AI Everywhere: Transforming Our World, Empowering Humanity
Summary
TLDRВ рамках специального мероприятия в Дарmouth прошла беседа с Мирой Мурати, одной из ведущих специалистов в области искусственного интеллекта и альумной инженерной школы. Руководитель Open AI обсудила разработку инновационных моделей, таких как ChatGPT и Dall-E, а также взглянула на будущие применения ИИ, проблемы безопасности и этические аспекты. Также была поднята тема влияния ИИ на рынок труда и образование, а также важность регулирования использования передовых технологий.
Takeaways
- 🌟 Мира Мурати, известная как главный технический директор в Open AI и выпускница 2012 года из школы инженерии Дарmouth, является одной из ведущих лидеров в области искусственного интеллекта в стране.
- 🎓 В рамках мероприятия Дарmouth была представлена честь приветствовать Миру Мурати и Джоффа Блэкберна, который является членом совета директоров Дарmouth и бывшим старшим вице-президентом по глобальным медиа и развлечениям в Amazon.
- 🏗️ Мира Мурати начала свою карьеру после окончания школы в аэрокосмической промышленности, затем перешла в Tesla, где работала над моделями Model S и Model X, и после этого присоединилась к стартапу, где применяла AI и компьютерное зрение в пространственном общении.
- 🤖 Она была привлечена к Open AI из-за его миссии по созданию безопасного искусственного общего интеллекта и возможности работать над научными исследованиями в этой области.
- 📈 В Open AI Мира взяла на себя роль развития преобразующих моделей, таких как ChatGPT и Dall-E, которые открывают новые горизонты для будущих генеративных технологий ИИ.
- 🔧 Важной частью работы Open AI является понимание и улучшение безопасности и контроля ИИ, включая предотвращение несанкционированного доступа к интернету и самостоятельных действий систем.
- 🧠 Обсуждение включало в себя тему развития ИИ и его влияния на различные отрасли, с утверждением, что технология ИИ будет оказывать влияние на все аспекты когнитивной работы.
- 👨🏫 Высшее образование, по мнению Миры, должно использоваться для продвижения инноваций в образовании и создания высококачественного, доступного и персонализированного обучения с использованием ИИ.
- 🛠️ Мира подчеркнула значимость обучения навыкам, необходимым для работы с новыми технологиями, и умению адаптироваться к быстрому развитию в области ИИ.
- 🌐 Важно обеспечивать этичность и безопасность ИИ, включая вопросы авторских прав, биометрических прав и предотвращение неприкосновенности личности с помощью ИИ.
- 💡 В заключение, Мира Мурати дала совет студентам о важности изучать с радостью и любопытством, а также уменьшения стресса, связанного с будущим карьерным путем.
Q & A
Какое событие проходит в видеотранскрипте?
-В видеотранскрипте проходит специальное событие - беседа с Мирой Мурати, одной из ведущих специалистов в области искусственного интеллекта и альумнажкой инженерного факультета Дартмутского колледжа.
Какое звание у Алексис Абрамсон?
-Алексис Абрамсон является деканом Thayer School of Engineering в Дартмутском колледже.
Что известна о Мирове Мурати?
-Миру Мурати известна как главный технический директор в OpenAI и альумнажкой инженерного факультета Дартмутского колледжа 2012 года. Она признана за ее pioneeering work в области AI, включая развитие моделей ChatGPT и Dall-E.
Какой особой гостевой присутствует на мероприятии?
-Особый гость на мероприятии - Джой Буоламвини, известная своими работами в области этики искусственного интеллекта и алгоритмической справедливости.
Какой опыт Мира Мурати в области инноваций?
-Мира Мурати имеет опыт работы в аэрокосмической отрасли, а также в Tesla, где она работала над моделями Model S и Model X. Она также интересуется применением AI и компьютерного зрения в автономном вождении и пространственном вычислении.
Какие технологии разрабатывает OpenAI?
-OpenAI разрабатывает преобразующие модели, такие как ChatGPT и Dall-E, которые открывают новые горизонты для будущих генеративных технологий AI.
Что такое генеративные AI технологии и как они работают?
