Goodness of Fit
Summary
TLDRThe chi-squared goodness of fit test is used to compare observed categorical data against a set of expected proportions. In this example, data from OkCupid users in San Francisco is compared to percentages from the General Social Survey regarding sexual orientation. The test investigates whether the observed distribution matches the expected distribution, with the null hypothesis assuming the proportions are the same. The process involves calculating expected counts, using sample sizes and hypothesized proportions, and testing the difference between observed and expected counts to determine statistical significance.
Takeaways
- 😀 The chi-squared goodness of fit test is used for categorical variables with more than two categories, helping to assess if observed data matches expected proportions.
- 😀 It is useful when testing if observed proportions align with a specific set of claimed values, such as survey results or known distributions.
- 😀 An example involves comparing sexual orientation data from the OkCupid website with data from the General Social Survey (GSS) in 2012.
- 😀 The chi-squared test compares the actual observed data against the expected proportions, based on known percentages, rather than comparing two separate sample sets.
- 😀 The null hypothesis of the test assumes that the observed proportions are equal to the claimed proportions from a survey or known distribution.
- 😀 In the example, the null hypothesis posits that the proportions of people identifying as gay/lesbian, bisexual, and straight match the GSS data from 2012.
- 😀 The alternative hypothesis suggests that at least one of the observed proportions differs from the expected proportions, indicating a mismatch between the two sets of data.
- 😀 The chi-squared test statistic is calculated by summing the squared differences between observed and expected values, divided by the expected values for each category.
- 😀 The expected values are computed using the formula: Expected Count = Total Sample Size × Hypothesized Proportion.
- 😀 In the example with 59,946 OkCupid users, the expected number of gay or lesbian individuals would be 959.14, calculated using the hypothesized proportion of 0.016.
- 😀 The chi-squared statistic for this test is calculated by applying the formula to all categories, leading to a final test statistic of 15,086.35 for this particular example.
Q & A
What is the chi-squared goodness of fit test used for?
-The chi-squared goodness of fit test is used to investigate whether the observed frequencies of a categorical variable match a set of expected frequencies, based on a specific hypothesis or distribution.
In the example from the General Social Survey, what percentages are given for sexual orientation in 2012?
-According to the General Social Survey in 2012, 1.6% of Americans identified as gay or lesbian, 2.5% identified as bisexual, and 95.9% identified as straight.
How does the chi-squared goodness of fit test differ from a chi-squared test of independence?
-The chi-squared goodness of fit test compares observed data to a theoretical distribution, whereas the chi-squared test of independence compares two categorical variables to see if they are independent of each other.
What is the null hypothesis for a chi-squared goodness of fit test in this example?
-The null hypothesis is that the long-run probabilities for each sexual orientation category (gay/lesbian, bisexual, and straight) in the OkCupid sample are equal to the percentages given by the General Social Survey (1.6%, 2.5%, and 95.9%, respectively).
What does the alternative hypothesis for this chi-squared test suggest?
-The alternative hypothesis suggests that at least one of the long-run probabilities for sexual orientation categories in the OkCupid sample differs from the claimed percentages in the General Social Survey.
How is the test statistic for a chi-squared goodness of fit test calculated?
-The test statistic is calculated by taking the observed count minus the expected count for each category, squaring the result, dividing by the expected count, and summing this value over all categories.
What is the formula for calculating the expected count in a chi-squared goodness of fit test?
-The expected count for each category is calculated as the sample size (n) multiplied by the hypothesized proportion (P) for that category under the null hypothesis: Expected Count = n * P.
How do we check if the expected counts are correct?
-To check the expected counts, we can ensure that the sum of all expected counts across categories adds up to the total sample size.
What were the expected counts for each category in the OkCupid sample, and how were they calculated?
-For the OkCupid sample, the expected counts were calculated by multiplying the sample size (59,946) by the respective proportions from the General Social Survey. For example, the expected number of gay or lesbian individuals was 59,946 * 0.016 = 959.14.
What was the test statistic value for this chi-squared goodness of fit test, and how is it interpreted?
