Peramalan Kuantitatif 1
Summary
TLDRThe video delves into quantitative forecasting methods, focusing on time series analysis. Key techniques discussed include moving averages, weighted moving averages, and exponential smoothing. The importance of understanding components like trend, seasonality, and cyclic variations is emphasized, along with practical examples of applying these methods to forecast demand. Additionally, the video covers how to measure forecasting errors using metrics like Mean Absolute Deviation (MAD), Mean Square Error (MSE), and Mean Absolute Percentage Error (MAPE). The session concludes with a preview of upcoming topics on trend analysis and associative models.
Takeaways
- 😀 Time series forecasting involves both qualitative and quantitative approaches, which are essential in predicting future data trends.
- 😀 Quantitative forecasting is split into two main models: time series models (such as moving averages) and associative models.
- 😀 Time series analysis includes key components like trend, seasonality, randomness, and cycles, which must be understood to interpret data correctly.
- 😀 Trend refers to a consistent increase or decrease in data over time, while seasonality refers to recurring patterns, such as in retail or tourism.
- 😀 Random variation in data reflects unpredictable fluctuations due to unique events or conditions, making it critical to account for in forecasting.
- 😀 Cycles in time series data are long-term patterns, often linked to external factors like political conditions or long-term planning.
- 😀 Moving averages are a simple method for smoothing time series data and predicting future values based on past periods.
- 😀 Weighted moving averages apply different weights to past periods, giving more importance to recent data, which is useful when trends start to emerge.
- 😀 Exponential smoothing is another method where forecasts are updated by blending the previous forecast with the actual data, using a smoothing constant (alpha).
- 😀 Choosing the right value of alpha in exponential smoothing is crucial, as a higher alpha is useful when trends change rapidly, and a lower alpha is for stable trends.
- 😀 Forecast errors, including measures like Mean Absolute Deviation (MAD), Mean Squared Error (MSE), and Mean Absolute Percentage Error (MAPE), help evaluate the accuracy of predictions and select the best model.
Q & A
What are the two main types of forecasting models discussed in the transcript?
-The two main types of forecasting models discussed are time series models and associative models.
What is the naive approach in forecasting?
-The naive approach assumes that the demand in the upcoming period will be the same as the demand in the previous period. For example, if sales in January were 68 units, it assumes February will have the same sales.
What is the moving average method used for in forecasting?
-The moving average method is used to smooth out data by averaging past periods to predict future values. It is typically used when trends are small or absent.
What are the components of time series data in forecasting?
-The components of time series data include trend (a long-term increase or decrease), seasonal (patterns that repeat over time), random variations (unpredictable data points), and cycles (long-term fluctuations tied to external factors like political conditions).
How is the weighted moving average different from a simple moving average?
-The weighted moving average assigns different weights to different periods, giving more importance to recent data points. This is in contrast to the simple moving average, where each period is treated equally.
How does exponential smoothing work in forecasting?
-Exponential smoothing adjusts predictions by combining previous forecasts with actual demand data, weighted by a constant alpha. The formula used is FT = FT-1 + α(At-1 - FT-1), where FT is the forecast, α is the smoothing constant, and At-1 is the actual demand.
What is the importance of the smoothing constant (α) in exponential smoothing?
-The smoothing constant (α) controls how much weight is given to the most recent actual data. A higher α responds more to recent changes, while a lower α gives more weight to the past forecast.
What are some potential problems with moving average methods?
-Some issues with moving averages include reduced sensitivity to actual changes in the data and the inability to capture trends or seasonal patterns effectively, especially as the number of periods increases.
What is meant by 'forecast error' in forecasting models?
-Forecast error refers to the difference between the actual demand and the predicted forecast. It is used to evaluate the accuracy of forecasting models.
What methods are used to measure the forecast error?
-Forecast error can be measured using metrics like Mean Absolute Deviation (MAD), Mean Squared Error (MSE), and Mean Absolute Percentage Error (MAPE). These metrics assess the average size and squared deviations of forecast errors.
