OpenAI O3: A Breakthrough in Agentic Coding?
Summary
TLDRThis video provides an in-depth comparison between Gemini 2.5 Pro and OpenAI's latest models (O3 and O4 Mini). The analysis highlights Gemini's impressive performance and cost-efficiency, especially in benchmarks like the humanities exam and GPQA, where it outperforms others at a lower cost. However, while O3 is still a strong contender for code-specific tasks, Gemini shines in general performance. Despite not being AGI, Gemini's ability to use tools and agentic workflows makes it an exciting addition to the AI landscape. The video concludes with the presenter inviting viewers to share their own experiences with these new models.
Takeaways
- 😀 Gemini 2.5 Pro has strong performance in various benchmarks, especially in comparison to OpenAI's models like GPT-4.
- 😀 Benchmark comparisons highlight that Gemini 2.5 Pro outperforms other models in specific areas like humanities exams and multi-challenge tasks.
- 😀 Despite its strong performance, Gemini 2.5 Pro lags behind GPT-3 in terms of cost-efficiency for some benchmarks.
- 😀 The performance-to-cost ratio of Gemini 2.5 Pro is impressive, especially for tasks such as PhD-level question answering.
- 😀 For complex coding tasks, GPT-3 and its derivatives are still considered the new standard, though Gemini 2.5 Pro shows promise.
- 😀 OpenAI's latest models excel at agentic workflows, using tools effectively to enhance their performance in coding tasks.
- 😀 While OpenAI’s models perform well in coding, they still make notable mistakes, pointing out that they are not yet at AGI levels.
- 😀 Code generation capabilities in OpenAI models are top-tier, and they perform exceptionally well in available benchmarks for coding tasks.
- 😀 The use of tools and reasoning models in OpenAI’s latest models is a key development, marking a significant step forward in AI capabilities.
- 😀 The video creator encourages viewers to share their experiences with the new models and provide feedback on their performance in real-world applications.
Q & A
What are the main strengths of OpenAI's latest models, especially in terms of their performance on coding tasks?
-The latest OpenAI models excel in coding, with state-of-the-art performance in benchmarks. They can handle complex coding tasks, offer useful UI design assistance, and perform agentic reasoning in workflows. Their ability to use tools for multi-step tasks and iterating on solutions is a standout feature, though they are still prone to some mistakes.
How does the performance of Gemini 2.5 Pro compare to OpenAI's models in coding-related benchmarks?
-While Gemini 2.5 Pro performs relatively well in various benchmarks, OpenAI's models such as GPT-4 mini demonstrate a better cost-to-performance ratio. OpenAI models tend to outperform Gemini 2.5 Pro in terms of PhD-level question answering and some other general tasks, but Gemini remains more cost-efficient.
What role does cost play in the comparison between Gemini 2.5 Pro and OpenAI's models?
-Cost plays a significant role in comparing the two models. Gemini 2.5 Pro offers similar performance to OpenAI's models in some areas but at a much lower cost, especially in tasks like PhD-level question answering (GPQA). However, OpenAI's models, such as the GPT-4 mini, deliver better value in terms of cost for performance on specific tasks.
What are some of the key benchmarks used to evaluate OpenAI’s models and Gemini 2.5 Pro?
-Key benchmarks include the Humanities last exam, multi-challenge benchmarks, and the GPQA (PhD-level question answering) tests. These benchmarks provide insights into how each model handles complex text-based tasks and compares in terms of raw performance and cost-effectiveness.
What limitations do the OpenAI models have, despite their advanced capabilities?
-Despite their impressive capabilities, OpenAI's models are not yet close to achieving AGI. They still make basic mistakes in coding and have limitations in understanding or solving edge cases. While their tool usage and agentic workflows are advanced, they are not perfect and need further improvement.
What makes OpenAI's models stand out when it comes to using tools for agentic workflows?
-OpenAI’s models excel in using tools for agentic workflows by chaining together tasks in a multi-step process. This allows the models to reason through complex tasks and act on them step by step, a feature not commonly seen in other models, especially in coding and problem-solving scenarios.
How do the OpenAI models perform in specific coding tasks compared to previous versions?
-OpenAI's latest models perform better in coding tasks than previous versions, offering state-of-the-art results in coding benchmarks. However, while they excel in most areas, they still make occasional errors, particularly in complex coding scenarios, which prevents them from being considered as fully reliable AGI systems.
What is the significance of multi-step reasoning in the context of OpenAI’s latest models?
