Penentuan Banyaknya Cluster Optimal
Summary
TLDRThis video discusses methods for determining the optimal number of clusters in cluster analysis, particularly for non-hierarchical clustering. The presenter outlines two main approaches: subjective determination based on practical constraints and data-driven determination using criteria like Within Sum of Squares (WSS) and the silhouette coefficient. The elbow method is introduced to identify the point where adding more clusters no longer significantly reduces WSS, while the silhouette coefficient measures both cohesion and separation of clusters. Ultimately, the analyst combines these insights to decide the optimal cluster count, making the process both analytical and context-driven.
Takeaways
- 😀 Cluster analysis often raises the question of how to determine the optimal number of clusters.
- 😀 The first method of determining the optimal number of clusters is subjective, based on the analyst's judgment and considerations.
- 😀 The second method is data-driven, where specific criteria or metrics are used to assess the optimal number of clusters.
- 😀 An example of using subjective judgment is determining the maximum number of clusters based on practical constraints, such as the ability to send a limited number of different messages to customers.
- 😀 The desired characteristics for good clustering are cohesion (objects within the same cluster are similar) and separation (clusters are distinct from each other).
- 😀 The 'Within Sum of Squares' (WSS) is a metric used to evaluate the homogeneity of clusters. The goal is to minimize this value.
- 😀 The Elbow Rule is a technique for finding the optimal number of clusters by identifying where the WSS starts to level off and no longer shows significant improvement.
- 😀 The Silhouette Coefficient is another metric for assessing clustering quality, where higher values indicate better separation and cohesion within clusters.
- 😀 A higher Silhouette Coefficient suggests that the clusters are well-separated and cohesive, providing an indication of good clustering performance.
- 😀 The final decision on the optimal number of clusters should be based on the recommendations from these metrics, but ultimately it is up to the data analyst or scientist to make the final judgment.
Q & A
What is the main focus of the video?
-The main focus of the video is to explain how to determine the optimal number of clusters in cluster analysis, particularly in unsupervised classification.
What are the two strategies mentioned for determining the optimal number of clusters?
-The two strategies are: 1) Subjective determination, based on the analyst's considerations and external constraints. 2) Data-driven determination, which uses statistical criteria derived from the data patterns.
How does the subjective determination method work in cluster analysis?
-In subjective determination, the optimal number of clusters is based on external factors or practical limitations, such as the ability to send a specific number of messages to customers, rather than purely on the data itself.
What is the role of cohesion in cluster analysis?
-Cohesion refers to the homogeneity within a cluster, meaning that the objects within a cluster should be similar to each other. High cohesion indicates that the cluster is well-defined.
What does separation mean in the context of clustering?
-Separation refers to the distinction between different clusters. It means that the clusters should be as different from each other as possible. High separation ensures that objects in different clusters are dissimilar.
What is the Within-Sum of Squares (WSS) method?
-The Within-Sum of Squares (WSS) method is a criterion that measures the variance within each cluster. A lower WSS indicates more cohesive clusters, and the goal is to minimize this value to determine the optimal number of clusters.
How does the elbow method help in determining the optimal number of clusters?
-The elbow method helps by plotting the WSS values for different numbers of clusters and looking for the point where the rate of decrease in WSS slows down significantly. This point, known as the 'elbow,' indicates the optimal number of clusters.
What is the Silhouette Coefficient and how is it used in cluster analysis?
-The Silhouette Coefficient measures how well each object fits within its cluster compared to other clusters. A higher silhouette score indicates better clustering, and the optimal number of clusters is the one that maximizes the average silhouette score.
How does the Silhouette Coefficient formula work?
-The Silhouette Coefficient formula is (B - A) / max(A, B), where A is the average distance from an object to all other objects in the same cluster (cohesion) and B is the average distance from the object to objects in the nearest different cluster (separation).
Can the results of clustering always be considered definitive?
-No, the results of clustering should be viewed as recommendations rather than definitive answers. The final decision on the optimal number of clusters depends on the context and specific goals of the analysis.
Outlines
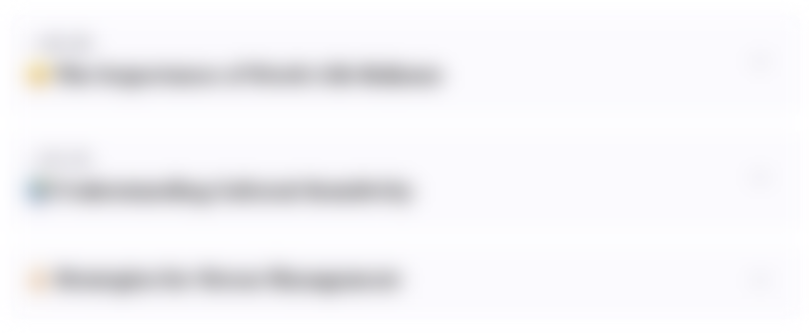
This section is available to paid users only. Please upgrade to access this part.