Outlines
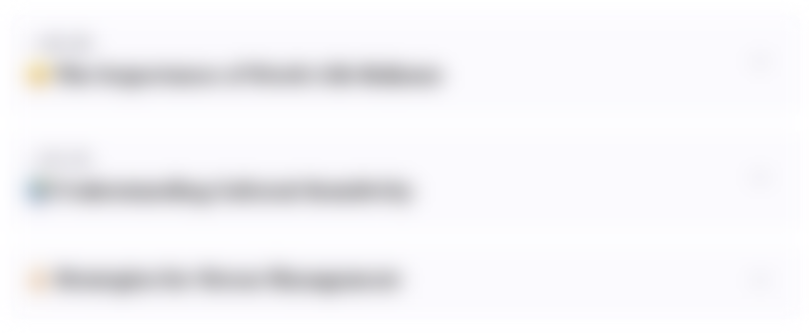
This section is available to paid users only. Please upgrade to access this part.
Upgrade NowMindmap
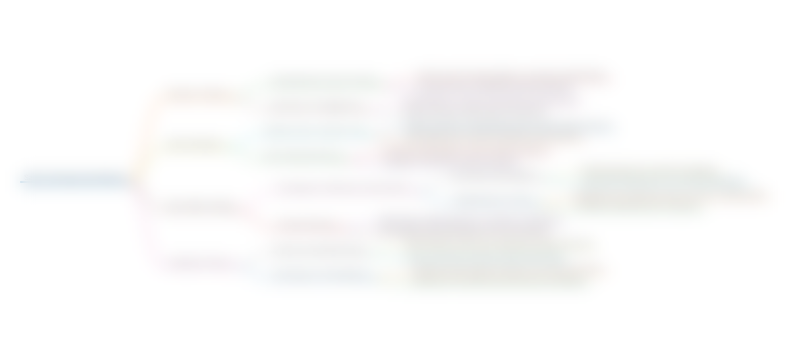
This section is available to paid users only. Please upgrade to access this part.
Upgrade NowKeywords
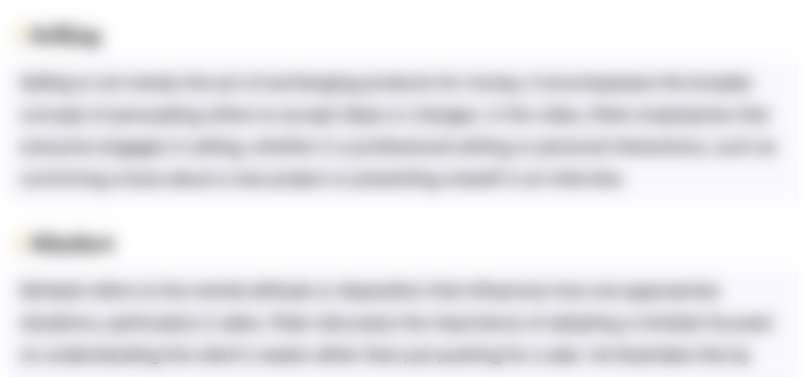
This section is available to paid users only. Please upgrade to access this part.
Upgrade NowHighlights
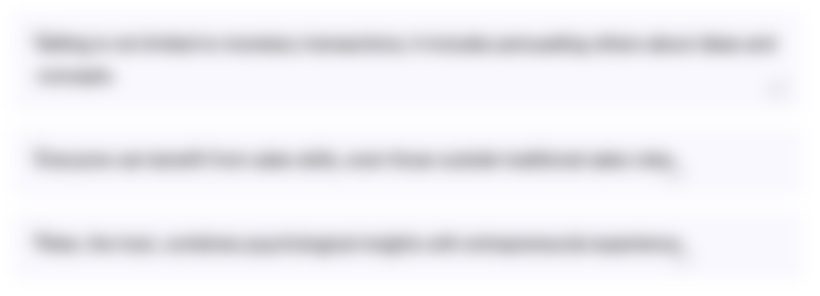
This section is available to paid users only. Please upgrade to access this part.
Upgrade NowTranscripts
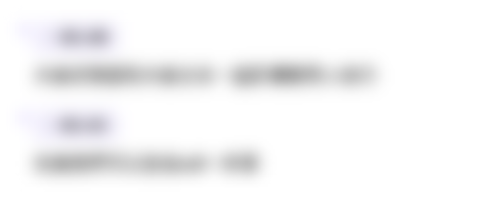
This section is available to paid users only. Please upgrade to access this part.
