Horizon 2023 | Ramesh Raskar | MIT Decentralized AI
Summary
TLDRDecentralized AI aims to shift from centralized systems controlled by large organizations to a more distributed model, where data is shared across independent entities. This approach enhances privacy, encourages collaboration, and fosters innovation. Key challenges include privacy-preserving machine learning, incentivizing participation, verifying trustworthy actors, and creating effective user interfaces. While decentralized AI has vast potential in sectors like healthcare and fintech, it also presents risks, such as malicious actors and coordination issues. Overcoming these challenges could enable decentralized AI to revolutionize industries and become a more ethical and sustainable alternative to centralized AI.
Takeaways
- 😀 Decentralized AI allows for AI systems to operate across independent, disjointed entities, unlike centralized AI where data and governance are controlled by large organizations.
- 😀 Centralized AI faces sustainability issues as data is often owned by individuals or organizations that are unwilling to share it, while decentralized AI enables collaboration without requiring full data sharing.
- 😀 Four main challenges of decentralized AI: 1) Privacy-preserving machine learning, 2) Incentivizing participation, 3) Verifying good actors, and 4) Creating user-friendly interfaces.
- 😀 Web3 technology, which is based on peer-to-peer and distributed frameworks, provides key building blocks for decentralized AI systems.
- 😀 Decentralized AI has the potential to democratize AI by creating a collective intelligence, much like human society, where individuals contribute to a greater whole.
- 😀 In healthcare, decentralized AI could have accelerated the global response to COVID-19 by enabling data-sharing between countries and healthcare systems early on.
- 😀 Decentralized AI enables personalized agents, or digital twins, that act on behalf of individuals or organizations, optimizing decision-making and collaboration across sectors.
- 😀 Risks of decentralized AI include rapid coordination between AI agents leading to unintended outcomes, misinformation, and adversarial behavior that could disrupt systems.
- 😀 Decentralized AI should initially be deployed in low-risk areas to test its effectiveness before expanding to more critical sectors like healthcare, finance, and energy.
- 😀 Localized AI systems for specific tasks can be aggregated to create a more powerful, collective intelligence, especially in sensitive areas like healthcare and finance where privacy is crucial.
- 😀 Decentralized AI is an emerging field with the potential to become a transformative technology, solving real-world problems in innovative ways and surpassing traditional AI models over time.
Q & A
What is the main difference between decentralized AI and centralized AI?
-Centralized AI involves large organizations controlling data, computing power, and governance, which is becoming unsustainable. Decentralized AI, on the other hand, enables collaboration across independent entities, allowing them to share partial information while keeping data distributed and private.
What are the four main problems that need to be solved for decentralized AI to work?
-The four main problems are: 1) privacy-preserving machine learning, 2) incentivizing disinterested entities to participate and share data, 3) verifying that entities are good actors, and 4) creating user-friendly dashboards to manage the decentralized AI system.
How does Web 3.0 contribute to the development of decentralized AI?
-Web 3.0 provides a peer-to-peer, distributed framework that can be used to build decentralized AI systems. Many of the building blocks of Web 3.0, such as decentralized networks and secure data protocols, can support the infrastructure needed for decentralized AI.
What are some potential applications of decentralized AI in the healthcare sector?
-In healthcare, decentralized AI could enable global collaboration between clinics and hospitals, sharing data and insights to improve the speed and accuracy of diagnoses. For instance, during a pandemic, decentralized AI could help share information about diseases, leading to faster responses and better outcomes.
What role do personalized AI agents play in decentralized AI?
-Personalized AI agents, or digital twins, work on behalf of individuals or organizations to collect data, make decisions, and perform simulations. These agents facilitate more efficient decision-making and provide a tailored approach to various sectors, such as healthcare or finance.
What are some of the risks associated with decentralized AI?
-Risks include the rapid coordination of AI systems, which could lead to unintended or adversarial outcomes. There’s also the concern of misinformation, manipulation, and security breaches. Therefore, decentralized AI should be deployed cautiously, starting in low-risk scenarios.
