Googles GEMINI 1.5 Just Surprised EVERYONE! (GPT-4 Beaten Again) Finally RELEASED!
Summary
TLDR谷歌最新发布的Gemini 1.5模型引发了广泛关注,其强大的处理能力令人惊叹。这一迭代模型能够处理长达3小时的视频、22小时的音频,以及高达700万词或1000万标记的数据,准确率高达99至100%。Gemini 1.5在文本、视觉和音频处理方面均有显著提升,尤其在长文本和多模态任务上展现出了卓越的理解和分析能力。通过分析长达432页的阿波罗11号任务记录、处理大量编程代码,以及理解和分析电影场景等示例,Gemini 1.5证明了其对大数据量和复杂情境的高效处理能力。这一模型的推出不仅展示了谷歌在人工智能领域的领先地位,也为未来的AI应用开辟了新的可能性。
Takeaways
- 😮 谷歌推出了革命性的Gemini 1.5模型,可以处理最长3小时的视频、22小时的音频和700万个单词。
- 🤩 Gemini 1.5在文本、视觉和音频方面均表现出色,其准确率达到99%-100%,远远超过现有的大多数模型。
- 🧠 Gemini 1.5能够通过简单的图片或文字描述,准确理解并找到视频或文本中的特定内容。
- 💪 Gemini 1.5的上下文窗口长达100万个令牌,可以极大扩展AI系统的应用范围。
- 💻 Gemini 1.5展示了惊人的能力,可以浏览数百万行的代码,并根据用户的需求修改和改进代码。
- 🎥 Gemini 1.5还能够处理数小时的视频内容,并精确找到某一帧上的关键信息。
- 🌍 Gemini 1.5可以像人类一样利用词典和语法书进行英语到卡曼语的准确翻译。
- 📊 Gemini 1.5在数学、科学、编码和指令遵循等多个基准测试中表现优异,大幅领先现有模型。
- 🏭 谷歌使用了大量的TPU加速计算资源来训练Gemini 1.5,使其能够处理多模态和多语言数据。
- 🤯 Gemini 1.5的推出将重塑AI格局,给其他公司施加了巨大的竞争压力。
Q & A
谷歌最新发布的是什么模型?
-谷歌发布了新的Gemini模型家族的1.5版本。
Gemini 1.5模型的最大context长度能达到多少?
-Gemini 1.5模型最大能够处理3小时的视频、22小时的音频或700万单词(1000万个token)的文本。
Gemini 1.5模型的准确率如何?
-Gemini 1.5模型在处理大规模数据时,准确率可达99%到100%。
Gemini模型家族有几个版本?各自负责什么任务?
-Gemini模型家族有三个版本:Gemini Ultra用于复杂任务,Gemini Pro 1.0和1.5用于长时间或大规模数据任务,Gemini 1.5专门用于更大更繁琐的长期上下文任务。
Gemini 1.5模型在哪些方面表现更好?
-相较于之前的Gemini Pro 1.0版本,Gemini 1.5在文本、视觉和音频处理上都有显著提升。相较于Gemini Ultra,Gemini 1.5在视觉和音频方面表现略好。
文中展示了哪些Gemini 1.5处理大规模数据的实例?
-文中展示了Gemini 1.5处理432页阿波罗11号文字记录、3js代码库以及44分钟巴斯特·基顿电影的实例。
在电影问答的实例中,Gemini 1.5都展现了哪些能力?
-Gemini 1.5能够从44分钟的电影中准确定位某个特定时间点的情景细节,并结合图画输入进行多模态推理和时间戳定位。
谷歌是如何评估Gemini 1.5模型的准确性的?
-谷歌通过特制的“视频海量搜索”和“文本海量搜索”任务来测试Gemini 1.5在处理大规模数据时的准确性,结果显示Gemini 1.5在这些任务上准确率可达99%以上。
Gemini 1.5模型的性能如何?
-从展示的基准测试来看,Gemini 1.5在数学、科学推理、编码、指令跟随等多个领域都超越了之前的版本,整体性能大幅提升。
谷歌是如何训练Gemini 1.5模型的?
