Pertemuan 1 - Data Science
Summary
TLDRIn this Data Science course introduction, the lecturer, Mega Bagus Lambang I, outlines the course structure, focusing on assignments, a midterm, and a project-based learning approach. Data science is described as a multidisciplinary field, combining mathematics, statistics, and domain expertise. The lecture highlights the difference between data science and data analytics, emphasizing the predictive capabilities of data science. The importance of understanding and processing big data—characterized by volume, velocity, variety, and veracity—is stressed, along with its role in gaining competitive business advantages and making informed decisions.
Takeaways
- 😀 Data science is a multidisciplinary field that combines mathematics, statistics, and domain knowledge to extract insights and make informed decisions.
- 😀 The course grading consists of three main components: assignments (35%), mid-term exams (25%), and a project (40%) where students work in groups of 3 to 5.
- 😀 A data scientist needs to understand three key areas: mathematics/statistics, hacking skills (data manipulation), and domain expertise (understanding the context of the data).
- 😀 Machine learning is at the intersection of mathematics/statistics and hacking skills, serving as a core aspect of data science.
- 😀 Data science is distinct from data analytics: data science involves data processing, decision-making, and prediction, while data analytics mainly focuses on providing summaries and insights.
- 😀 The course will explore the application of data science through projects, such as analyzing student data (e.g., graduation rates, challenges faced in specific courses).
- 😀 Data science is critical because it allows companies to process vast amounts of data and extract meaningful insights, leading to better business decisions.
- 😀 Big data, which includes massive datasets exceeding 1 petabyte, requires specialized tools and techniques to manage and analyze, as it's beyond the capabilities of standard computers.
- 😀 Big data is characterized by 4 V's: Volume (large data size), Velocity (data generated rapidly), Variety (different types of data), and Veracity (data quality and accuracy).
- 😀 Companies that can effectively process big data gain a competitive edge, enabling them to make better decisions and lead in their industries.
- 😀 A key to effective decision-making is moving from raw data to processed information, allowing for more informed and lower-risk business choices.
Q & A
What are the three main components for grading in this Data Science course?
-The three main components are: Assignments (35%), Mid-term Exam (25%), and the Final Project (40%).
What is the primary focus of Data Science?
-Data Science is a multidisciplinary field that combines mathematics, statistics, and domain knowledge to extract insights from data and support decision-making.
What are the three key areas necessary to become a skilled Data Scientist?
-The three key areas are: Mathematics & Statistics, Hacking Skills (technical proficiency with data), and Domain Expertise (understanding the context of the data being analyzed).
How does Data Science differ from Data Analytics?
-Data Science goes beyond analyzing data by focusing on prediction and decision-making, whereas Data Analytics primarily involves summarizing and visualizing data.
What are the 4 V's that characterize Big Data?
-The 4 V's of Big Data are Volume (large data size), Velocity (speed of data generation), Variety (different types of data), and Veracity (data quality and reliability).
Why is it important to understand Domain Expertise in Data Science?
-Domain Expertise is important because understanding the specific context of the data helps ensure accurate analysis and relevant insights, especially when dealing with specialized areas like healthcare or finance.
What does the final project in this course involve?
-The final project involves applying Data Science skills to solve a real-world problem. Students will work in groups to analyze data, gain insights, and present their findings.
How does Big Data differ from smaller datasets like an Excel file?
-Big Data is much larger in scale, often exceeding 1 petabyte, and requires specialized tools and infrastructure to process. In contrast, smaller datasets like an Excel file can be managed with conventional software.
What role does Machine Learning play in Data Science?
-Machine Learning combines statistics and technical data manipulation skills to build models that can predict outcomes and analyze large datasets, forming the core of many Data Science applications.
How can Data Science impact decision-making in a company?
-Data Science helps companies by analyzing data to uncover patterns, optimize processes, and predict future trends, ultimately enabling more informed and effective decision-making.
