Applications of Simulation in Supply Chain Management
Summary
TLDR本次直播活动由麻省理工学院(MIT)的供应链管理硕士课程主办,Miguel Rodriguez Garcia和Paulo Sosa共同主持。活动邀请了拥有超过八年供应链管理经验的工业工程师Yashar Ahmadov作为嘉宾演讲者。Yashar目前是亚马逊的高级仿真数据科学家,他利用仿真、数学优化和数据科学工具解决复杂的供应链问题。直播内容包括Yashar关于仿真的介绍、在库存管理、运输和系统动力学中的应用,以及一个实时演示,展示了如何在短时间内创建供应链的可视化仿真。此外,还讨论了何时使用以及何时避免使用仿真,并提供了相关工具和技能的建议。
Takeaways
- 🎓 这是麻省理工学院(MIT)供应链管理微硕士课程的第二次也是最后一次现场活动,由Miguel Rodriguez Garcia和Paulo Sosa主持。
- 👨🏫 Miguel和Paulo分别负责SCx供应链基础和SCx供应链动态课程,并鼓励参与者完成微硕士项目以申请MIT或其他大学的供应链管理混合硕士项目。
- 🤖 嘉宾演讲者Yashar Ahmadov拥有超过八年的供应链管理工作经验,目前在亚马逊担任高级仿真数据科学家,使用仿真、数学优化和数据科学工具解决复杂的供应链问题。
- 🔍 Yashar介绍了供应链中仿真的概念,包括确定性与随机性仿真、静态与动态仿真、连续与离散仿真等不同类型的仿真。
- 📈 仿真的优势在于增加现实感、研究危险系统、瓶颈分析、回答“如果……会怎样”的问题、结果可复现以及易于与管理层沟通。
- 🚫 然而,在某些情况下不应使用仿真,例如当可以使用常识分析,或者当系统行为无法验证,或者过度承诺而无法满足项目期望时。
- 🛠️ Yashar推荐了一款名为Analogic的基于Java的仿真软件,它提供了GIS地图、空间标记工具、2D/3D仿真以及特定行业的库。
- 📊 在库存管理中,仿真可以帮助进行需求预测、安全库存优化、订单点、提前期和ABC分析等任务。
- 🚚 仿真还可以用于交通流量建模、机场、港口运营、公共交通、交通安全等领域,许多仿真软件包含GIS地图,方便模拟实际路线。
- 💻 Yashar通过一个实际的供应链仿真案例,展示了如何快速创建供应链的视觉仿真,包括制造点、配送中心、零售商和卡车的移动。
- 🔧 他建议学习Java编程基础和特定仿真软件的操作,这对于从事供应链仿真领域的职业发展至关重要。
Q & A
MIT的供应链管理微硕士课程包含哪些课程?
-MIT的供应链管理微硕士课程包含五个课程,其中包括供应链基础(SCx)、供应链动态(SC3x)、供应链分析(SCx Supply Chain Analytics)、供应链设计(SC2x Supply Chain Design)以及供应链管理的混合硕士课程。
Yashar Ahmadov在亚马逊担任什么职位?
-Yashar Ahmadov在亚马逊担任高级仿真数据科学家,他使用仿真、数学优化和数据科学工具来解决复杂的供应链问题。
什么是数字孪生技术?
-数字孪生技术是一种创建物理实体如工厂、港口、仓库、零售商等的数字表示的方法,这样我们就可以在数字环境中进行实验和分析。
在供应链管理中,模拟的主要作用是什么?
-在供应链管理中,模拟主要用于理解、预测系统行为和评估不同的选择方案。它可以帮助进行'what-if'分析,即在不同情况下系统将如何表现。
模拟可以分为哪些类型?
-模拟可以分为确定性模拟和随机性模拟。确定性模拟中没有随机变量,输入相同总是得到相同的输出;而随机性模拟中包含随机变量,更贴近现实生活的不确定性。
为什么在供应链管理中使用基于代理的模拟?
-基于代理的模拟允许模拟从非常低的抽象级别到非常高的抽象级别,可以模拟个体行为规则和不同代理之间的交互,提供了极大的灵活性和复杂性处理能力。
模拟的优点有哪些?