-Генеративные AI технологии - это системы, способные создавать новые данные, такие как текст, изображения или видео, на основе обучения на больших объемах данных с использованием глубоких нейросетей.
Какие аспекты безопасности и этики рассматриваются в разработке AI?
-В разработке AI рассматриваются аспекты безопасности, таких как предотвращение несанкционированного доступа к интернету и самостоятельных действий систем, а также социальные последствия, такие как влияние на рабочие места и необходимость регулирования.
Какие возможности для инноваций предлагается в области образования с использованием AI?
-AI предлагает возможности для создания высококачественного, доступного и персонализированного образования, способного удовлетворять индивидуальные потребности обучающихся по всему миру.
Какие изменения в отраслях могут быть вызваны применением AI?
-Применение AI может привести к изменениям во всех отраслях, особенно в области когнитивной работы, включая финансы, контент, медиа, здравоохранение и многие другие.
Какие проблемы могут возникнуть в связи с правами на создание и биометрическими данными при использовании AI?
-Проблемы, связанные с правами на создание, могут включать в себя вопросы о согласии, компенсации и авторских правах при использовании данных для обучения моделей AI. Биометрические данные, такие как голоса и лица, вызывают вопросы о подделке и правах на личность.
Что такое 'Spec' в контексте разработки AI и как он помогает?
-Spec - это инструмент, предоставляющий прозрачность в значения, заложенные в систему AI. Он может быть понятен как 'конституция' для AI-систем, которая развивается и становится более точкой со временем.
Какой подход к тестированию и развертыванию использует OpenAI для своих продуктов?
-OpenAI использует итерационное развертывание, начиная с ограниченного доступа для экспертов и 'красного командования' для изучения рисков, а затем постепенного расширения доступа, собирая обратную связь и понимая крайние случаи.
Какие меры предосторожности применяются для предотвращения негативных последствий использования технологий AI?
-OpenAI разрабатывает и использует такие меры, как водмаркировка, политики контента, инструменты для определения глубоких подделок и распространения информации, а также партнерства с различными общественными группами для управления рисками и проблемами, связанными с AI.
Какие взгляды Алексис Абрамсон на будущее образования и его роль в интеграции AI?
-Алексис Абрамсон видит, что образование должно интегрировать AI для продвижения творчества и знаний, предоставляя высококачественное и доступное образование, способное адаптироваться к индивидуальным потребностям обучающихся.
Какой совет Алексис Абрамсон дает студентам Дартмутского колледжа?
-Алексис Абрамсон советует студентам изучать с меньшим стрессом, изживать учебу с радостью и любопытством, а также стремиться к общему пониманию различных областей знаний.
Outlines
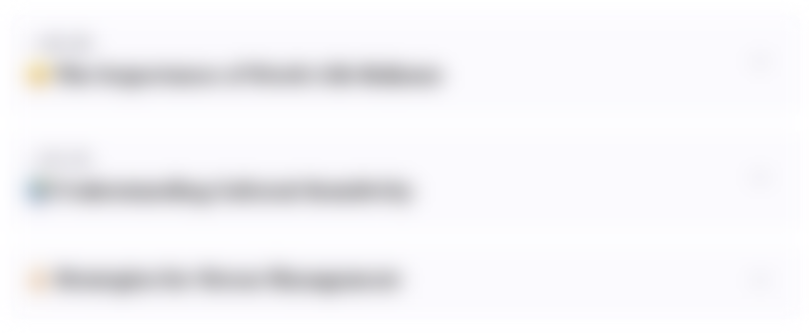
This section is available to paid users only. Please upgrade to access this part.
Upgrade NowMindmap
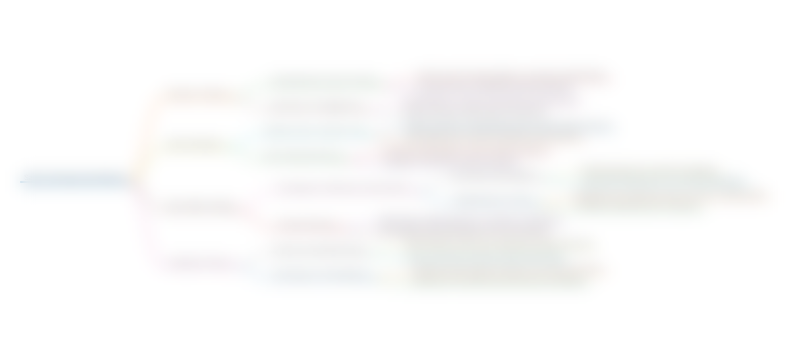
This section is available to paid users only. Please upgrade to access this part.
