Introduction to Spatial Statistics #GIS #Maps #Data Science
Summary
TLDRThis introductory lecture on spatial statistics explores the key concepts of quantifying spatial patterns, relationships, and changes over time. It distinguishes spatial statistics from traditional statistics by emphasizing the importance of spatial relationships and geographical features. The video covers topics like central features, weighted mean centers, and hot spot analysis using ArcGIS Pro, demonstrating how various statistical methods can be applied to real-world data. Viewers learn how to use tools like mean and median center calculations, as well as assess clusters and distributions, to draw meaningful conclusions about spatial data patterns. The lecture offers hands-on demonstrations with crime data and census tract analysis.
Takeaways
- ๐ Spatial statistics focuses on quantifying patterns, relationships, and changes over time in geographical features.
- ๐ Unlike traditional statistics, spatial statistics accounts for spatial relationships, not just attribute values.
- ๐ Space is fundamental in spatial statistics, as the location and its relationships are key to understanding patterns and trends.
- ๐ Spatial statistics helps answer questions like whether phenomena are clustered or dispersed in space.
- ๐ The mean center is used to find the spatial average of feature coordinates, which helps determine central points in a dataset.
- ๐ The median center is useful for identifying the central tendency of data that is resistant to outliers, such as in crime analysis.
- ๐ Spatial statistics can help determine relationships between attributes and locations, such as store locations influenced by crime rates.
- ๐ Itโs important to understand the type of spatial data being analyzed, whether discrete or continuous, as this affects the statistical methods used.
- ๐ Significance tests, such as confidence intervals, are used in spatial statistics to determine whether observed patterns are meaningful or due to chance.
- ๐ Spatial analysis can reveal clusters of phenomena, like high crime areas, and help assess if these clusters are statistically significant.
- ๐ Tools like ArcGIS Pro allow spatial statistic methods like mean center, median center, and hotspot analysis to be easily applied for analyzing geographic distributions.
Q & A
What is the primary goal of spatial statistics?
-The primary goal of spatial statistics is to quantify patterns and relationships between geographical features and track changes over time, aiming to understand whether a pattern or relationship exists due to random chance or if it's meaningful.
How does spatial statistics differ from traditional statistics?
-Unlike traditional statistics, which work with attribute values alone (like numbers), spatial statistics accounts for spatial relationships, considering the location and proximity of data points to understand the underlying patterns.
Why is space fundamental to spatial statistics?
-Space is fundamental because spatial statistics focuses on the relationships between locations and their spatial arrangement. For example, it helps in understanding whether phenomena like crime rates or wildlife migration are clustered or randomly distributed.
What is an example of how spatial statistics might be used in real-world applications?
-One example is determining whether deer herds are traveling in a specific direction, or if crime rates in a city are concentrated in particular neighborhoods, using spatial statistics to analyze the spatial patterns.
What is the null hypothesis in spatial statistics?
-The null hypothesis in spatial statistics assumes that no pattern or relationship exists, and any observed pattern is due to random chance. Statistical tests are used to determine whether this hypothesis can be rejected.
What types of spatial data representations exist, and how do they influence statistical methods?
-Spatial data can be represented as discrete (such as point locations) or continuous (such as raster data). The choice of representation influences the statistical methods used, as different approaches are needed for analyzing patterns in each type of data.
How do you interpret the results of spatial statistics?
-The interpretation of spatial statistics typically involves analyzing patterns such as clustering, dispersion, or randomness, determining the significance of these patterns, and evaluating the strength of relationships through statistical measures like confidence intervals.
What are the potential pitfalls when interpreting spatial statistical results?
-Pitfalls can include issues like scale effects, boundary and edge effects, and data quality concerns. It's also important to consider factors like proximity, distance (straight line vs. travel time), and the influence of control groups when interpreting results.
What role do weights play in spatial statistics?
-Weights in spatial statistics allow certain features or attributes to be emphasized more than others when calculating statistics, such as in weighted averages, where some data points are considered more significant based on their attributes.
