Using Generative AI to Tackle Global Challenges
Summary
TLDRCarrie Briskey, VP of AI at Nvidia, discusses the transformative impact of generative AI, highlighting its evolution from early models to the current multimodal capabilities. She emphasizes the importance of customization for domain-specific applications and the challenges of integrating AI into production environments, including latency optimization and security. Briskey showcases real-world applications in healthcare, weather forecasting, and virtual agents, illustrating how generative AI is enhancing efficiency and driving innovation across various industries.
Takeaways
- 🚀 Generative AI is at the forefront of a technological revolution, enabled by accelerated computing platforms, infrastructure, and software.
- 📈 The introduction of the Transformer architecture marked a paradigm shift, allowing models to leverage unlabeled datasets and increase in size and experimentation, leading to improved accuracy.
- 🔢 There has been a significant surge in computational demands with the expansion of model complexity, exemplified by the GPT mixture of experts 1.8 trillion parameter model requiring extensive training time.
- 🌐 Generative AI has expanded beyond computer vision to encompass natural language processing, speech recognition, and deep recommender systems, with models trained on domain-specific languages.
- 🎨 The integration of different generative models and their modalities, termed multimodal, enhances capabilities and was demonstrated by the release of GPT-40.
- 📅 The year 2023 was marked by experimentation with AI models, while 2024 is expected to be the year of moving generative AI into production environments.
- 🛠️ Customization of open validation models is crucial for adapting them to specific domains, requiring data, methodology, tools, and a 'Foundry' approach.
- 🔒 Security, latency optimization, and health checks are among the new challenges enterprises face when integrating AI into their production environments.
- 💡 An example of domain-specific language customization is Nvidia's use of 'Vivid', their proprietary design code, to create a co-pilot for chip designers.
- 🌡️ The trade-off between higher accuracy and compute footprint, including latency, is a critical consideration for deploying AI models.
- 🌍 Generative AI applications span various domains such as drug discovery in healthcare, weather forecasting, and virtual agents for customer support, each with specific optimization needs.
Q & A
Who is Carrie Brisky and what is her role at Nvidia?
-Carrie Brisky is the Vice President of AI Models Software and Services at Nvidia. She heads up software product management for several of Nvidia's model software platforms and microservices.
What technological revolution is currently being discussed in the industry?
-The current technological revolution being discussed is the advent of generative AI, which is enabled by powerful platforms, infrastructure, and software collectively known as accelerated computing.
What was the impact of the Transformer architecture on AI models?
-The Transformer architecture marked a paradigm shift by harnessing unlabeled datasets, which facilitated an increase in model size and experimentation, leading to more accurate AI models like chat GPT.
How did the introduction of the Transformer architecture change the computational demands for AI models?
-The introduction of the Transformer architecture led to a rapid expansion in model complexity and correspondingly increased the demand for computing resources, with some models requiring extensive time and powerful hardware to train.
What is the significance of multimodal generative AI?
-Multimodal generative AI enhances capabilities by integrating different models and combining their modalities, allowing for the generation of a wide range of outputs such as pixels, code, characters, and molecular structures.
What was the 'aha moment' for AI in November 2022 that Carrie Brisky referred to?
-The 'aha moment' in November 2022 was the announcement of chat GPT, which made people realize the transformative potential of AI beyond just computer vision and as a tool for enterprise applications.
What are some of the challenges enterprises face when moving generative AI into production environments?
-Enterprises face challenges such as latency optimization, health checks, and security scanning when moving generative AI into production environments.
Why is customization of AI models important for enterprises?
-Customization is important for enterprises to adapt open validation models to their specific domains, requiring data, methodology tools, and knowledge to tailor the models for their unique needs.
What is the role of 'Nims' in deploying AI models for enterprise applications?
-Nims, or optimized models for inference and enterprise applications, play a crucial role in standardizing the deployment of AI models, ensuring they can run securely and with optimized latency next to data centers.
How is Nvidia addressing the issue of inference latency in AI models?
-Nvidia addresses inference latency by optimizing models through techniques like post-training quantization, distillation, and compression, and by providing platforms that allow for real-time interaction with AI models.
What are some of the domains where generative AI is being applied according to the script?
-Generative AI is being applied in domains such as healthcare for drug discovery, weather forecasting for more accurate predictions, and as virtual co-pilots or agents to boost productivity and efficiency in various sectors.