-The test statistic for this chi-squared goodness of fit test was 15,086.35. This statistic is used to assess how well the observed data fits the expected data; a higher value suggests a greater discrepancy between observed and expected values, indicating that the null hypothesis may not be valid.
Outlines
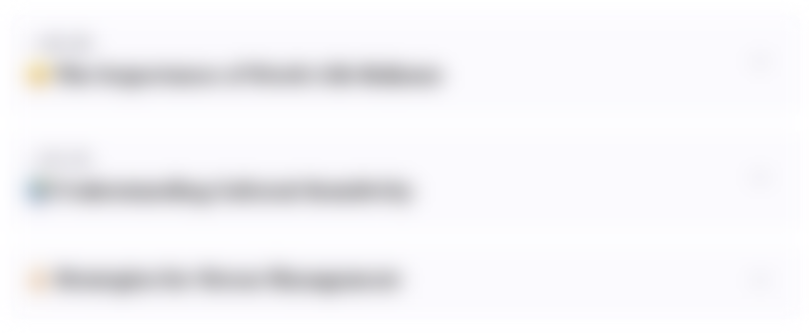
This section is available to paid users only. Please upgrade to access this part.
Upgrade NowMindmap
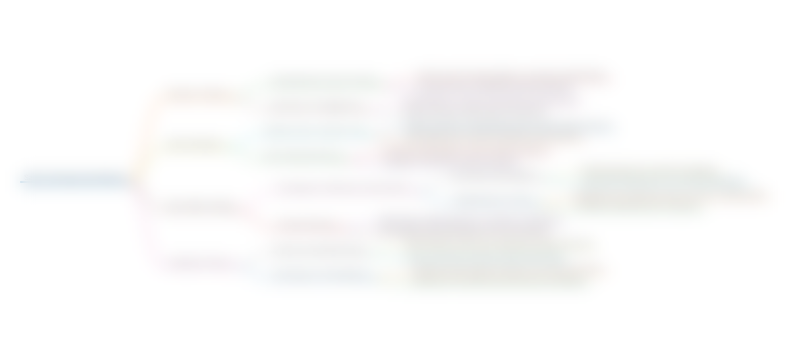
This section is available to paid users only. Please upgrade to access this part.
Upgrade NowKeywords
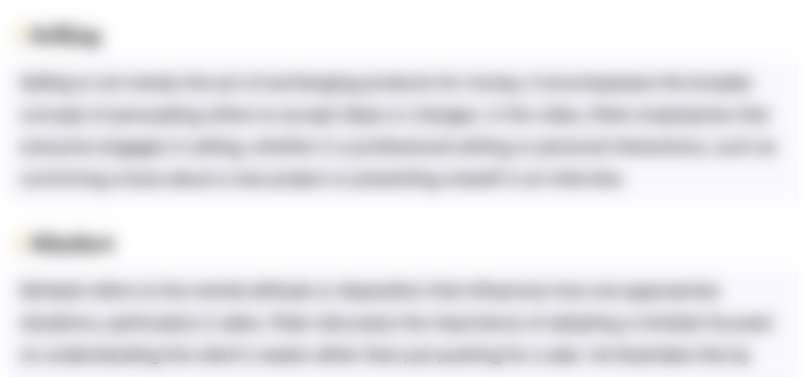
This section is available to paid users only. Please upgrade to access this part.
Upgrade NowHighlights
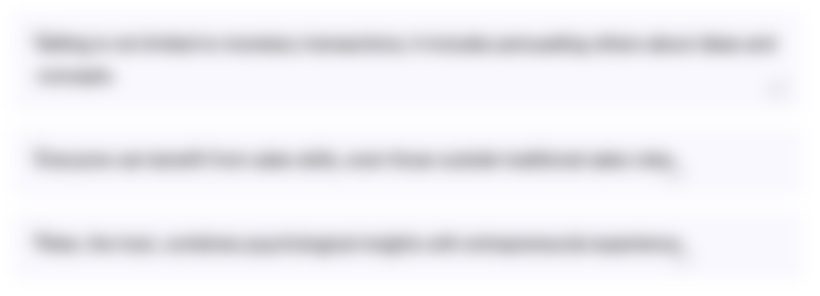
This section is available to paid users only. Please upgrade to access this part.