Upgrade NowKeywords
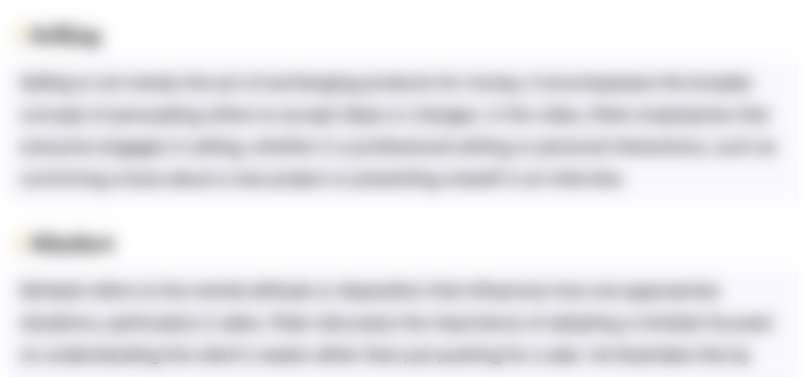
This section is available to paid users only. Please upgrade to access this part.
Upgrade NowHighlights
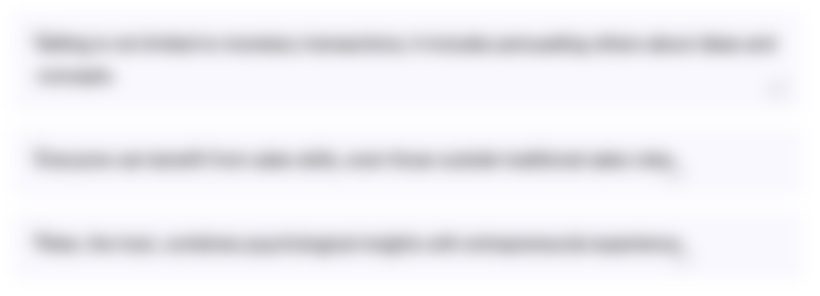
This section is available to paid users only. Please upgrade to access this part.
Upgrade NowTranscripts
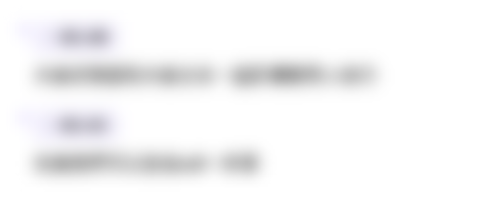
This section is available to paid users only. Please upgrade to access this part.
