Wk 4 part5
Summary
TLDRThis video delves into the use of t-tests in statistical analysis, focusing on comparing population values using sample data. It highlights the three main types of t-tests: the single sample t-test, the two-sample t-test, and the paired t-test, with real-world applications such as monitoring environmental conditions or energy consumption. The script demonstrates how to analyze energy data, using the example of Western Australiaβs energy use compared to the national average, through a statistical t-test. The process of hypothesis testing, plotting, and interpreting results is emphasized to inform further discussions and policy decisions.
Takeaways
- π T-tests are used to compare population values, not sample values, to draw conclusions about the underlying populations.
- π There are three main types of t-tests: single sample t-test, two sample t-test, and paired t-test.
- π The single sample t-test compares a sample to a known population value, like monitoring energy consumption against a standard.
- π The two sample t-test compares two independent samples to determine if there is a significant difference between them.
- π The paired t-test compares two related samples, often in a before-and-after context, where there is a structural link between the samples.
- π Real-world examples of t-tests in use include environmental monitoring, energy consumption studies, and social science research.
- π A common application of a single sample t-test is comparing energy consumption in a specific region to a national average.
- π Energy consumption in households is influenced by factors like appliance use, heating, cooling, and geographical location.
- π When analyzing energy consumption, we can use t-tests to determine if a region's energy use significantly differs from a known average.
- π After conducting a t-test, the p-value is used to assess if the difference between sample and population values is statistically significant.
- π Itβs important to follow up statistical results with practical discussions, considering sample representativeness, sample size, and central limit theorem implications.
Q & A
What is the primary purpose of a t-test in statistical analysis?
-A t-test is used to compare sample data against population values in order to determine if there is a statistically significant difference. It helps to make inferences about population parameters based on sample statistics.
What are the three types of t-tests mentioned in the transcript?
-The three types of t-tests are: the single sample t-test, the two-sample t-test, and the paired t-test. Each type serves different purposes depending on the structure and relationship of the samples being compared.
How does a single sample t-test work in practice?
-A single sample t-test compares the mean of a sample to a known population mean to determine whether there is a significant difference. For example, it can be used to compare energy consumption in a specific area to the national average.
What distinguishes the two-sample t-test from other types of t-tests?
-The two-sample t-test compares two independent samples to determine whether there is a significant difference between them. It is commonly used in a broader range of applications compared to the single sample or paired t-tests.
What is the key feature of a paired t-test?
-The paired t-test is used when there is a structural relationship between the two samples being compared, such as before-and-after data or matched pairs. It examines the differences within pairs rather than comparing two independent groups.
In the context of energy consumption, why is the t-test relevant?
-The t-test is relevant to energy consumption studies as it allows for comparisons between observed data (e.g., energy usage in households) and expected values (e.g., national averages), helping to assess whether observed differences are statistically significant.
How is the null hypothesis formulated in a single sample t-test for energy use?
-In a single sample t-test for energy use, the null hypothesis is that the mean energy consumption in the sample is equal to the known national average, such as 16.5 kilojoules per day.
What does a p-value less than 0.05 indicate in a t-test?
-A p-value less than 0.05 indicates that the null hypothesis can be rejected, meaning there is a statistically significant difference between the sample mean and the population mean. In this case, it suggests that the energy consumption differs from the national average.
Why is it important to consider the sample size when interpreting t-test results?
-The sample size is important because a small sample might not represent the population accurately, leading to unreliable results. A larger sample size allows for a more robust application of statistical principles like the central limit theorem.
How does the central limit theorem relate to the interpretation of t-test results?
-The central limit theorem states that, for sufficiently large sample sizes, the sampling distribution of the sample mean will be approximately normally distributed. This allows for valid statistical inferences using the t-test, even if the original data distribution is not normal.
