What Makes Behavox's LLM Different From Any Other AI Tool?
Summary
TLDRThe video discusses the key differences between specialized large language models (SLLMs), like Behav LLM 2.0, and general-purpose models such as GPT-4. Behav LLM 2.0 is designed for specific industries like compliance and financial services, offering more accurate responses in these areas but lacking general knowledge. The transcript highlights the challenges faced in developing a specialized AI, including sourcing high-quality data, overcoming hallucinations in general models, and the proprietary nature of training data. The video also hints at future API access for clients to fine-tune the model for their own use.
Takeaways
- π The Behav LLM 2.0 is a specialized large language model (SLLM), unlike general models like GPT-4 or Gemini, which are designed to cover a wide range of topics.
- π General AI models like GPT-4 are compared to sailboats, designed to handle many topics but prone to hallucinations as they dive deeper into specialized content.
- π Behav LLM 2.0 sacrifices general knowledge in favor of specializing in high-value areas like compliance and financial services.
- π GPT-4 includes a variety of data, such as Wikipedia, books, and code, but also includes uncurated data like Reddit discussions, which can lead to unreliable outputs.
- π One of the biggest challenges with general AI models like GPT-4 is the presence of hallucinations, especially when the model handles highly specialized topics like compliance.
- π Behav 2.0's training process involved building an LLM from scratch, using specialized data sets from equity research, regulatory websites, and financial textbooks.
- π Behav LLM 2.0 uses a structured, organized data set, whereas GPT-4βs training data is proprietary and cannot be validated or accessed by users.
- π The cost of acquiring specialized data sets for training large language models can be extremely high, sometimes reaching millions of dollars.
- π Behav LLM 2.0 benefits from data shared by customers, particularly in niche languages like Danish, which have smaller, less accessible data sets.
- π Behav is focusing on providing API access to its LLM next year, enabling users to fine-tune and integrate it into their own applications for specialized tasks like compliance and financial services.
Q & A
What is the key difference between Behav LLM 2.0 and GPT-4?
-Behav LLM 2.0 is a specialized large language model (SLLM) built for specific industries like compliance and financial services. In contrast, GPT-4 is a general-purpose model that covers a broad range of topics but is prone to hallucination and inaccuracies in specialized areas.
Why are general models like GPT-4 compared to sailboats?
-General models like GPT-4 are compared to sailboats because they are versatile and can handle a variety of topics. However, they are not highly specialized in any one area and may struggle with accuracy when diving deep into niche subjects.
What are the risks associated with using general models like GPT-4 for specialized tasks?
-The risks include hallucinations, where the model provides incorrect or fabricated information, especially when dealing with specialized topics like compliance or finance. General models also rely on data sources like Reddit, where unqualified users can provide misleading information.
What makes Behav LLM 2.0 more suitable for compliance tasks?
-Behav LLM 2.0 is designed with highly curated data, including equity research, regulatory websites, and financial textbooks. This specialization ensures that the model provides accurate and reliable information tailored for compliance tasks, making it more trustworthy in regulated environments.
How does Behav LLM 2.0 handle its data differently from GPT-4?
-Behav LLM 2.0 organizes its training data in a transparent and neat way, allowing users to view the data. In contrast, GPT-4's training data is proprietary and not shared, preventing users from validating its accuracy or understanding its sources.
What challenges did Behav face while developing its LLM?
-Behav faced significant challenges in acquiring large, specialized data sets, which required investment and partnerships. Additionally, the infrastructure needed to process and store vast amounts of data was costly, and there were difficulties in sourcing data from customers who were hesitant to share their sensitive information.
How did Behav acquire the data used to train its model?
-Behav acquired its data through partnerships with customers who donated their data, as well as purchasing specialized materials like textbooks and training materials from organizations that train analysts. This data set includes equity research, regulatory sites, and financial texts.
What role does customer data play in Behavβs AI development?
-Customer data is crucial for Behavβs AI development, especially in improving language capabilities for niche areas like Danish or specialized compliance tasks. However, the data is encrypted and kept behind secure systems, with the company needing to request permission from customers to use it for training purposes.
Why is the Reddit dataset considered problematic for GPT-4?
-The Reddit dataset is problematic because it contains a large volume of user-generated content, which often lacks expertise, particularly in specialized fields like investments. This leads to inaccuracies in the modelβs responses, as it cannot verify the credentials of users contributing information.
What are the future plans for Behav LLM 2.0?