Upgrade NowBrowse More Related Video

Европа в сфере ИИ бросает вызов технологическим гигантам из США
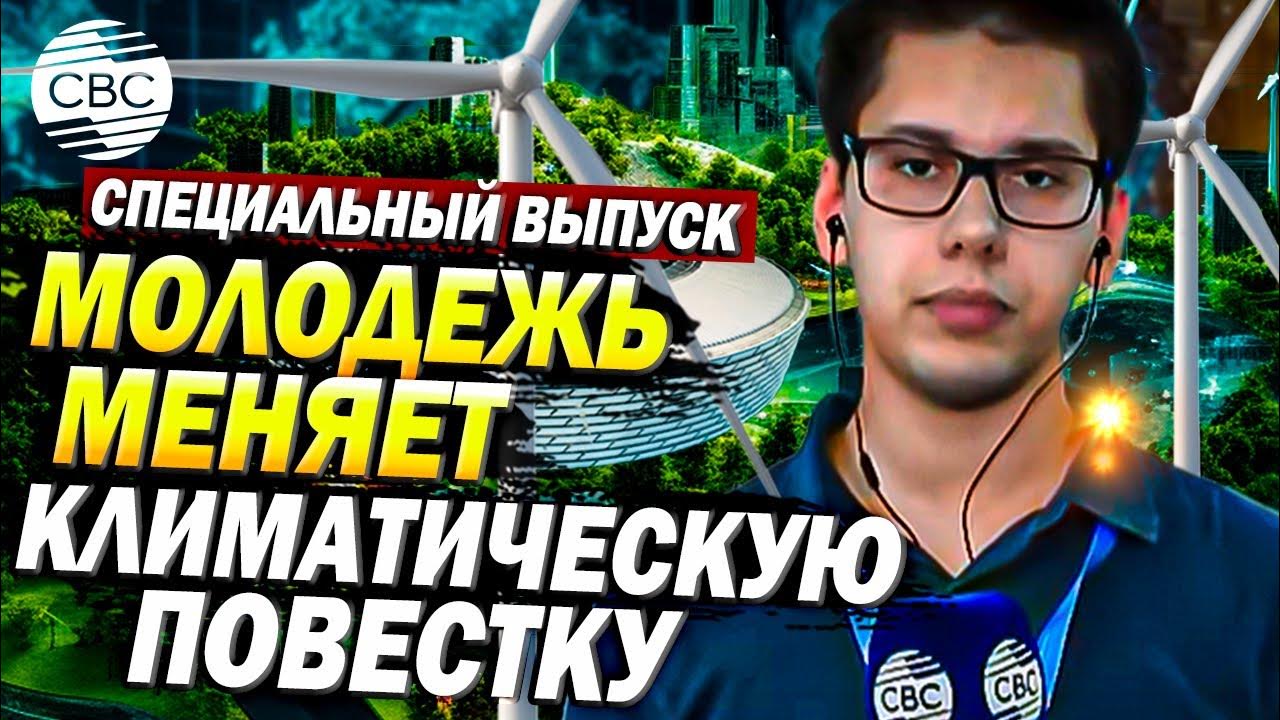
COP29 в Баку: Молодежь на передовой борьбы с климатическими вызовами

The Deutsch Files III