Why is decentralized AI particularly relevant in sectors like healthcare and finance?
-In healthcare and finance, data privacy is critical. Decentralized AI allows sensitive data to remain under the control of individuals or organizations, promoting collaboration while ensuring that privacy and security are maintained.
How could decentralized AI have helped in the COVID-19 pandemic?
-Decentralized AI could have facilitated the global sharing of health data and insights across countries and healthcare providers. By using this collective intelligence, the world could have responded more quickly to the pandemic and developed solutions for conditions like COVID-19 pneumonia.
What challenges need to be overcome to realize the full potential of decentralized AI?
-The key challenges include developing secure and private machine learning techniques, creating incentives for participation, ensuring good actor verification, and designing intuitive, user-friendly interfaces for managing decentralized systems.
What is the future outlook for decentralized AI?
-Decentralized AI has the potential to become more significant than current centralized AI, especially as it addresses real-world problems in sectors like healthcare and finance. The future of decentralized AI depends on advancing research, building cross-sector collaboration, and establishing secure and ethical frameworks for its implementation.
Outlines
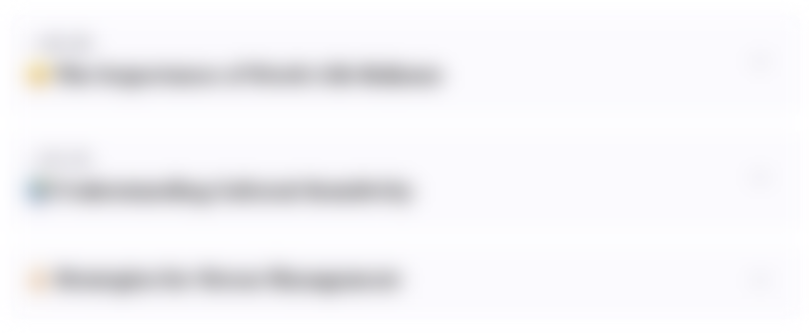
This section is available to paid users only. Please upgrade to access this part.
Upgrade NowMindmap
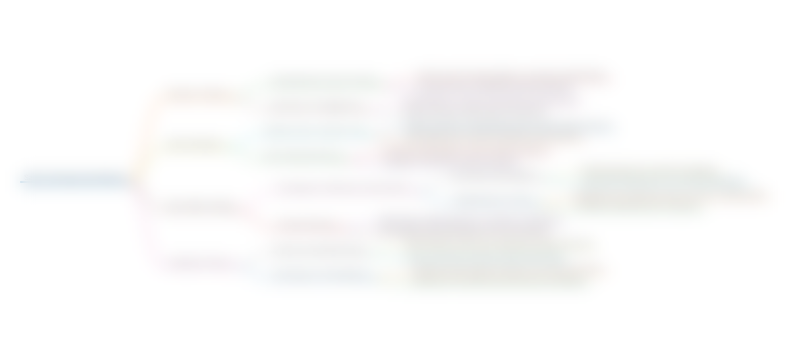
This section is available to paid users only. Please upgrade to access this part.
Upgrade NowKeywords
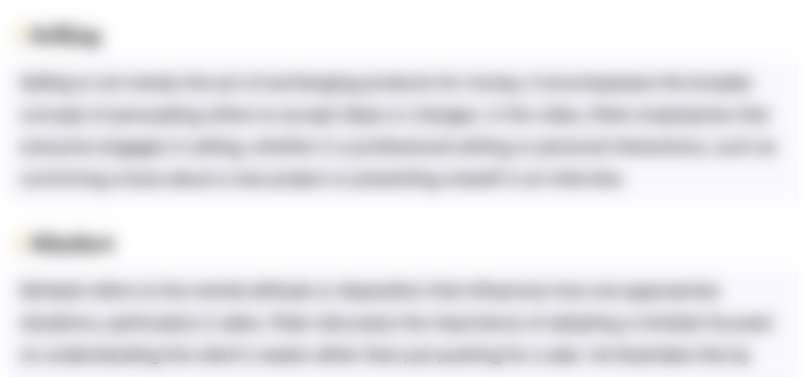
This section is available to paid users only. Please upgrade to access this part.