Upgrade NowMindmap
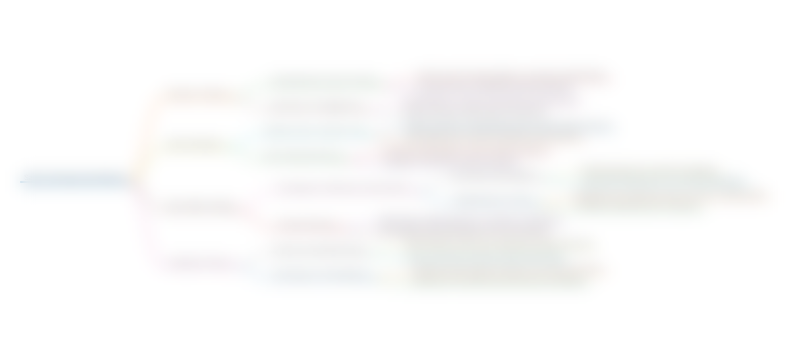
This section is available to paid users only. Please upgrade to access this part.
Upgrade NowKeywords
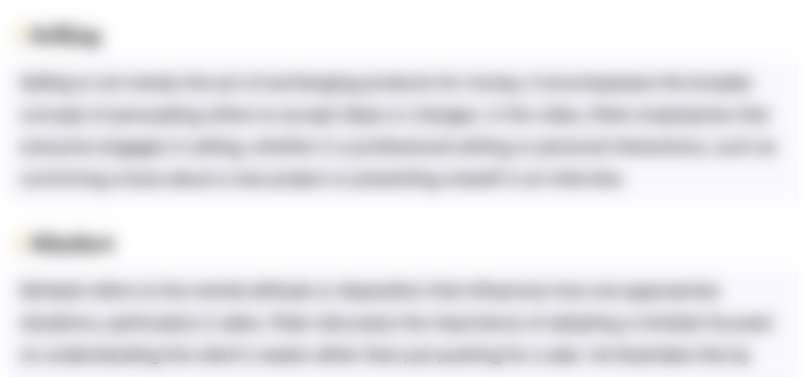
This section is available to paid users only. Please upgrade to access this part.
Upgrade NowHighlights
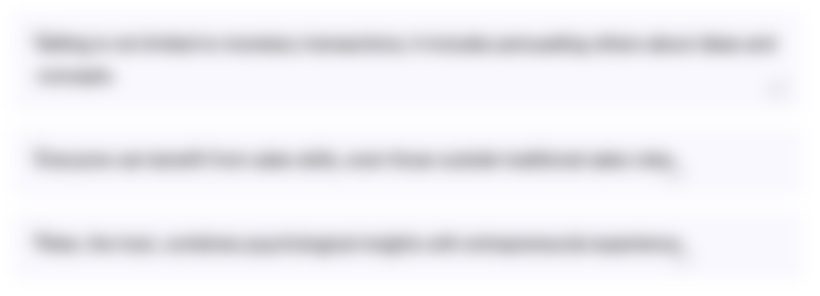
This section is available to paid users only. Please upgrade to access this part.
Upgrade NowTranscripts
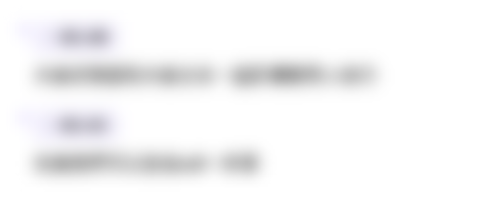
This section is available to paid users only. Please upgrade to access this part.