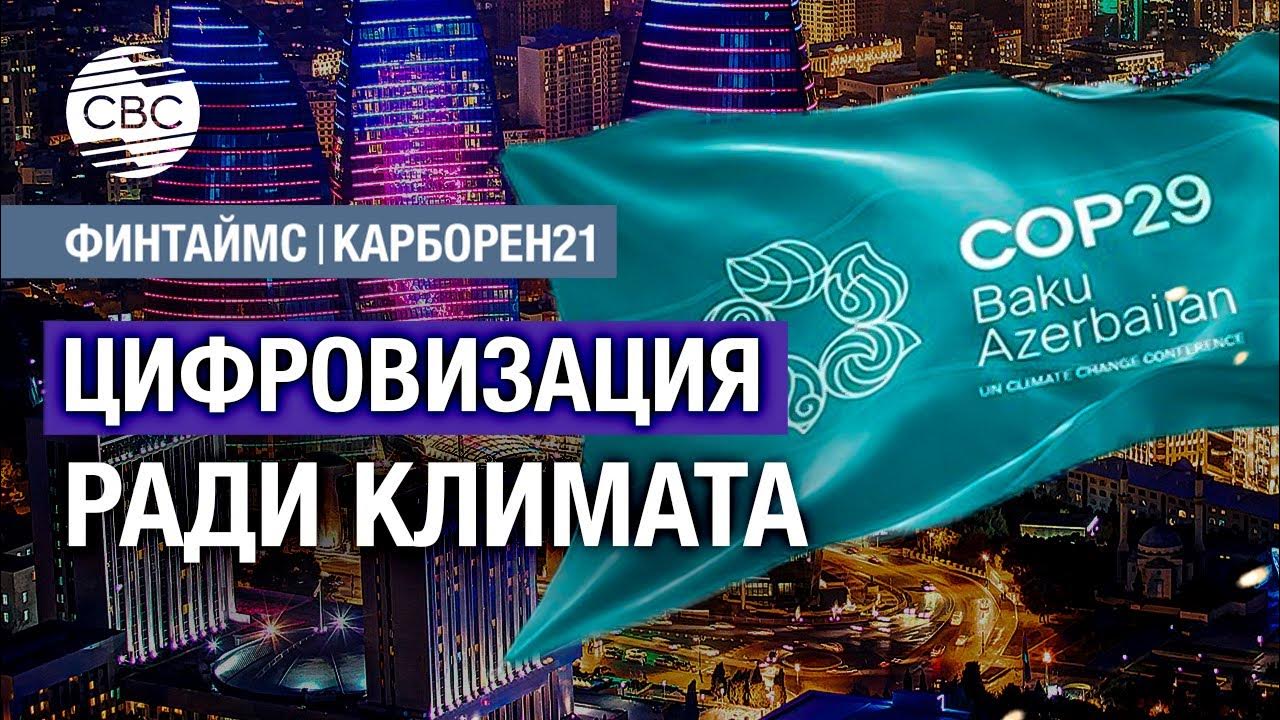
Как Азербайджан готовится к СОР29

Ханкенди и Шуша становятся центром образования Карабаха
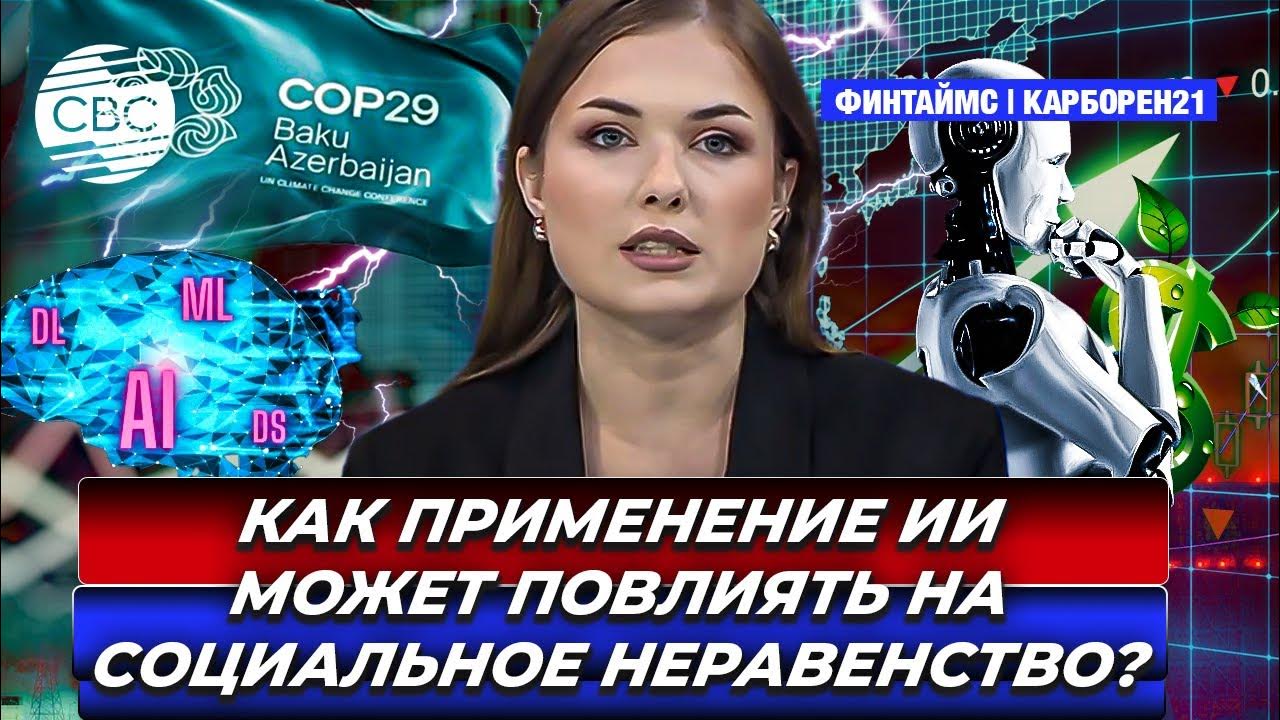
Роль цифровых технологий и искусственного интеллекта в борьбе с изменением климата
5.0 / 5 (0 votes)