します。
技術の進歩により収集できる情報が増えたことの利点は何ですか?
-技術の進歩により、スポーツ活動や生理的反応に関する多様な情報を収集することが容易になり、それらの情報を運動場面に活用するためのアイデアや意見が重要になります。
Outlines
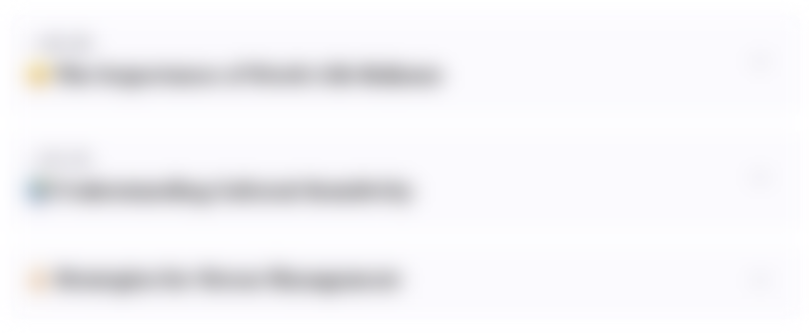
This section is available to paid users only. Please upgrade to access this part.
Upgrade NowMindmap
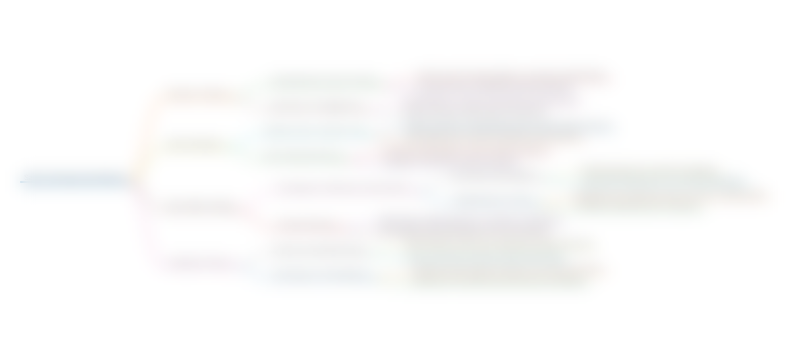
This section is available to paid users only. Please upgrade to access this part.
Upgrade NowKeywords
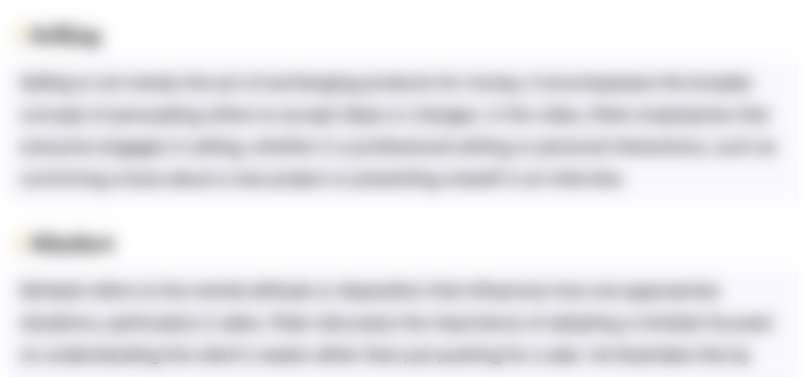
This section is available to paid users only. Please upgrade to access this part.
Upgrade NowHighlights
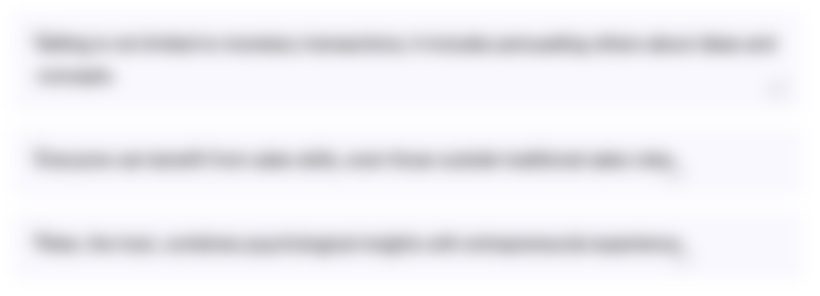
This section is available to paid users only. Please upgrade to access this part.