How does hot spot analysis differ from a heat map in spatial statistics?
-Hot spot analysis identifies statistically significant clusters of features based on their attributes, while a heat map simply visualizes the frequency or intensity of features in a given area, without considering statistical significance.
Outlines
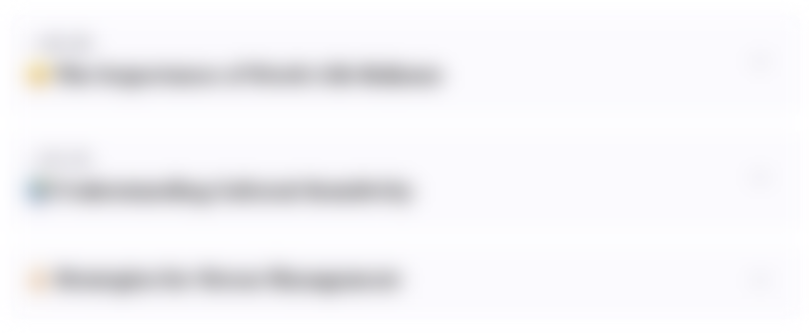
This section is available to paid users only. Please upgrade to access this part.
Upgrade NowMindmap
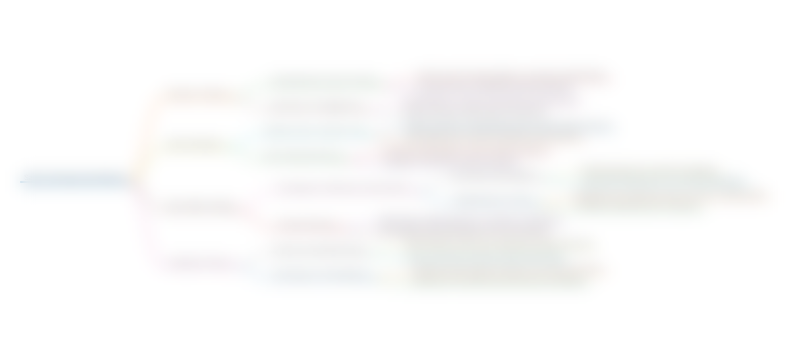
This section is available to paid users only. Please upgrade to access this part.
Upgrade NowKeywords
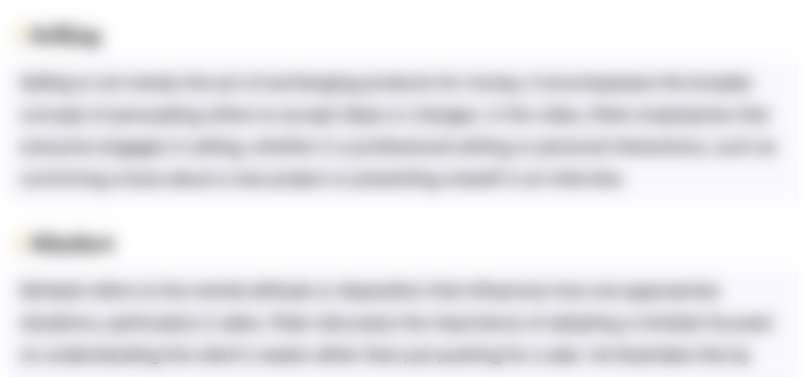
This section is available to paid users only. Please upgrade to access this part.
Upgrade NowHighlights
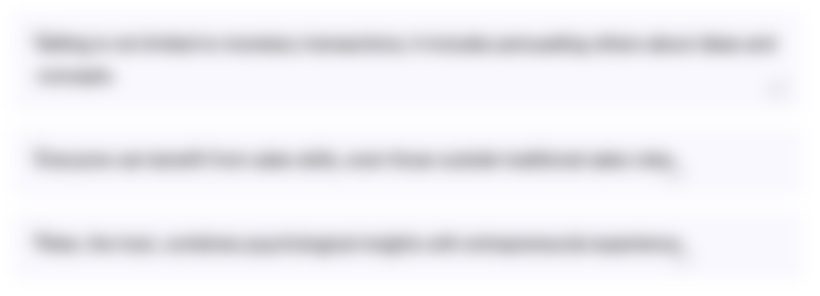
This section is available to paid users only. Please upgrade to access this part.