-Behav plans to offer API access to its model next year, allowing users to fine-tune it for their specific applications. This will enable industries outside of compliance, such as finance and front-office operations, to leverage the specialized model for their needs.
Outlines
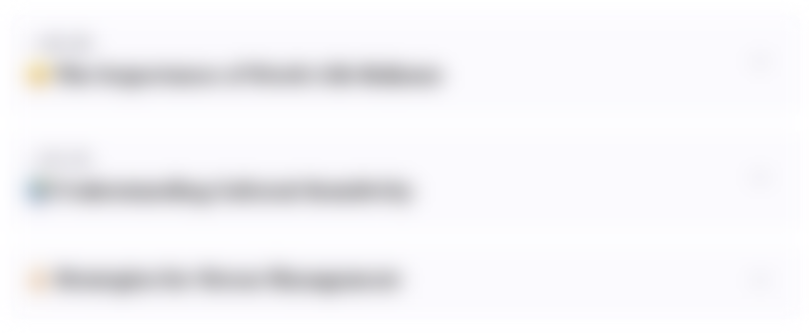
This section is available to paid users only. Please upgrade to access this part.
Upgrade NowMindmap
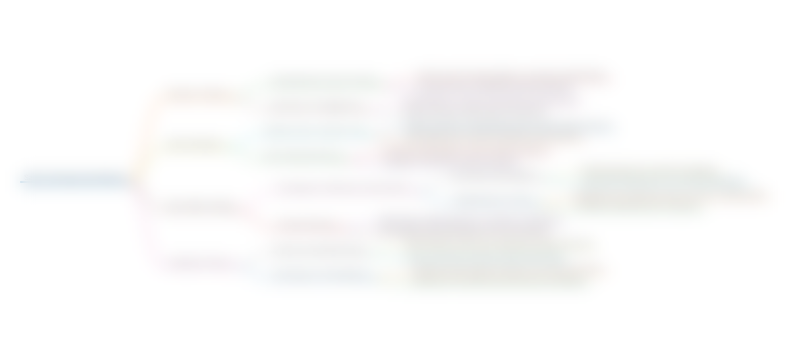
This section is available to paid users only. Please upgrade to access this part.
Upgrade NowKeywords
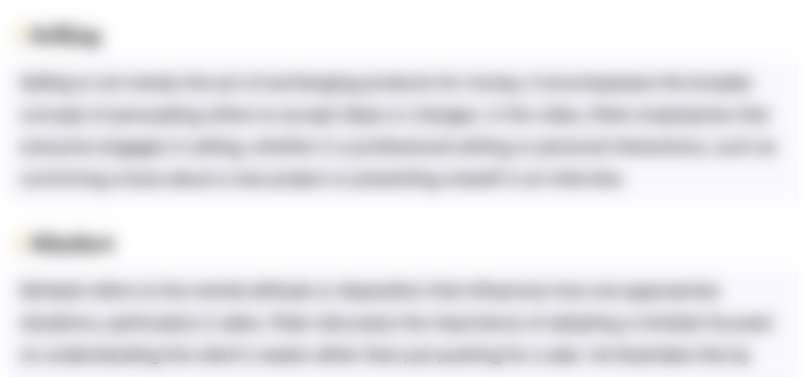
This section is available to paid users only. Please upgrade to access this part.
Upgrade NowHighlights
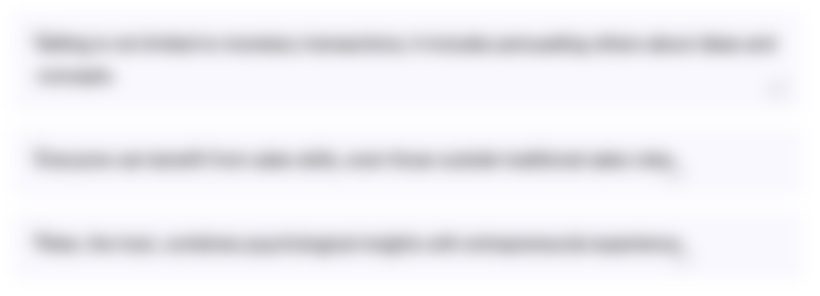
This section is available to paid users only. Please upgrade to access this part.
Upgrade NowTranscripts
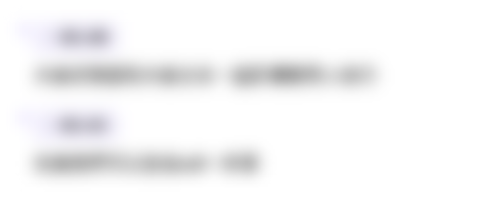
This section is available to paid users only. Please upgrade to access this part.
