Movie Recommender System in Python with LLMs
Summary
TLDRThis video tutorial guides viewers through building a movie recommender system in Python using a local large language model and a vector store. It utilizes a Netflix dataset from Kaggle, transforming movie and TV show information into textual representations. These are then embedded into a 4,096-dimensional vector space by llama 2, allowing similar content to cluster together. The system stores vectors in a Facebook AI Similarity Search (FAISS) database for efficient similarity searches, providing movie recommendations based on user preferences or imagined scenarios.
Takeaways
- ๐ฌ The video is about building a movie recommender system using a large language model and a vector store in Python.
- ๐ The process involves using a dataset from Kaggle, specifically a Netflix dataset containing information about TV shows and movies.
- ๐ Features of the dataset include the title, description, cast, release year, genre, and other details of movies and TV shows.
- ๐ The script describes creating a textual representation of each movie or TV show by combining its features into a single string.
- ๐ง A large language model, in this case, llama 2, is used to embed these textual representations into a high-dimensional vector space.
- ๐ The expectation is that similar movies will be closer in vector space, relying on the intelligence of the language model to determine similarity.
- ๐ The embeddings are stored in a vector store called FAISS (Facebook AI Similarity Search), provided by Facebook.
- ๐ The vector store can then be used to find the top recommendations for a given movie by comparing the movie's vector to others in the store.
- ๐ง The video includes a step-by-step guide on installing the necessary Python packages and the llama 2 model, as well as coding the recommender system.
- ๐ The script also covers how to perform a similarity search using the vector store to recommend movies similar to a given movie.
- ๐ก The video concludes by demonstrating the recommender system with examples, including creating a hypothetical movie and getting recommendations based on it.
Q & A
What is the main topic of the video?
-The main topic of the video is building a movie recommender system using a large language model and a vector store in Python.
Which dataset is used in the video for building the recommender system?
-The video uses a dataset from Kaggle, specifically the Netflix dataset containing information about TV shows and movies.
What features does the Netflix dataset contain?
-The Netflix dataset contains features such as the title, description, cast, release year, genre, and other details of movies and TV shows.
How does the large language model contribute to the recommender system?
-The large language model contributes by taking the textual representation of a movie and embedding it into a high-dimensional vector space, allowing for the identification of similar movies based on their vector proximity.
What is the name of the vector store used in the video?
-The vector store used in the video is called FAISS, which stands for Facebook AI Similarity Search.
What is the dimension of the vectors produced by the large language model in this video?
-The large language model, in this case, llama 2, produces vectors with a dimension of 4096.
How does the recommender system determine which movies are similar?
-The recommender system determines similarity by comparing the vector representations of movies in the vector space, where closer vectors indicate more similar movies.
What Python packages are needed to implement the recommender system as described in the video?
-The Python packages needed are numpy, pandas, FAISS, and requests.
How does the video demonstrate the process of creating a textual representation of a movie?
-The video demonstrates this by creating a function that formats a multi-line string containing various attributes of a movie from a data frame, such as type, title, director, cast, release year, genre, and description.
What is the purpose of the 'create_textual_representation' function in the script?
-The 'create_textual_representation' function is used to generate a textual representation of each movie from the data frame, which includes details like type, title, director, cast, release year, genre, and description.
How can the recommender system be tested with a new or hypothetical movie?
-The recommender system can be tested by creating a textual representation of a new or hypothetical movie, embedding it using the large language model, and then performing a similarity search to find the most similar existing movies in the vector store.
What is the significance of the embedding process in the context of the recommender system?
-The embedding process is significant as it translates the textual information of movies into a numerical format that can be compared and analyzed within the vector space, which is essential for the similarity search and recommendation functionality.
How does the video handle the installation and usage of the large language model llama 2?
-The video instructs viewers to install llama 2 by downloading it from the official website, using the command line to pull the model, and then utilizing it locally for generating embeddings from textual representations.