Outlines
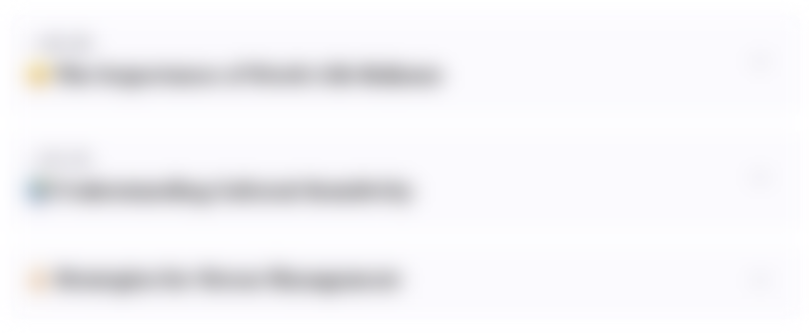
This section is available to paid users only. Please upgrade to access this part.
Upgrade NowMindmap
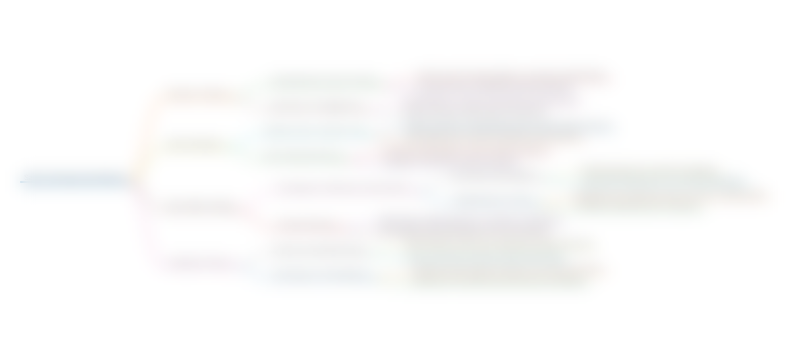
This section is available to paid users only. Please upgrade to access this part.
Upgrade NowKeywords
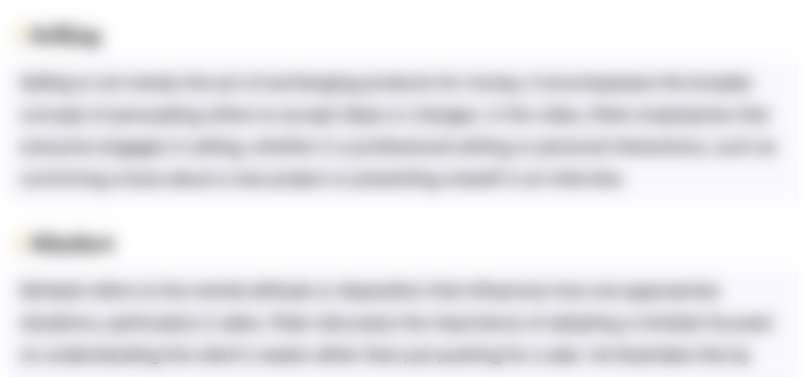
This section is available to paid users only. Please upgrade to access this part.
Upgrade NowHighlights
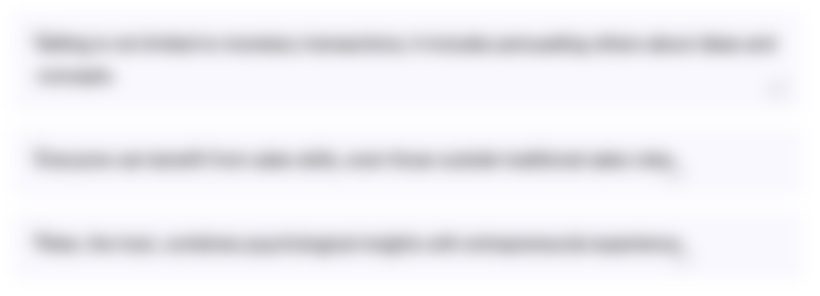
This section is available to paid users only. Please upgrade to access this part.
Upgrade NowTranscripts
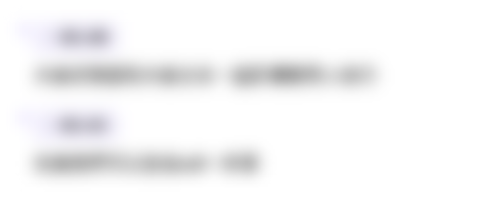
This section is available to paid users only. Please upgrade to access this part.
Upgrade Now5.0 / 5 (0 votes)