Upgrade NowBrowse More Related Video
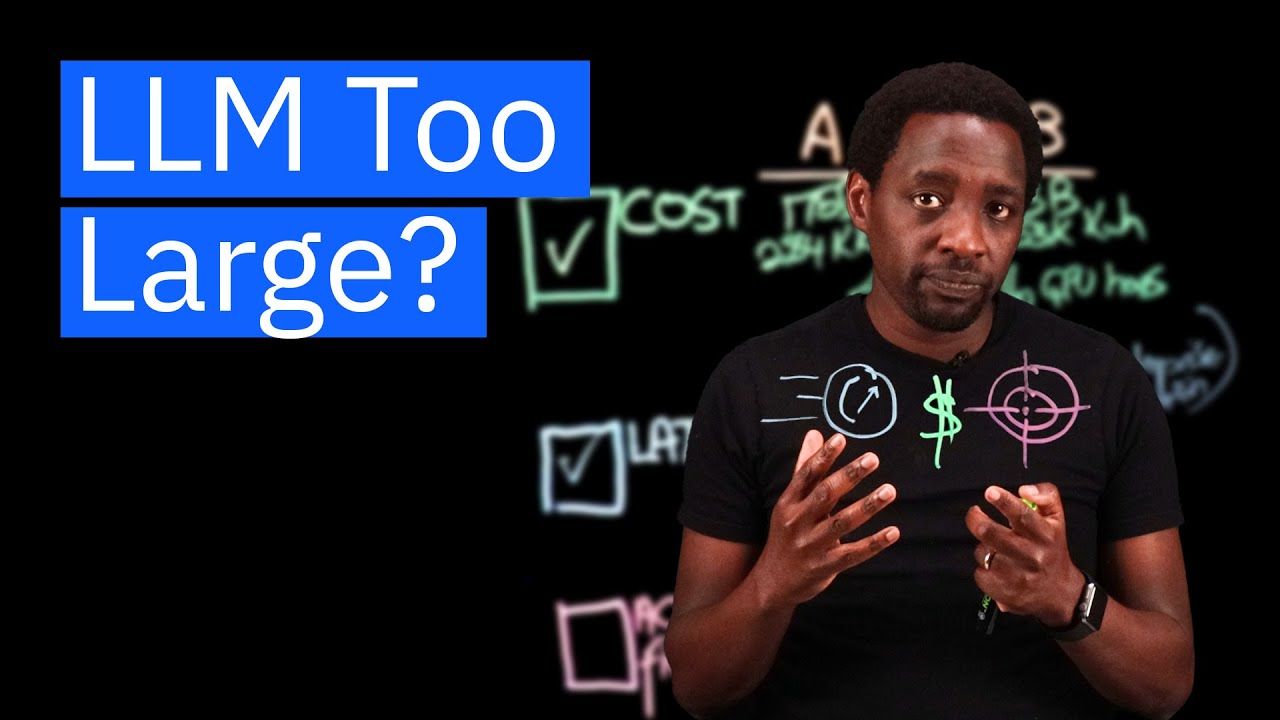
Large Language Models: How Large is Large Enough?