-Multi-step reasoning is crucial for complex tasks, allowing the models to break down problems into smaller, manageable parts and solve them sequentially. This capability enhances their ability to tackle tasks that require deeper understanding and iterative problem-solving, which is important for both coding and general AI applications.
How does the cost efficiency of Gemini 2.5 Pro impact its use for general users?
-The cost efficiency of Gemini 2.5 Pro makes it an attractive option for general users, especially for tasks where raw performance is not as critical as budget constraints. The model performs well on specific tasks like GPQA at a much lower cost compared to OpenAI’s higher-cost models, making it a good option for those looking for value in general applications.
What are the future possibilities for improving OpenAI's models based on the current benchmarks and feedback?
-Future improvements for OpenAI’s models may include reducing errors in coding, refining their tool usage for more advanced workflows, and improving general reasoning abilities. Additionally, addressing edge cases and refining performance in cost-effective ways will help bring these models closer to AGI, making them more reliable and capable for a wider range of tasks.
Outlines
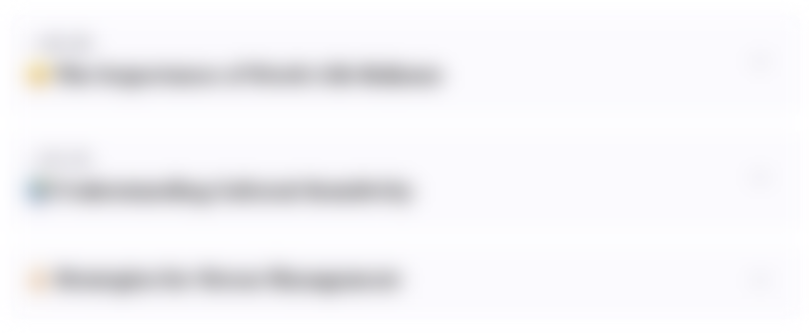
This section is available to paid users only. Please upgrade to access this part.
Upgrade NowMindmap
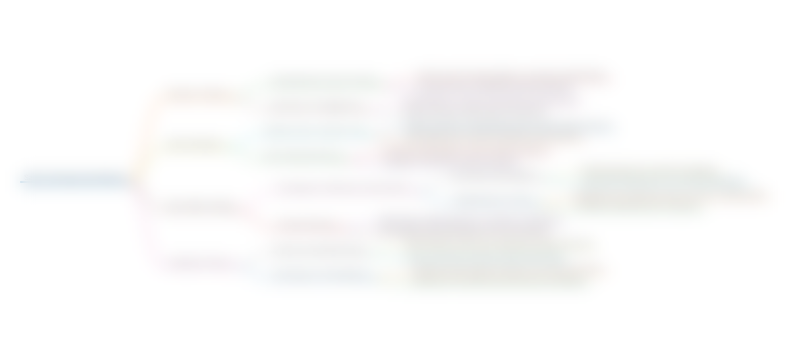
This section is available to paid users only. Please upgrade to access this part.
Upgrade NowKeywords
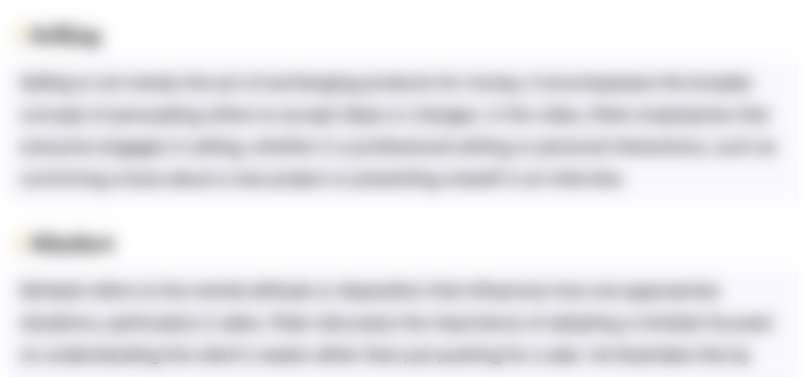
This section is available to paid users only. Please upgrade to access this part.
Upgrade NowHighlights
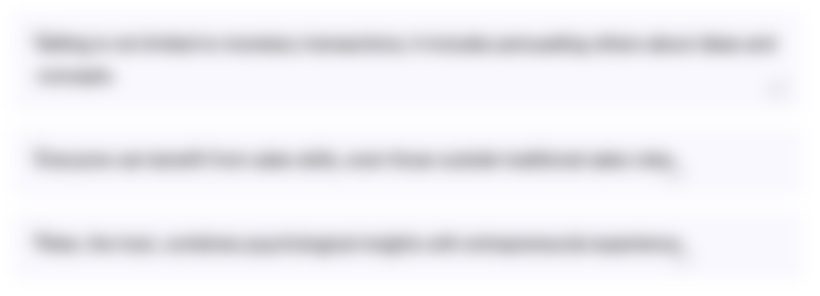
This section is available to paid users only. Please upgrade to access this part.