What is the final output of the recommender system when a user queries for movie recommendations?
-The final output of the recommender system is a list of the top five most similar movies to the user's query, which could be based on an existing movie from the dataset or a hypothetical movie created by the user.
Outlines
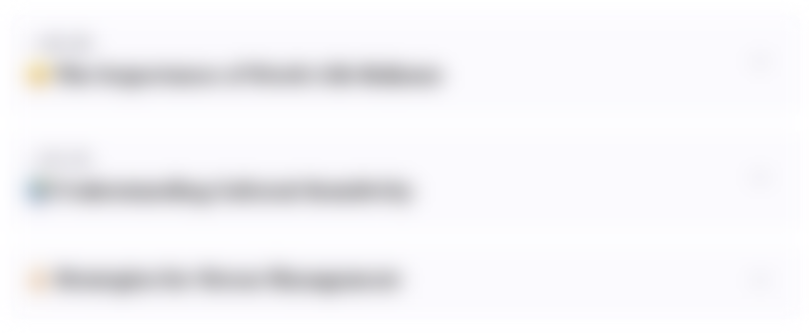
This section is available to paid users only. Please upgrade to access this part.
Upgrade NowMindmap
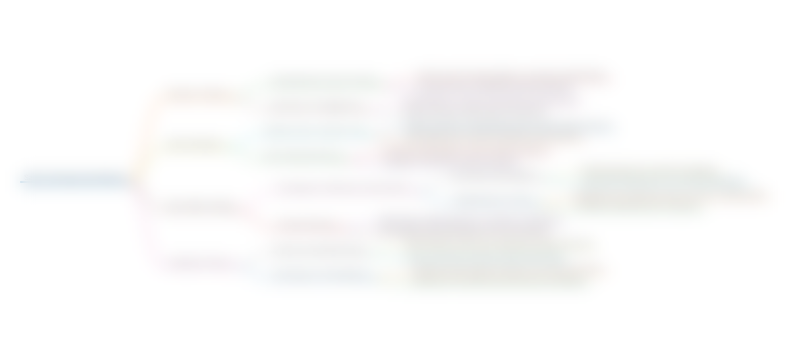
This section is available to paid users only. Please upgrade to access this part.
Upgrade NowKeywords
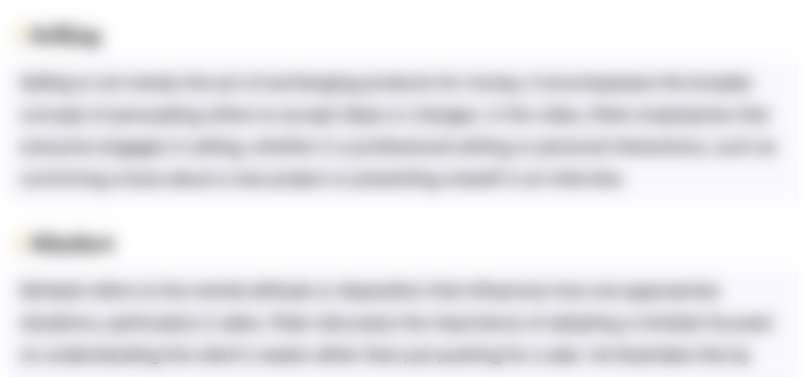
This section is available to paid users only. Please upgrade to access this part.
Upgrade NowHighlights
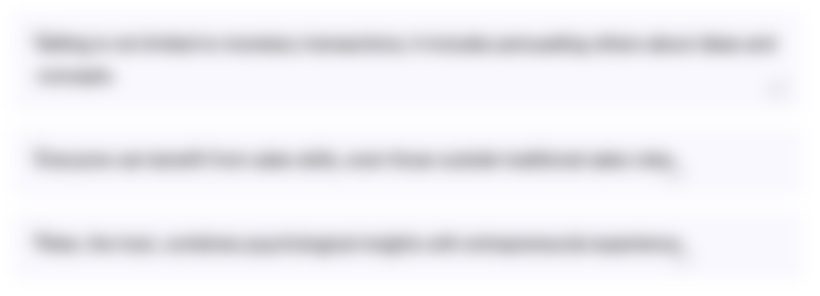
This section is available to paid users only. Please upgrade to access this part.