Upgrade NowBrowse More Related Video
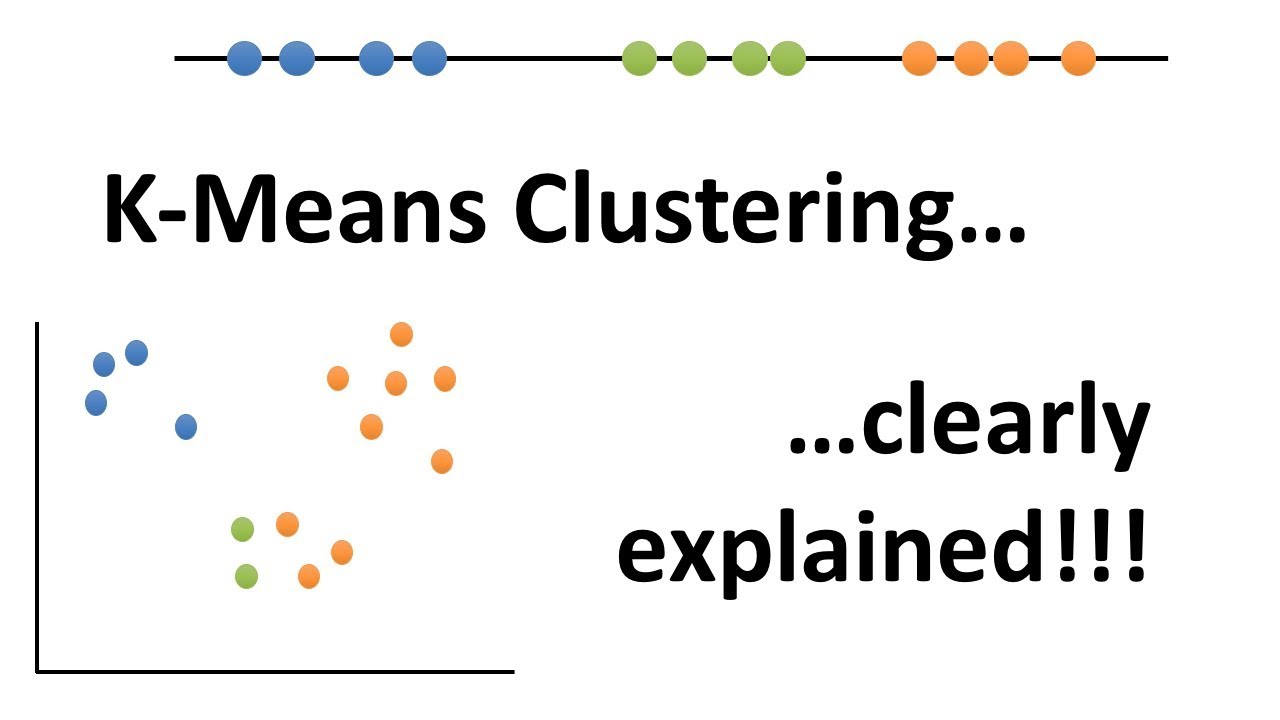
StatQuest: K-means clustering

K-Means Clustering Algorithm with Python Tutorial

Hierarchical Cluster Analysis [Simply explained]
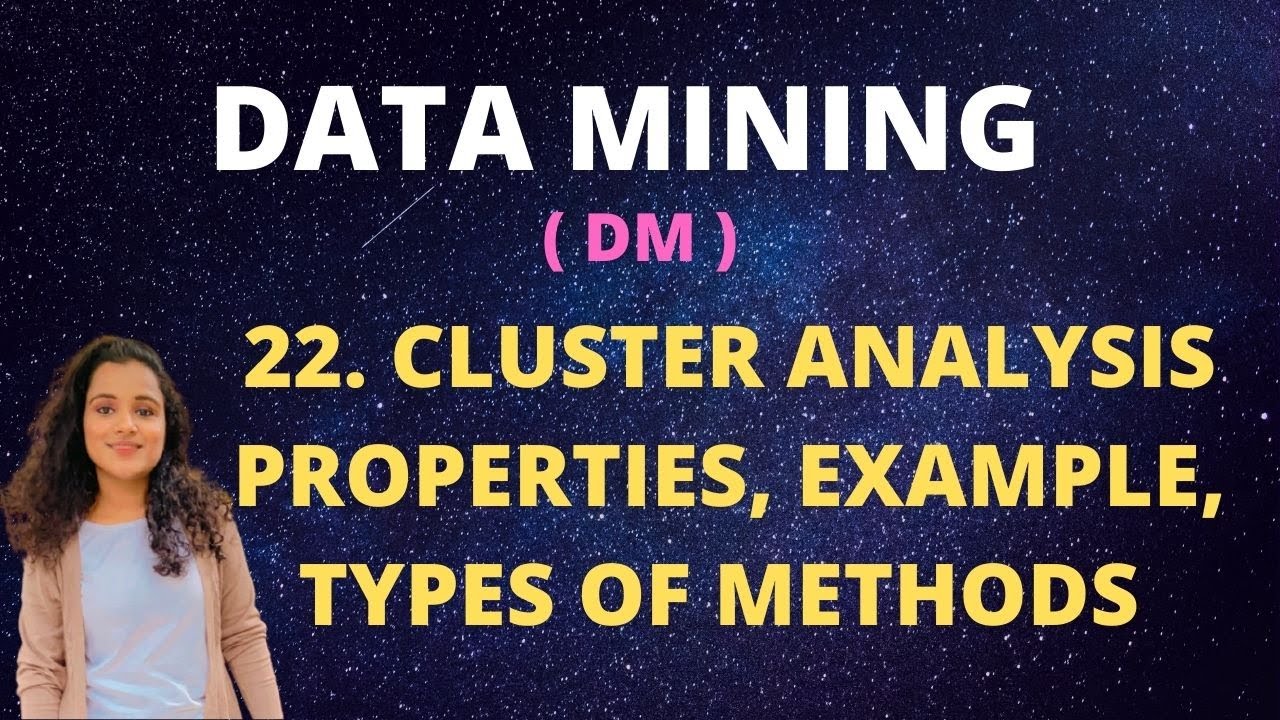
