p-hacking: What it is and how to avoid it!
Summary
TLDRIn this StatQuest episode, Josh Starmer explains P-hacking, a practice where researchers manipulate data analysis to achieve statistically significant results, leading to false positives. He uses the example of testing drugs for virus recovery to illustrate how P-hacking can occur. The video warns against cherry-picking data and emphasizes the importance of proper sample size determination through power analysis to avoid false positives. It also introduces methods like the false discovery rate to compensate for multiple testing problems.
Takeaways
- 🔬 P-hacking refers to the misuse of statistical analyses to produce false positives, which can lead to incorrect conclusions in research.
- 📊 In the context of drug testing, P-hacking can occur when researchers continue to test different drugs until they find one that appears to work, based on a statistically significant p-value.
- 📈 The script illustrates the concept using a normal distribution to show how recovery times from a virus can be analyzed, and how selecting specific data points can lead to misleading results.
- 🤔 The importance of not cherry-picking data is emphasized; researchers should not only test and report on the data that supports their hypotheses.
- 📉 The script explains the multiple testing problem, where conducting many tests increases the likelihood of obtaining false positives due to the arbitrary p-value threshold of 0.05.
- 🛠 To combat P-hacking, the script suggests using methods like the false discovery rate, which adjusts p-values to account for multiple comparisons and reduces the chance of false positives.
- 🔎 The concept of power analysis is introduced as a way to determine the appropriate sample size before conducting an experiment, which can help prevent P-hacking by ensuring sufficient data to detect true effects.
- 🚫 The script warns against the temptation to add more data to a study after observing a p-value close to the significance threshold, as this can increase the risk of false positives.
- 📝 The necessity of including all p-values from all tests in any adjustment method is highlighted to ensure the validity of the statistical analysis.
- 🎓 The video concludes with a call to action for viewers to learn more about statistical methods to avoid P-hacking, such as through further StatQuest videos on power analysis.
Q & A
What is P-hacking?
-P-hacking refers to the misuse and abuse of analysis techniques, which can lead to being fooled by false positives.
Why is P-hacking a problem in statistical analysis?
-P-hacking is a problem because it can lead to false positives, which means incorrectly rejecting the null hypothesis when there is no actual effect.
What is the significance level typically used in statistical tests?
-The significance level typically used in statistical tests is 0.05, which means that there is a 5% chance of a false positive.
What is the multiple testing problem?
-The multiple testing problem occurs when doing a lot of tests, which increases the likelihood of encountering false positives.
How can the false discovery rate help address the multiple testing problem?
-The false discovery rate adjusts p-values to account for multiple comparisons, usually resulting in larger p-values and reducing the number of false positives.
What is a power analysis and why is it important?
-A power analysis is performed before an experiment to determine the appropriate sample size needed to have a high probability of correctly rejecting the null hypothesis.
Why is it incorrect to add more data to a test with a p-value close to 0.05?
-Adding more data to a test with a p-value close to 0.05 can increase the chance of a false positive, as the initial p-value calculation already considered the data available.
What should one do when they get a p-value close to but not less than 0.05?
-When a p-value is close to but not less than 0.05, one should conduct a power analysis to determine the correct sample size rather than adding more data to the existing test.
What is the role of the null hypothesis in the context of P-hacking?
-In the context of P-hacking, the null hypothesis is that there is no difference between groups or conditions. P-hacking can lead to the incorrect rejection of this null hypothesis due to false positives.
How can one avoid P-hacking in their statistical analyses?
-To avoid P-hacking, one should calculate p-values for all tests, adjust for multiple comparisons using methods like the false discovery rate, and conduct power analyses to determine appropriate sample sizes.
Outlines
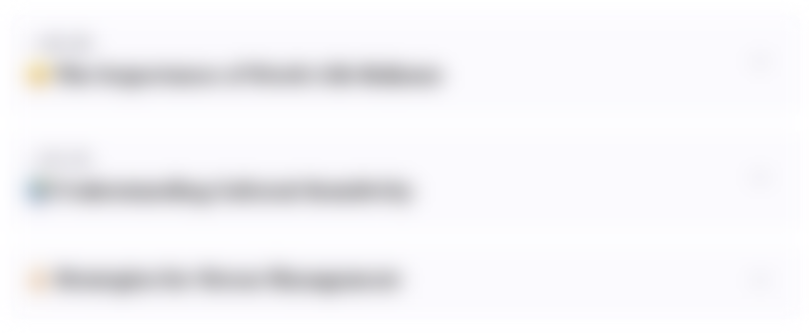
This section is available to paid users only. Please upgrade to access this part.