-模拟的优点包括增加现实感、可以模拟现有或不存在的系统、可以研究危险系统而无需风险、瓶颈分析、回答'what-if'问题、结果可复现、易于与管理层沟通等。
在什么情况下不应该使用模拟?
-如果可以通过常识分析解决,或者当没有资源去建立和验证模拟模型,或者不能满足项目期望,或者系统行为结构不良时,就不应该使用模拟。
在供应链模拟中,如何定义和使用代理?
-在供应链模拟中,代理是代表现实世界中的实体,如制造站点、仓库、卡车等的对象。你可以在代理内部定义它们的行为和参数,这类似于面向对象编程中创建类和对象的概念。
模拟中常见的陷阱有哪些?
-常见的陷阱包括试图一开始就模拟整个复杂性,而不是逐步增加复杂度;以及在开发过程中缺乏与利益相关者的沟通,没有让他们了解模拟的工作原理和结果。
如何将模拟与优化结合起来使用?
-模拟和优化可以结合使用,例如,先通过优化算法确定供应链网络的最优结构,然后通过模拟来验证这一结构在不同场景下的表现。此外,机器学习模型可以用于为模拟提供决策参数。
Outlines
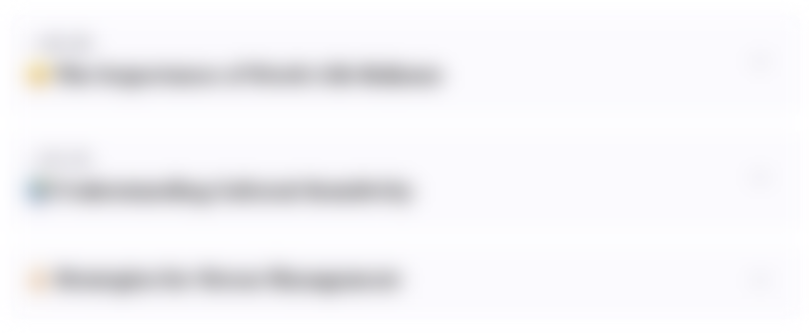
This section is available to paid users only. Please upgrade to access this part.
Upgrade NowMindmap
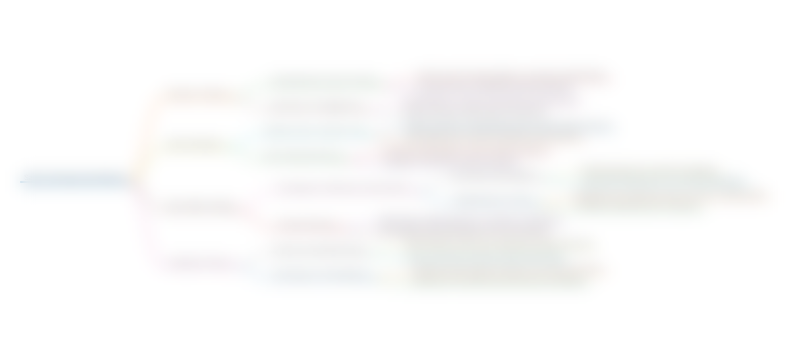
This section is available to paid users only. Please upgrade to access this part.
Upgrade NowKeywords
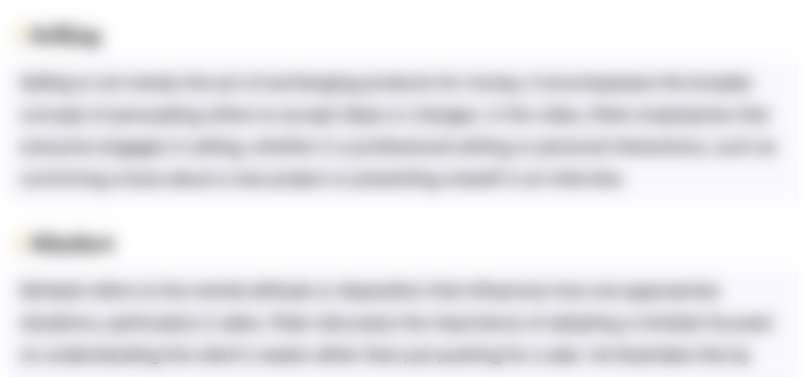
This section is available to paid users only. Please upgrade to access this part.