Upgrade NowBrowse More Related Video
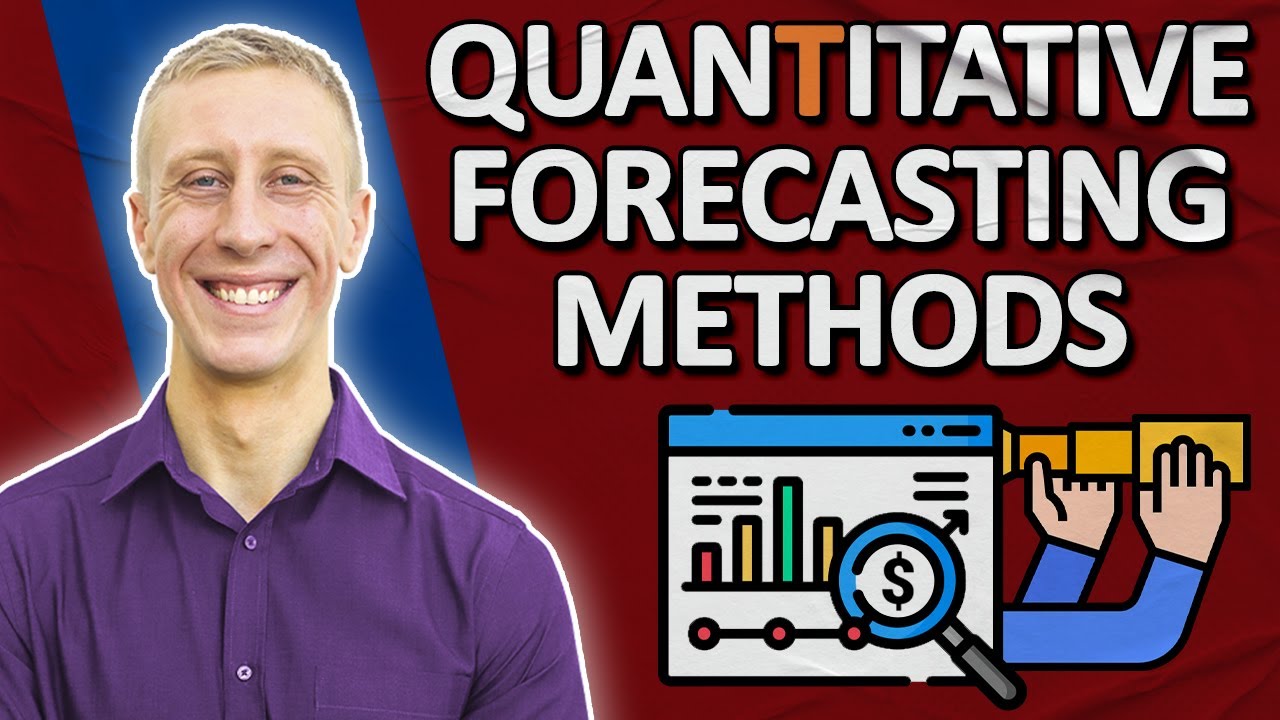
Quantitative Forecasting Methods in Business Operations
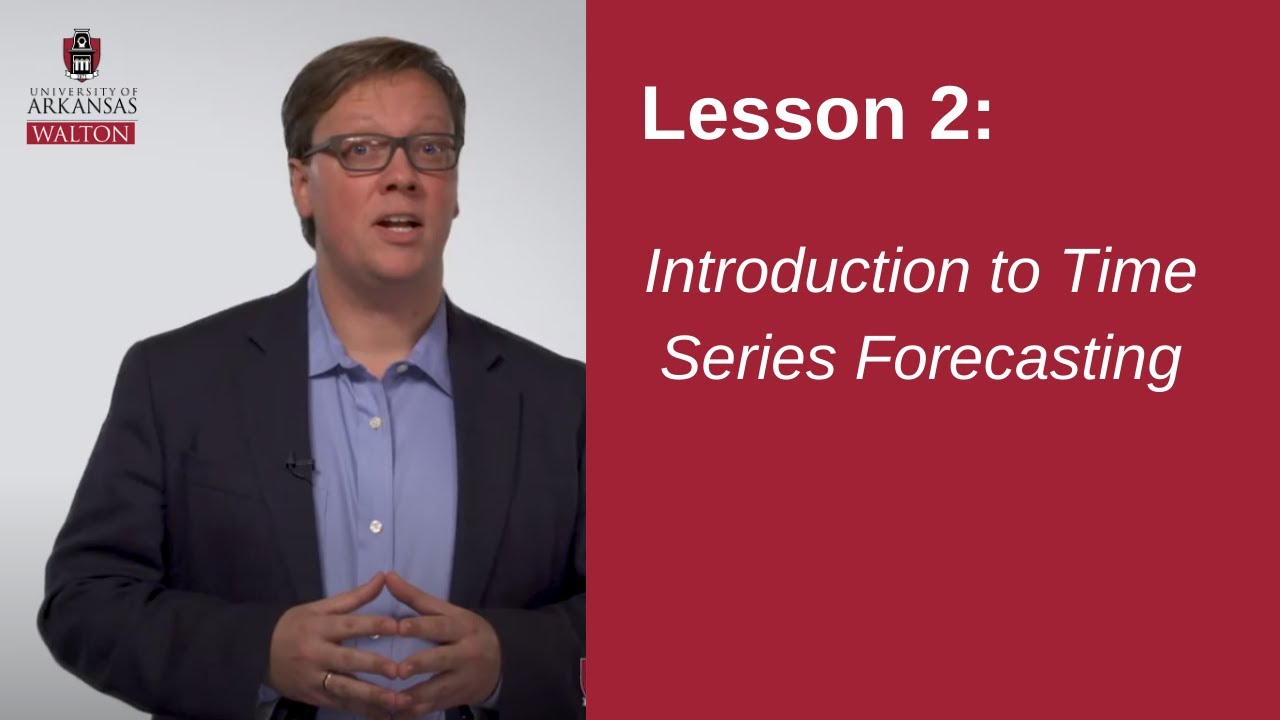
Introduction to Time Series Forecasting | SCMT 3623