Upgrade NowTranscripts
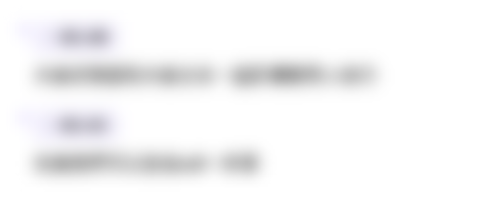
This section is available to paid users only. Please upgrade to access this part.
Upgrade NowBrowse More Related Video
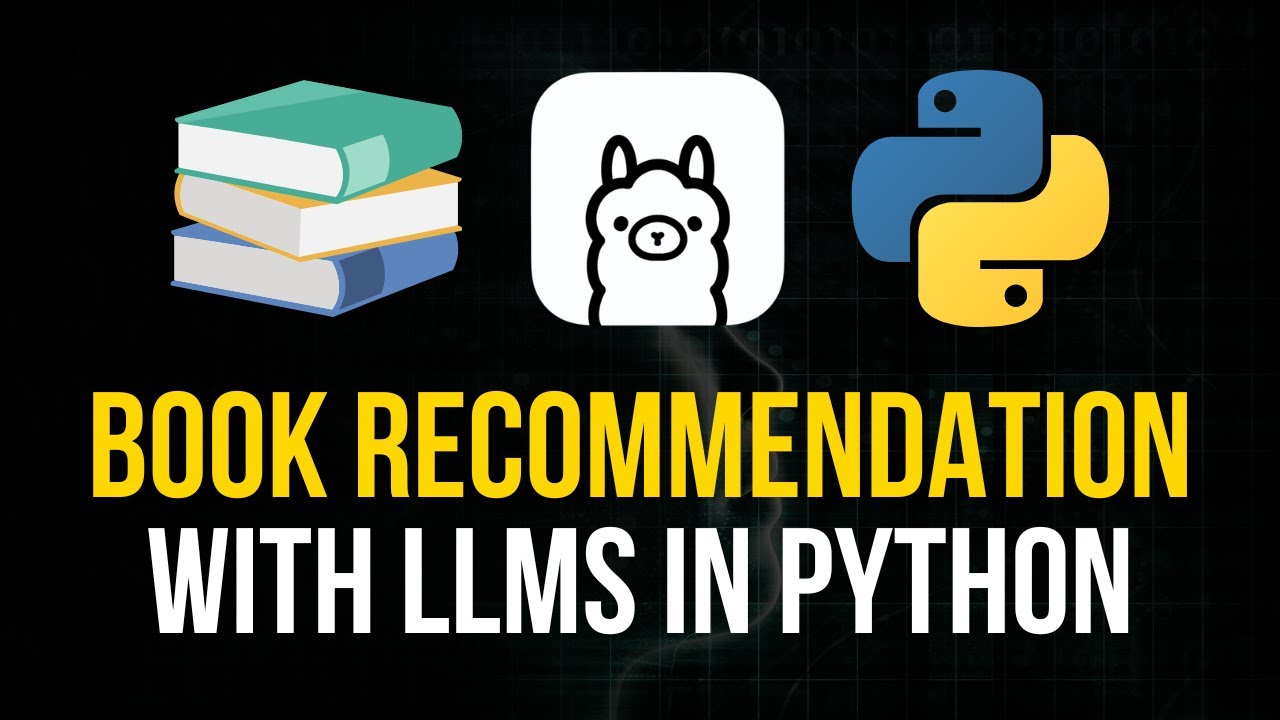
Book Recommendation System in Python with LLMs
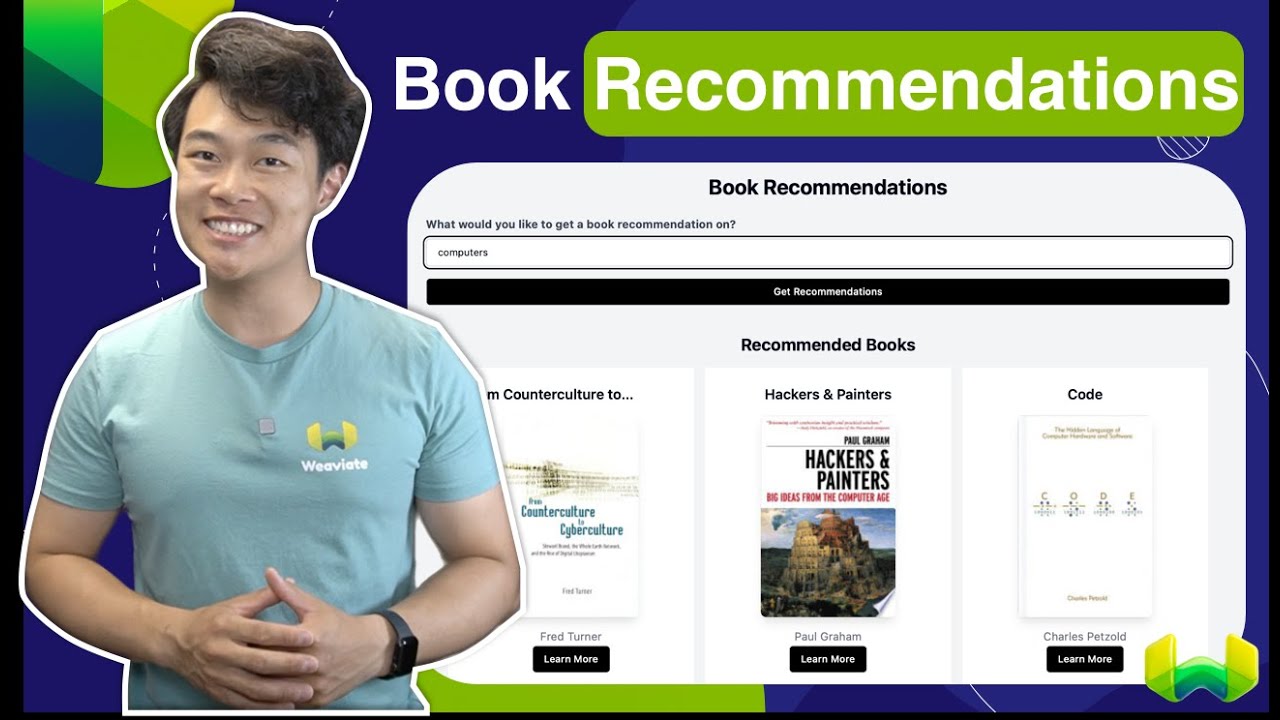
