Ilmu Data #5 - Varians dan Standar Deviasi
Summary
TLDRThis video explains the concepts of variance and standard deviation, both key statistical tools used to measure the spread or dispersion of data around its mean. Variance calculates the average of squared differences from the mean, while standard deviation is simply the square root of variance, offering a more intuitive interpretation. The video illustrates these concepts using a height dataset, showing how variance and standard deviation help us understand data distribution, detect outliers, and apply this understanding in real-world data analysis like Principal Component Analysis (PCA). Standard deviation is emphasized as the more interpretable measure compared to variance.
Takeaways
- 😀 Variance and standard deviation are statistical measures used to assess the spread or dispersion of data points from the mean.
- 😀 A larger variance or standard deviation indicates data that is more spread out from the mean, while a smaller value means the data is closer to the mean.
- 😀 Variance is calculated by squaring the difference between each data point and the mean, while standard deviation is the square root of variance.
- 😀 Variance cannot be easily interpreted directly because it is in squared units, whereas standard deviation is in the same units as the data, making it easier to understand.
- 😀 To calculate variance, first subtract the mean from each data point, square the differences, sum them, and then divide by the total number of data points.
- 😀 Standard deviation is important because it allows for a more intuitive understanding of data spread compared to variance, which is abstract and harder to interpret.
- 😀 When the absolute values of data differences are used instead of squaring, the data’s true dispersion may not be captured accurately.
- 😀 Variance is useful in further statistical processes like Principal Component Analysis (PCA) for calculating covariance and eigenvectors.
- 😀 Standard deviation is used to categorize data as being close to the mean, slightly above or below average, or as extreme outliers.
- 😀 In a normal distribution, about 68% of data lies within one standard deviation of the mean, 95% within two standard deviations, and 99% within three standard deviations.
- 😀 Variance and standard deviation both help identify and classify data ranges, with standard deviation providing a clearer interpretation for practical applications like detecting outliers or classifying data as ‘average’, ‘above average’, or ‘below average’.
Q & A
What is the primary difference between variance and standard deviation?
-The primary difference is that variance measures the average squared deviation from the mean, while standard deviation is the square root of variance. Standard deviation is easier to interpret as it has the same unit as the data, whereas variance has squared units.
Why is variance difficult to interpret directly?
-Variance is difficult to interpret directly because its units are squared, making it less intuitive when compared to the original data units.
How is the variance of a data set calculated?
-Variance is calculated by subtracting the mean from each data point, squaring the differences, summing all the squared differences, and then dividing the sum by the total number of data points (for a population).
Why do we square the differences from the mean when calculating variance?
-Squaring the differences ensures that all deviations, whether positive or negative, are treated equally, and it also magnifies larger deviations, making the measure more sensitive to extreme values.
How is standard deviation related to variance?
-Standard deviation is simply the square root of variance. It brings the measure back to the original unit of measurement, making it easier to interpret and compare to the original data.
What is the practical advantage of using standard deviation over variance?
-Standard deviation is easier to interpret because it is in the same unit as the original data, whereas variance is in squared units. This makes standard deviation more useful for real-world applications like classifying data points or understanding data dispersion.
How does the standard deviation help in classifying data?
-Standard deviation helps by creating ranges (e.g., one standard deviation above or below the mean) to classify data points. It allows us to categorize values as close to average, high, or low based on their distance from the mean.
What happens when you apply standard deviation in a normal distribution?
-In a normal distribution, about 68% of the data lies within one standard deviation of the mean, 95% lies within two standard deviations, and 99.7% lies within three standard deviations.
Can you explain what an 'outlier' is in the context of standard deviation?
-An outlier is a data point that is significantly different from the other values, typically lying outside three standard deviations from the mean. It is considered an extreme value that doesn't fit within the general pattern of the data.
Why is the value of variance (e.g., 2419) not directly interpretable?
-Variance is not directly interpretable because it is in squared units, making it harder to relate to the original data. It is mainly used for further calculations, such as computing the standard deviation or in more complex statistical methods.
Outlines
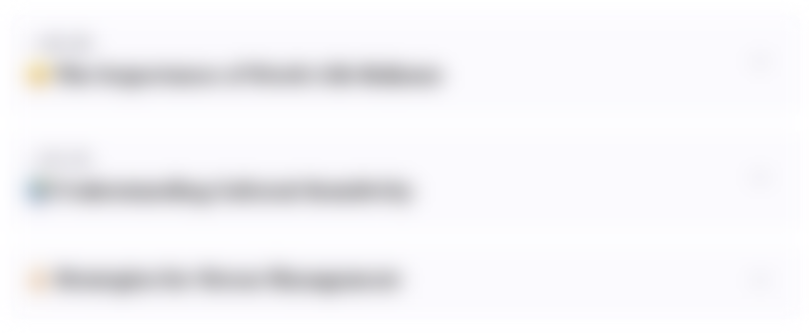
This section is available to paid users only. Please upgrade to access this part.