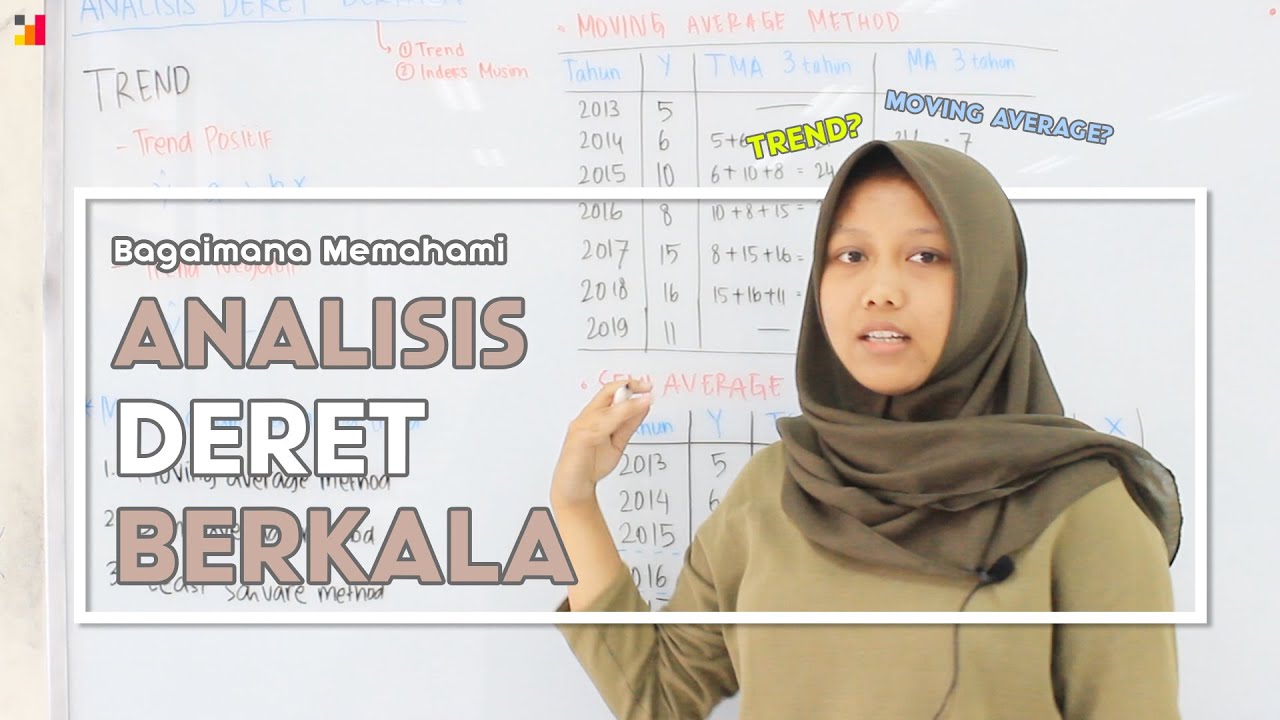
Analisis Deret Berkala - Pengantar Statistika Ekonomi dan Bisnis (Statistik 1) | E-Learning STA
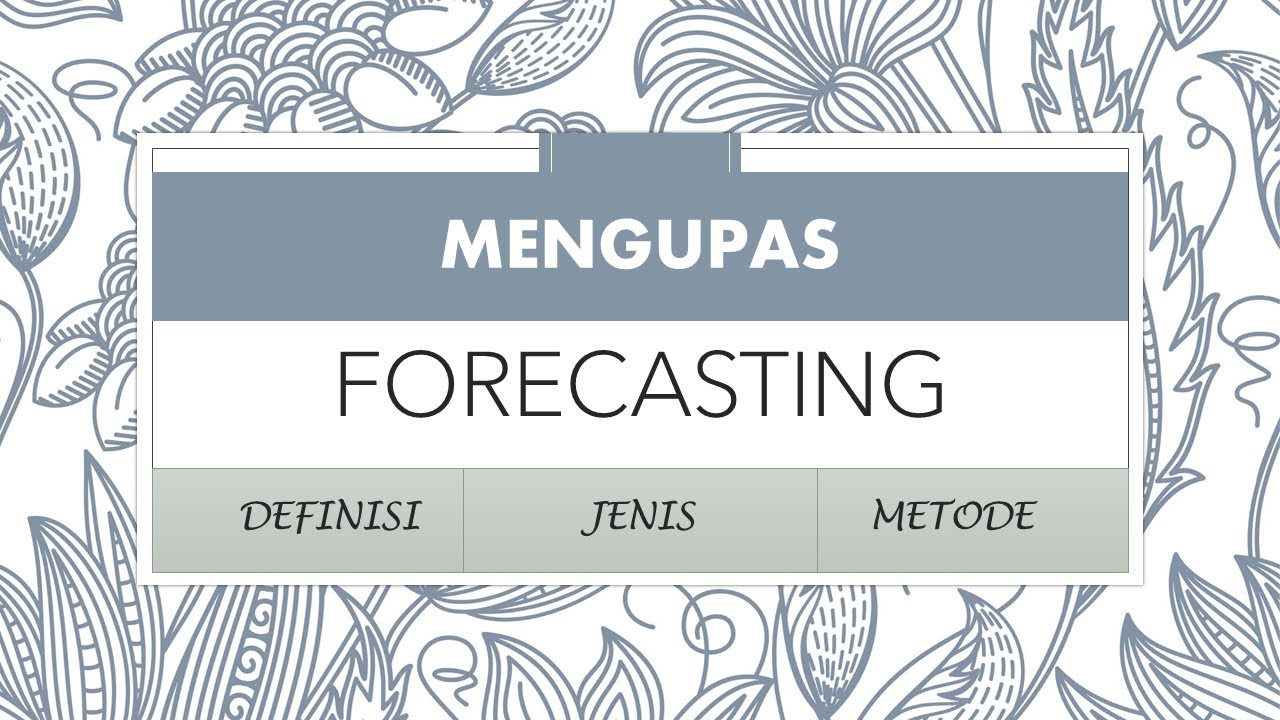
Metode Peramalan (Forecasting)
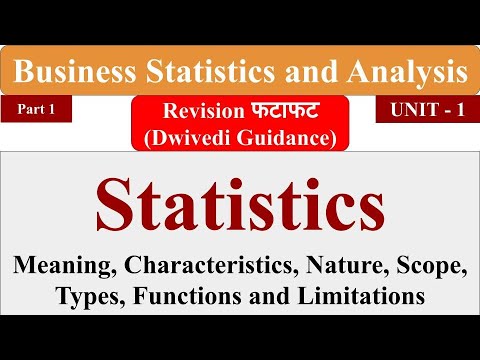
Business Statistics and Analytics, business statistics and analytics aktu notes, mba, bba, dwivedi
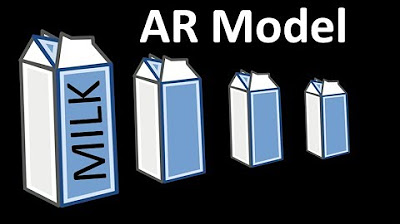
Time Series Talk : Autoregressive Model
5.0 / 5 (0 votes)