How to Build a Recommendation System with AI and Semantic Search
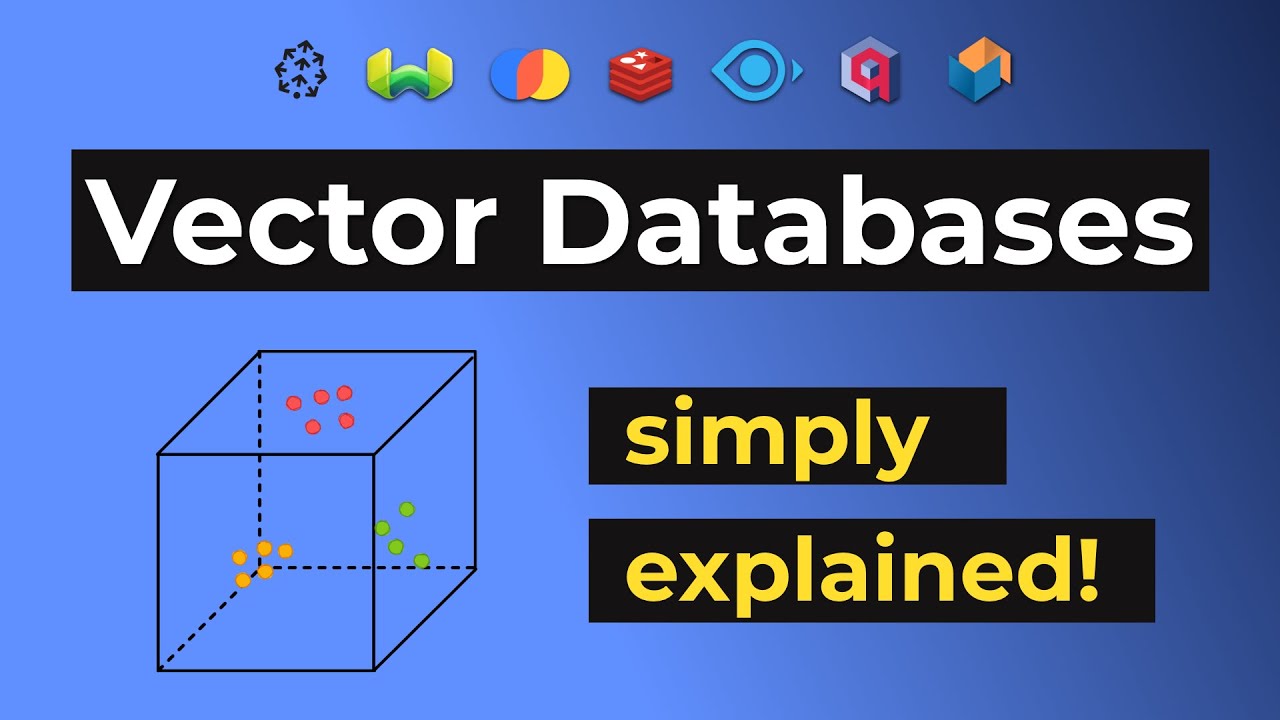
Vector Databases simply explained! (Embeddings & Indexes)