Upgrade NowTranscripts
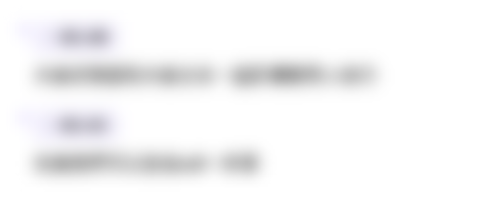
This section is available to paid users only. Please upgrade to access this part.
Upgrade NowBrowse More Related Video
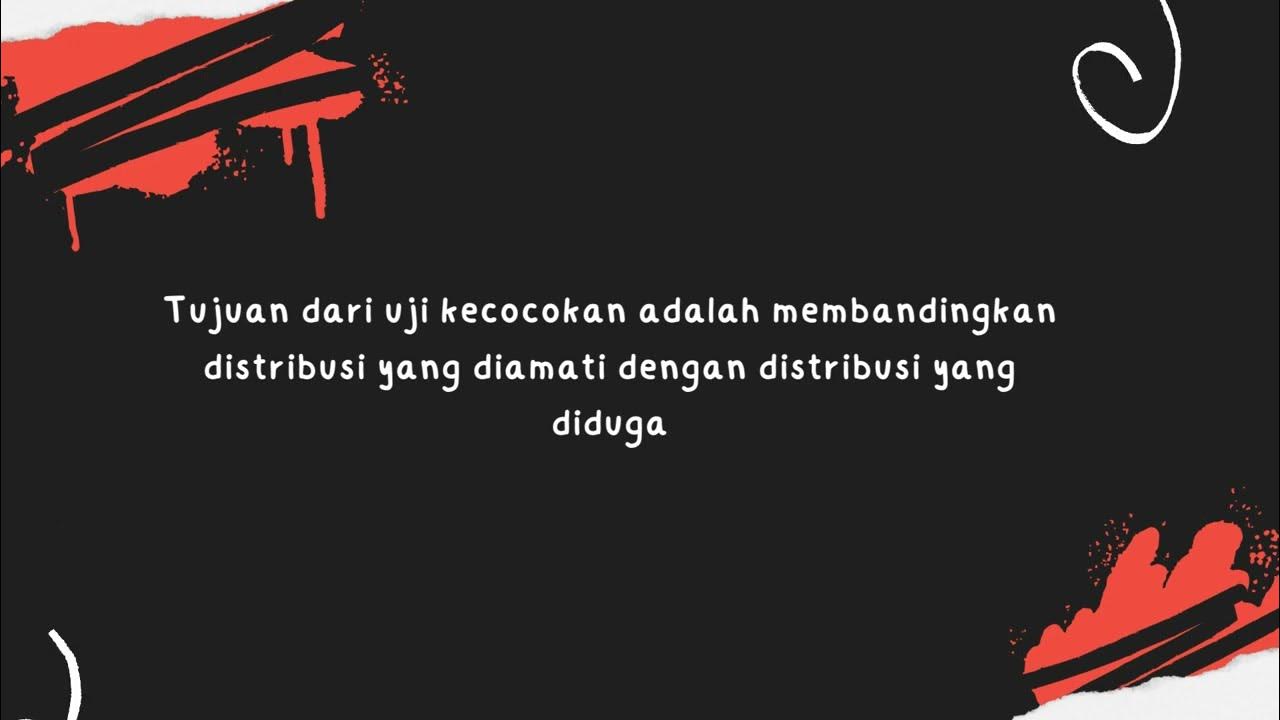