Upgrade NowTranscripts
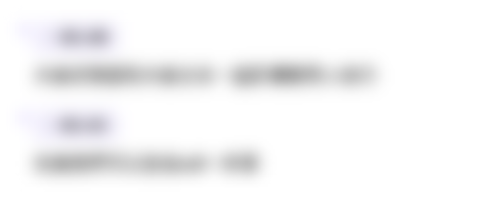
This section is available to paid users only. Please upgrade to access this part.
Upgrade NowBrowse More Related Video
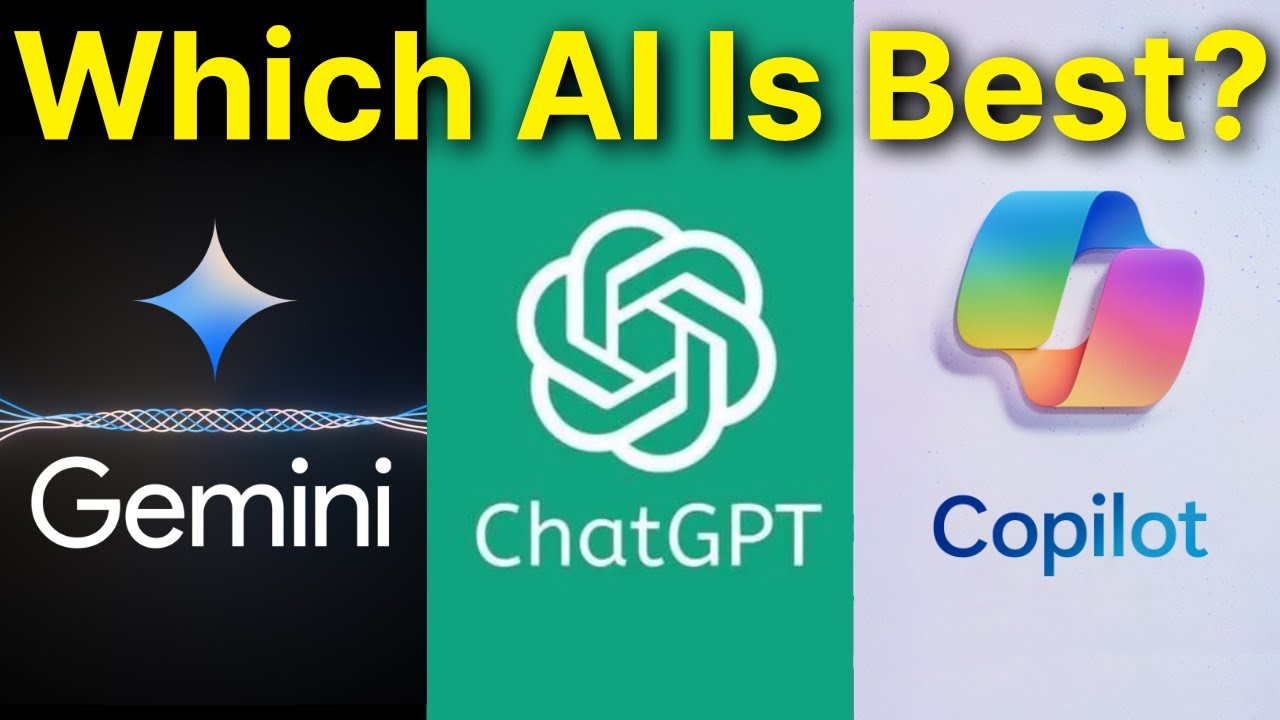
The Best AI In 2024 Is Not What You Think: Chat GPT Vs Google Gemini Vs Copilot

Ranking: Which LLMs are the BEST FOR 2025? (Ranking Every LLM Released in 2024!)