#22 Cluster Analysis - Properties, Categories Of Methods |DM|
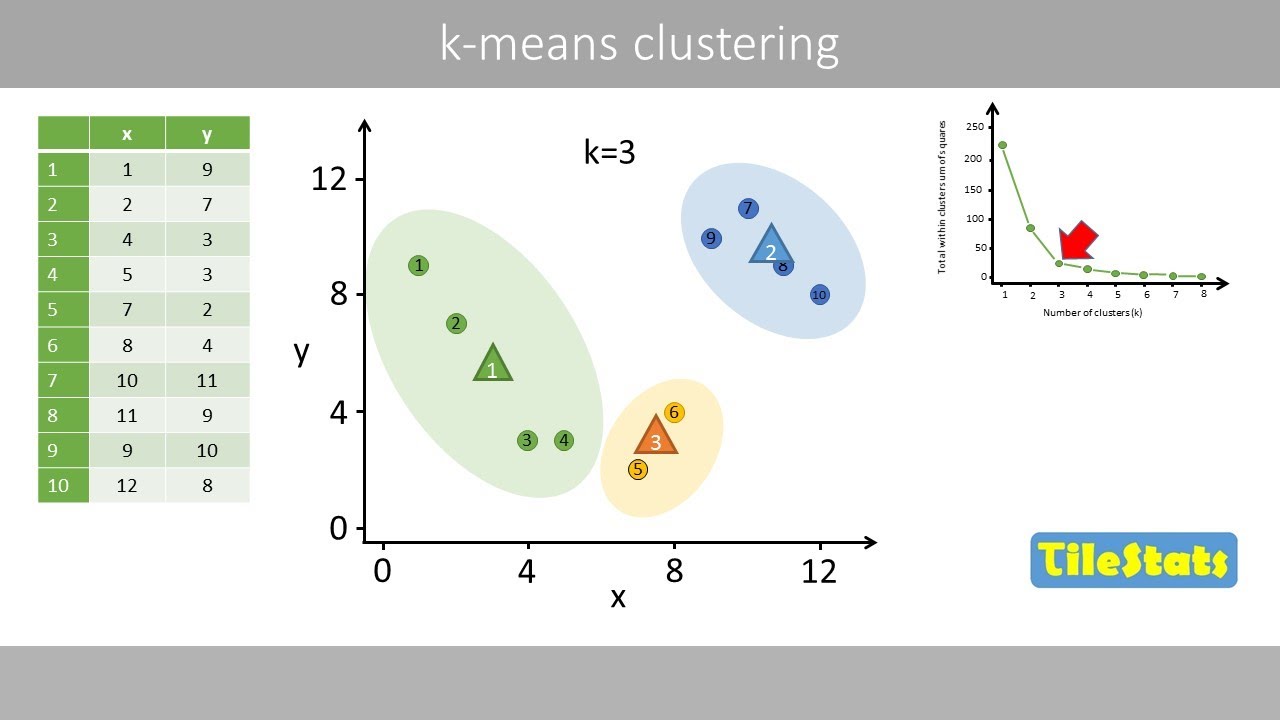
k-means clustering - explained

Agglomerative Hierarchical Clustering Single link Complete link Clustering by Dr. Mahesh Huddar
5.0 / 5 (0 votes)