Upgrade NowTranscripts
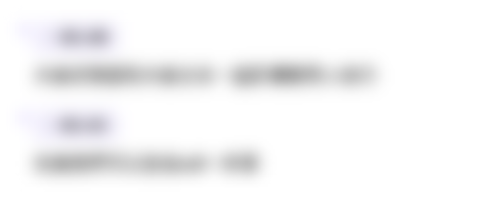
This section is available to paid users only. Please upgrade to access this part.
Upgrade NowBrowse More Related Video
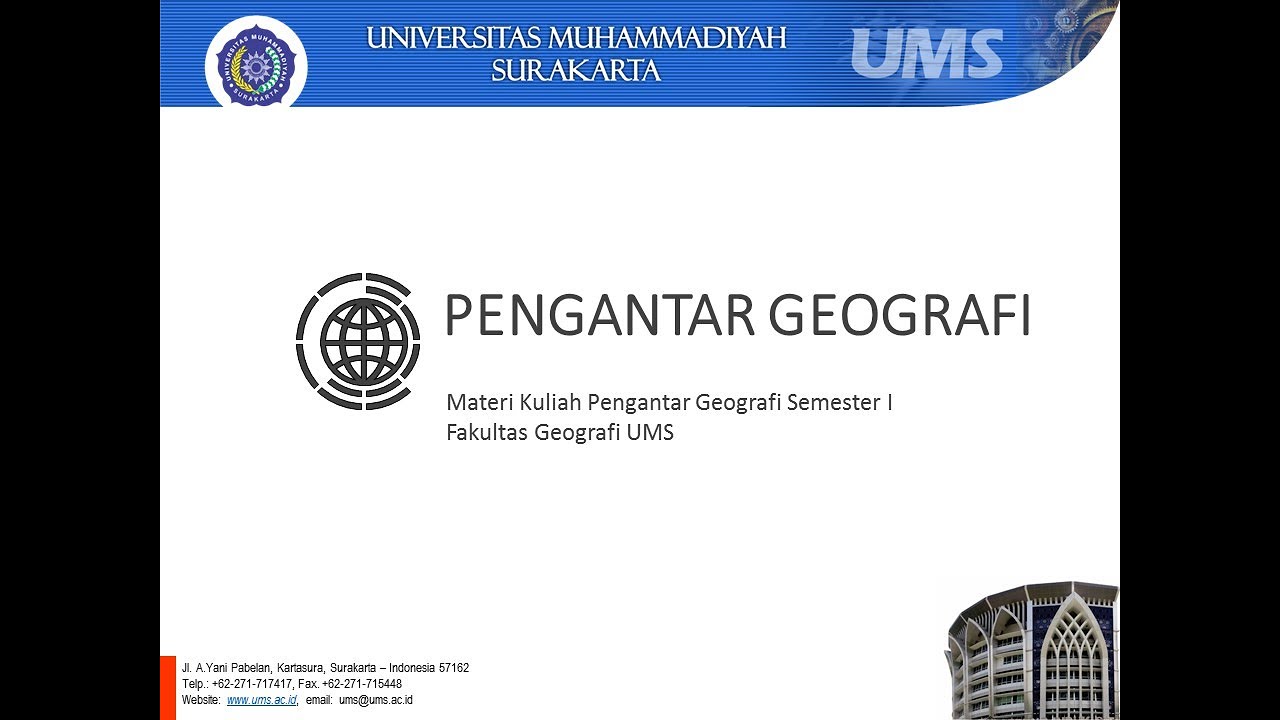
Pengantar Geografi I Pendekatan Keruangan (Spatial Approach)
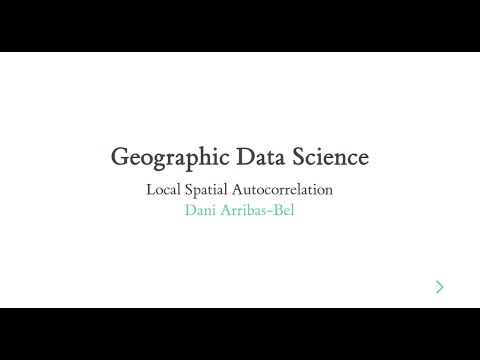