Outlines
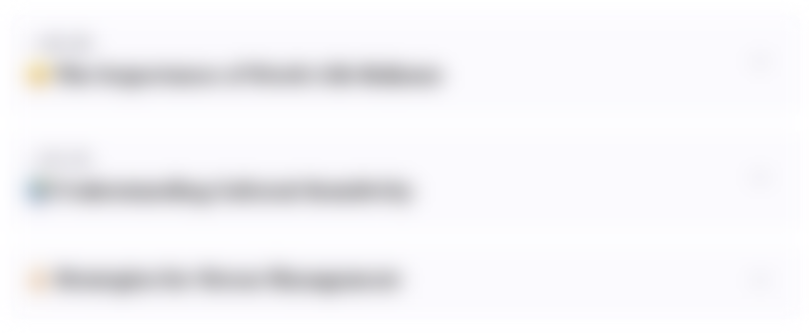
This section is available to paid users only. Please upgrade to access this part.
Upgrade NowMindmap
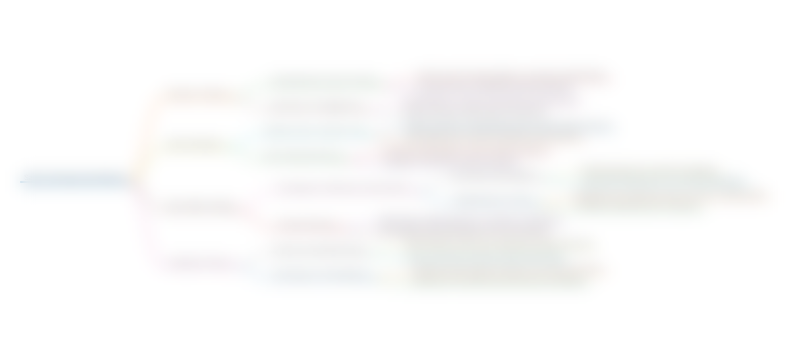
This section is available to paid users only. Please upgrade to access this part.
Upgrade NowKeywords
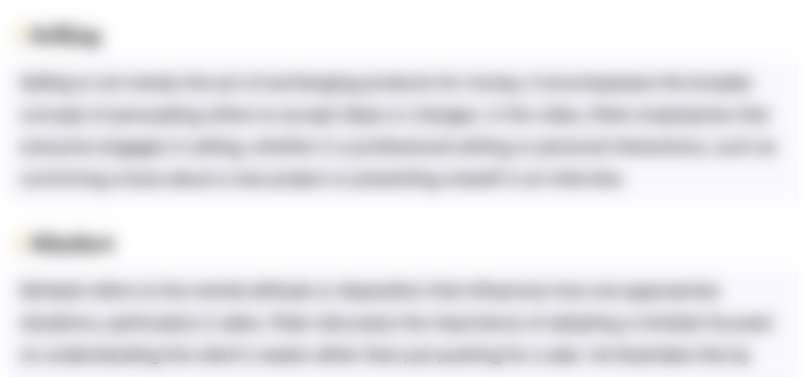
This section is available to paid users only. Please upgrade to access this part.
Upgrade NowHighlights
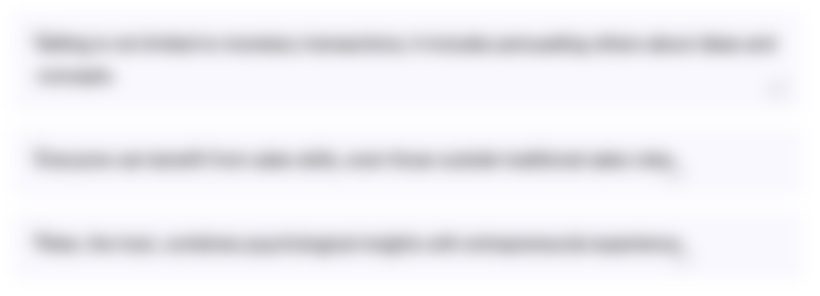
This section is available to paid users only. Please upgrade to access this part.
Upgrade NowTranscripts
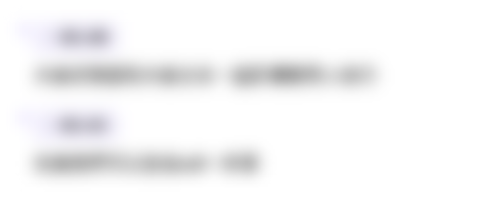
This section is available to paid users only. Please upgrade to access this part.
Upgrade Now5.0 / 5 (0 votes)