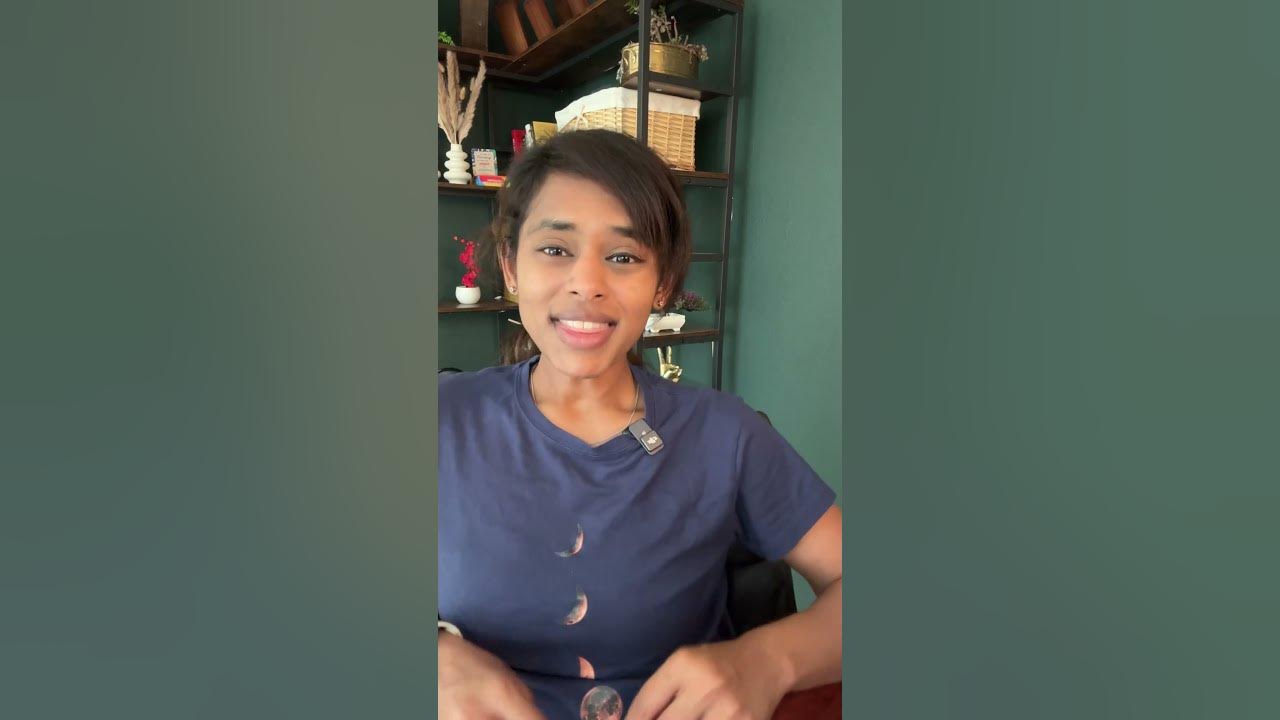
Introduction to Generative AI (Day 10/20) What are vector databases?
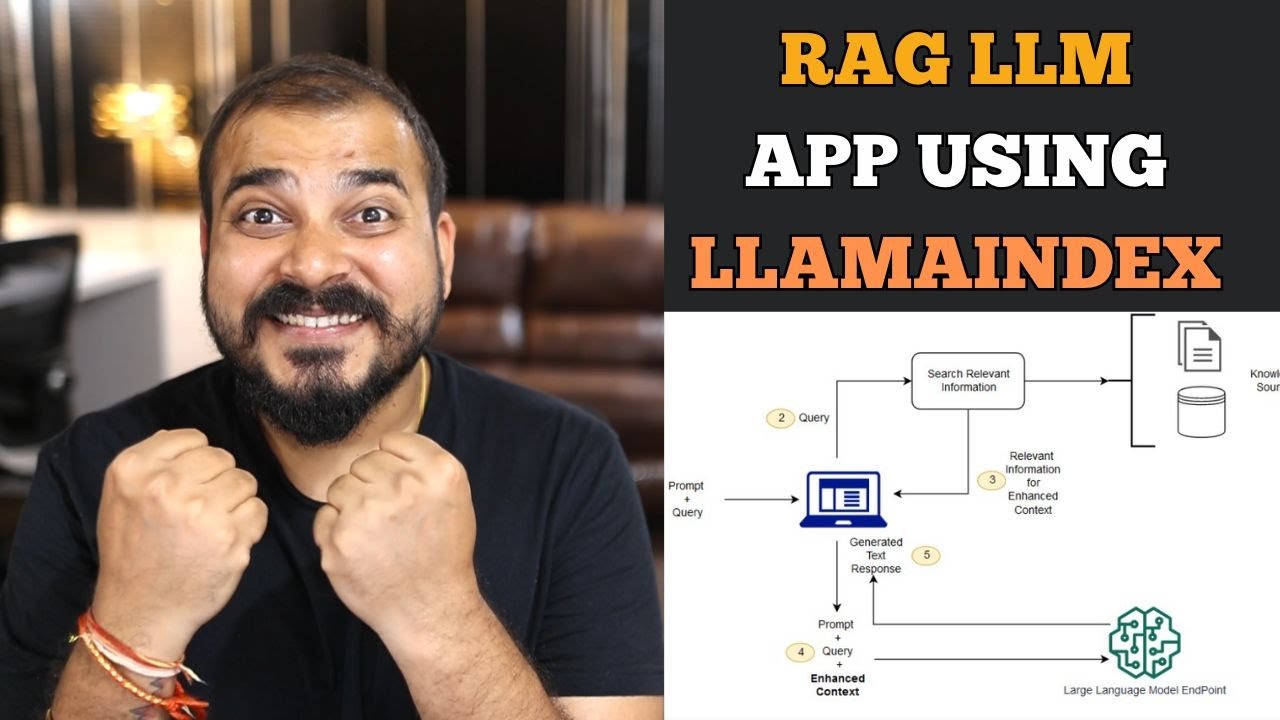
End to end RAG LLM App Using