Upgrade NowHighlights
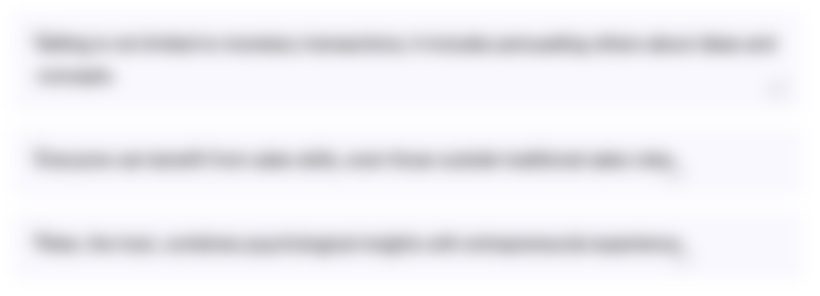
This section is available to paid users only. Please upgrade to access this part.
Upgrade NowTranscripts
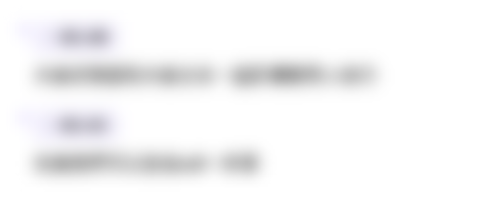