Upgrade NowTranscripts
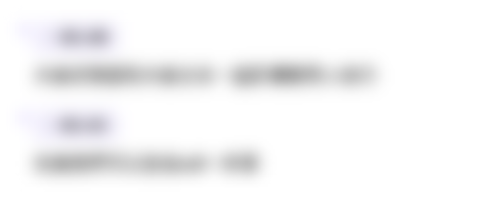
This section is available to paid users only. Please upgrade to access this part.
Upgrade NowBrowse More Related Video

STFC - Officer Tool & Spocks Club just got Better!! They Talk to the Game now!!!!!!!
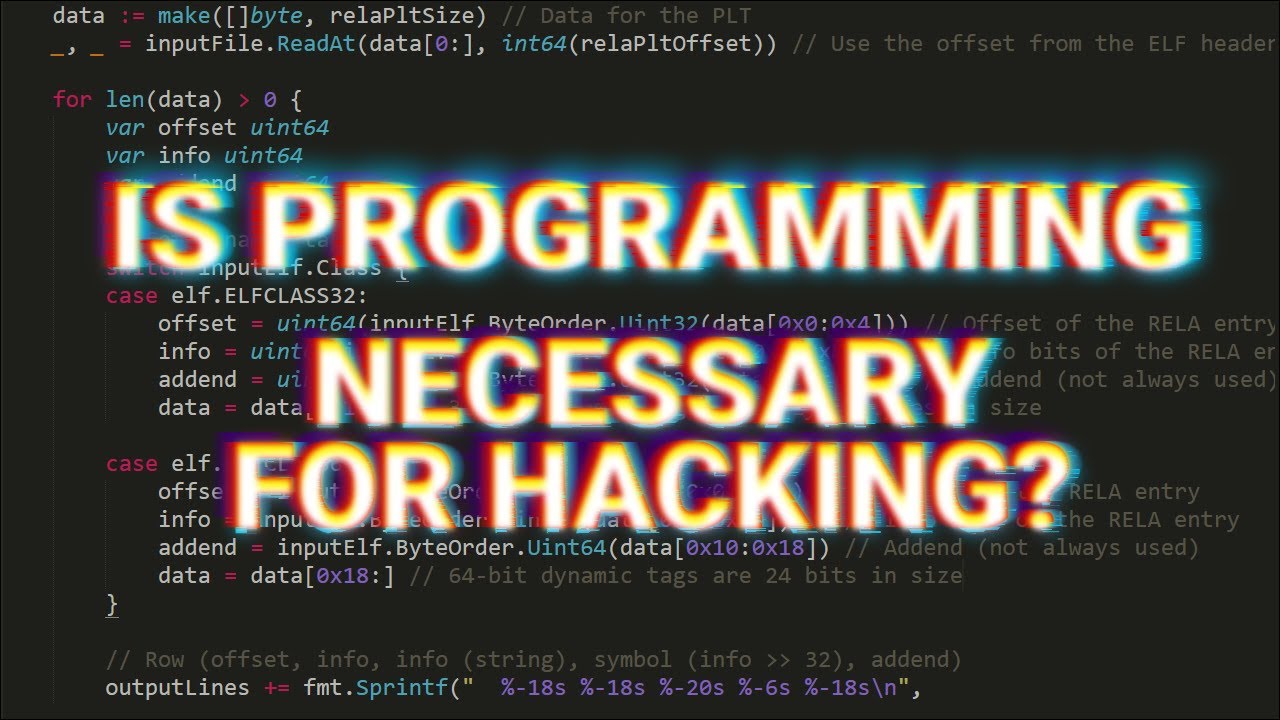
Is Programming Necessary to be a Successful Hacker?