Upgrade NowHighlights
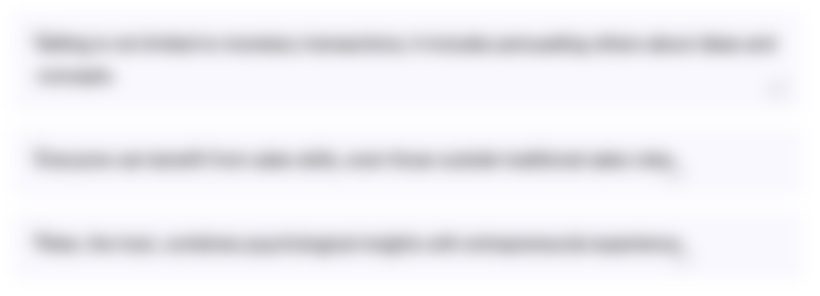
This section is available to paid users only. Please upgrade to access this part.
Upgrade NowTranscripts
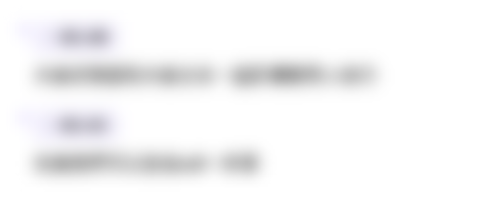