Upgrade NowMindmap
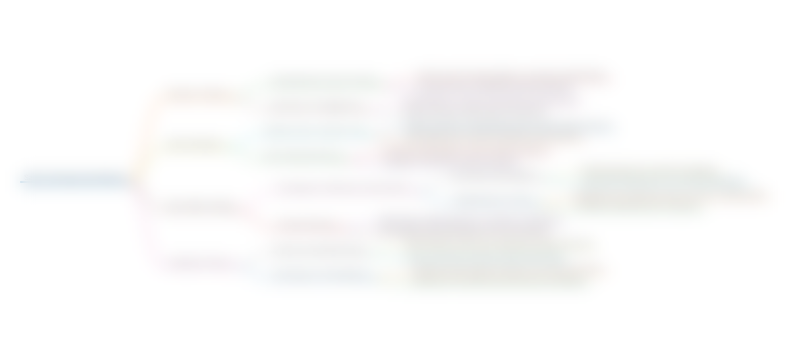
This section is available to paid users only. Please upgrade to access this part.
Upgrade NowKeywords
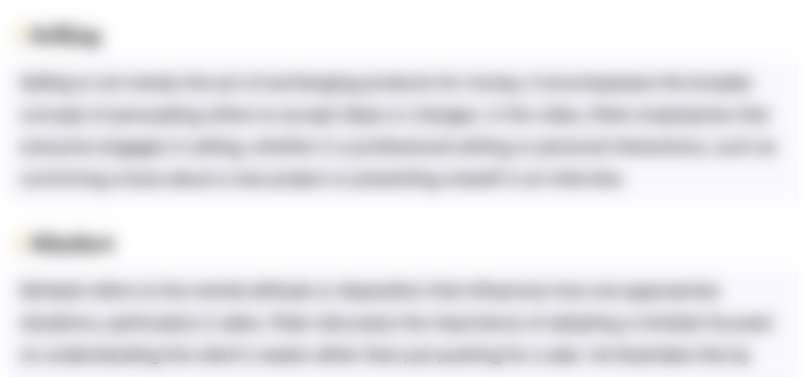
This section is available to paid users only. Please upgrade to access this part.
Upgrade NowHighlights
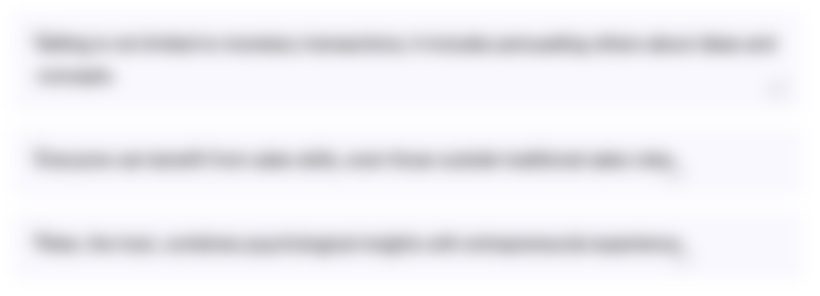
This section is available to paid users only. Please upgrade to access this part.
Upgrade NowTranscripts
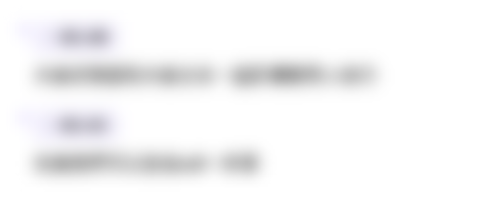
This section is available to paid users only. Please upgrade to access this part.