Outlines
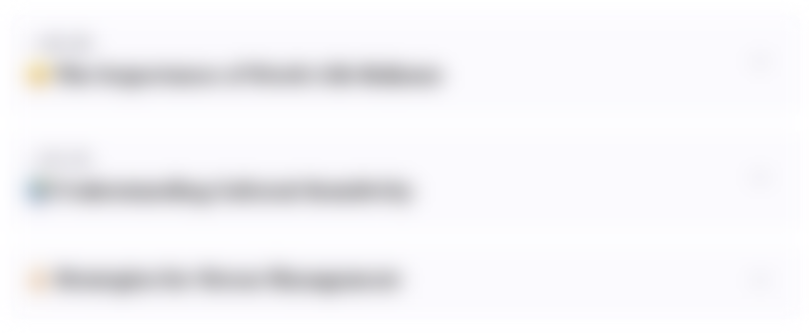
This section is available to paid users only. Please upgrade to access this part.
Upgrade NowMindmap
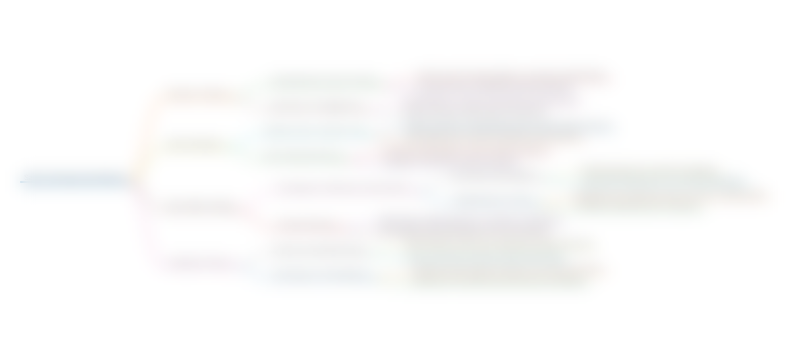
This section is available to paid users only. Please upgrade to access this part.
Upgrade NowKeywords
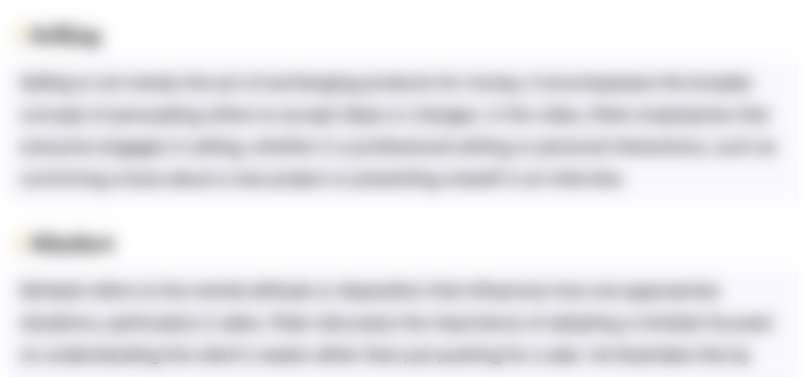
This section is available to paid users only. Please upgrade to access this part.
Upgrade NowHighlights
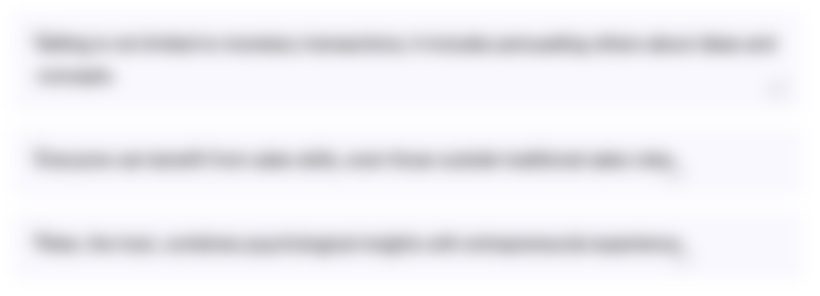
This section is available to paid users only. Please upgrade to access this part.
Upgrade NowTranscripts
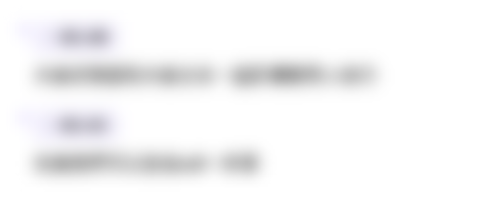
This section is available to paid users only. Please upgrade to access this part.