This section is available to paid users only. Please upgrade to access this part.
Upgrade NowBrowse More Related Video
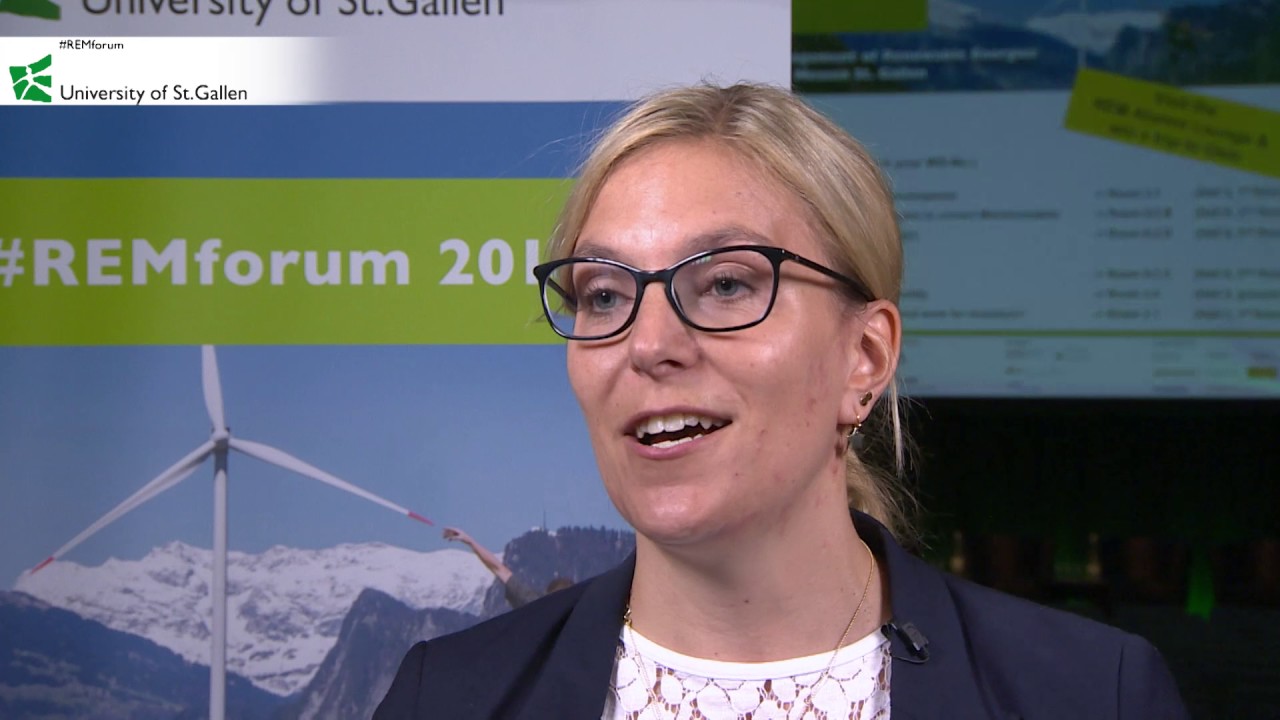
Sandra Trittin, Swisscom Energy Solutions, at #REMforum 2017