Upgrade NowBrowse More Related Video
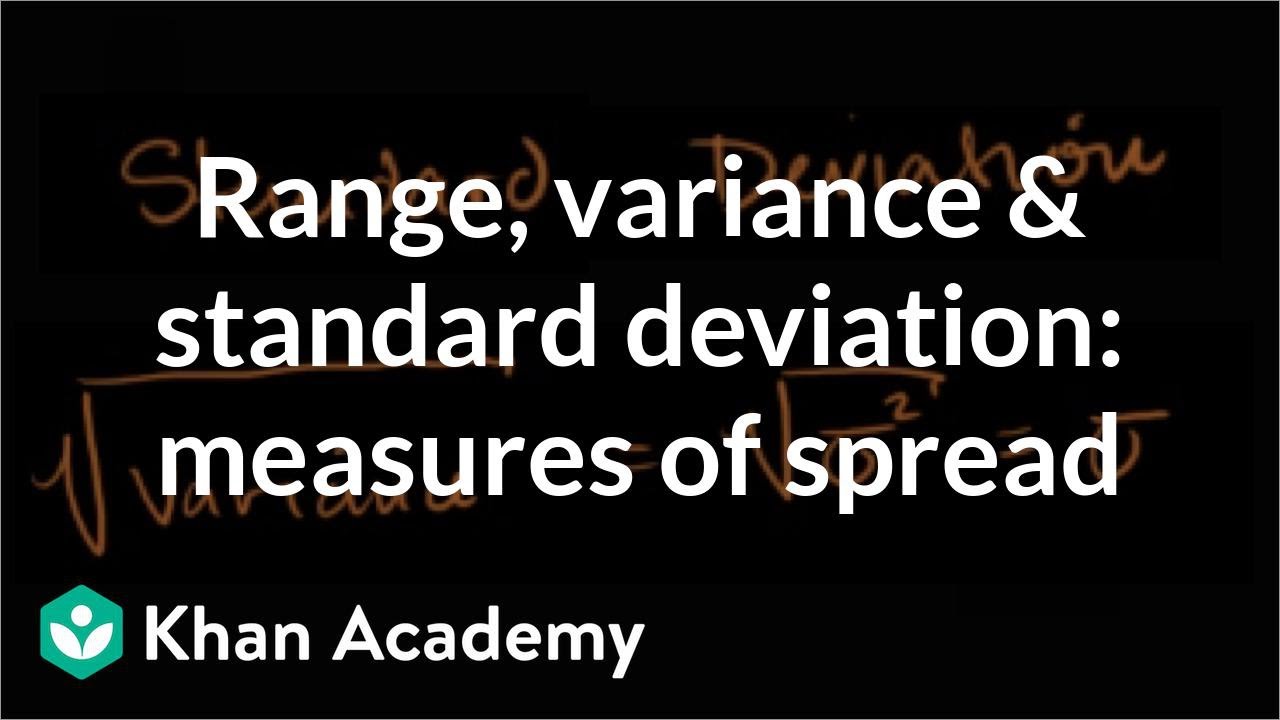
Range, variance and standard deviation as measures of dispersion | Khan Academy
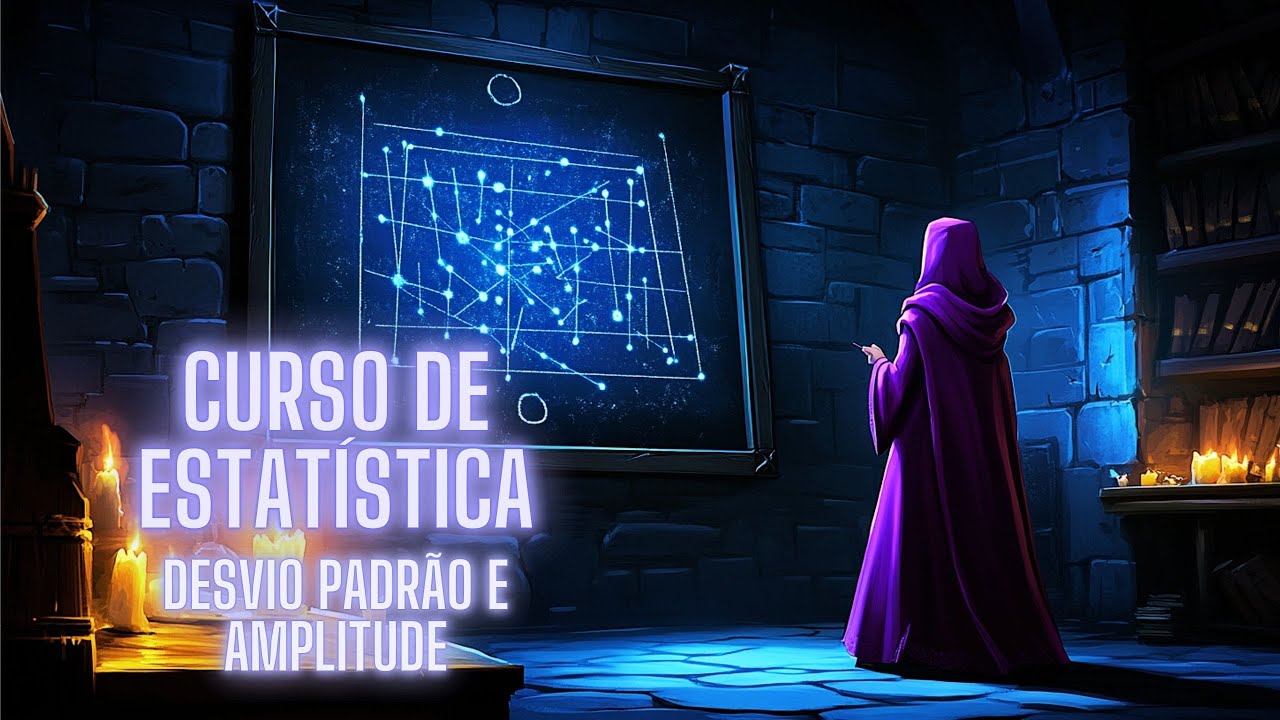
APRENDA ESTATÍSTICA 10: Medidas de Dispersão