Google Gemini vs Google Assistant - Which One Is Better? (Tested On The Pixel 8 Pro)
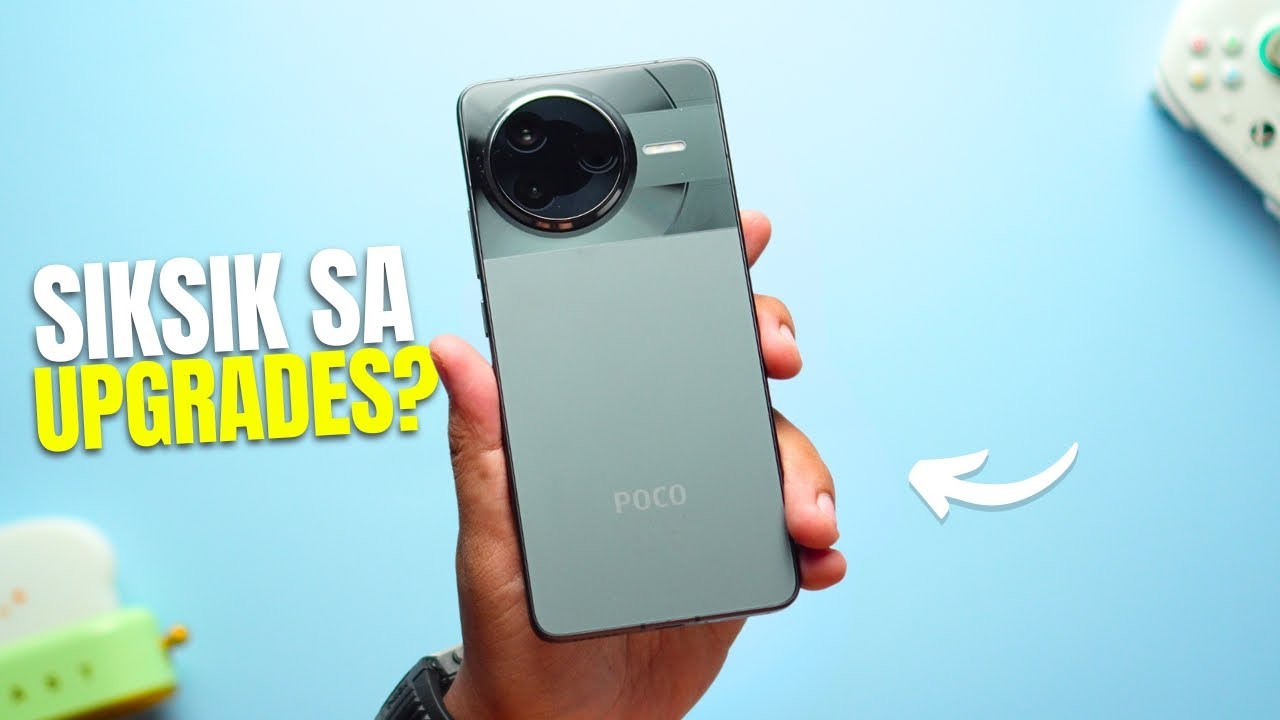
POCO F7 PRO: TAMA lang na NAG-ANTAY Ka Para DITO!!
