NEW: Nobody Expected Alex Karp to Say This
Summary
TLDRIn this discussion, the speakers explore the evolution of AI and its transformative impact on business. They highlight the importance of tackling complex problems to improve software performance and engineering culture. The conversation delves into the future of AI, focusing on its role in optimizing business functions, real-time decision-making, and portfolio management. The speakers emphasize the ongoing improvement of AI models, stressing the importance of practical applications over theoretical promises. They also acknowledge the societal challenges AI poses, especially regarding unequal benefits for those who implement it effectively. Ultimately, the conversation reveals a dynamic outlook on the integration of AI into various industries.
Takeaways
- 😀 Raising the complexity of challenges leads to more innovative and performant solutions in technology development.
- 😀 A higher standard of problem-solving results in the development of smarter and more autonomous systems.
- 😀 Intelligence is often measured using proxy indicators, such as IQ, but performance is the true indicator of success in technology.
- 😀 By improving the quality of models and technology, deployment friction decreases, making it easier to implement AI systems in core business functions.
- 😀 AI models are improving at an exponential rate, and the focus should be on deploying them effectively to maximize their value.
- 😀 A strong focus on data ingestion and optimizing capital allocation in real-time is essential for advancing AI in business.
- 😀 In the next two years, we will see more practical applications of AI as theoretical ideas give way to actionable solutions.
- 😀 The impact of AI on society may disproportionately benefit certain groups, potentially causing societal and investor challenges.
- 😀 The ability to deploy models more effectively increases as their autonomy grows, allowing businesses to better integrate AI into their operations.
- 😀 Focusing on results rather than theoretical promises is crucial when evaluating the effectiveness of AI applications and technology solutions.
- 😀 Continuous learning and adaptation are key in advancing AI and improving its real-world applications, as progress is often unpredictable.
Q & A
How does raising the complexity of a problem improve performance in AI development?
-By raising the complexity of a problem, engineers are pushed to develop better solutions, which ultimately improves the quality of the software. This approach forces the team to focus on harder challenges, making the underlying models more performant and fostering a culture of excellence within the organization.
What is the role of 'Nontology' in optimizing AI performance?
-Nontology is a tool that helps micro-control inputs, whether they come from humans or large language models. It ensures that inputs are optimized within an institutional context, which is essential for improving the performance and accuracy of AI models in real-world applications.
Why is it important to focus on fairness and integrity in AI development?
-Focusing on fairness and integrity helps build stronger, more reliable models. By embedding these values into the core of the problem-solving approach, organizations ensure that the solutions they develop are both ethical and effective, leading to better overall performance and greater societal trust.
What is the main challenge for enterprises when adopting new software or technology?
-The main challenge for enterprises is the high friction involved in adopting new technology. Even if a product has strong potential, it can be difficult for businesses to integrate it into their operations. The value proposition must be significantly better than what the enterprise can already do in-house.
How does the progression of AI models impact their deployment in real-world scenarios?
-As AI models improve, they become more autonomous and capable of handling complex tasks with less supervision. This reduces the need for extensive infrastructure to support them, making deployment easier and more efficient in core business functions. Improved models also allow for broader application and integration into various industries.
What is the expected trend in AI model development over the next few years?
-The trend is that AI models will continue to get better with increased autonomy, accuracy, and capability. This will lead to a reduction in deployment friction and an expansion of AI applications across different sectors, making AI more integral to business operations and financial decision-making.
What role does continual learning play in the development of AI models?
-Continual learning allows teams to adapt to the rapid advancements in AI technology. By learning from past implementations and mistakes, organizations can iterate quickly and refine their models to produce better results over time. This adaptability is crucial for staying competitive in an ever-evolving technological landscape.
What are the potential societal impacts of AI advancements?
-AI advancements could disproportionately benefit those who implement the technology effectively, leading to economic and social inequalities. While AI has the potential to uplift many sectors, those who are more skilled at deploying AI could gain a competitive advantage, raising concerns about societal and investor imbalances.