*M* Uji Kecocokan: Frekuensi yang Diduga Sama dan yang Tidak Sama dengan Microsoft Excel dan SPSS
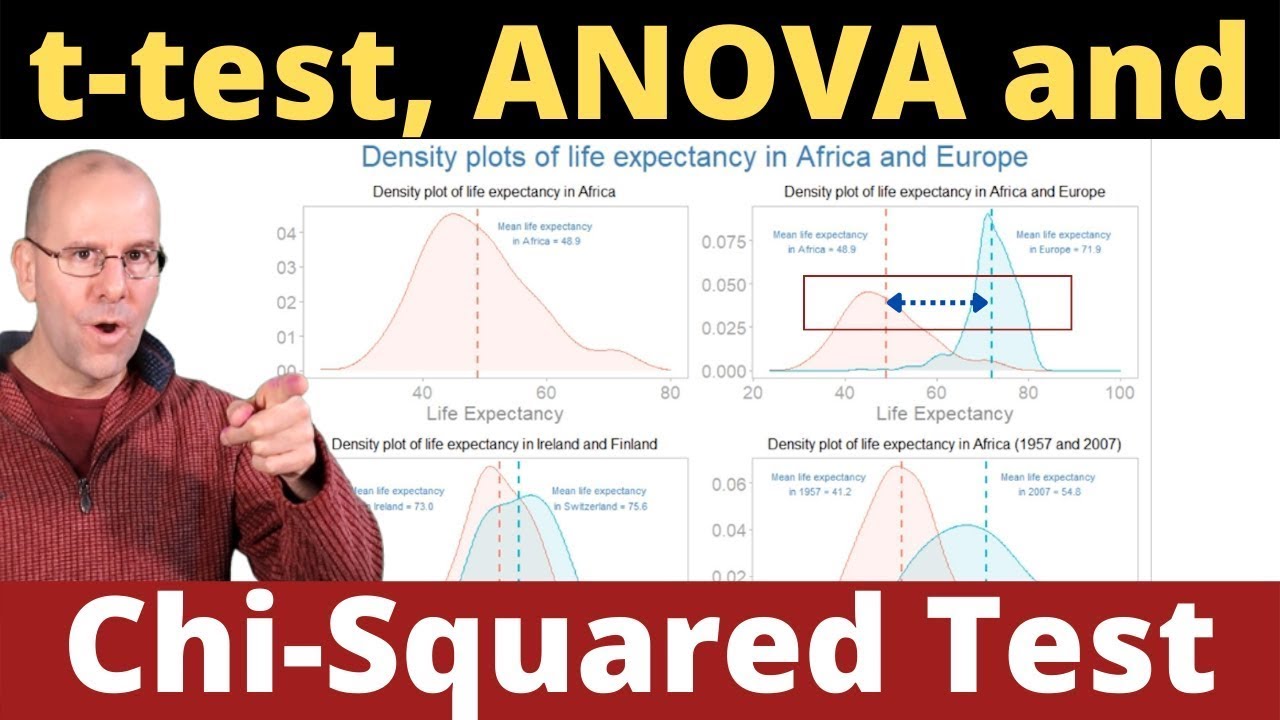
T-test, ANOVA and Chi Squared test made easy.