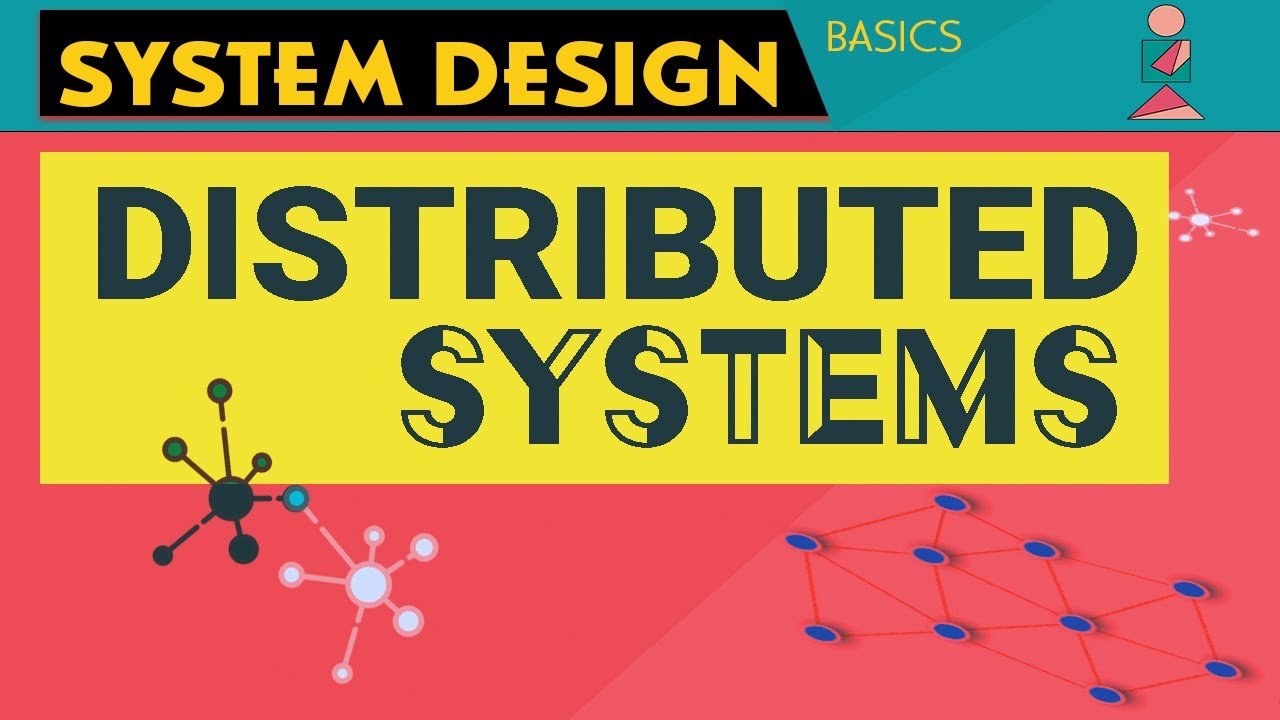
Distributed Systems Explained | System Design Interview Basics
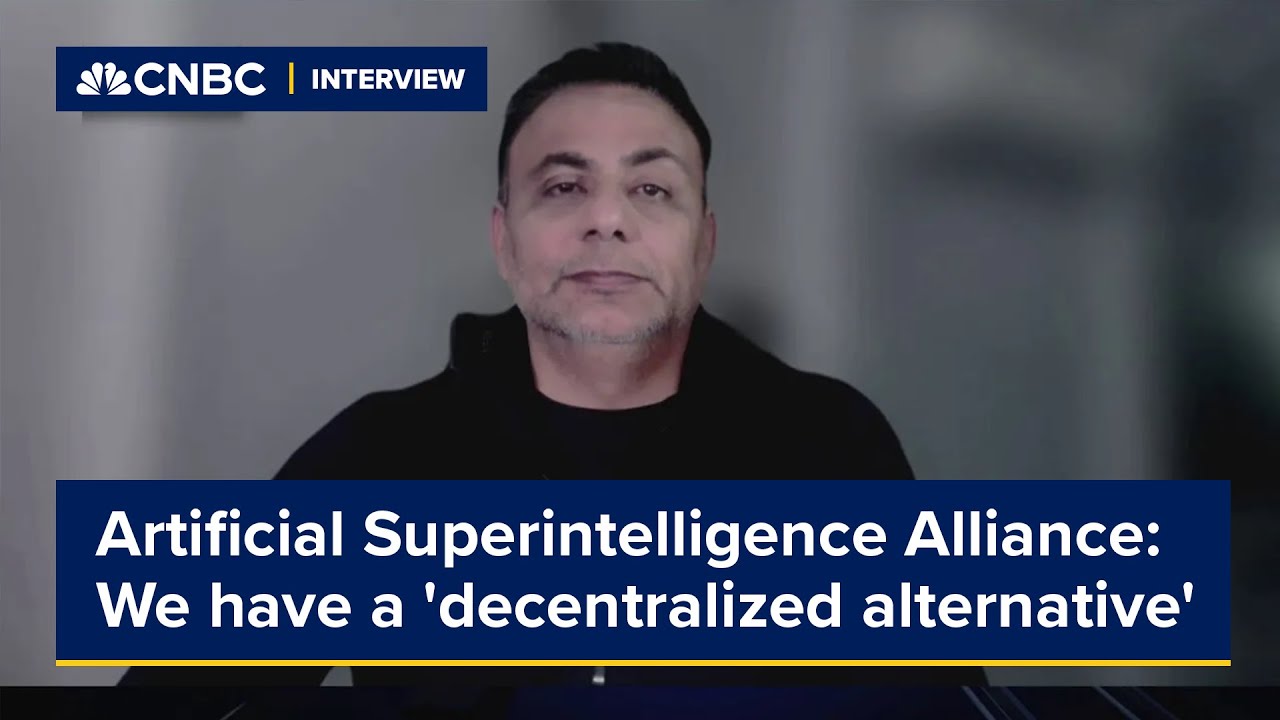
Artificial Superintelligence Alliance chairman: We have a 'decentralized alternative'