How can companies evaluate the true effectiveness of AI models?
-To evaluate the effectiveness of AI models, companies should focus on the actual results produced rather than the claims made by vendors. It's important to assess how well the AI performs in real-world scenarios and compare it to other models. This approach ensures that organizations prioritize practical outcomes over marketing or theoretical promises.
What does the speaker suggest as the best way to approach AI implementation?
-The speaker suggests starting with a minimal, practical implementation and using it to demonstrate its effectiveness. By showing results and gaining internal buy-in, the solution can be scaled and refined over time. This approach minimizes risk and ensures that the technology is truly beneficial before investing heavily.
Outlines
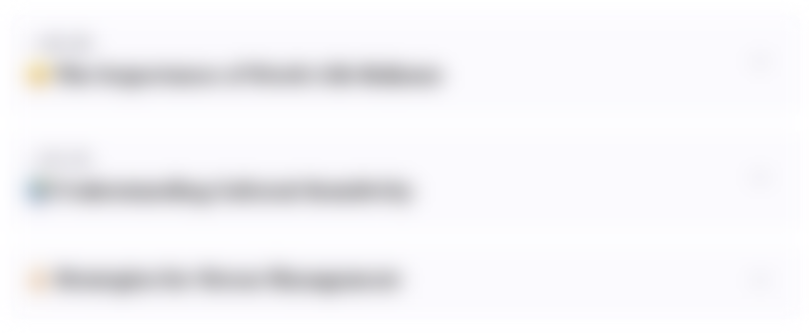
This section is available to paid users only. Please upgrade to access this part.
Upgrade NowMindmap
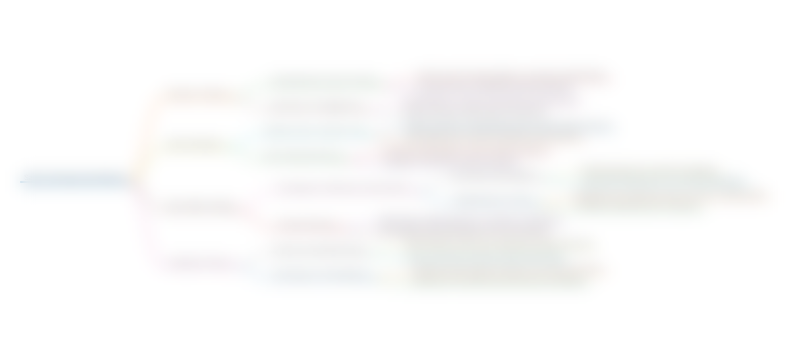
This section is available to paid users only. Please upgrade to access this part.
Upgrade NowKeywords
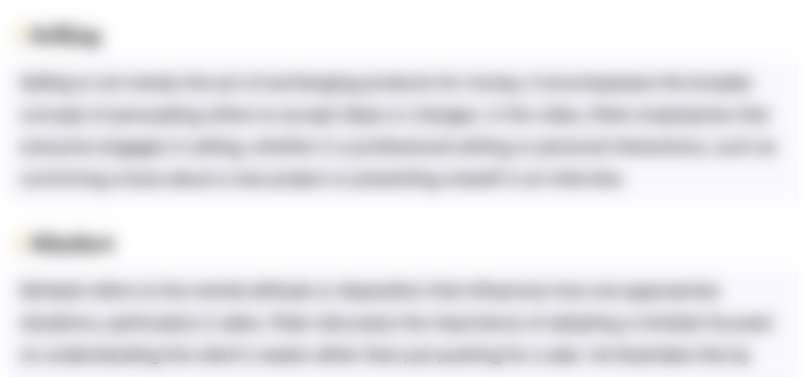
This section is available to paid users only. Please upgrade to access this part.