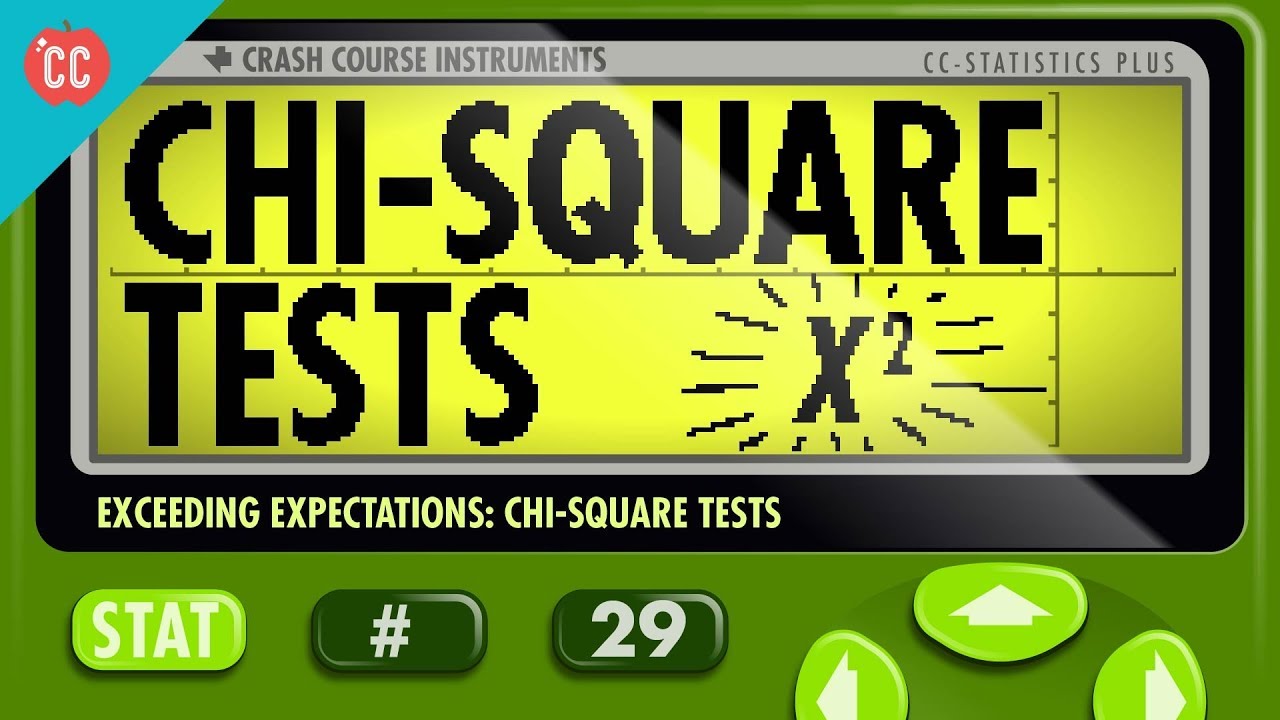
Chi-Square Tests: Crash Course Statistics #29

Chi Square in Genetics & Examples (AP Biology)
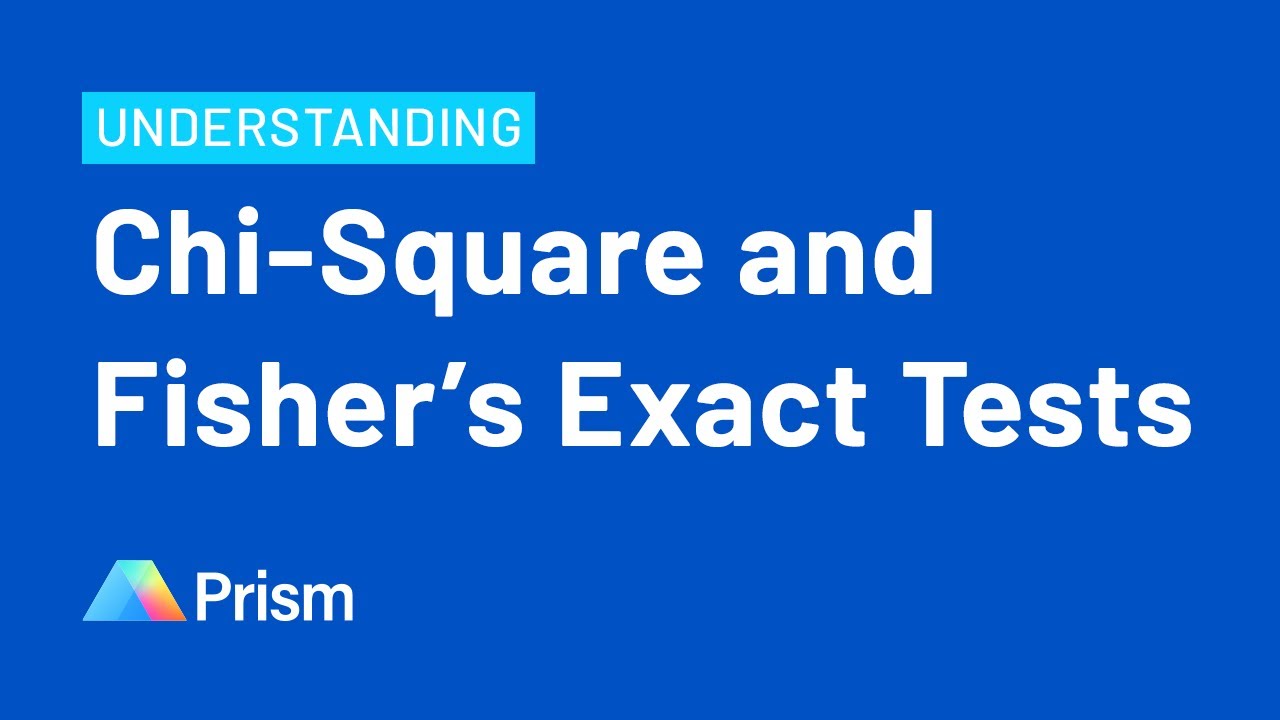