Upgrade NowMindmap
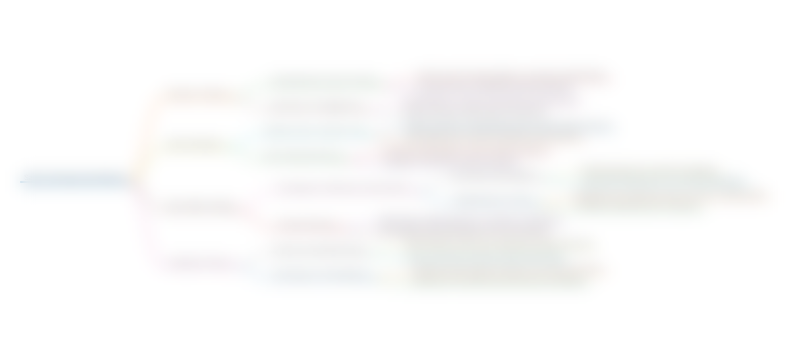
This section is available to paid users only. Please upgrade to access this part.
Upgrade NowKeywords
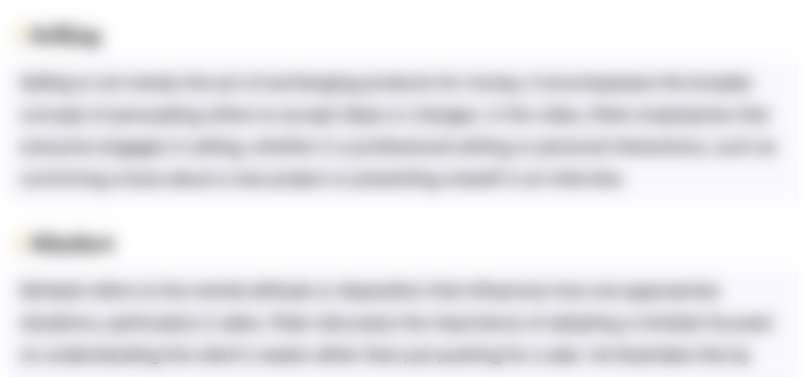
This section is available to paid users only. Please upgrade to access this part.
Upgrade NowHighlights
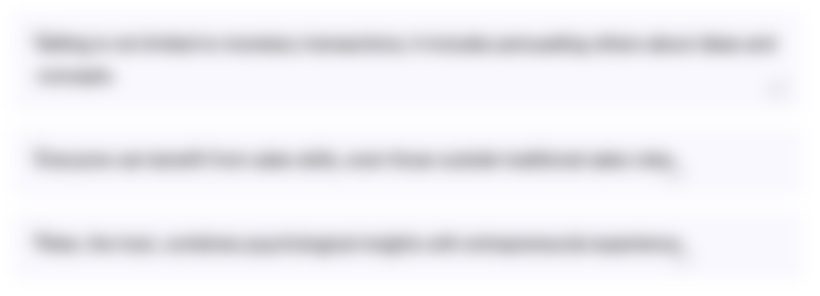
This section is available to paid users only. Please upgrade to access this part.
Upgrade NowTranscripts
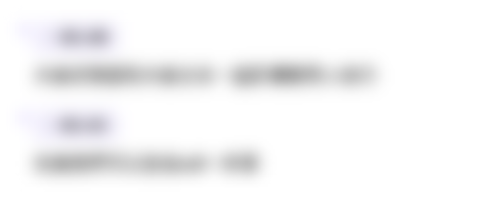
This section is available to paid users only. Please upgrade to access this part.