【圧倒的に差がつく】現役プロコーチが伝授!質問力の磨き方
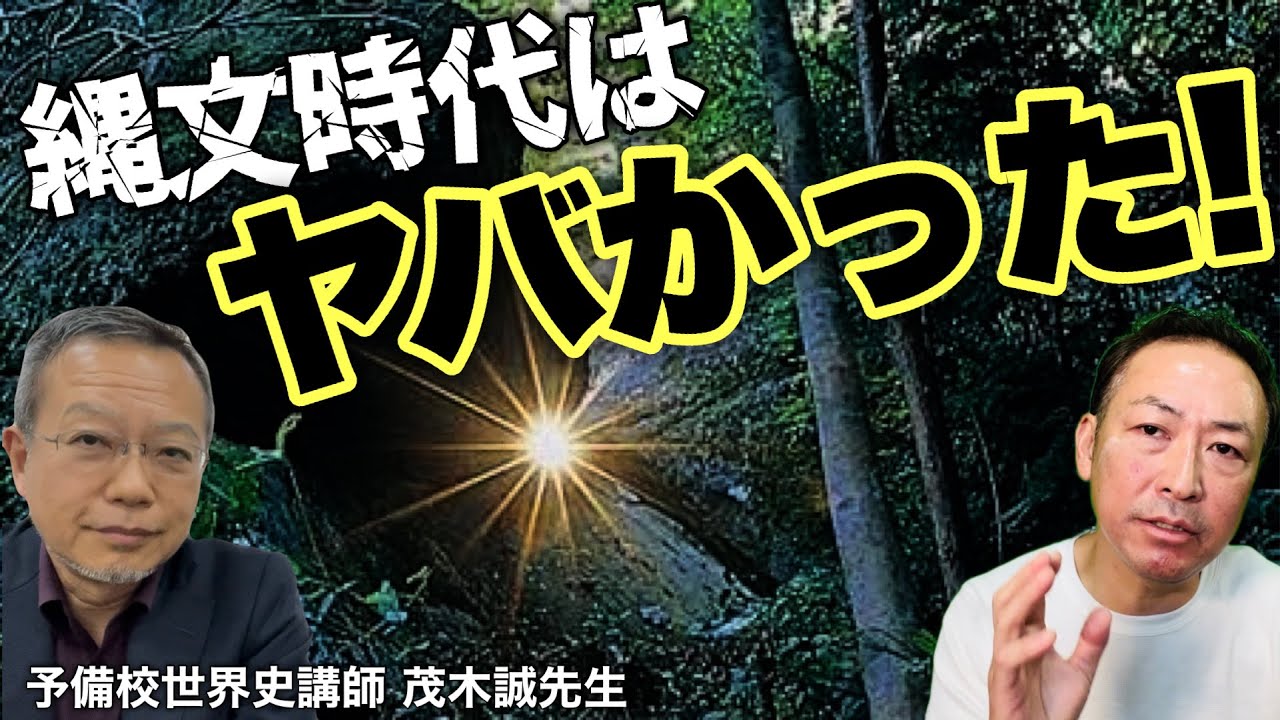
【日本のルーツ】縄文時代はヤバかった! 今こそ知るべき縄文人の能力と神社の関係性(茂木誠×石田和靖)@maomao96363
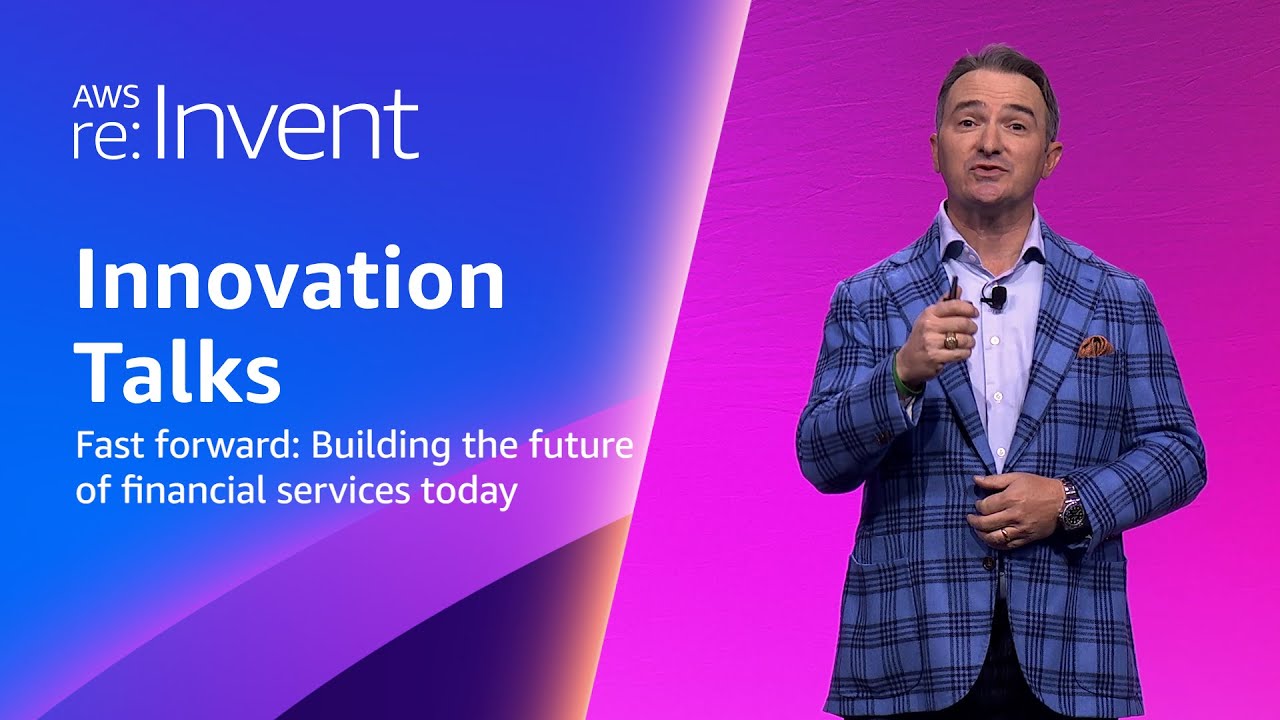