-Gemini 1.5模型使用谷歌的TPU V4加速器和分布式训练,在多个数据中心使用多种多模态和多语言数据进行训练。
Outlines
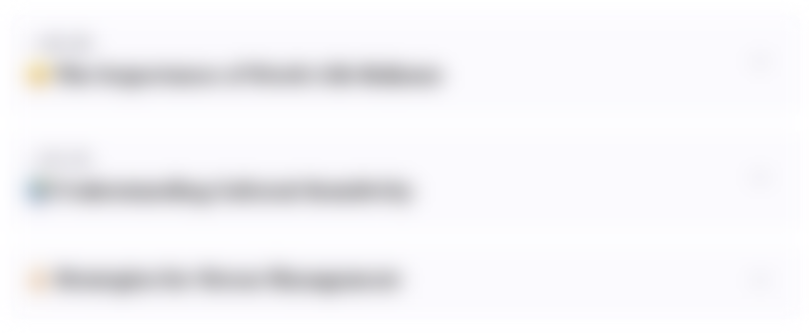
This section is available to paid users only. Please upgrade to access this part.
Upgrade NowMindmap
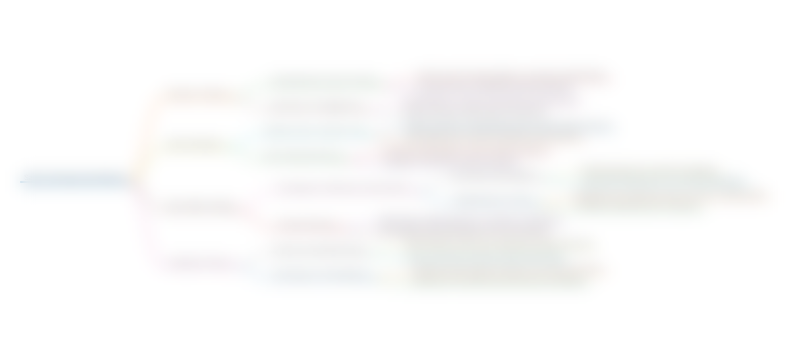
This section is available to paid users only. Please upgrade to access this part.
Upgrade NowKeywords
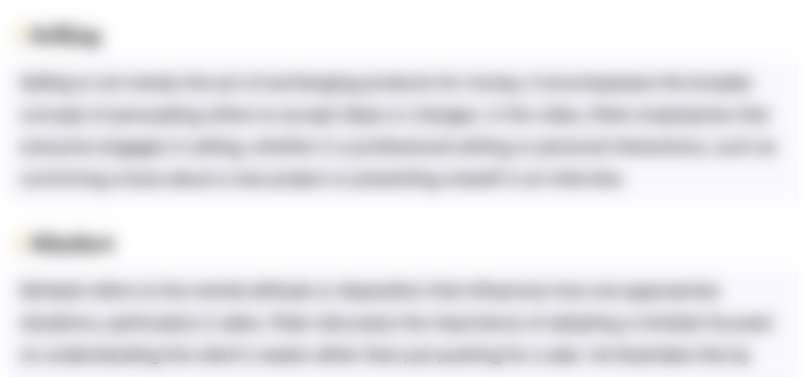
This section is available to paid users only. Please upgrade to access this part.
Upgrade NowHighlights
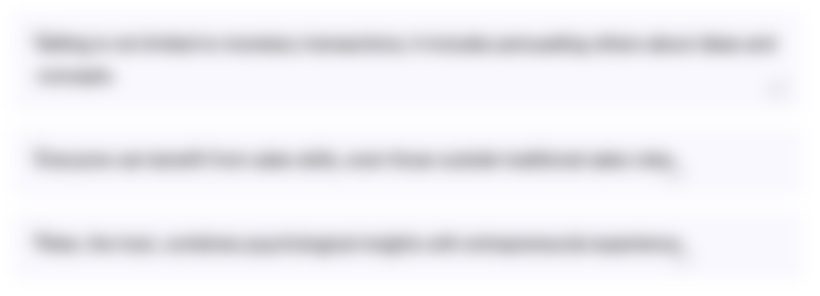
This section is available to paid users only. Please upgrade to access this part.
Upgrade NowTranscripts
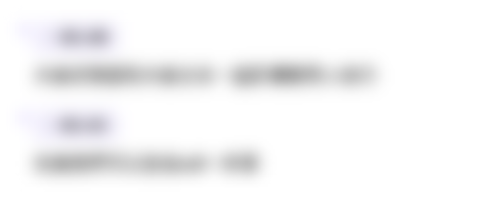
This section is available to paid users only. Please upgrade to access this part.
Upgrade Now5.0 / 5 (0 votes)