Upgrade NowBrowse More Related Video

p-values: What they are and how to interpret them

STAT115 Chapter 5.3 Multiple Hypotheses Testing and False Discovery Rate
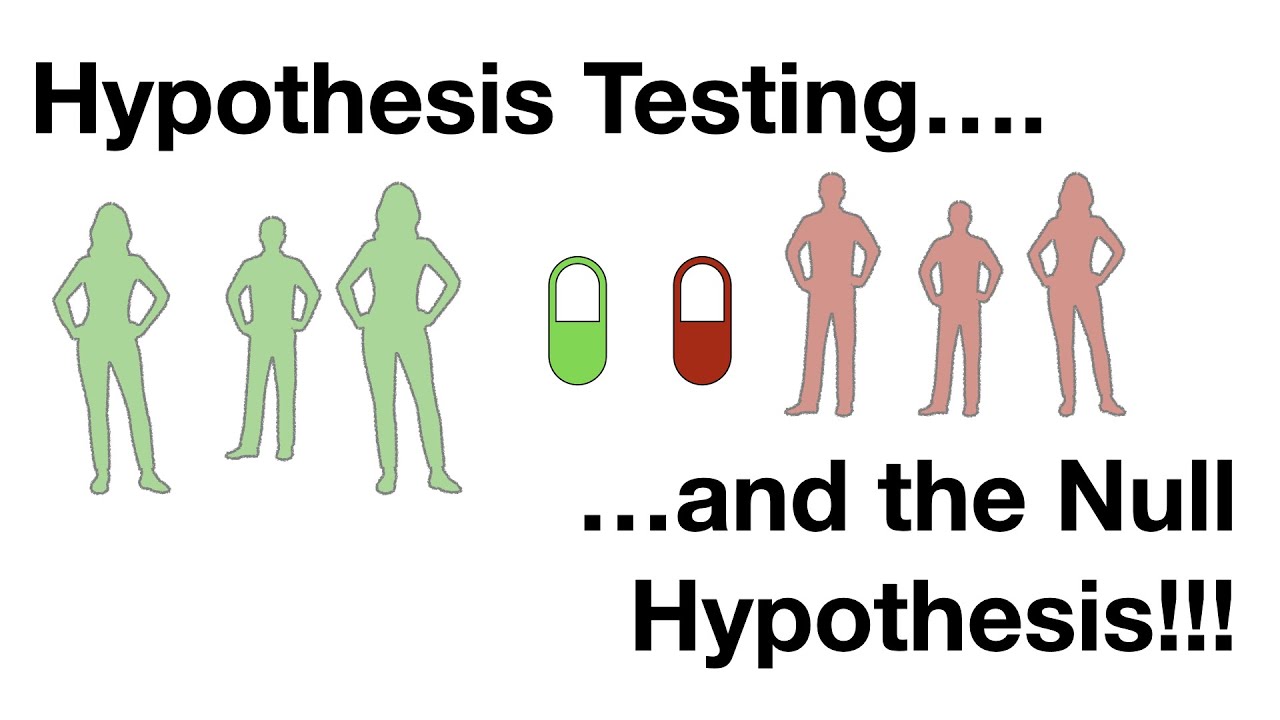
Hypothesis Testing and The Null Hypothesis, Clearly Explained!!!