This section is available to paid users only. Please upgrade to access this part.
Upgrade NowBrowse More Related Video
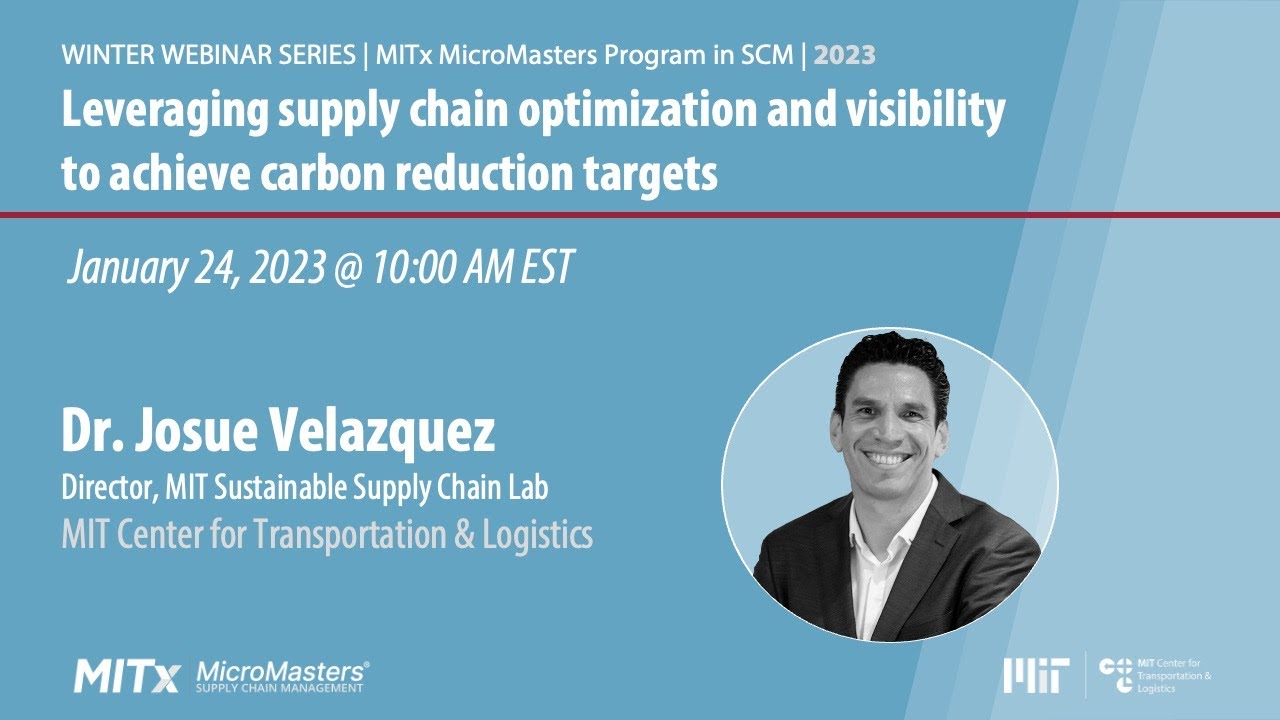
Leveraging supply chain optimization and visibility to achieve carbon reduction targets
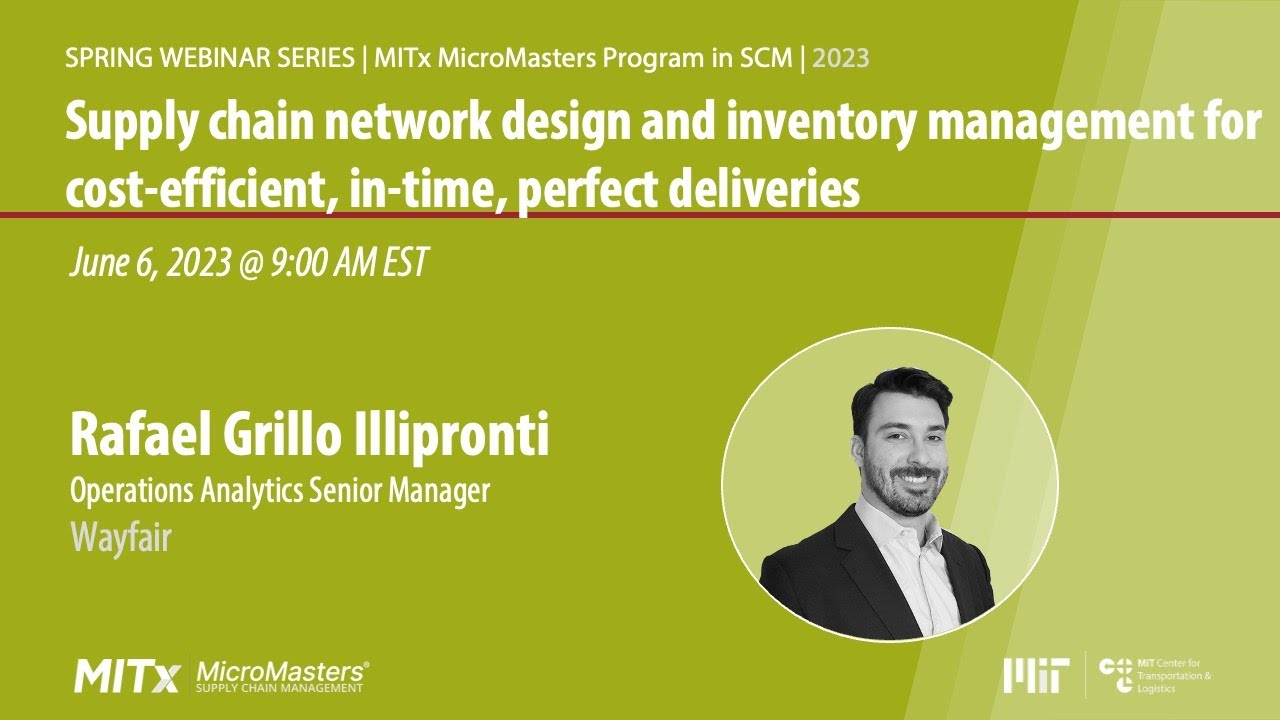