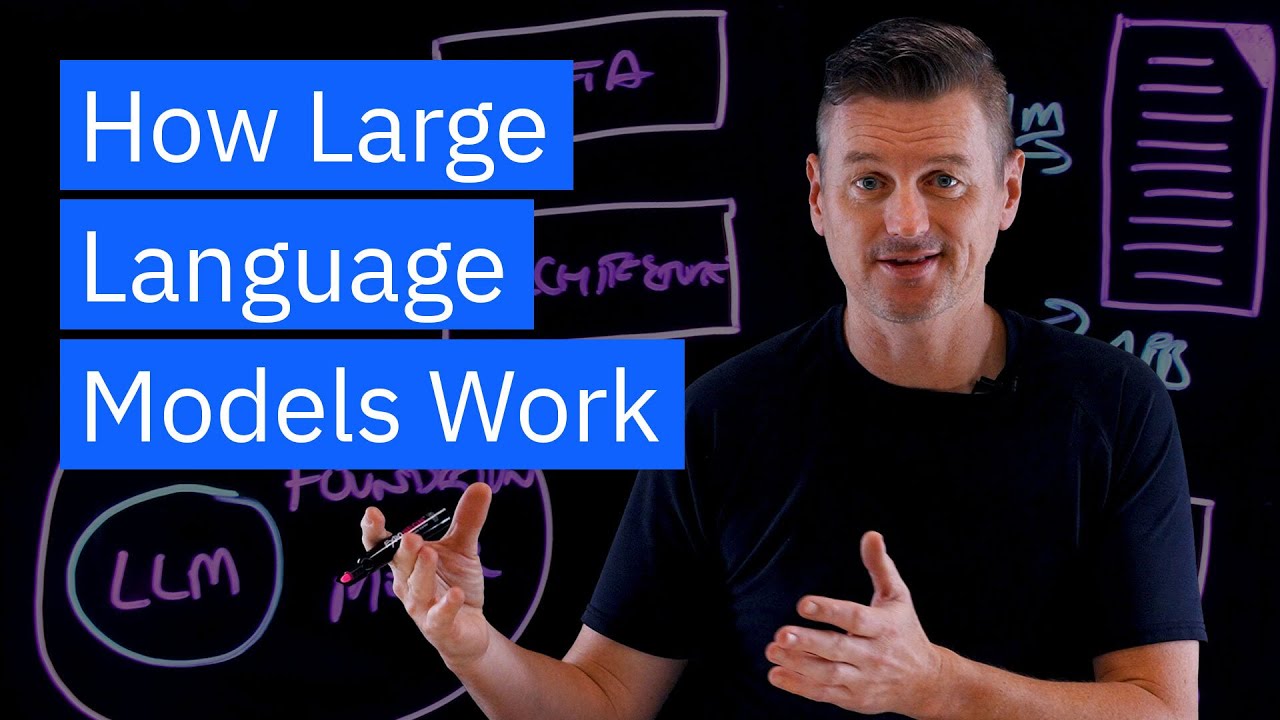
How Large Language Models Work

Should You Use Open Source Large Language Models?
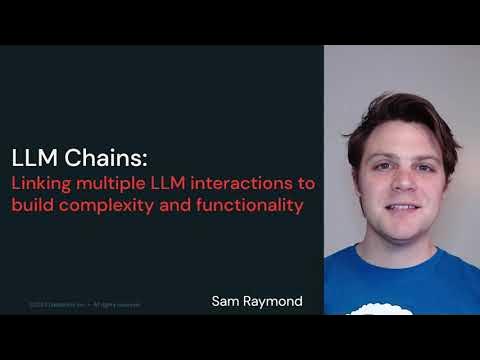
LLM Module 3 - Multi-stage Reasoning | 3.4 LLM Chains
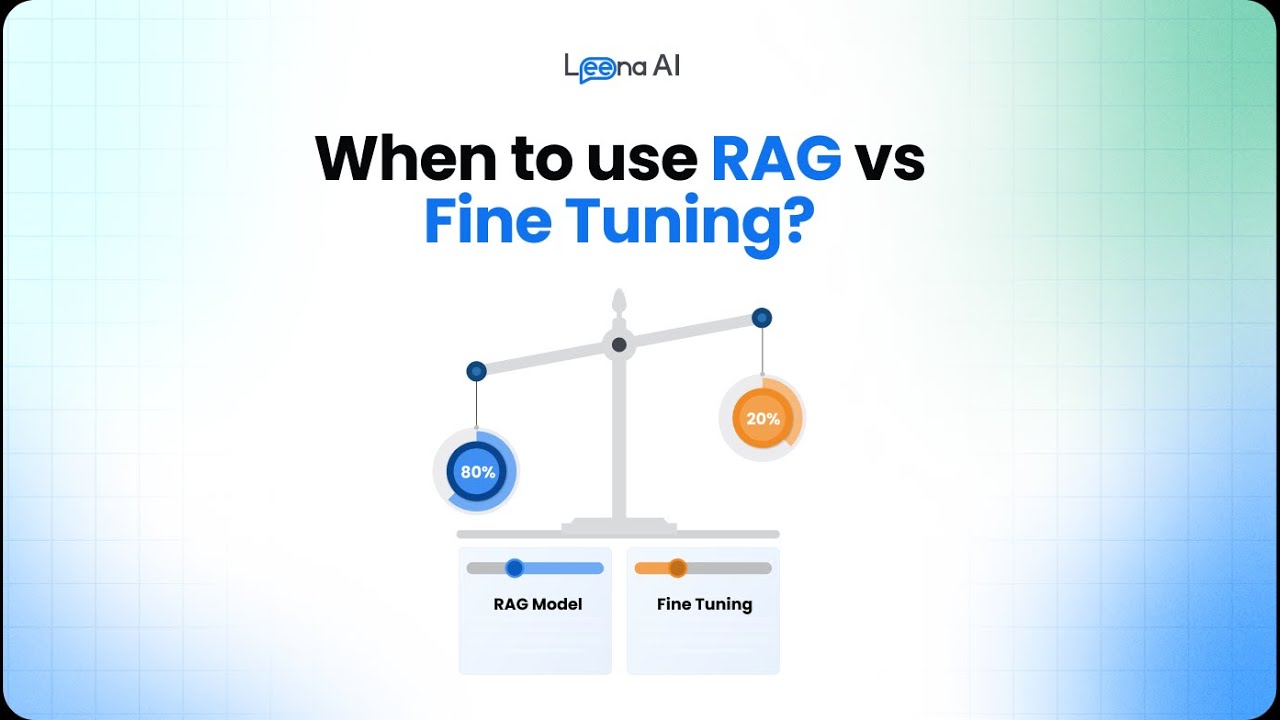
Generative AI 101: When to use RAG vs Fine Tuning?