Upgrade NowHighlights
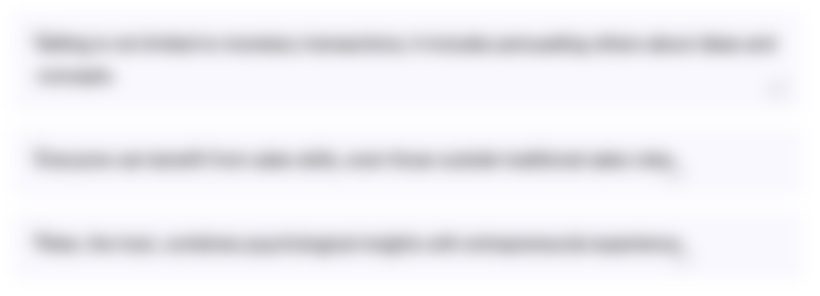
This section is available to paid users only. Please upgrade to access this part.
Upgrade NowTranscripts
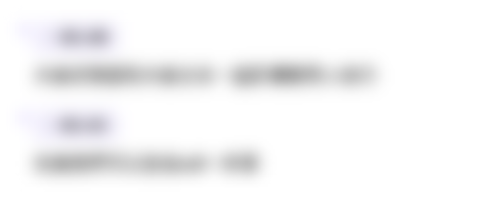
This section is available to paid users only. Please upgrade to access this part.