Supply chain network design and inventory management for cost-efficient, in-time, perfect deliveries

Strategic Sourcing in Supply Chain: Elevating Procurement for Business Success
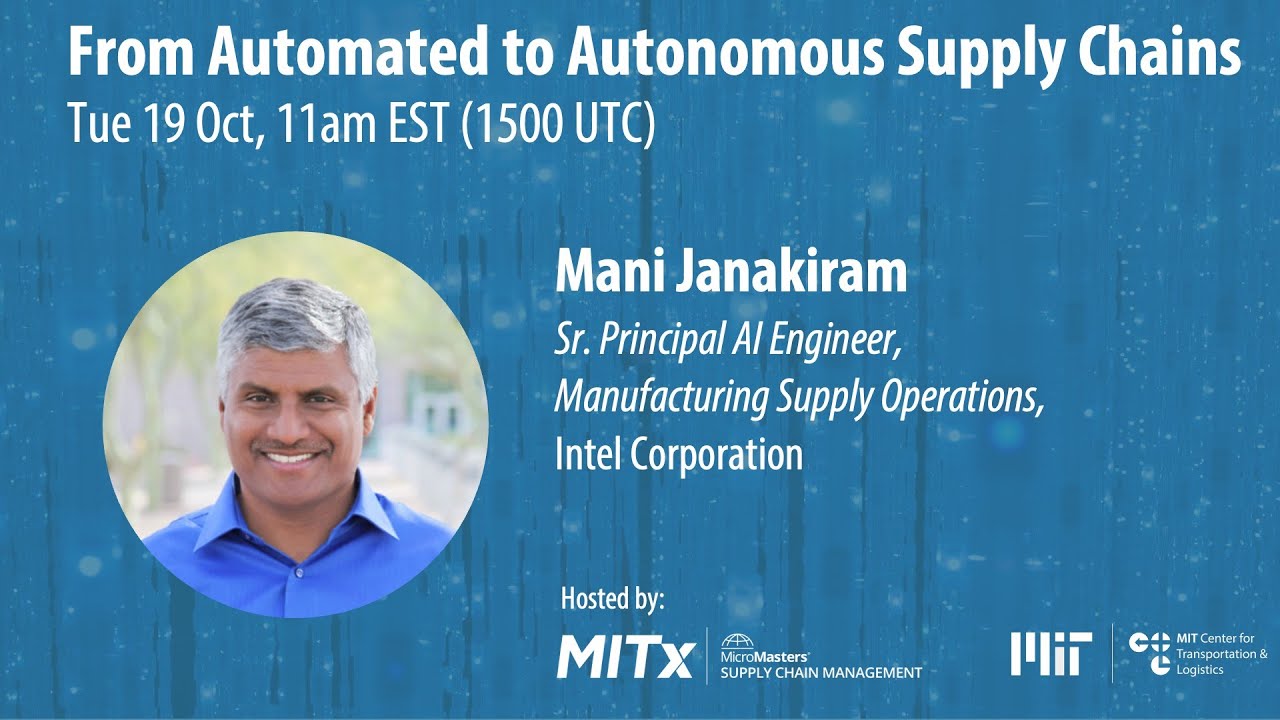