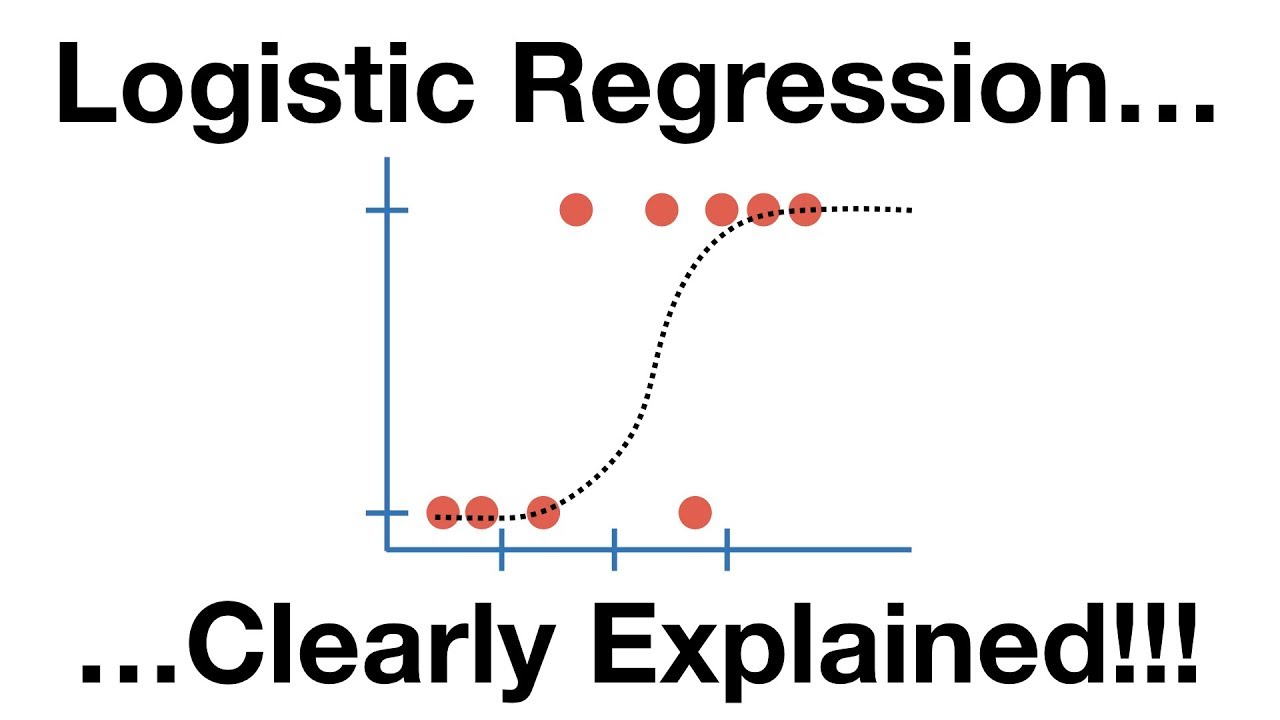
StatQuest: Logistic Regression
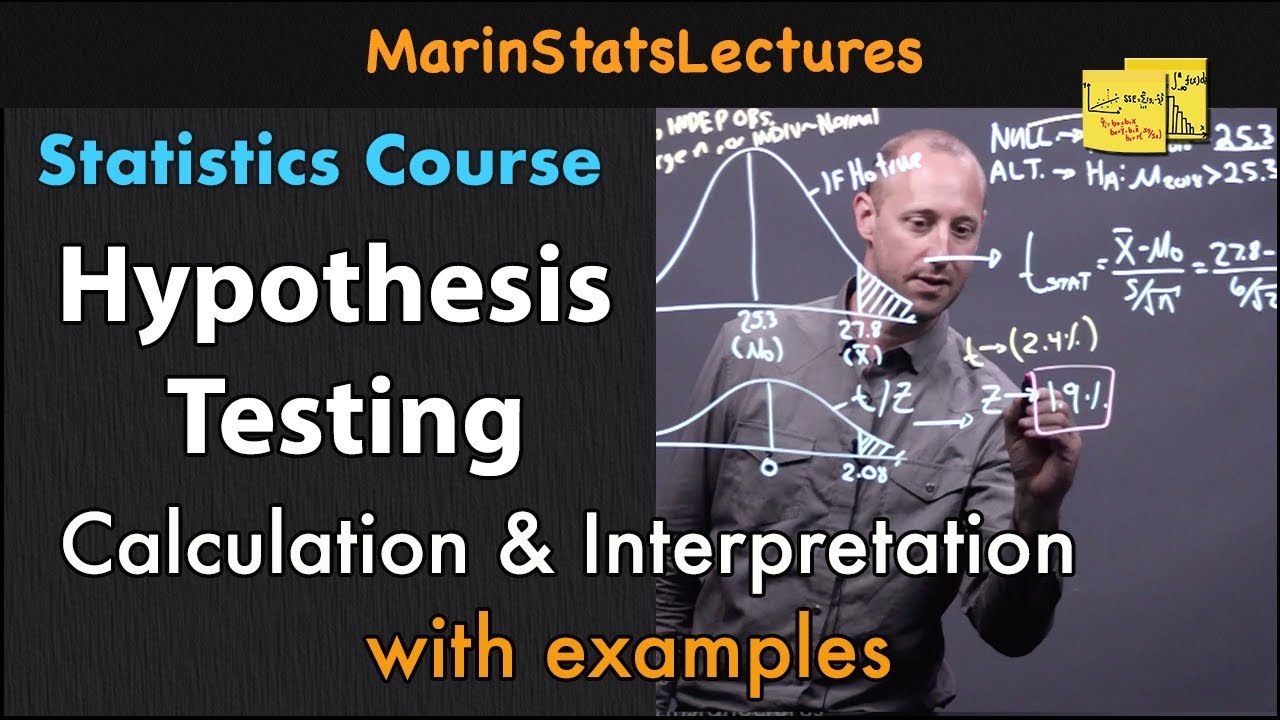
Hypothesis Testing: Calculations and Interpretations| Statistics Tutorial #13 | MarinStatsLectures

How to Perform and Interpret Independent Sample T-Test in SPSS
5.0 / 5 (0 votes)