Upgrade NowBrowse More Related Video

The AI Future Has Arrived: Here's What You Should Do About It
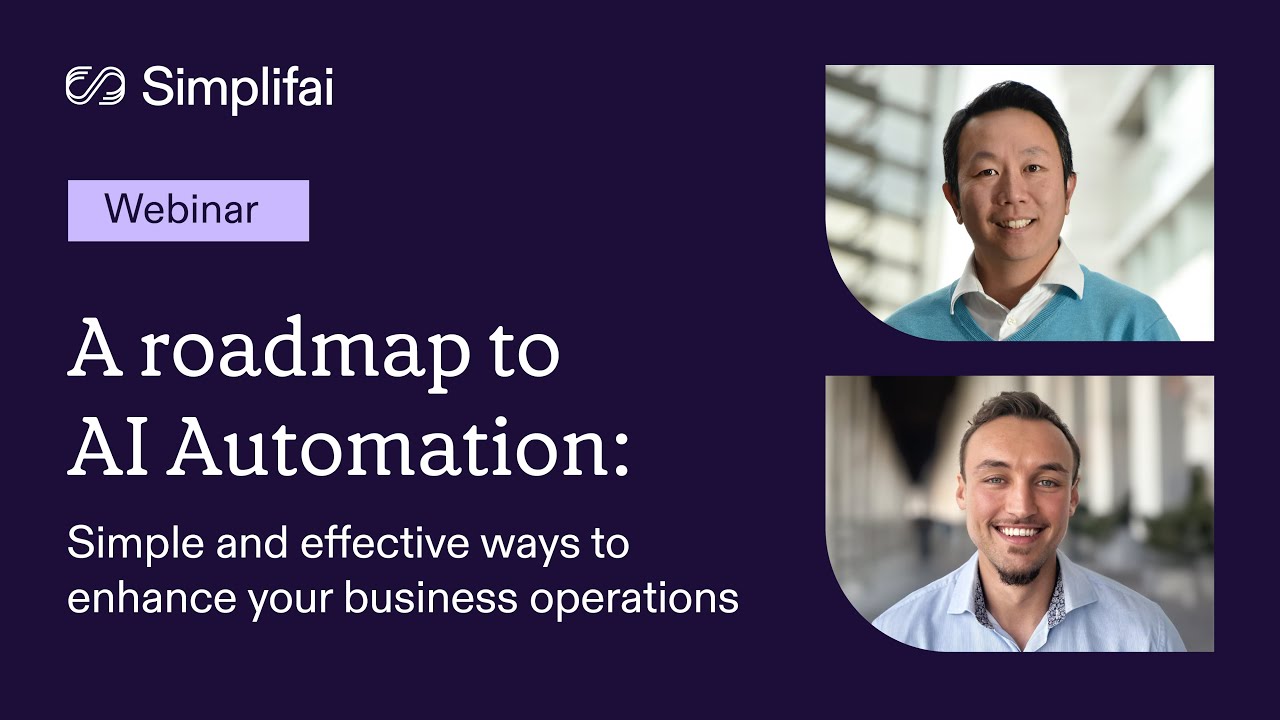
Webinar: A Roadmap to AI Automation
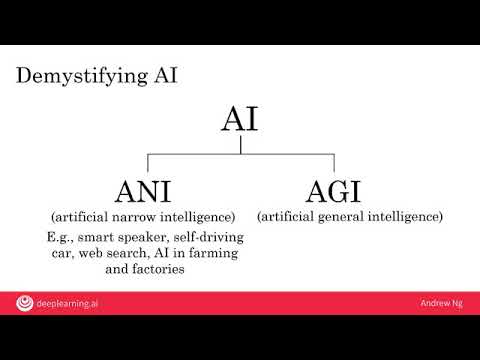
Week 1 - Video 1 - Introduction
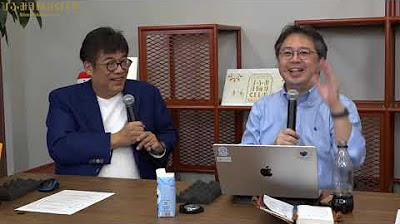