From Automated to Autonomous Supply Chains
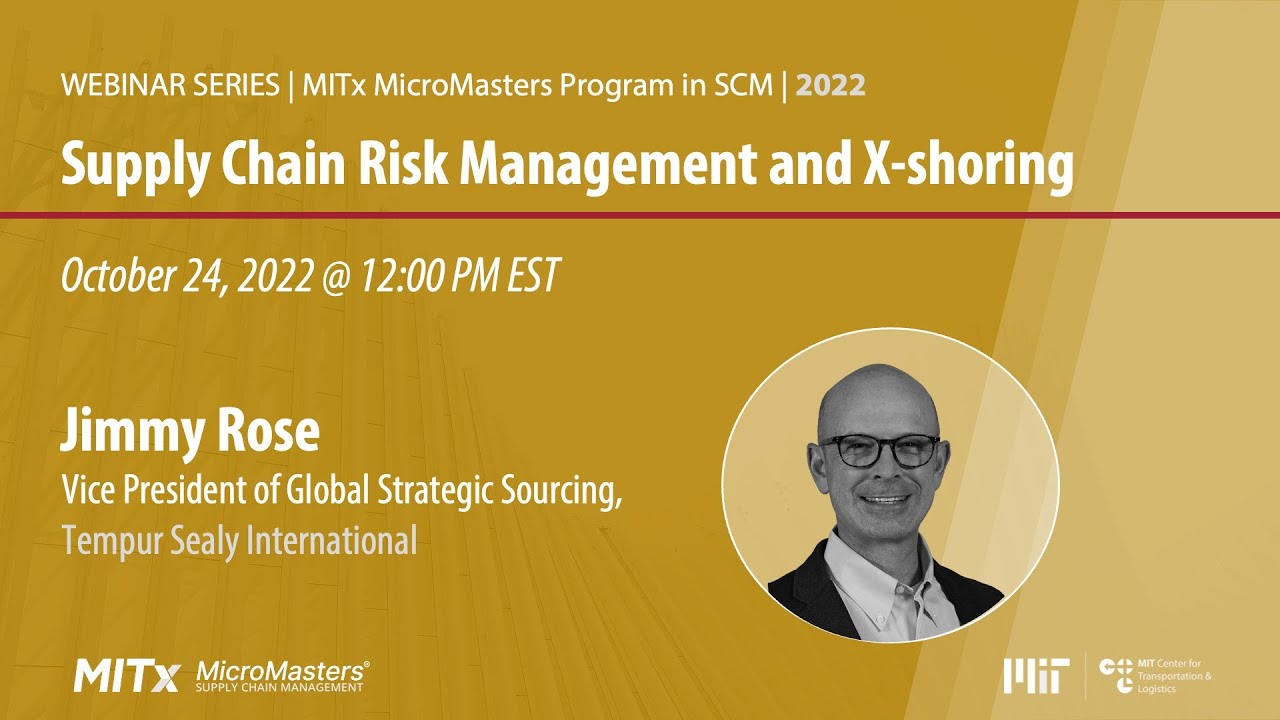
Supply Chain Risk Management and X shoring
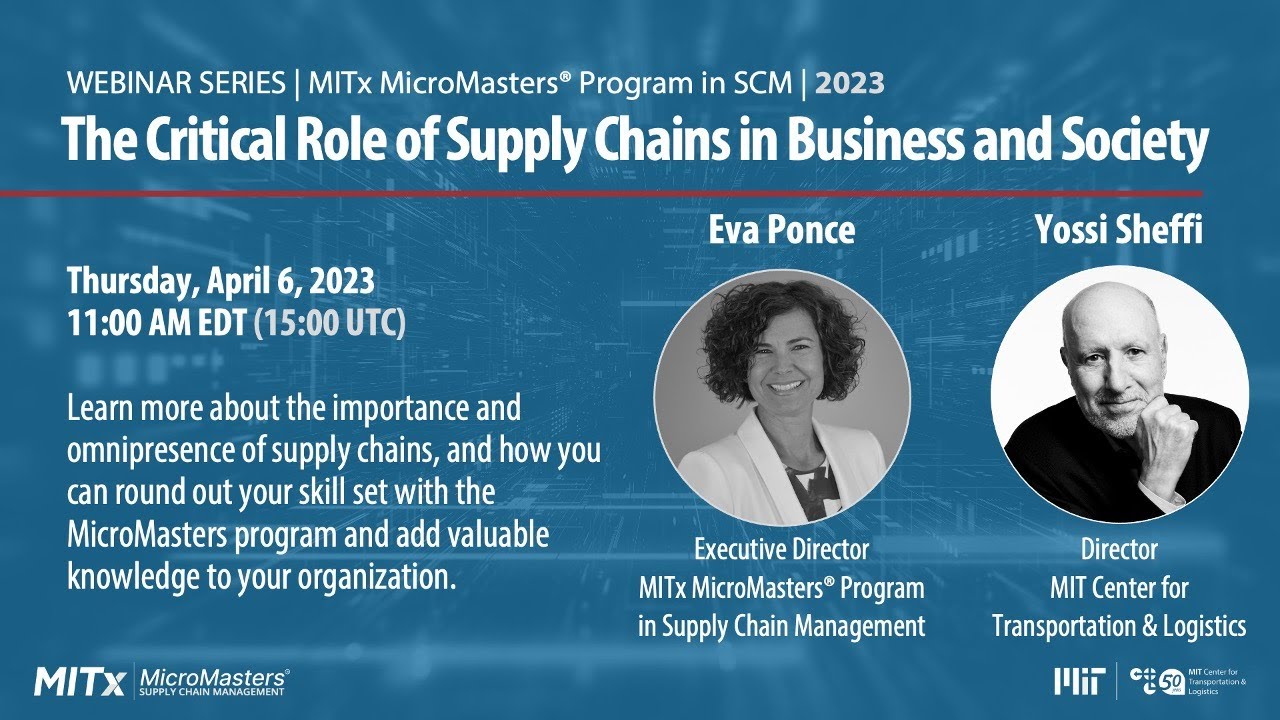
The Critical Role of Supply Chains in Business and Society
5.0 / 5 (0 votes)