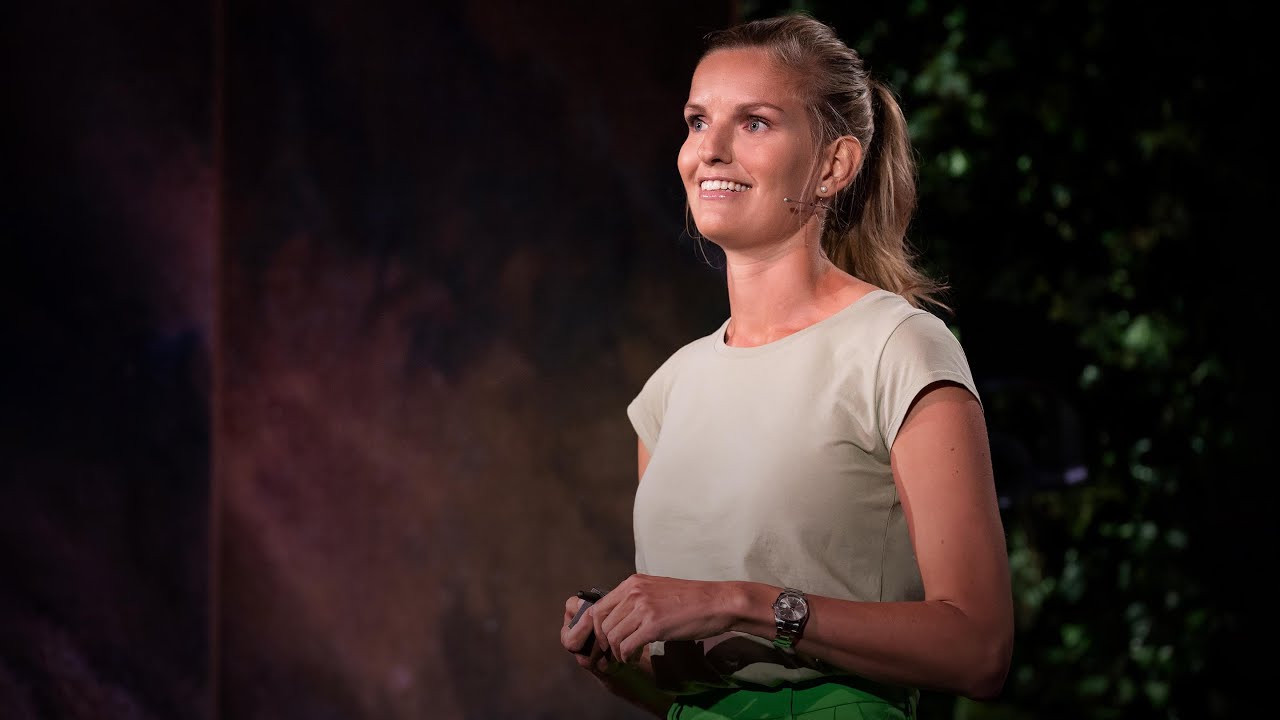
Great Leadership Is a Network, Not a Hierarchy | Gitte Frederiksen | TED
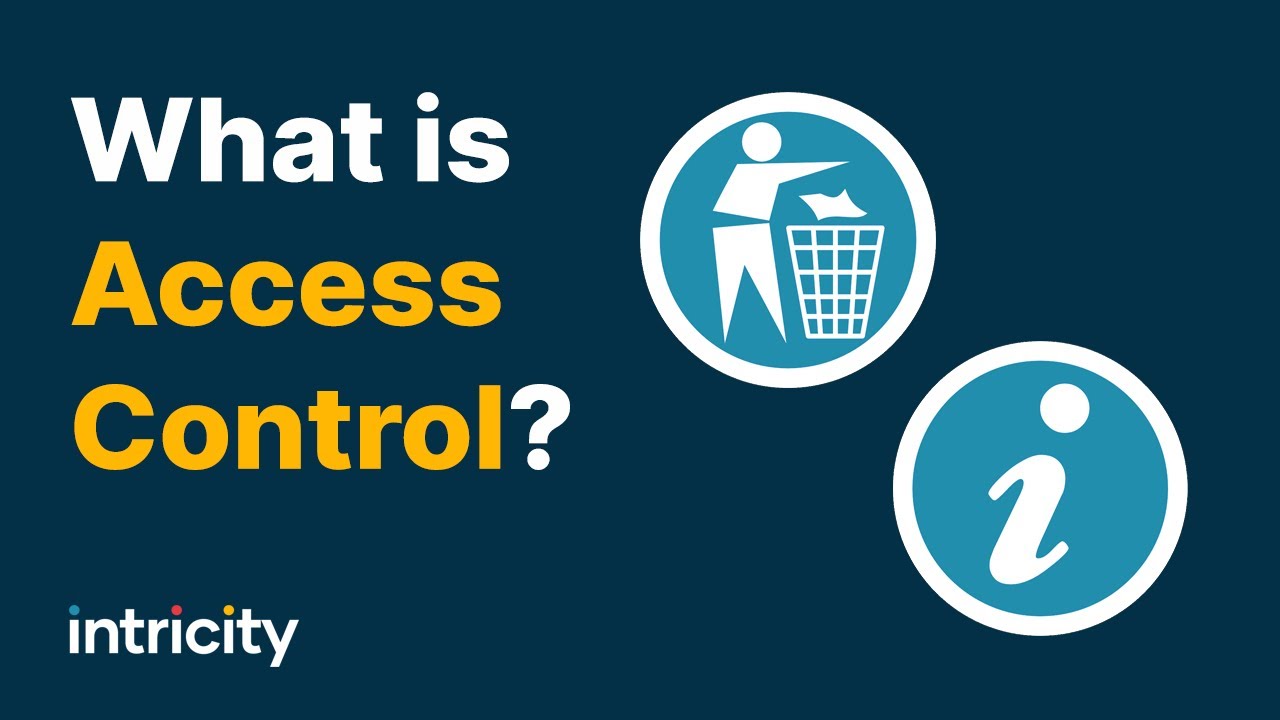
What is Access Control?