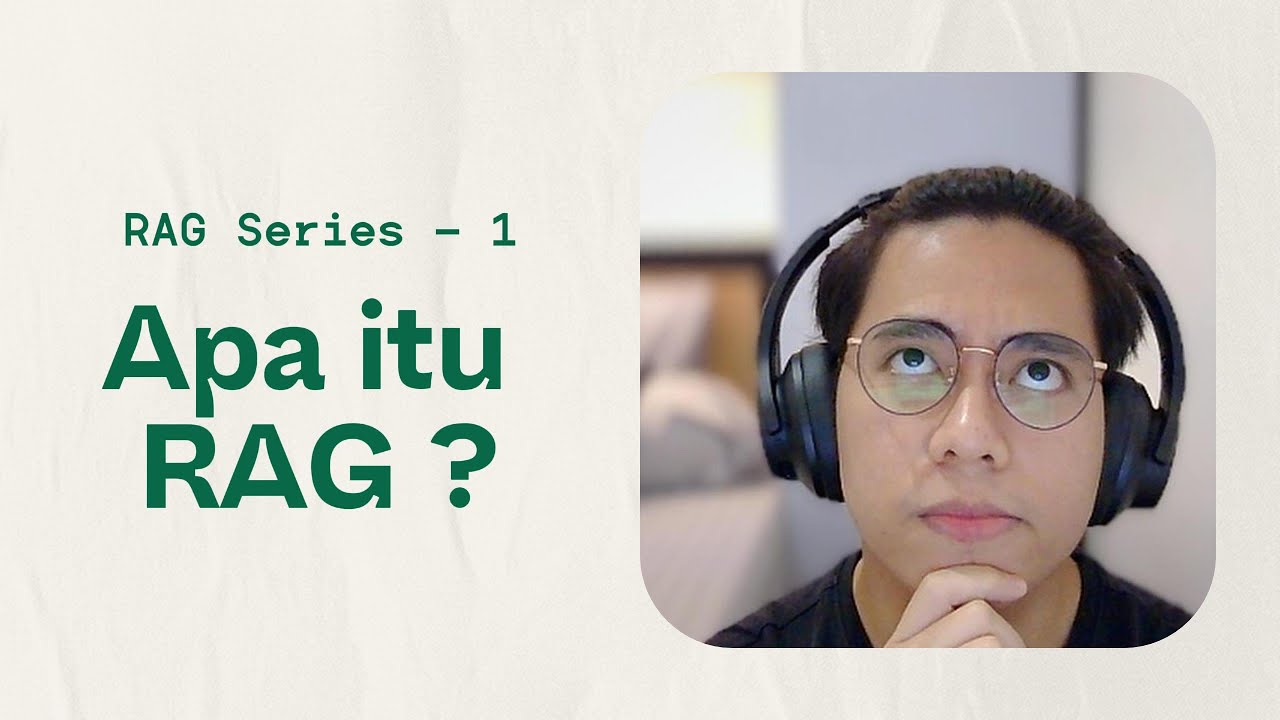
[RAG Series #1] Hanya 10 menit paham bagaimana konsep dibalik Retrieval Augmented Generation (RAG)
5.0 / 5 (0 votes)