Local Spatial Autocorrelation - A Course on Geographic Data Science

Identifikasi Permukiman Tradisional - Identifikasi Elemen Permukiman

Spatial Database Management System (SDBMS) - Spatial Data Science and Applications

GIS Module 11: Spatial Modeling
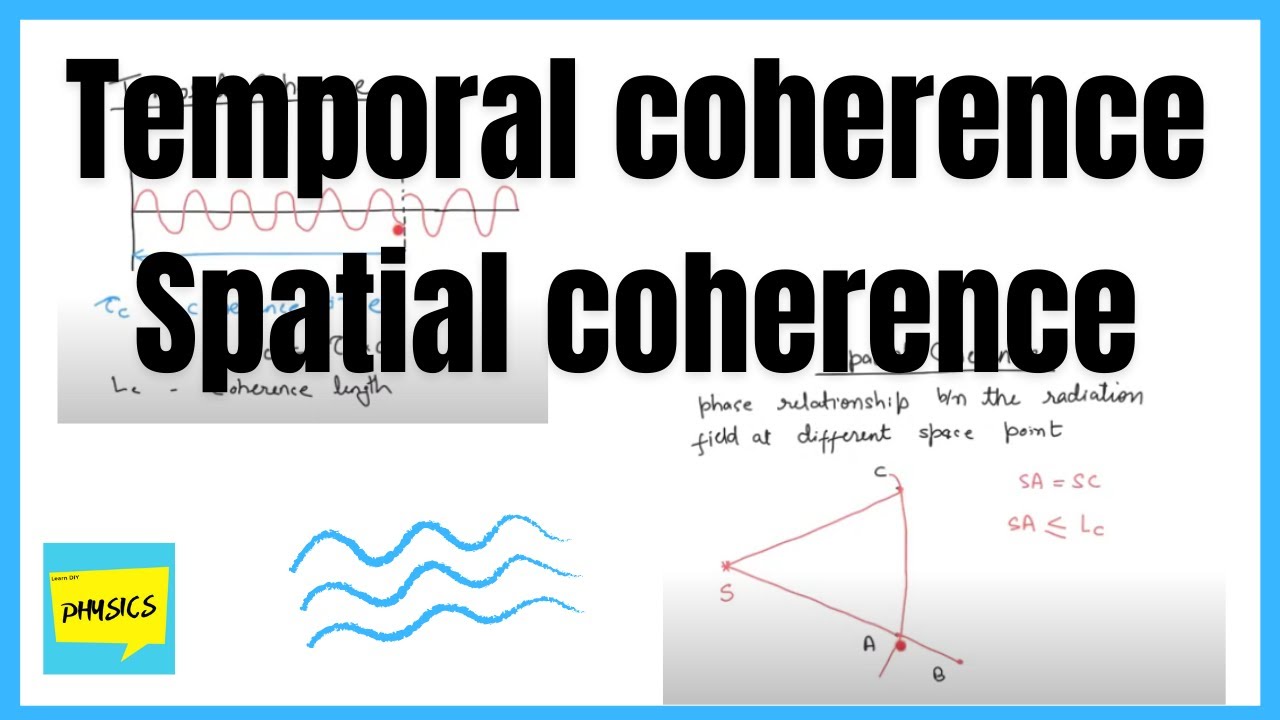
Temporal and spatial coherence
5.0 / 5 (0 votes)