Llamaindex and OpenAI- Indexing and Querying Multiple pdf's
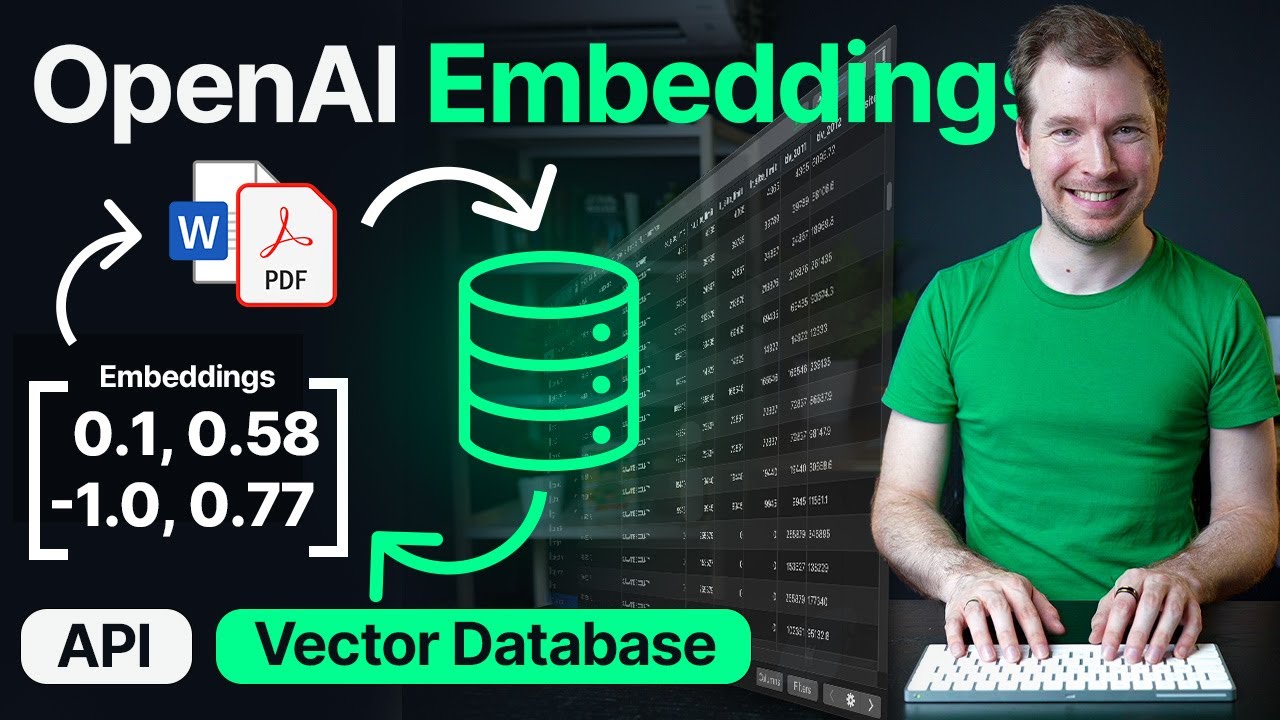
OpenAI Embeddings and Vector Databases Crash Course
5.0 / 5 (0 votes)