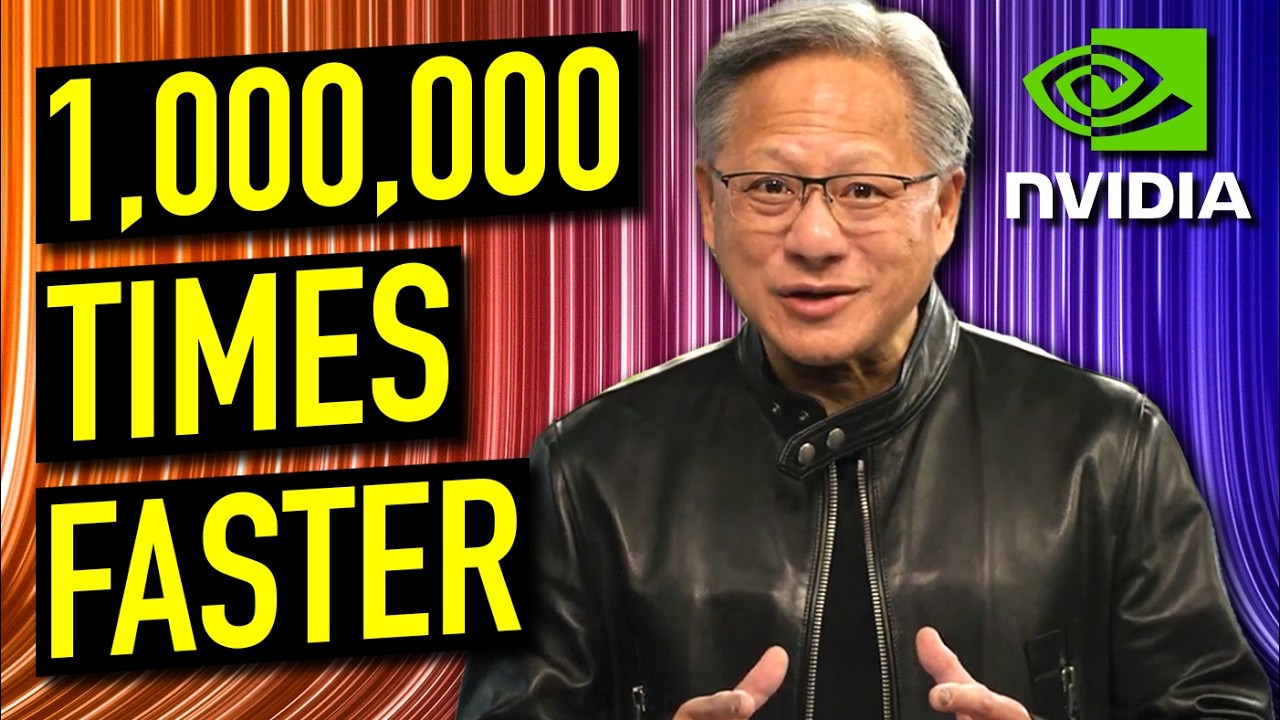
NVIDIA CEO Jensen Huang Leaves Everyone SPEECHLESS (Supercut)
5.0 / 5 (0 votes)