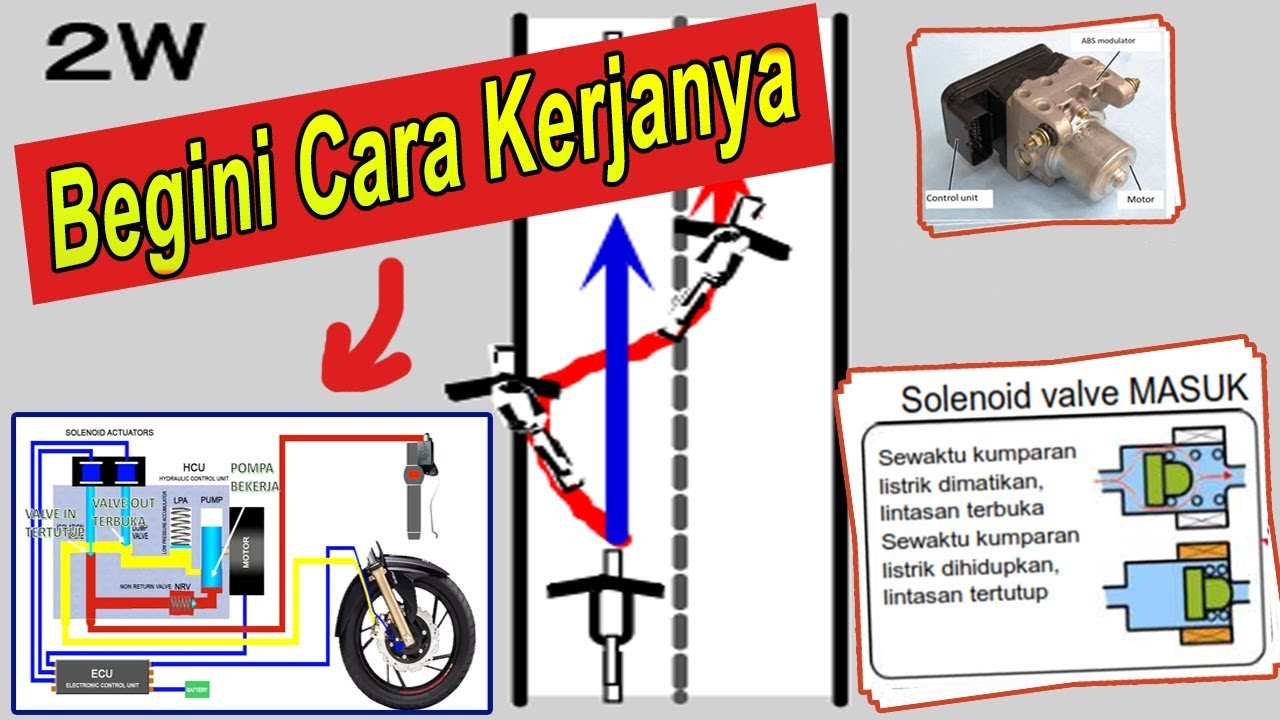
Cara Kerja Rem ABS Sepeda Motor
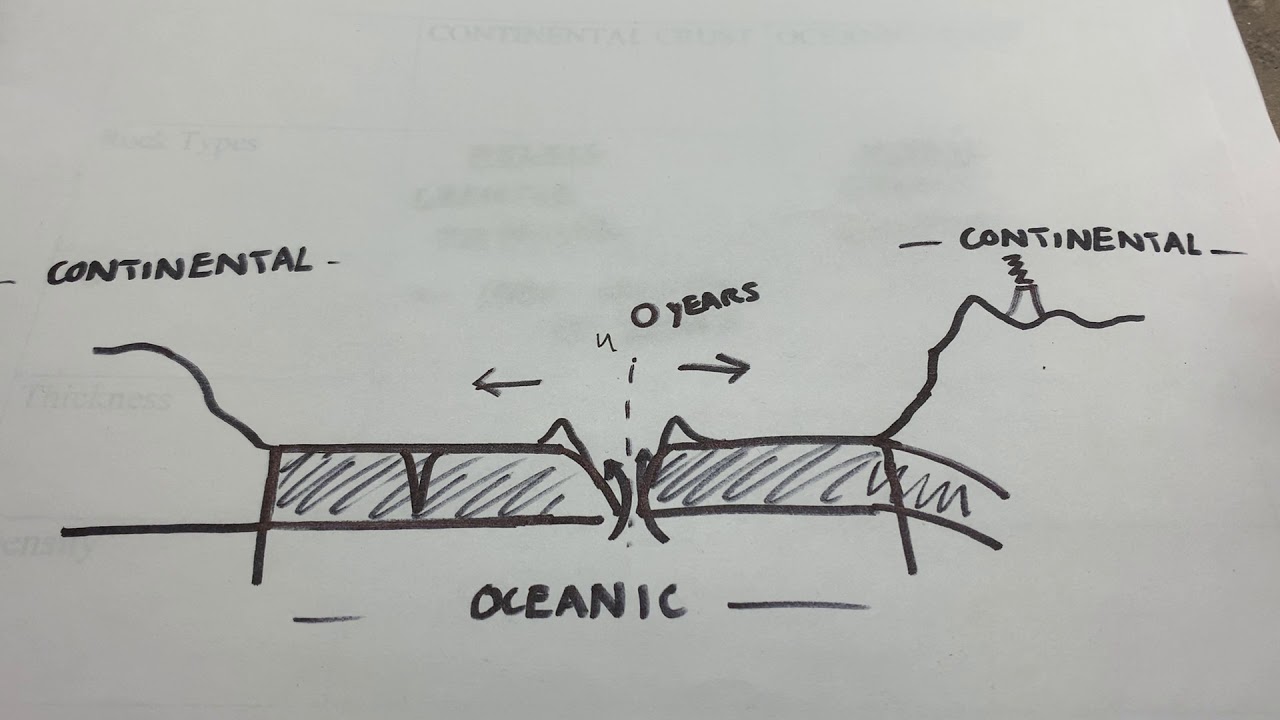
Continental Crust vs Oceanic Crust
5.0 / 5 (0 votes)