Upgrade NowBrowse More Related Video

Pertemuan 1. Proses Manufaktur _ Pendahuluan
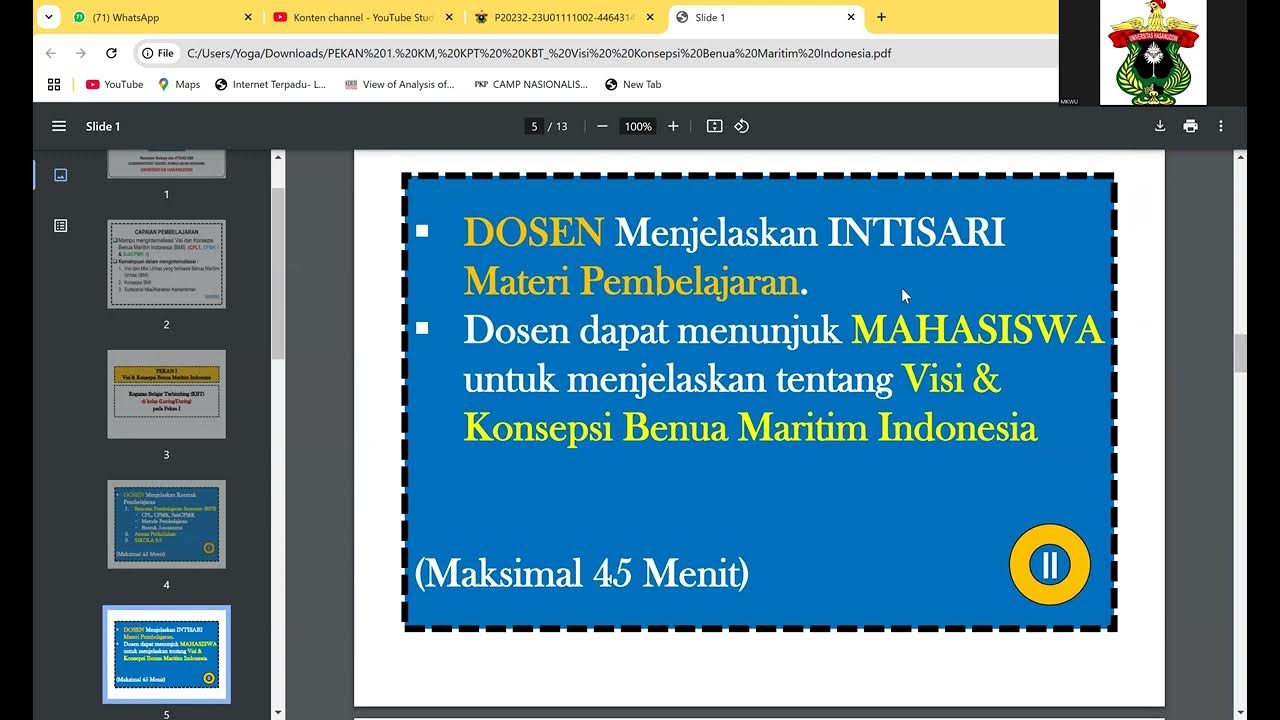
PEKAN I WBI BMI Video Teknis Kuliah Interaktif