Statistika Part. 4 | Ukuran Penyebaran, Ragam, Varian, Simpangan Rata-rata, Simpangan Baku
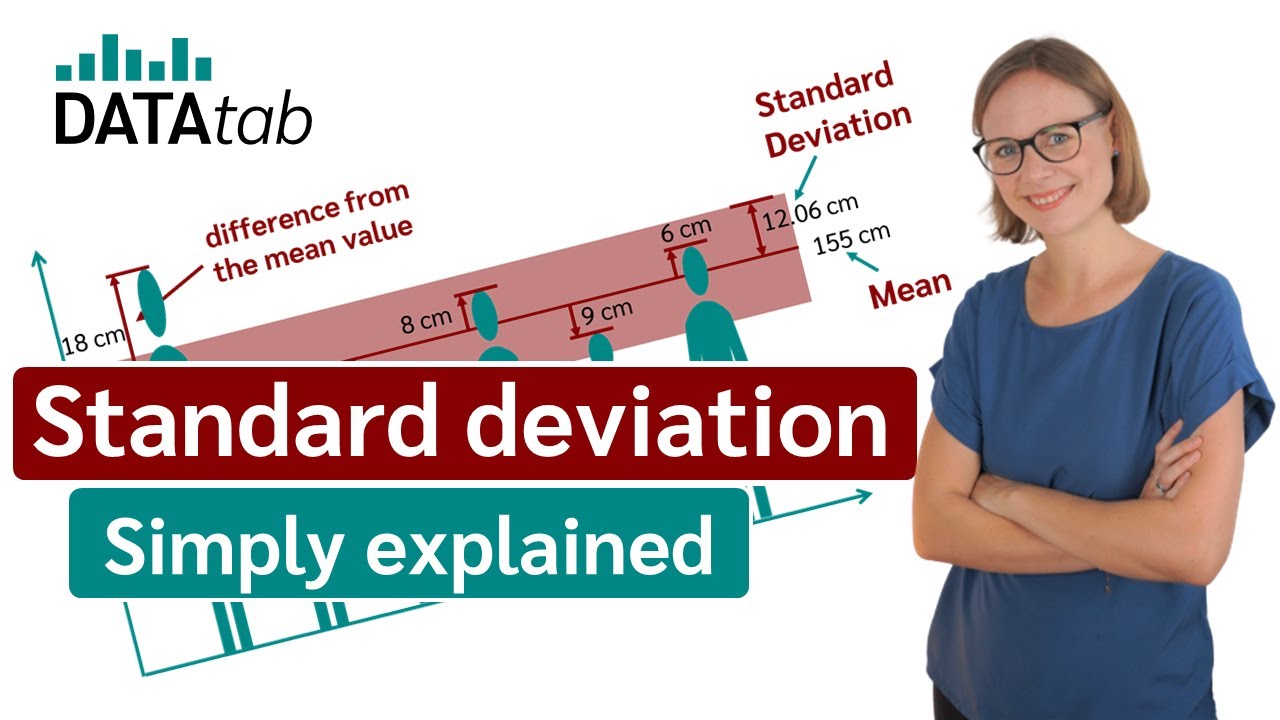
Standard deviation (simply explained)

MÉDIA, MEDIANA, VARIÂNCIA E DESVIO PADRÃO | ESTATÍSTICA #01

ESTADISTICA DESCRIPTIVA.- PARAMETROS DE CENTRALIZACION Y DISPERSION.
5.0 / 5 (0 votes)