Understanding Chi-Square and Fisher's Exact Tests
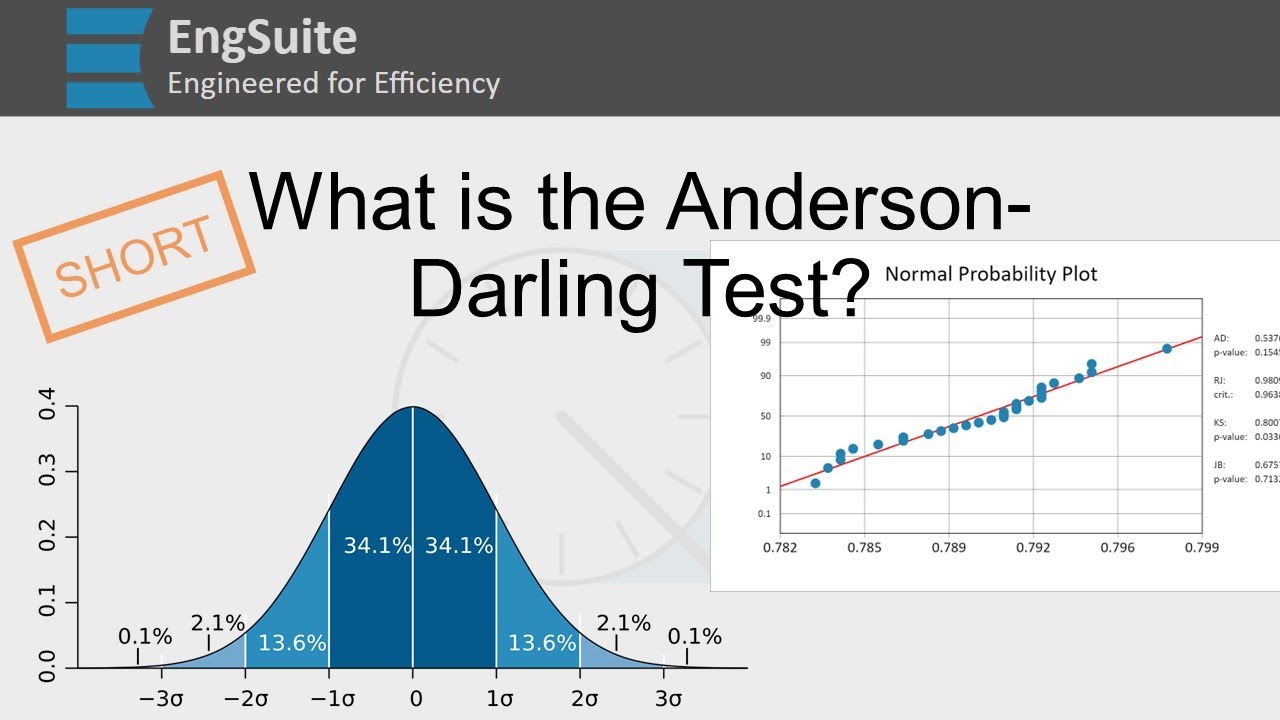
Introduction to the Anderson-Darling Test (short) - Engineering Statistics
5.0 / 5 (0 votes)