ひふみ目論見倶楽部【準備運動編】安宅和人さんと一緒に未来について考えました。
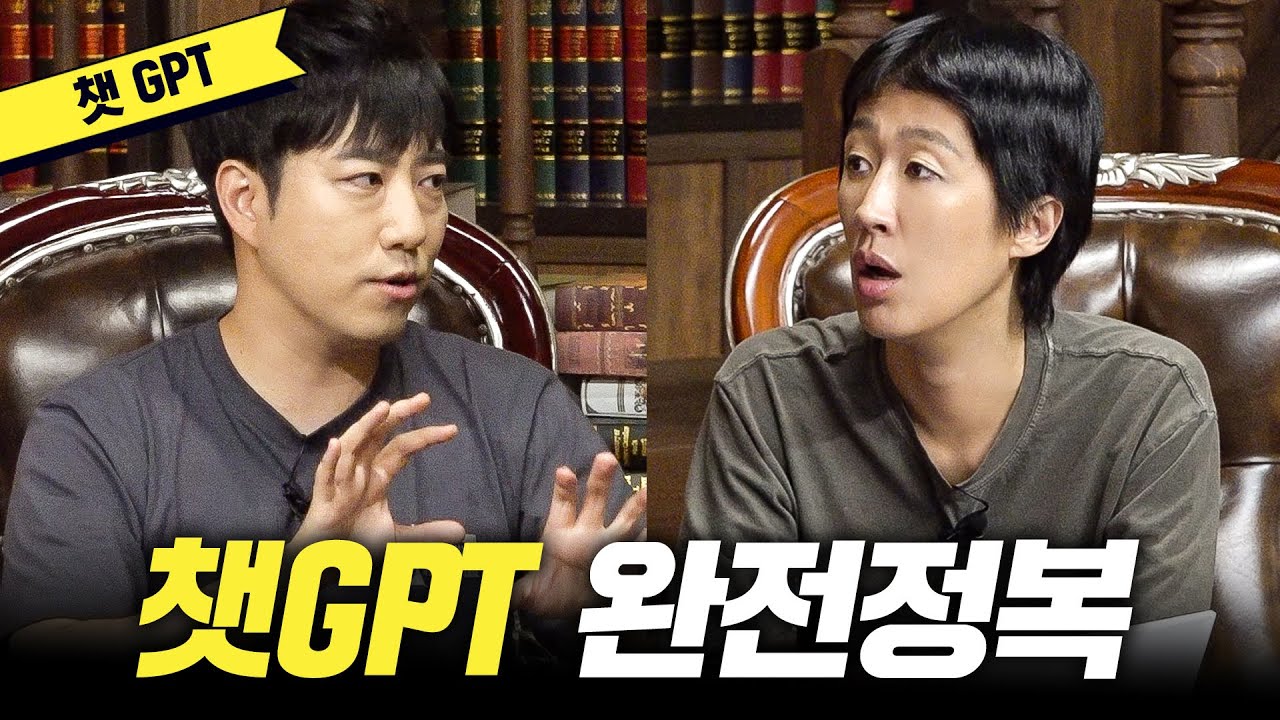
There is no easier explanation than this, 'Chat GPT' (Hong Jin Kyung, Unreal Science, Orbit)
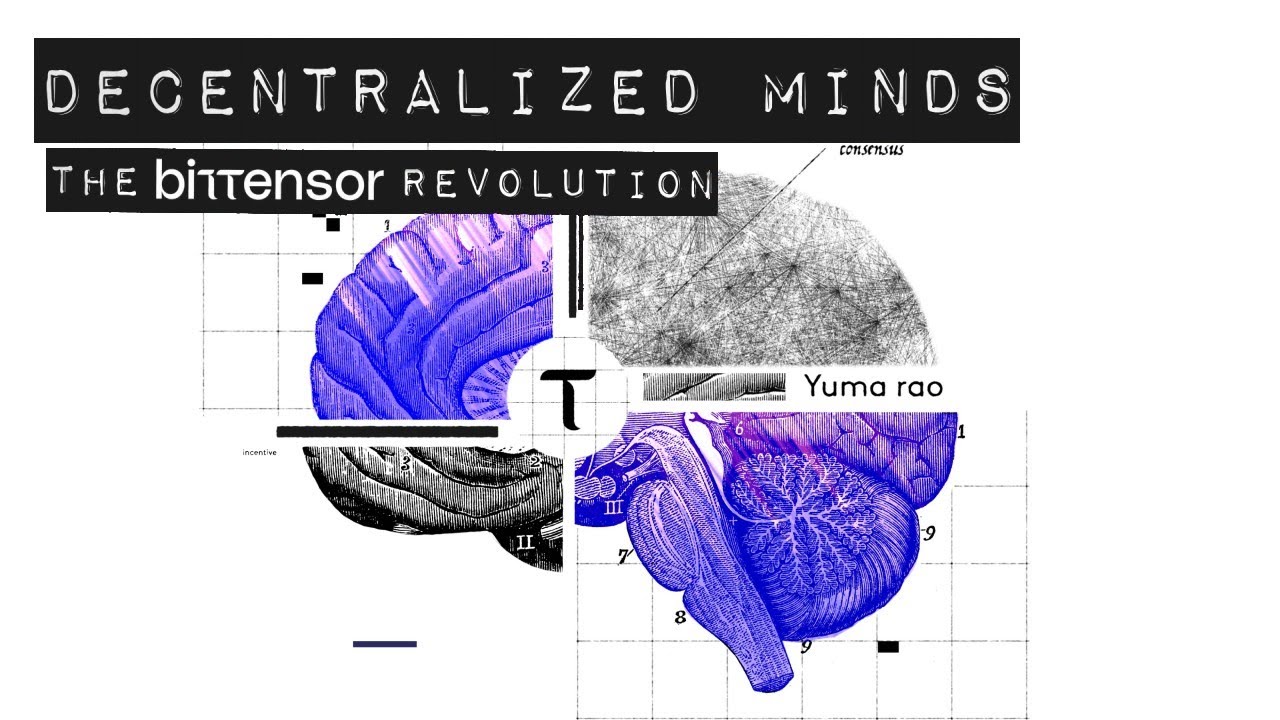
Decentralized Minds, The Bittensor Revolution (Full documentary)
5.0 / 5 (0 votes)