AWS re:Invent 2023 - Fast forward: Building the future of financial services today (FSI203)
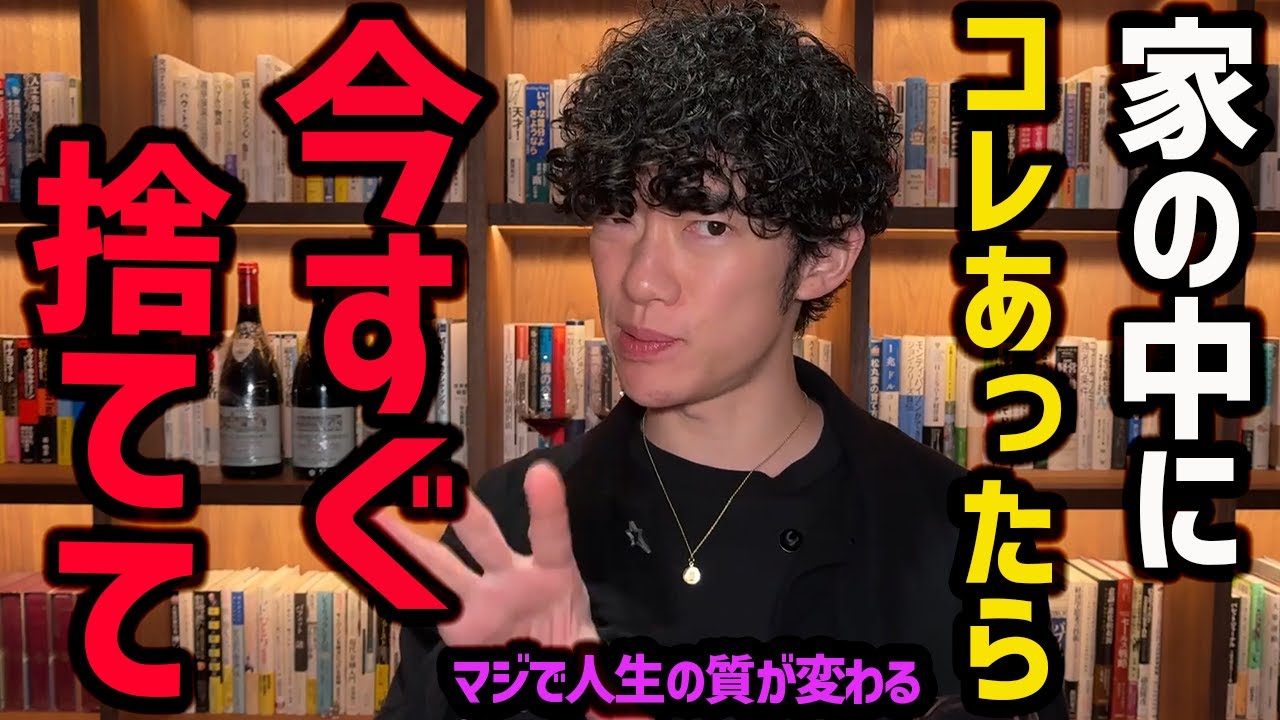
99%あなたの家にもある【真っ先に捨てるべきもの】
5.0 / 5 (0 votes)