PEKAN II WBI BMI Video Teknis Kuliah Interaktif
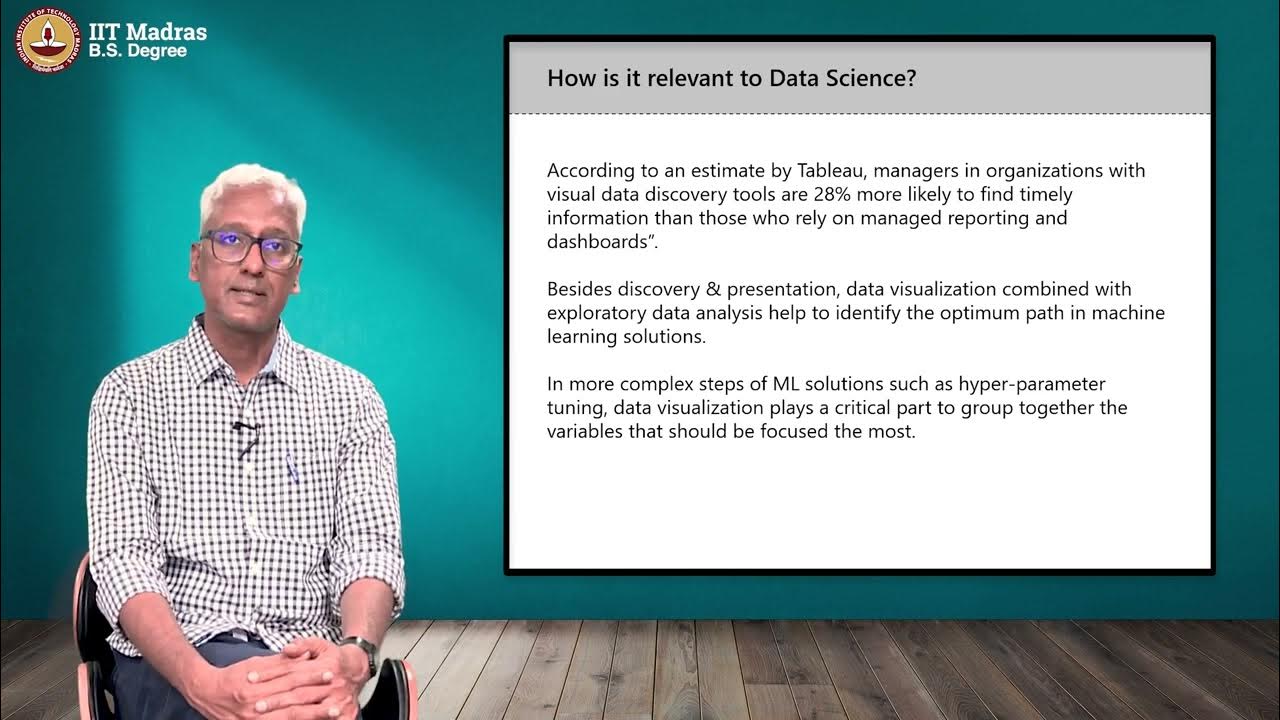
Introduction to Data Visualization - Intro
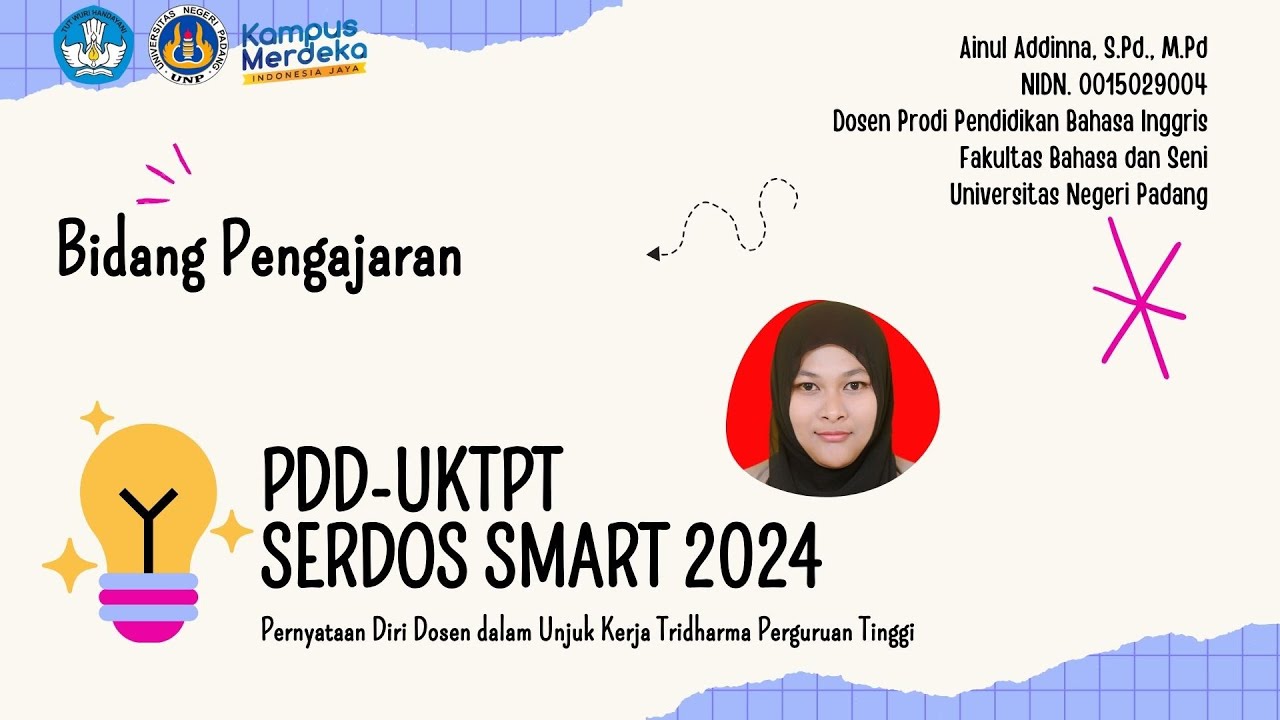
PDD UKTPT Bidang Pengajaran Ainul Addinna, S.Pd., M.Pd
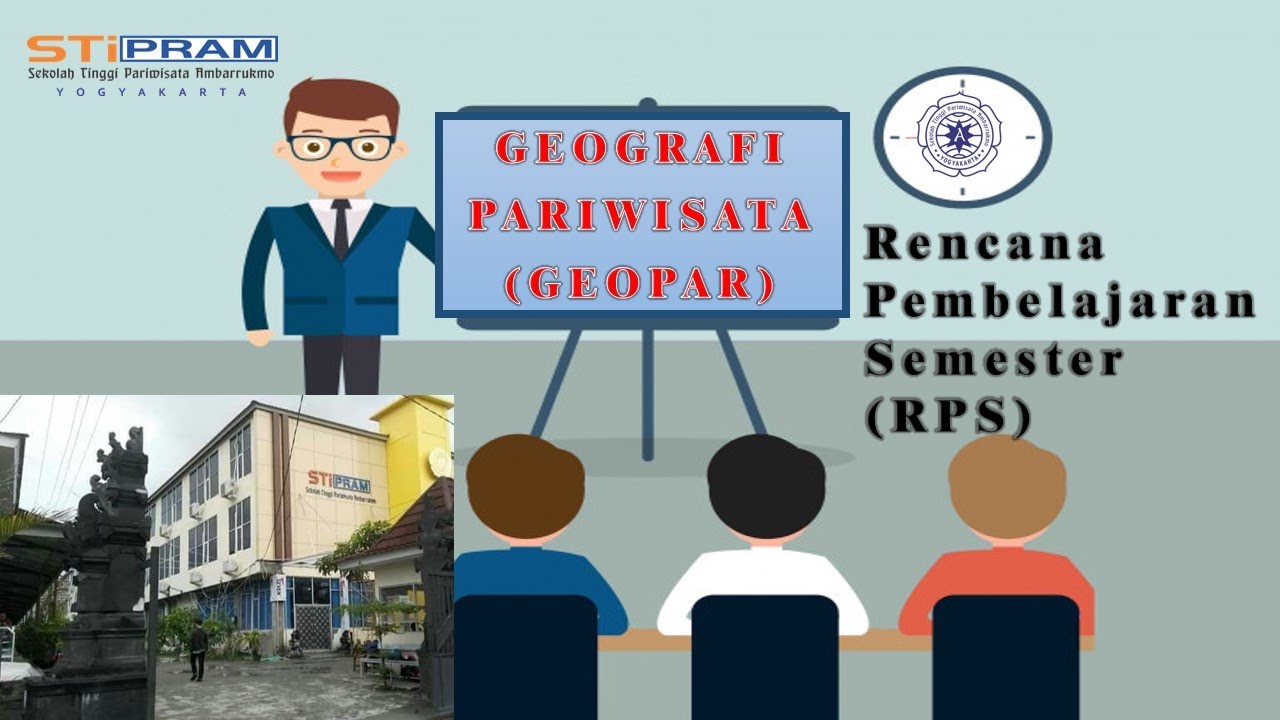
Penjelasan Awal Mata Kuliah Geografi Pariwisata (RPS) - STIPRAM Yogyakarta
5.0 / 5 (0 votes)